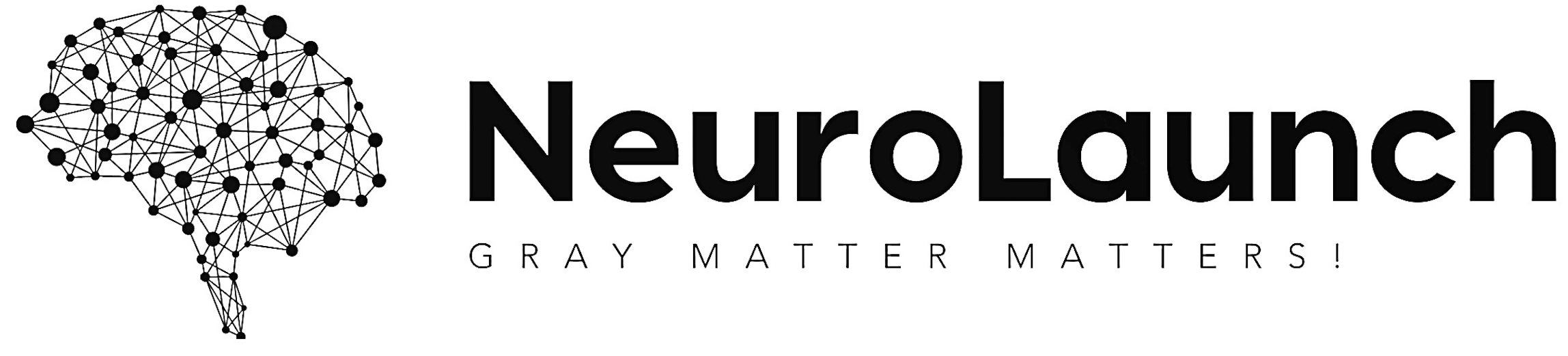

Travel Behavior: Exploring the Factors That Shape Our Journeys
The complex tapestry of factors that shape our travel decisions holds the key to unlocking insights into the ever-evolving world of tourism and transportation. As we embark on this journey to unravel the mysteries of travel behavior, we’ll discover a fascinating interplay of psychological, socioeconomic, environmental, and technological influences that guide our wanderlust.
Picture yourself standing at the crossroads of a bustling airport, surrounded by a sea of travelers from all walks of life. Each person carries a unique story, a personal motivation that has led them to this moment. Some are business professionals jetting off to close deals, while others are adventure-seekers ready to explore uncharted territories. Families reunite, couples embark on romantic getaways, and solo travelers set out to find themselves.
But what exactly drives these diverse travel choices? How do we decide where to go, when to go, and how to get there? The answer lies in the intricate web of factors that shape our travel behavior.
Travel behavior, in essence, encompasses the decisions and actions individuals take when planning and executing their journeys. It’s a complex dance of internal desires and external influences, a delicate balance between practicality and dreams. Understanding these patterns is crucial not only for the tourism and transportation industries but also for anyone seeking to decode the human psyche and our innate desire to explore.
The Psychology of Wanderlust: What Makes Us Want to Travel?
At the heart of travel behavior lies a potent cocktail of psychological factors that fuel our wanderlust. It’s a heady mix of motivation, personal goals, attitudes, and cultural influences that shape our travel decisions in ways we might not even realize.
Let’s start with motivation – the spark that ignites our desire to travel. For some, it’s the thrill of adventure, the need to break free from the mundane and experience something extraordinary. Others might be driven by a quest for knowledge, seeking to broaden their horizons and gain new perspectives. And then there are those who travel for relaxation, escaping the stresses of daily life to find peace in new surroundings.
Our attitudes and perceptions towards travel play a crucial role too. Some view travel as a luxury, a reward for hard work, while others see it as a necessary part of personal growth and education. These perceptions are often shaped by our upbringing, cultural background, and life experiences.
Speaking of cultural background, it’s fascinating to see how our values and beliefs influence our travel choices. For instance, some cultures place a high value on family vacations, while others might prioritize solo adventures or spiritual pilgrimages. These cultural nuances add rich layers to the tapestry of global travel behavior.
Risk tolerance is another intriguing factor. Are you the type who seeks out adrenaline-pumping activities in far-flung destinations, or do you prefer the comfort of familiar places? Your answer to this question can significantly impact your travel decisions. It’s worth noting that our risk tolerance often changes over time, influenced by life experiences and personal growth.
Money Talks: The Socioeconomic Factors Behind Our Travel Choices
While our hearts may yearn for exotic adventures, our wallets often have the final say in our travel decisions. The socioeconomic factors that shape our travel behavior are as diverse as they are influential.
Income and disposable wealth are, unsurprisingly, major players in this arena. The amount of money we can allocate to travel directly impacts the frequency, duration, and luxury level of our trips. However, it’s not just about how much money we have, but also how we choose to spend it. Some might scrimp and save for a once-in-a-lifetime luxury vacation, while others prefer frequent budget-friendly getaways.
Education level and career choices also play a significant role in shaping our travel behavior. Higher education often correlates with a greater desire for cultural experiences and international travel. Moreover, certain careers, such as those in international business or academia, may necessitate frequent travel, shaping one’s attitudes and habits over time.
Family structure and lifecycle stage are other crucial factors to consider. A young couple without children might have different travel preferences compared to a family with school-age kids or empty nesters. These life stages often dictate not only where we travel but also when and how we do it.
Let’s not forget the power of social networks and peer influence. In today’s interconnected world, our travel choices are increasingly shaped by the experiences and recommendations of our friends, family, and even social media influencers. The family influence on buying behavior extends to travel decisions as well, often shaping our preferences from a young age.
The World Around Us: Environmental and Situational Influences
As much as our internal factors drive our travel behavior, the external world plays an equally important role. The allure of specific destinations, the whims of weather, and the realities of global politics all contribute to the complex equation of travel decision-making.
Destination attributes and attractions are often the primary draw for travelers. The promise of pristine beaches, historic landmarks, or vibrant cultural scenes can be irresistible. But it’s not just about what a place has to offer – it’s also about how these offerings align with our personal interests and goals.
Climate and seasonality wield significant influence over our travel choices. The appeal of a sun-soaked beach vacation during a dreary winter or the allure of snow-capped mountains for winter sports enthusiasts demonstrates how weather patterns shape our travel behavior. Seasonal events, like cherry blossom viewing in Japan or the Northern Lights in Scandinavia, can also drive travel decisions.
In an ever-changing world, political stability and safety concerns have become increasingly important factors in travel behavior. Travelers are more conscious than ever about the security situation in potential destinations, often adjusting their plans based on current events and travel advisories.
Transportation infrastructure and accessibility can make or break a destination’s appeal. The ease of getting to and around a place significantly impacts travel decisions. This is where passenger behavior comes into play, as the quality of transportation experiences can shape future travel choices.
The Digital Nomad’s Compass: Technology’s Impact on Modern Travel
In the age of smartphones and high-speed internet, technology has revolutionized the way we plan, book, and experience travel. The digital landscape has become an integral part of modern travel behavior, influencing our decisions in ways that were unimaginable just a few decades ago.
Social media and online reviews have become the new word-of-mouth, shaping our perceptions of destinations and accommodations. A stunning Instagram photo or a glowing TripAdvisor review can spark wanderlust and influence travel choices. This digital age has transformed how we engage in search behavior , with travelers often spending hours researching and comparing options online before making decisions.
Mobile apps and digital travel planning tools have put the power of a travel agent in our pockets. From flight booking to itinerary planning, these tools have made travel more accessible and customizable than ever before. They’ve also changed how we behave during our trips, with real-time navigation, translation, and recommendation apps enhancing our experiences on the go.
Virtual and augmented reality are adding new dimensions to travel experiences. Virtual tours can help travelers preview destinations and make informed decisions, while augmented reality apps can enhance real-world experiences by providing interactive information about attractions and landmarks.
The rise of the sharing economy has significantly impacted accommodation choices. Platforms like Airbnb have transformed how travelers think about lodging, offering unique, local experiences that challenge traditional hotel stays. This shift has not only affected travel behavior but also local economies and urban dynamics in popular destinations.
The Changing Face of Travel: Emerging Trends and Future Directions
As we look to the horizon, several emerging trends are reshaping travel behavior in exciting and meaningful ways. These shifts reflect changing values, technological advancements, and a growing awareness of our impact on the world.
Sustainable and eco-friendly travel choices are gaining momentum as travelers become more conscious of their environmental footprint. This trend is influencing everything from transportation choices to accommodation preferences, with many seeking out eco-certified options. The rise of sustainable behavior is not just a passing fad but a fundamental shift in how we approach travel.
Experiential and transformative travel is another trend that’s changing the landscape. More travelers are seeking authentic, immersive experiences that go beyond traditional sightseeing. Whether it’s learning a new skill, volunteering, or engaging in cultural exchanges, the focus is on personal growth and meaningful connections.
The lines between business and leisure travel are blurring with the rise of “bleisure” travel. Business travelers are increasingly extending their trips to include leisure activities, while remote work opportunities are allowing people to combine work and travel in new ways. This trend is reshaping how we think about work-life balance and travel.
Solo travel, particularly among women, is on the rise. This trend reflects changing social norms and a growing desire for independence and self-discovery through travel. It’s also driving changes in the travel industry, with more services and accommodations catering to solo travelers.
As we wrap up our exploration of travel behavior, it’s clear that the factors influencing our journeys are as diverse as the destinations we visit. From the psychological motivations that spark our wanderlust to the socioeconomic realities that shape our choices, from the environmental factors that guide our decisions to the technological tools that facilitate our adventures – travel behavior is a complex and fascinating field of study.
Understanding these patterns is crucial not just for the tourism and transportation industries but for anyone seeking to comprehend human behavior and decision-making processes. As traveler behavior continues to evolve, so too will the strategies employed by businesses and destinations to attract and cater to visitors.
Looking ahead, the future of travel behavior research promises to be as exciting as the journeys we undertake. Advances in data analytics, artificial intelligence, and behavioral sciences will likely provide even deeper insights into why we travel the way we do. As our world becomes increasingly interconnected, understanding these patterns will be key to creating sustainable, enjoyable, and meaningful travel experiences for all.
So, the next time you find yourself planning a trip or boarding a plane, take a moment to reflect on the myriad factors influencing your choices. Your journey is not just a physical movement from one place to another – it’s a complex interplay of psychology, economics, environment, and technology. And in understanding this interplay, we unlock the secrets to not just better travel experiences, but a deeper understanding of ourselves and our place in this vast, beautiful world.
References:
1. Pearce, P. L. (2005). Tourist behaviour: Themes and conceptual schemes. Channel View Publications.
2. Cohen, S. A., Prayag, G., & Moital, M. (2014). Consumer behaviour in tourism: Concepts, influences and opportunities. Current Issues in Tourism, 17(10), 872-909.
3. Gössling, S., Scott, D., & Hall, C. M. (2020). Pandemics, tourism and global change: a rapid assessment of COVID-19. Journal of Sustainable Tourism, 29(1), 1-20.
4. Xiang, Z., & Gretzel, U. (2010). Role of social media in online travel information search. Tourism Management, 31(2), 179-188.
5. Buhalis, D., & Law, R. (2008). Progress in information technology and tourism management: 20 years on and 10 years after the Internet—The state of eTourism research. Tourism Management, 29(4), 609-623.
6. Tussyadiah, I. P., & Pesonen, J. (2016). Impacts of peer-to-peer accommodation use on travel patterns. Journal of Travel Research, 55(8), 1022-1040.
7. Dolnicar, S. (2020). Designing for more environmentally friendly tourism. Annals of Tourism Research, 84, 102933.
8. Urry, J. (2002). The tourist gaze. Sage Publications.
9. McKercher, B., & Chen, F. (2015). Travel as a life priority? Asia Pacific Journal of Tourism Research, 20(7), 715-729.
10. World Tourism Organization (UNWTO). (2021). International Tourism Highlights, 2020 Edition. UNWTO, Madrid. https://www.e-unwto.org/doi/book/10.18111/9789284422456
Similar Posts
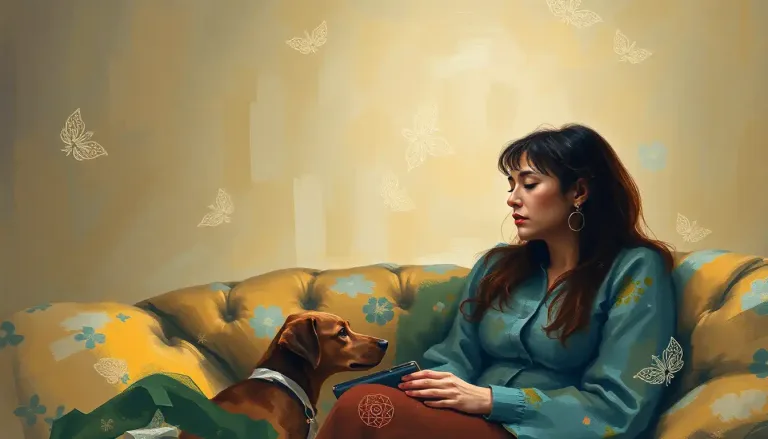
Textual Behavior: Decoding Digital Communication Patterns
From emojis to punctuation patterns, our digital texts speak volumes about who we are, revealing a fascinating tapestry of age, culture, and personality in the words we type. In this age of instant messaging and social media, our fingers have become the paintbrushes of our digital personas, crafting intricate portraits of ourselves with every keystroke….
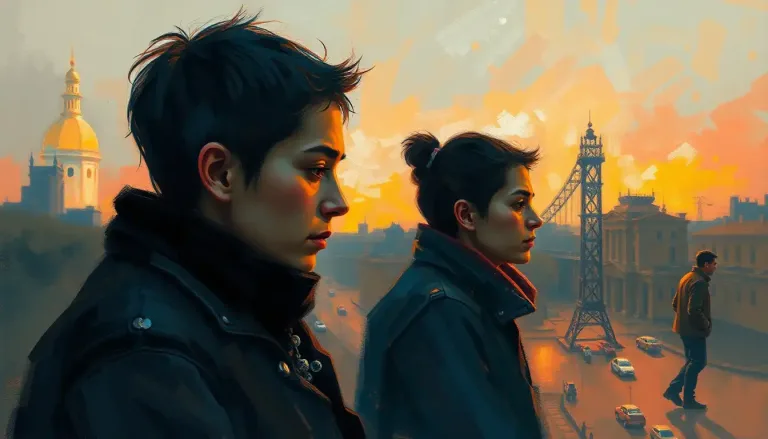
Subversive Behavior: Exploring Its Forms, Causes, and Impacts on Society
From whispered dissent to revolutionary fervor, subversive behavior has the power to reshape the very fabric of society, challenging the status quo and igniting the flames of change. It’s a force that has toppled empires, sparked cultural revolutions, and sent ripples through the annals of history. But what exactly is subversive behavior, and why does…
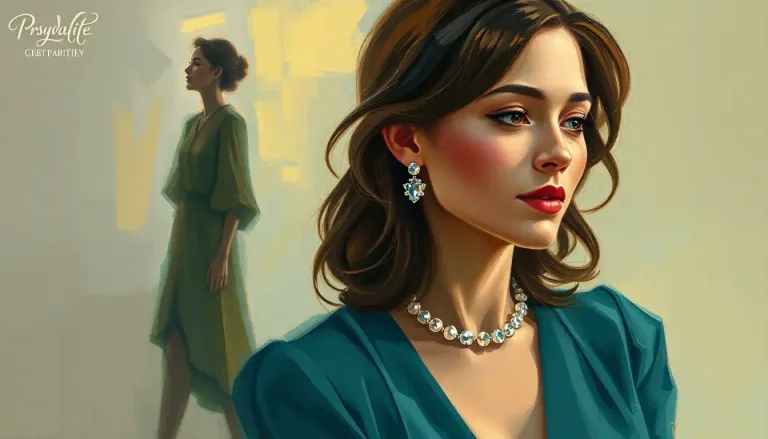
Classy Behavior: Mastering Elegance and Grace in Modern Society
Elegance and grace, once the hallmarks of a bygone era, have become increasingly rare commodities in our fast-paced, modern society—yet their value has never been greater. In a world where instant gratification and casual interactions dominate, the art of classy behavior stands out like a beacon of refinement. But what exactly does it mean to…
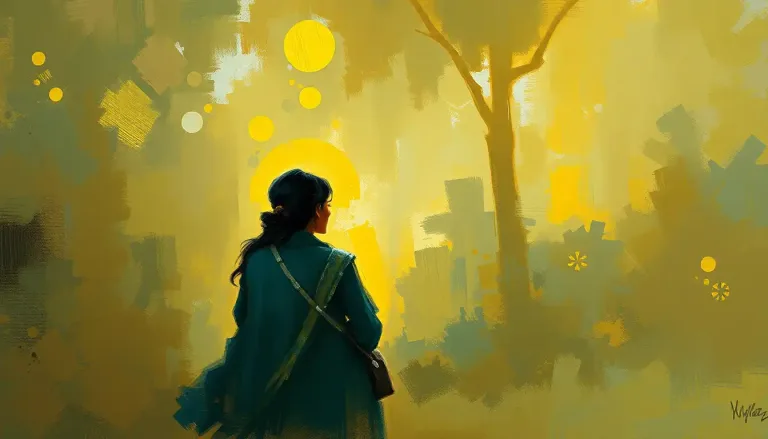
Manish Behavior: Exploring Its Meaning, Origins, and Cultural Implications
A peculiar term with a history as intriguing as its implications, “manish behavior” has long been a subject of fascination, confusion, and controversy in our society. It’s a phrase that conjures up images of women adopting traditionally masculine traits, but is that really what it means? Let’s dive into this complex topic and unravel the…
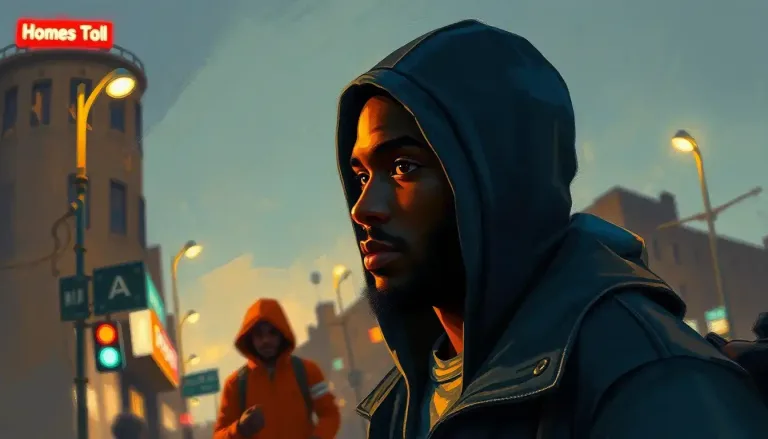
Hood Behavior: Unraveling the Complex Dynamics of Urban Street Culture
From the gritty streets to the hallowed halls of academia, the complex tapestry of hood behavior has long been a subject of fascination, fear, and misunderstanding. It’s a world that’s often misrepresented, misinterpreted, and misunderstood by those looking in from the outside. But what exactly is “hood behavior,” and why does it matter? Let’s start…
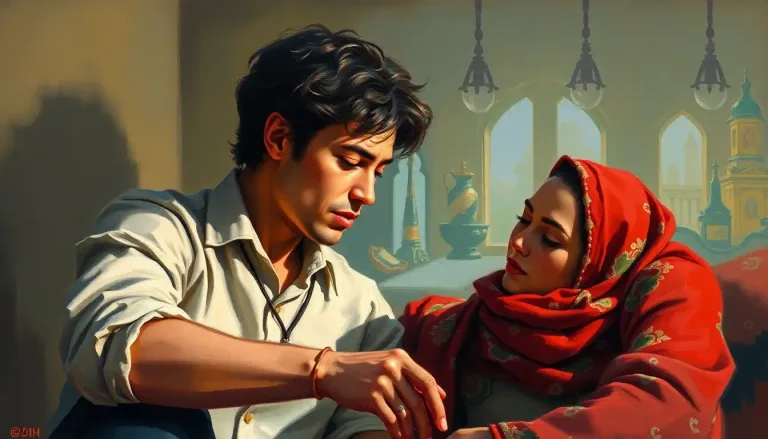
Satire’s Impact on Behavior Change: Exploring Its Powerful Influence
From biting political jabs to poignant social critiques, satire has long been a catalyst for change, wielding the power to reshape attitudes, challenge norms, and inspire action in ways that traditional discourse often fails to achieve. It’s a peculiar beast, this thing we call satire. One moment, it’s making us chuckle at the absurdity of…
Leave a Reply Cancel reply
Your email address will not be published. Required fields are marked *
Save my name, email, and website in this browser for the next time I comment.
Towards a Comprehensive Conceptual Framework of Active Travel Behavior: a Review and Synthesis of Published Frameworks
- Built Environment and Health (MJ Nieuwenhuijsen and AJ de Nazelle, Section Editors)
- Open access
- Published: 13 July 2017
- Volume 4 , pages 286–295, ( 2017 )
Cite this article
You have full access to this open access article
- Thomas Götschi 1 ,
- Audrey de Nazelle 2 ,
- Christian Brand 3 &
- Regine Gerike 4
on behalf of the PASTA Consortium
10k Accesses
90 Citations
37 Altmetric
Explore all metrics
Purpose of Review
This paper reviews the use of conceptual frameworks in research on active travel, such as walking and cycling. Generic framework features and a wide range of contents are identified and synthesized into a comprehensive framework of active travel behavior, as part of the Physical Activity through Sustainable Transport Approaches project (PASTA). PASTA is a European multinational, interdisciplinary research project on active travel and health.
Recent Findings
Along with an exponential growth in active travel research, a growing number of conceptual frameworks has been published since the early 2000s. Earlier frameworks are simpler and emphasize the distinction of environmental vs. individual factors, while more recently several studies have integrated travel behavior theories more thoroughly.
Based on the reviewed frameworks and various behavioral theories, we propose the comprehensive PASTA conceptual framework of active travel behavior. We discuss how it can guide future research, such as data collection, data analysis, and modeling of active travel behavior, and present some examples from the PASTA project.
Similar content being viewed by others
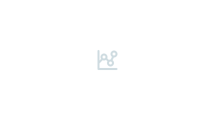
Policies to Promote Active Travel: Evidence from Reviews of the Literature
Built environment interventions to increase active travel: a critical review and discussion.
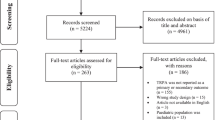
Correlates and determinants of transport-related physical activity among adults: an interdisciplinary systematic review
Avoid common mistakes on your manuscript.
Introduction
Sustainable transport modes, and in particular walking and cycling, have gained growing interest by decision makers, planners, and the general public as potential solutions to challenges rooted in urban transport, including environmental, economic, and health issues [ 1 , 2 ]. This trend is also reflected in an exponentially growing body of research addressing a wide range of aspects of active travel, including identifying and quantifying determinants of active travel behavior [ 3 ], assessing the effectiveness and sustainability of measures to promote it [ 4 , 5 , 6 ], understanding and remedying safety related issues [ 7 ], developing methods to measure or survey active travel [ 8 ], and assessing effects and impacts of active travel on travel, health, and environmental outcomes through various pathways [ 9 , 10 , 11 ].
Studies from various fields, such as transport and health, have repeatedly identified and confirmed the role of specific determinants of walking and cycling and regularly presented quantitative effect estimates [ 12 ]. However, most studies concentrate on a particular domain of influence, such as the policy context, the built environment, the social environment, or personal and trip attributes. Often, they are also limited by their specific topical perspectives, such as transport issues or public health. “Transport studies” tend to ignore health both as a motivation and as an outcome of active travel, while “health studies” often ignore the role of competing modes of transport. While taken together the whole body of knowledge does paint a fairly extensive qualitative picture of determinants of active travel, it remains challenging to build robust comprehensive models combining quantitative estimates. Namely, coefficients derived from different studies are not adjusted for each other, they may be based on different scales and definitions, and they tend to stem from different contexts and populations. A more holistic quantitative understanding of determinants of active travel, however, would help answer some of the most practice-relevant questions. In particular, it would provide more robust evidence to identify effective measures and policies and rationalize how these are prioritized. One possible application would be to better integrate walking and cycling into travel demand models, which to date tend to represent active travel poorly [ 13 , 14 ].
To develop a more holistic quantitative understanding of determinants of active travel and how it could be promoted, larger more comprehensive studies with a broad scope need to be conducted. We argue that for such endeavors to succeed, it is crucial to acquire the best possible conceptual understanding of the relationships between relevant determinants and active travel behavior and potential confounders and mediators a priori.
This paper thus aims to (1) review the use of conceptual frameworks in active travel research, and, based on these frameworks, (2) to propose a comprehensive framework that covers the abovementioned domains and can guide future research to observe, explain, and model active travel behavior. We aim to first systematically identify and describe key features of conceptual frameworks for active travel and then apply these in a novel, systematic and comprehensive framework developed within the scope of the Physical Activity through Sustainable Transport Approaches (PASTA) project [ 15 •], a multinational, interdisciplinary research project on active travel and health. The so-called PASTA framework was initially developed and used to determine contents of the longitudinal PASTA survey, a broad data collection effort about active travel and physical activity, their determinants, and associated crash risks [ 16 ]. While a visualization of such a framework could hardly be comprehensive with regards to topical scope, level of detail, or methodological issues for all active travel related research, we aim to combine as many concepts as possible identified by previous work; we aim to do this systematically; and we aim to provide generalizable guidance on how to be more systematic in developing frameworks for active travel related research. In the final section, we discuss the value of such efforts and provide examples of how the PASTA framework can guide specific research efforts.
Literature Review
A systematic effort was taken to comprehensively identify conceptual frameworks for active travel published in the scientific literature. However, despite clearly defined search terms, multiple steps to identify relevant publications, and systematic summaries of identified publications, this review remains exploratory, because “conceptual framework” is a loosely defined term and presumably some researchers may use a framework as a working tool without presenting or referring to it in publications or specifying which theories they are built on.
Systematic literature database searches of PubMed and the Transport Research International Documentation (TRID) database were complemented with systematic scans of references listed in publications identified as relevant (see Appendix 1 for search terms and hits.)
Identified hits were inspected with regards to relevance for the objectives of this review using a standardized categorization form filled out by at least two independent reviewers for each study. The form captured the scope of the reviewed publications, the purpose of the framework, the audience it was aimed for, its novelty, underlying theories, and area of research. Particular focus was put on unique or novel concepts and visual framework features that could contribute towards a more comprehensive framework.
The Results section describes the range of frameworks deemed within scope of the review and presents an overview (Table 1 ) and summaries of the most relevant publications.
Synthesis of Reviewed Frameworks and Development of the PASTA Conceptual Framework for Active Travel
Based on the identified conceptual frameworks of active travel, the study of behavioral theories, and our understanding of key issues of active travel behavior, we developed a more comprehensive conceptual framework for active travel behavior. The term comprehensive reflects our intention to integrate research-relevant aspects of active travel including various topical domains and structural features in a compact and well-balanced way. To facilitate readability, we present a simplified version in the main text and refer to Appendix 3 for a detailed version (for additional versions, see the supplemental materials available in https://www.researchgate.net/profile/Thomas_Goetschi/publications ).
Specifically, the framework aims to address aspects of relevance to the PASTA study and presumably other research projects, namely, guidance to identify data needs, data sources, and collection methods; data hierarchies, such as clusters or levels of aggregation; the integration of transport and health perspectives with a focus on active travel; and importance of context in measuring and evaluating active travel interventions. Finally, the framework allows testing elements of established theories and latest thinking in (active) travel behavior research.
Overview of the Identified Frameworks
The literature search yielded over 200 hits in PubMed and TRID. After scanning abstract and titles for relevance, reviewing references, and adding some publications identified previously, 65 publications were kept for further review. Appendix 1 provides an overview of the selection process.
The main scope of two thirds of these publications was on active travel behavior [ 48/65 ], while about one third also focused on physical activity [ 23/65 ] or safety [ 8/65 ]. In terms of audience, the publications appeared to target about equally transport researcher, health researchers, and planners, whereas health professionals, policy makers, and advocates seemed somewhat less represented.
About two thirds of the identified publications were considered to provide and discuss conceptual frameworks [ 43/65 ], in the sense of aiming to illustrate causal, temporal, spatial, or hierarchical relationships between active travel outcomes and factors that explain these. Around 10 to 15 papers instead presented frameworks for planning, data collection, modeling, designing interventions, or conducting evaluations.
About half of all publications presented at least in parts new contents [ 36/65 ], whereas the others referred to previously published frameworks [ 29/65 ] or theories [ 10/65 ]. Publications not providing substantial new conceptual contributions most often referred to existing frameworks, such as the socio-ecological model [ 17 ], or relevant theories, such as the theory of planned behavior [ 18 ].
Among those frameworks considered conceptual and new [ N = 26] (see Table 1 ), about two thirds took a more general big picture perspective [ 21/26 ], whereas in one third the framework guided a specific project [ 9/26 ]. The majority covered the domains of active travel behavior [ 18/26 ] and its determinants [ 24/26 ], whereas four frameworks were concerned with impacts of active travel. Frameworks on active travel behavior split about equally between walking [ 12/18 ] and cycling [ 11/18 ], with almost half of all frameworks covering both modes. Most publications centered around the individual level [ 22/26 ], while many additionally considered the built environment [ 18/26 ] and households [ 13/26 ] as distinct structural levels. Only eight studies treated trips as a separate level. Temporal structures were absent in most frameworks [ 20/26 ] with few exceptions indicating feedback loops [ 6/26 ] or changes over time (before/after) [ 3/26 ]. About three quarters provided illustrations of the frameworks.
In terms of determinants of active travel, built environment [ 23/26 ], infrastructure measures [ 21/26 ], psychological [ 17/26 ] and socio-demographic factors [ 19/26 ] obtained about equal attention. Among the frameworks addressing travel behavior [ 18/26 ] , most described active travel in generic terms, such as walking [ 13/18 ], cycling [ 12/18 ], or even physical activity [ 9/18 ], but several frameworks were more nuanced by investigating different modes of travel, journey purposes (including walking or cycling for recreation or transport), and various quantitative measures, like frequency or duration. The few frameworks that address impacts of active travel [ 4 ] covered environmental (e.g., carbon emissions), health, and safety outcomes.
In the following section, we review a selection of frameworks considered most appropriate in providing distinct features to the development of a more comprehensive framework of active travel behavior. These features are briefly highlighted. Illustrations of some of these frameworks can be found in the supplemental materials available in https://www.researchgate.net/profile/Thomas_Goetschi/publications .
Review of Selected Frameworks
Pikora et al. [ 19 ] and Saelens et al. [ 20 ] were among the earliest publications showing conceptual frameworks for active travel behavior. Their frameworks are imbedded in a socio-ecological model [ 17 ], focusing on different layers of environmental (ecological) and individual (socio-psychological) factors explaining active travel outcomes. Such models are widely applied in health behavioral science, as reviewed for example by Sallis et al. [ 21 ]; they describe the role of the combined effects of psychosocial and environmental variables (i.e., community, policy) to explain physical activity. They imply that a combination of environmental and personal level factors will best explain behavior and that addressing both—or multiple—domains will allow for the development of most effective interventions. Saelens’ model, derived from a review of planning literature, distinguishes active travel for transport vs. recreation, as well as stronger and weaker links between factors.
Ogilvie et al. [ 4 , 22 ] presented a framework with the purpose to measure and evaluate changes in active travel and physical activity behavior resulting from physical infrastructure interventions as part of the longitudinal cohort study “iConnect” [ 23 ]. The iConnect model builds on the framework presented by Saelens et al. [ 20 ], which is expanded beyond active travel to include overall travel behavior, overall physical activity, and imputed impacts such as carbon emissions from motorized travel [ 4 ]. In addition, psychosocial factors, such as habit and social norms based on the extended theory of planned behavior [ 18 , 24 ], as well as social environment factors are introduced as key factors affecting behavior change. The framework applies Pawson and Tilley’s “realistic evaluation” framework [ 25 ] that postulates to distinguish and specify “contexts, mechanisms, and outcomes” (so-called CMO configurations) in order to evaluate how interventions work, for whom, and in what circumstances.
Panter et al. [ 26 ] built on a framework on school travel proposed by Macmillan et al. [ 27 , 28 ] and Pikora’s socio-ecological model for physical activity [ 19 ]. Focusing on active travel to school, they distinguished environmental and individual factors, paying particular attention to the interplay of parents’ and youth’s perceptions affecting mode choice for school travel. Within environmental factors, neighborhoods, destinations, and routes are distinguished, expanding the socio-ecological structure to include travel-specific elements. Also on the topic of parent youth relations when it comes to mode choice, Pont et al. [ 29 ] provide a framework most notable for its depiction of the parent child relation, as one of few examples illustrating a process over time.
Based on an extensive literature review, Burbidge and Goulias [ 30 ] proposed a conceptual framework mainly combining elements of the theory of planned behavior [ 18 ] and decision field theory [ 31 ], which they complemented with additional factors identified from the literature, such as infrastructure and residential location selection. At the core is a mode choice process, which is influenced by personal attributes, infrastructure and environment, time allocation, and various related factors. The choice process itself, however, is not explored in detail.
Schneider [ 32 ] explored the choice process in more detail proposing a theory of routine mode choice decisions in the wider context of policies attempting to shift trips from motorized to non-motorized modes. The theory suggests a five-step mode choice process, consisting of (1) awareness and availability of possible mode choices, (2) safety and security, (3) convenience and cost, (4) enjoyment, which then determine tradeoffs between the possible mode choices, and finally (5) habit, which reinforces earlier choices. Socio-demographic characteristics serve as moderators of these concepts. The theory builds on numerous insights from travel behavior research and psychology [ 18 , 33 , 34 ] and is substantiated with empirical evidence from qualitative interviews of San Francisco Bay Area residents.
Based on Maslow’s theory of human motivation [ 35 ], Alfonzo [ 36 ] presents a similar hierarchy of walking needs, where the individual first assesses feasibility, then accessibility, safety, comfort, and “pleasurability.” This is linked with moderating processes defined by life-cycle circumstances to determine outcomes. The life-cycle circumstances themselves include regional, group, and individual level factors such as climate, culture, and psychological factors, respectively (among others).
Singleton [ 37 ••, 38 ] proposed a framework based on an extensive review of travel behavior theories with the intention to improve direct applicability to active travel forecasting models. Its travel decision-making process also includes a hierarchy of travel needs, which are mediated by individual perceptions and decision rules (e.g., how a shorter trip distance is weighted against a higher crash risk [ 39 , 40 , 41 ]). At the start of the process is an activity, which results in a travel demand, or motivation, or desire to travel.
Martin et al. [ 42 ] explore the choice process with a specific focus on the mechanisms of policies. The study reviewed publications on policies that provide financial incentives to promote active travel, illustrating traditional economics (i.e., utility maximization, [ 39 ]) and psychological behavior theories (including behavioral economics) in terms of specific choice formulations and examples of policies addressing these [ 43 , 44 ].
In their extensive review of travel behavior and psychological theories, Van Acker et al. [ 34 ] synthesize numerous concepts of relevance to active travel. Their conceptual model emphasizes the distinction between reasoned influences on behavior, such as perceptions, preferences, and attitudes, and unreasoned influences driven by habits and impulsiveness. Feedback loops indicate the possibility of changes over time. Behavior in this framework is depicted as set of levels that range from the most short-term travel behavior to activity and locational behavior, all the way to lifestyle. Individuals’ behavior is further determined by opportunities and constraints, which present themselves at the individual level, as well as through the social and spatial environment.
In a similarly broad review of mode choice literature, De Witte et al. [ 45 •] emphasize an interdisciplinary perspective, identifying and distinguishing determinants by rationalist (i.e., journey characteristics), socio-geographical (i.e., spatial indicators), and socio-demographic domains, which in combination with socio-psychological factors determine mode choice.
In addition, numerous studies have published frameworks of relevance in the context of active travel that are not directly concerned with active travel behavior, such as safety [ 46 , 47 •, 48 ], types of cyclists [ 49 ], or physical activity [ 50 ], or do not specifically address active travel, such as MINDSPACE, a framework of how public policy influences behavior [ 43 ]. Further, there is abundant literature on travel behavior in general, and numerous theories have conceptualized it, many of which are reflected in the reviewed active travel frameworks. We refer to others for overviews of relevant theories [ 34 , 37 ••, 51 ].
Synthesis of Results and Development of the PASTA Framework of Active Travel Behavior
Key features of conceptual frameworks for active travel.
In this section, we synthesize and discuss key features of identified and reviewed conceptual frameworks for active travel and related theories and describe how we develop these to build the comprehensive PASTA conceptual framework of active travel behavior (from here on referred to as the PASTA framework). Our aim hereby is to absorb as many relevant concepts encountered in the reviewed frameworks and pertinent theories into a single, systematically structured framework as possible. To do so, we distinguish three features of conceptual frameworks representing active travel behavior:
Behavioral decision or choice process
Structural scales and relationships, contents and topical domains.
Similar to others [ 30 , 37 ••, 38 ], we conceptualize the core behavioral process as a demand (or need, desire, intention, or motivation) to travel that is derived from the need or desire to participate in an activity (i.e., work, shop, escort kids to school, visiting friends or family). This triggers a choice process which produces a behavioral decision or response, namely a revealed choice or outcome. (See Fig. 1 . For a simplified diagram of the generic process, see supplemental materials available in https://www.researchgate.net/profile/Thomas_Goetschi/publications .)
Generic choice process for active travel-related behavioral decisions. The column on the left illustrates the process to identify considered choices. From these, one choice is selected through the process in the column on the right
In the transport context a choice is often discrete, short-term, and trip related (i.e., mode choice, route choice) [ 39 ], but it is noteworthy that behavioral outcomes can also be aggregates of many choices over time, such as individual attributes of interest in health research (e.g., minutes of cycling per week or long-term physical activity derived from active travel). Active travel warrants consideration of both perspectives, and in the detailed version of the PASTA framework, we distinguish the two (see Appendix 3 ). For a systematic listing of cycling related choices and outcomes, see supplemental materials available in https://www.researchgate.net/profile/Thomas_Goetschi/publications .
Activities have their own temporality (rhythm, frequency, ir/regularity) and spatiality (distance vis-à-vis anchor locations (home, primary employer, possibly childcare, school) and spatial variability). Many of these activities cannot be adequately represented as rational choice behavior—they are at best boundedly rational [ 52 ] because of constraints on information availability and processing. But even this is a gross simplification as it does not consider the social dimensions of taking part in those activities.
As such, the choice process may very well be too complex to be explained by a single theory or depicted in a simple diagram. Nonetheless, numerous published concepts and theories have provided helpful guidance for research. Similar to several others [ 26 , 30 , 36 , 37 ••], we conceptualize the choice process in our framework as the central pathway that takes into account objective and subjective factors or opportunities and propensities [ 53 ], respectively, to make a behavioral decision or reveal a choice.
Figure 1 depicts the generic process. At the beginning, a travel demand (or activity) is met with objective opportunities, resulting in many theoretically possible choices, each with their specific attributes. The first of two sub-processes, or iterations (vertical flows in the diagram), identifies a subset of considered choices , which then in a second process are assessed to identify the selected choice . In reality, these may not be perfectly distinct, may inform each other, or may run in parallel (as indicated by parallel arrows).
When based on reasoned behavior [ 18 , 54 ], as illustrated for example by van Acker [ 34 ], these selections are derived from some combination of the objective attributes of the possible choices (or opportunities), such as mode accessibility, trip duration, safety, etc., and a set of subjective factors (or propensities), such as perceptions, attitudes, values, rules, preferences, and norms [ 12 ]. In unreasoned behavior, on the other hand, a habit or impulse circumvents the reasoned choice process leading directly to a choice without (much) consideration of other factors [ 33 , 34 ].
We conceptualize the reason-based part of the choice process similar to a utility-based approach assuming utility maximization behavior, as is commonly done in transport modeling [ 13 , 37 ••, 39 , 55 ]. However, empirical random utility maximization models are often constrained to a small number of measurable utility factors (i.e., monetary and time cost), which fail to capture the multitude of considerations determining active travel choices (e.g., weather, safety, health benefits, pleasure [ 56 , 57 ]). Moreover, active travel decisions, possibly more so than is the case for motorized travel behavior, may be based on other decision rules than compensatory utility maximization, as pointed out by Singleton and Clifton [ 37 ••, 38 ]. We therefore conceptualize this part more loosely as a generic choice assessment , which compares any sort of subjective value a subject is to gain from a choice over an alternative (black boxes in diagram). This accommodates active travel-specific utility dimensions, such as perceptions of safety, or expected health benefits, which are relatively more important for active travel than for motorized travel, but also irrational preferences, fears, or principles (i.e., non-compensatory rules [ 37 ••]). It is important to point out that the choice assessment is limited by the factors a subject actually considers and its perception or information of these, which may be “highly subjective” or incomplete (i.e., bounded rationality [ 58 ]).
While habit and impulsiveness complicate the link between need and revealed behavior, their importance warrants inclusion in our framework. We therefore keep non-reasoned behaviors, like sticking to a habit [ 59 ], following an impulse to try something new, or taking risk against better knowledge, separate from the reasoned choice assessment. However, it seems plausible that both the reasoned and unreasoned pathways could influence the same decision.
The way the generic choice process manifests in a specific situation depends on numerous environmental and personal factors. We treat these as determinants outside of the generic choice process.
The distinction of structural scales, such as hierarchies or clustering between factors, is of practical relevance for data collection and analysis, the validity of an analysis, and various other practical and methodological aspects (e.g., intervention design). Among the reviewed frameworks, the most common distinction is between environmental and personal factors, but further visualizations of spatial, social, or temporal scales have been used. The degree to which frameworks distinguish such structures varies tremendously. Aligning frameworks along structural scales seems particularly helpful when the distinction serves some specific purpose (e.g., data collection, model structure). We underlay the PASTA framework with a hierarchical socio-spatial pyramid (see Appendix 2 , Fig. A2), building on the often-referenced socio-ecological framework [ 19 , 21 , 22 ], which we expand to include travel-specific sub-individual layers, such as activity (leading to one or multiple trips), trip origin and destination, departure time, route, and mode of travel. These structures equally guide where we present determinants and outcomes.
The generic PASTA framework (Fig. 2 ) represents a static view on active travel behavior as opposed to behavioral change . To a large extent, the temporal scale is captured within the socio-spatial structures, in the sense that highest resolution layers (i.e., trips) reflect more short-term phenomena, whereas at the larger layers (i.e., society or city), processes take longer (i.e., changes in factors or periods over which factors affect each other [ 34 ]). Explicitly visualizing temporality of relationships would be warranted in frameworks focusing on behavior change, such as developments over the life course [ 49 ], effects of interventions [ 22 , 23 ], or those depicting behavioral change along a sequence of defined concepts or stages [ 40 , 60 ]. In quasi-static frameworks, feedback loops or cascading concepts are commonly used to indicate temporality [ 29 ], and some concepts capture it implicitly (e.g., habit).
PASTA conceptual framework of active travel behavior. A more detailed version of the framework including a detailed reader’s guide is available in Appendix 3 . Additional variations of the framework are available in the supplemental materials in https://www.researchgate.net/profile/Thomas_Goetschi/publications
In most published frameworks, directional arrows are the instrument of choice to indicate some sort of relationship or association between factors (or concepts). Variations include dashed and bidirectional arrows, sometimes labeled. The usefulness of arrows seems directly dependent on the specificity of factors and the understanding of relationships. In fairly comprehensive frameworks, the sheer number of related factors often prohibits the illustration of all relationships and using arrows without systematic and transparent criteria can become misleading. Alternatively, or additionally, spatial proximity and clustering or overlapping of factors is used. In the PASTA framework, we indicate relationships or influence between factors predominantly by proximity, arrangement, and clustering of factors (i.e., without using arrows). Only key causal flows are suggested by braces and arrows. Further, we aim for consistent directions of logical pathways (i.e., determinants leading to outcomes). Namely we arrange pathways of social factors roughly from left to right and pathways of environmental factors from top to bottom. However, when applied for specific purposes, such as conceptualizing a specific analysis, more specific arrows become useful (see supplemental materials available in https://www.researchgate.net/profile/Thomas_Goetschi/publications .).
Finally, the above structures are only meaningful when populated with specific, meaningful contents. Comprehensiveness and level of detail are direct tradeoffs and ultimately depend on the specific purpose of a framework but generally impose a challenge for visualization (though to a lesser degree in electronic documents that can be zoomed in, see Appendix 1 ). We arrange factors by topical domains, such as natural and built environment, socio-demographics, personal propensities, psychological factors, etc. We capture obvious sub-factors in overarching concepts to improve the readability of the framework.
Practical Applications of the PASTA Framework in Active Travel Research
There are many practical applications of conceptual frameworks. In particular, in the context of conducting a (large) research project, we argue that the consideration of a detailed conceptual framework, and possibly the development of a study-specific version, is crucial. The choice of data sources, data collection methods, and survey contents and when and where to sample are crucial questions in successfully pursuing research objectives. In the PASTA project, the framework served as a valuable cross-reference to assure the inclusion of as many of the most relevant determinants and potential confounding variables as possible, while at the same time keeping topical areas well-balanced and user burden in check (adapted framework versions are available in the supplemental materials available in https://www.researchgate.net/profile/Thomas_Goetschi/publications ).
The challenges of comprehensively assessing covariates and mediating factors are even more aggravated in research on changes in active travel behavior over time. Effects of interventions are typically small, requiring utmost attention to control of confounders and modifiers. A comprehensive framework is a helpful tool to identify these and anticipate their roles [ 60 ]. In PASTA, the framework guides the evaluation of key measures to promote walking and cycling, selected across the participating cities. The framework allows us to identify the critical putative causal pathways by which we believe the active travel measures are likely to work and as such informed survey contents and how we analyze these. We illustrate an example application highlighting the effects of the construction of high quality cycle highways in London and Antwerp and through which pathways they affect cycling behavior and in particular “stages of change” [ 40 ] (supplemental materials available in https://www.researchgate.net/profile/Thomas_Goetschi/publications ). Simplified, cycle highways affect regular cyclists through the provision of better routes (more direct, pleasant, and safe), while for infrequent or potential cyclists, the main pathway presumably is through an improvement in perceived safety, which may help them pursue their intention to bike more or pick up cycling.
Finally, the framework can also be helpful to inform sampling schemes. In PASTA, relatively stable personal factors, like general health or attitudes, were surveyed only once as part of a baseline questionnaire, whereas the more short-term and temporally variable factors like travel or physical activity behavior were surveyed in frequent follow-up questionnaires every 2 weeks.
Conclusions
Conceptual frameworks have been used to visualize a wide range of aspects of active travel behavior. Despite a remarkable diversity in illustrations, several common features could be identified. To date, the PASTA framework provides a first-of-its-kind effort to systematically combine behavioral concepts, structural features, and a large number of determinants identified in the literature as part of a single, comprehensive framework to inform future works. In PASTA, the framework provided valuable guidance in developing survey contents and study design, and in particular, for the combination of research approaches from the transport and health disciplines on how to best measure active travel and related factors. We conclude that the systematic development and use of a conceptual framework can provide invaluable support to design and conduct more elaborate and comprehensive active travel studies need to address key research gaps.
Papers of particular importance, published recently, have been highlighted as: • Of importance •• Of major importance
Physical activity: built environment approaches combining transportation system interventions with land use and environmental design [Internet]. The guide to community preventive services (the community guide). 2017 [cited 2017 May 22] Available from: https://www.thecommunityguide.org/findings/physical-activity-built-environment-approaches
European Commission,. Roadmap to a single European transport area—towards a competitive and resource efficient transport system [Internet]. Brussels,; 2011 Mar. Available from: https://ec.europa.eu/transport/themes/strategies/2011_white_paper_en
Parkin J, Wardman M, Page M. Estimation of the determinants of bicycle mode share for the journey to work using census data. Transportation. 2008;35(1):93–109.
Article Google Scholar
Ogilvie D, Bull F, Cooper A, Rutter H, Adams E, Brand C, et al. Evaluating the travel, physical activity and carbon impacts of a “natural experiment” in the provision of new walking and cycling infrastructure: methods for the core module of the iConnect study. BMJ open. 2012;2(1).
Buehler R, Dill J. Bikeway networks: a review of effects on cycling. Transp Rev. 2015;30:1–19.
Google Scholar
Buehler R, Götschi T, Winters M. Moving toward active transportation: how policies can encourage walking and bicycling. Active Living Res 2016;1–8.
Reynolds CC, Harris MA, Teschke K, Cripton PA, Winters M. The impact of transportation infrastructure on bicycling injuries and crashes: a review of the literature. Environ Health. 2009;8:47.
Article PubMed PubMed Central Google Scholar
Dons E, Götschi T, Nieuwenhuijsen M, De Nazelle A, Anaya E, Avila-Palencia I, et al. Physical Activity through Sustainable Transport Approaches (PASTA): protocol for a multi-centre, longitudinal study. Energy balance-related behaviours. BMC Public Health. 2015;15(1).
Mueller N, Rojas-Rueda D, Cole-Hunter T, de Nazelle A, Dons E, Gerike R, et al. Health impact assessment of active transportation: a systematic review. Prev Med. 2015;76:103–14.
Article PubMed Google Scholar
Goodman A, Brand C, Ogilvie D. Associations of health, physical activity and weight status with motorised travel and transport carbon dioxide emissions: a cross-sectional, observational study. Environ Health. 2012;11(1):52.
Article PubMed PubMed Central CAS Google Scholar
Brand C, Goodman A, Ogilvie D. consortium iConnect, others. Evaluating the impacts of new walking and cycling infrastructure on carbon dioxide emissions from motorized travel: a controlled longitudinal study. Appl Energy. 2014;128:284–95.
Heinen E, Handy S. Similarities in attitudes and norms and the effect on bicycle commuting: evidence from the bicycle cities Davis and Delft. Int J Sustain Transp. 2012;6(1–6):257–81.
Kuzmyak JR, Walters J, Bradley M, Kockelman KM. Estimating bicycling and walking for planning and project development: a guidebook. 2014.
Singleton PA, Clifton KJ. Pedestrians in regional travel demand forecasting models: state-of-the-practice. In: Transportation Research Board 92nd Annual Meeting [Internet]. 2013 [cited 2017 Mar 9]. Available from: http://nacto.org/wp-content/uploads/2015/04/Ped_RegionalTravelDemandForecasting_Singleton.pdf
• Gerike R, De Nazelle A, Nieuwenhuijsen M, Panis LI, Anaya E, Avila-Palencia I, et al. Physical Activity through Sustainable Transport Approaches (PASTA): a study protocol for a multicentre project. BMJ Open. 2016;6(1). Describes study design and objectives of the PASTA project, which motivated this work.
Dons E, Götschi T, Nieuwenhuijsen M, de Nazelle A, Anaya E, Avila-Palencia I, et al. Physical Activity through Sustainable Transport Approaches (PASTA): protocol for a multi-centre, longitudinal study. BMC Public Health. 2015;15(1):1126.
Sallis JF, Cervero RB, Ascher W, Henderson KA, Kraft MK, Kerr J. An ecological approach to creating active living communities. Annu Rev Public Health. 2006;27:297–322.
Ajzen I. The theory of planned behavior. Organ Behav Hum Decis Process. 1991;50(2):179–211.
Pikora T, Giles-Corti B, Bull F, Jamrozik K, Donovan R. Developing a framework for assessment of the environmental determinants of walking and cycling. Soc Sci Med. 2003;56(8):1693–703.
Saelens BE, Sallis JF, Frank LD. Environmental correlates of walking and cycling: findings from the transportation, urban design, and planning literatures. Ann Behav Med. 2003;25(2):80–91.
Sallis JF, Owen N, Fisher EB. Ecological models of health behavior. Health behavior and health education: Theory, research, and practice 2008;4:465–486.
Ogilvie D, Bull F, Powell J, Cooper AR, Brand C, Mutrie N, et al. An applied ecological framework for evaluating infrastructure to promote walking and cycling: the iConnect study. Am J Public Health. 2011;101(3):473–81.
Sahlqvist S, Goodman A, Cooper AR, Ogilvie D. Change in active travel and changes in recreational and total physical activity in adults: longitudinal findings from the iConnect study. Int J Behav Nutr Phys Act. 2013;10(1):28.
Darker CD, French DP, Eves FF, Sniehotta FF. An intervention to promote walking amongst the general population based on an “extended” theory of planned behaviour: a waiting list randomised controlled trial. Psychol Health. 2010;25(1):71–88.
Article PubMed CAS Google Scholar
Pawson R. Evidence-based policy: in search of a method. Evaluation. 2002;8(2):157–81.
Panter JR, Jones AP, van Sluijs EMF. Environmental determinants of active travel in youth: a review and framework for future research. Int J Behav Nutr Phys Act. 2008;5(1):34.
McMillan TE. The relative influence of urban form on a child’s travel mode to school. Transp Res A Policy Pract. 2007;41(1):69–79.
McMillan TE. Urban form and a child’s trip to school: the current literature and a framework for future research. CPL bibliography. 2005 May 1;19(4):440–56.
Pont K, Ziviani J, Wadley D, Abbott R. The Model of Children’s Active Travel (M-CAT): a conceptual framework for examining factors influencing children’s active travel. Aust Occup Ther J. 2011;58(3):138–44.
Burbidge S, Goulias K. Active travel behavior. Transportation letters 2009;1(2):147–67.
Busemayer JR, Townsend JT. Decision field theory: a dynamic-cognitive approach to decision making in an uncertain environment. Psychol Rev. 1993;100(3):432–59.
Schneider RJ. Theory of routine mode choice decisions: an operational framework to increase sustainable transportation. Transp Policy. 2013;25:128–37.
Bamberg S, Ajzen I, Schmidt P. Choice of travel mode in the theory of planned behavior: the roles of past behavior, habit, and reasoned action. Basic Appl Soc Psychol. 2003;25(3):175–87.
Van Acker V, Van Wee B, Witlox F. When transport geography meets social psychology: toward a conceptual model of travel behaviour. Transp Rev. 2010;30(2):219–40.
Maslow A. Motivation and personality. New York: Harpers. Inc; 1954.
Alfonzo MA. To walk or not to walk? The hierarchy of walking needs. Environ Behav. 2005;37(6):808–36.
•• Singleton PA. A theory of travel decision-making with applications for modeling active travel demand [Internet]. Citeseer; 2013 [cited 2017 Mar 9]. Available from: http://citeseerx.ist.psu.edu/viewdoc/download?doi=10.1.1.643.2207&rep=rep1&type=pdf . Provides a comprehensive review of (travel) behavior theories, and focuses on conceptualizing active travel behavior.
Singleton PA, Clifton KJ. The theory of travel decision-making: a conceptual framework of active travel behavior. In: Transportation Research Board 94th Annual Meeting [Internet]. 2015 [cited 2017 Mar 9] Available from: http://pdxscholar.library.pdx.edu/trec_seminar/84/
McFadden D, others. Conditional logit analysis of qualitative choice behavior. 1973 [cited 2017 Mar 9]; Available from: https://elsa.berkeley.edu/reprints/mcfadden/zarembka.pdf
Prochaska JO, Velicer WF. The transtheoretical model of health behavior change. Am J Health Promot. 1997;12(1):38–48.
Schwartz SH, Howard JA. A normative decision-making model of altruism. Altruism and helping behavior 1981;189–211.
Martin A, Suhrcke M, Ogilvie D. Financial incentives to promote active travel: an evidence review and economic framework. Am J Prev Med. 2012;43(6):e45–57.
Dolan P, Hallsworth M, Halpern D, King D, Vlaev I. MINDSPACE: influencing behaviour for public policy. 2010 [cited 2017 Mar 9]; Available from: http://eprints.lse.ac.uk/id/eprint/35792
Pratt M, Macera CA, Sallis JF, O’Donnell M, Frank LD. Economic interventions to promote physical activity: application of the SLOTH model. Am J Prev Med. 2004;27(3 Suppl):136–45.
• De Witte A, Hollevoet J, Dobruszkes F, Hubert M, Macharis C. Linking modal choice to motility: a comprehensive review. Transp Res A Policy Pract. 2013;49:329–41. Comprehensive review of mode choice literature .
Schepers P, Hagenzieker M, Methorst R, Van Wee B, Wegman F. A conceptual framework for road safety and mobility applied to cycling safety. Accid Anal Prev. 2014;62:331–40.
• Götschi T, Garrard J, Giles-Corti B. Cycling as a part of daily life: a review of health perspectives. Transp Rev. 2016;36(1):45–71. Review of current research issues at the fringe of cycling and health.
Miranda-Moreno LF, Morency P, El-Geneidy AM. The link between built environment, pedestrian activity and pedestrian–vehicle collision occurrence at signalized intersections. Accid Anal Prev. 2011;43(5):1624–34.
Davies DG, Halliday ME, Mayes M, Pocock RL. Attitudes to cycling: a qualitative study and conceptual framework. TRL REPORT 266 [Internet]. 1997 [cited 2017 Mar 9]; Available from: https://trid.trb.org/view.aspx?id=575843
Bauman AE, Reis RS, Sallis JF, Wells JC, Loos RJ, Martin BW. Correlates of physical activity: why are some people physically active and others not? Lancet. 2012;380(9838):258–71.
Handy S. Critical assessment of the literature on the relationships among transportation, land use, and physical activity. Transportation Research Board and the Institute of Medicine Committee on Physical Activity, Health, Transportation, and Land Use Resource paper for TRB Special Report [Internet]. 2005 [cited 2017 Mar 9];282 Available from: http://citeseerx.ist.psu.edu/viewdoc/download?doi=10.1.1.471.2645&rep=rep1&type=pdf
Simon HA. Model of bounded rationality. Vol 3: empirically grounded economic reason 1997;
Chapin FS, Chapin FS Human activity patterns in the city: things people do in time and in space [Internet]. Wiley New York; 1974 [cited 2017 Mar 9]. Available from: http://library.wur.nl/WebQuery/clc/126158
Fishbein M. A theory of reasoned action: some applications and implications. 1979 [cited 2017 Mar 9]; Available from: http://psycnet.apa.org/psycinfo/1982-21121-001
McFadden D. Disaggregate behavioral travel demand’s RUM side. Travel Behaviour Research 2000;17–63.
Panter JR, Jones A. Attitudes and the environment as determinants of active travel in adults: what do and don’t we know? J Phys Act Health. 2010;7(4):551–61.
Pucher J, Buehler R. City cycling. Pucher J, Buehler R, editors Cambridge, Massachusetts, London, England: MIT Press; 2012.
Simon HA. Models of bounded rationality: empirically grounded economic reason [Internet]. Vol. 3. MIT press; 1982 [cited 2017 Mar 9]. Available from: https://books.google.ch/books?hl=de&lr=&id=9CiwU28z6WQC&oi=fnd&pg=PA1&dq=bounded+rationality+&ots=GJYS5mjG4m&sig=BqPrtFbx_TBX_QN0B1fMiTxozoI
Garling T, Axhausen KW. Introduction: habitual travel choice. Transportation 2003;30(1):1–11.
Bamberg S, Fujii S, Friman M, Gärling T. Behaviour theory and soft transport policy measures. Transp Policy. 2011;18(1):228–35.
Download references
Acknowledgments
PASTA ( http://www.pastaproject.eu /) is a 4-year project funded by the European Union’s Seventh Framework Program under EC-GA No. 602624-2 (FP7-HEALTH-2013-INNOVATION-1).
Consortium members are as follows: B. Alasya, E. Anaya, I. Avila-Palencia, D. Banister, I. Bartana, F. Benvenuti, F. Boschetti, C. Brand, J. Buekers, L. Carniel, G. Carrasco Turigas, A. Castro, M. Cianfano, A. Clark, T. Cole-Hunter, V. Copley, P. De Boever, A. de Nazelle, C. Dimajo, E. Dons, M. Duran, U. Eriksson, H. Franzen, M. Gaupp-Berghausen, R. Gerike, R. Girmenia, T. Götschi, F. Hartmann, F. Iacorossi, L. Int Panis, S. Kahlmeier, H. Khreis, M. Laeremans, T. Martinez, M. Meschik, P. Michelle, P. Muehlmann, N. Mueller, M. Nieuwenhuijsen, A. Nilsson, F. Nussio, J.P. Orjuela Mendoza, S. Pisanti, J. Porcel, F. Racioppi, E. Raser, S. Riegler, H. Robrecht, D. Rojas Rueda, C. Rothballer, J. Sanchez, A. Schaller, R. Schuthof, C. Schweizer, A. Sillero, L. Smidfeltrosqvist, G. Spezzano, A. Standaert, E. Stigell, M. Surace, T. Uhlmann, K. Vancluysen, S. Wegener, H. Wennberg, G. Willis, J. Witzell, and V. Zeuschner.
Author information
Authors and affiliations.
Epidemiology, Biostatistics and Prevention Institute, University of Zurich, Zurich, Switzerland
Thomas Götschi
Centre for Environmental Policy, Imperial College London, London, UK
Audrey de Nazelle
Transport Studies Unit, University of Oxford, Oxford, UK
Christian Brand
Institute of Transport Planning and Road Traffic, Technische Universität Dresden, Dresden, Germany
Regine Gerike
You can also search for this author in PubMed Google Scholar
- , I. Avila-Palencia
- , D. Banister
- , I. Bartana
- , F. Benvenuti
- , F. Boschetti
- , J. Buekers
- , L. Carniel
- , G. Carrasco Turigas
- , A. Castro
- , M. Cianfano
- , T. Cole-Hunter
- , V. Copley
- , P. De Boever
- , A. de Nazelle
- , C. Dimajo
- , U. Eriksson
- , H. Franzen
- , M. Gaupp-Berghausen
- , R. Gerike
- , R. Girmenia
- , T. Götschi
- , F. Hartmann
- , F. Iacorossi
- , L. Int Panis
- , S. Kahlmeier
- , H. Khreis
- , M. Laeremans
- , T. Martinez
- , M. Meschik
- , P. Michelle
- , P. Muehlmann
- , N. Mueller
- , M. Nieuwenhuijsen
- , A. Nilsson
- , F. Nussio
- , J.P. Orjuela Mendoza
- , S. Pisanti
- , J. Porcel
- , F. Racioppi
- , S. Riegler
- , H. Robrecht
- , D. Rojas Rueda
- , C. Rothballer
- , J. Sanchez
- , A. Schaller
- , R. Schuthof
- , C. Schweizer
- , A. Sillero
- , L. Smidfeltrosqvist
- , G. Spezzano
- , A. Standaert
- , E. Stigell
- , M. Surace
- , T. Uhlmann
- , K. Vancluysen
- , S. Wegener
- , H. Wennberg
- , G. Willis
- , J. Witzell
- & V. Zeuschner
Corresponding author
Correspondence to Thomas Götschi .
Ethics declarations
Conflict of interest.
Thomas Götschi, Audrey de Nazelle, Christian Brand, and Regine Gerike declare that they have no conflict of interest.
Human and Animal Rights and Informed Consent
This article does not contain any studies with human or animal subjects performed by any of the authors.
Additional information
This article is part of the Topical Collection on Built Environment and Health
Electronic supplementary material
(DOCX 765 kb)
Rights and permissions
Open Access This article is distributed under the terms of the Creative Commons Attribution 4.0 International License (http://creativecommons.org/licenses/by/4.0/), which permits unrestricted use, distribution, and reproduction in any medium, provided you give appropriate credit to the original author(s) and the source, provide a link to the Creative Commons license, and indicate if changes were made.
Reprints and permissions
About this article
Götschi, T., de Nazelle, A., Brand, C. et al. Towards a Comprehensive Conceptual Framework of Active Travel Behavior: a Review and Synthesis of Published Frameworks. Curr Envir Health Rpt 4 , 286–295 (2017). https://doi.org/10.1007/s40572-017-0149-9
Download citation
Published : 13 July 2017
Issue Date : September 2017
DOI : https://doi.org/10.1007/s40572-017-0149-9
Share this article
Anyone you share the following link with will be able to read this content:
Sorry, a shareable link is not currently available for this article.
Provided by the Springer Nature SharedIt content-sharing initiative
- Logic model
- Pathway diagram
- Behavior theories
- Determinants
Advertisement
- Find a journal
- Publish with us
- Track your research
- View Record

TRID the TRIS and ITRD database
Concepts of Travel Behavior Research
In the first part this chapter proposes a conceptual framework for travel behavior research through a definition of the scope of the research topic, essentially human activity schedules, and a conceptualization of the traveler as a network actor negotiating infrastructure and human networks and dealing with the social content of the activities undertaken. In the second part of the chapter an operationalization of this framework through the dynamic microsimulation of daily life nested within the microsimulation of longer-term projects and choices.
- Find a library where document is available. Order URL: http://worldcat.org/isbn/9780080448534
- Abstract reprinted with permission from Elsevier.
- Axhausen, Kay W
- Publication Date: 2007
- Media Type: Print
- Edition: First
- Features: Figures; Maps; References;
- Pagination: pp 165-185
- Monograph Title: Threats from Car Traffic to the Quality of Urban Life: Problems, Causes, and Solutions
Subject/Index Terms
- TRT Terms: Activity choices ; Behavior ; Human factors ; Microsimulation ; Research ; Schedules ; Travel behavior ; Travelers
- Uncontrolled Terms: Daily activity plans
- Subject Areas: Highways; Planning and Forecasting; Public Transportation; Research; Safety and Human Factors; Society; I72: Traffic and Transport Planning;
Filing Info
- Accession Number: 01076811
- Record Type: Publication
- ISBN: 9780080448534
- Files: TRIS
- Created Date: Sep 21 2007 1:55PM

To read this content please select one of the options below:
Please note you do not have access to teaching notes, concepts of travel behaviour research.
Threats from Car Traffic to the Quality of Urban Life
ISBN : 978-0-08-044853-4 , eISBN : 978-0-08-048144-9
Publication date: 12 April 2007
Acknowledgements
This chapter draws on Axhausen (2006) and discussions during my lecturing and supervision. I gratefully acknowledge the input from those taking part.
Axhausen, K.W. (2007), "Concepts of Travel Behaviour Research", Gärling, T. and Steg, L. (Ed.) Threats from Car Traffic to the Quality of Urban Life , Emerald Group Publishing Limited, Leeds, pp. 165-185. https://doi.org/10.1108/9780080481449-009
Emerald Group Publishing Limited
Copyright © 2007 Emerald Group Publishing Limited
All feedback is valuable
Please share your general feedback
Report an issue or find answers to frequently asked questions
Contact Customer Support
Travel Behavior and Urban Land Use
Chapter 2 travel behavior theories.
Two different perspectives, individual and collective, can explain travel behavior. When people contextualizing travel as a personal choice or decision-making, the traveler as a subject make mode choices, driving or not. When travel behavior is understood as a social phenomenon, researcher observe and understand the trips as a whole. The two perspectives derived two schools of theory, mode choice and human mobility. In the school of mode choice, travel distance could be treat as an independent variable, a part of travel cost, or could be decided in the next step after mode choice , such as route choice. In the school of human mobility, driving distance grab more attentions.
2.1 Mode Choices (Travel as a subject)
Are ‘decision’ and ‘choice’ the same when discussing travel modes? Literally, a ‘choice’ is one decision given all available options at the same time. While ‘decision’ is a broader concept. A decision could be a schedule with a combination of many choices, such as modes, destination, and activities. A decision related to travel behavior could even include bicycle or car purchase, and relocation. This section will start from the theories of mode choice, then extend to a broader discussion of decision processes.
2.1.1 Rational Choice Theory
For prescriptive, analytical everyday decision-making, rationality is a basic assumption in reasoned behavior or rational choice theories ( Edwards 1954 ; Von Neumann and Morgenstern 1944 ) .
This category is also called ‘Normative Decision Theory,’ which assume people a traveler is an ideal decision maker who are full rational. It requires three necessary steps including information collection, utility evaluation, and choice making.
- Expected Utility Theory (EUT)
Traditional economics focus on the utility evaluation and come up with the Expected Utility Theory (EUT) which is also called Consumer Choice Theory. The rule of EUT is Random Utility Maximization (RUM) ( Ben-Akiva and Lerman 1985 ; McFadden 1973 ) . This classical theory claims that customer always choose the one most appropriate by comparing the advantages and disadvantages of a range of alternatives, evaluating the benefits and costs of each possible outcome. Eventually travelers will select the optimal solution with the maximum ‘utility’ from the choice set.
In real life, Rational Choice Theory can not accurately describe the actual human behavior. Individuals do not often collect and analyse all the relevant information. They are not ‘ideal’ and are not able to calculate the utility for all possible alternatives with perfect accuracy. In many cases, the travel decision is not regarded as the ‘best’ one to achieve travelers’ desired objective. Many other theories were developmed to fix these issues.
2.1.2 Bounded Rational Behavior
Bounded rationality focused on the limitation of self-control ( March and Simon 2005 ) . In reality, individuals are behaving under many constraints including incomplete information, limited time, and cognitive capacity. The observed behaviors often are not optimal and are inconsistent with ‘pure’ rationality. Bounded rationality claims that, when people make decisions under constraints, heuristics and rules of thumb are more common than statistical inference. People are satisfied with a ‘good enough’ decision unless there is a definitively better alternative. The recently witnessed events would have stronger effects on an individual’s decision than others ( Camerer, Loewenstein, and Rabin 2004 ) .
2.1.3 Theory of Planned Behavior
In psychology, many theories and models are developed to explain people’s decision-making processes. 4
Ajzen and Fishbein ( 1977 ) proposed the Theory of Reasoned Action (TRA) to understand people’s behavioral intentions and actual behaviors. They found two deciding psychological elements as attitudes and subjective norms . Ajzen ( 1991 ) adds a new part of Perceived Behavioral Control (PBC) and renames TRA as Theory of Planned Behavior (TPB).
Attitudes are personal evaluation and it means how people prefer or are against performing an activity. For example, a commuter might choose transit in spite of the longer travel time because this person believes that transit is an environment-friendly transport mode.
Subjective norm is the social pressure from others. In the example above, choosing transit is because of other people’s normative expectations rather than personal desirability.
PBC represents some nonvolitional factors such as time, budget, and resources. PBC is assessed by the individual’s perception of ease or difficulty of the behavior. PBC is one reason of the different between intentions and actual behaviors, which is called attitude-behavior gap ( Kollmuss and Agyeman 2002 ; Lane and Potter 2007 ) . In this case, a commuter might choose transit because this person is confident in catching the bus every day.
Based on RUM models, McFadden ( 2001 ) proposes a similar framework called the choice process including attitudes, perception, and preference. This framework is further developed to hybrid choice model (HCM) and non-RUM decision protocols ( Ben-Akiva et al. 2002 ) .
Two meta-analyses found that intentions to drive, perceived behavioral control, habits and past behavior play the primary roles in travel mode choice. Among these factors, PBC have the strongest effects on private car use. People don’t want to reduce the car use because they think it is very inconvenient. The effect of attitudes is modest while subjective norms have weak effect on car use ( Lanzini and Khan 2017 ; Gardner and Abraham 2008 ) .
2.1.4 Prospect Theory
Kahneman and Tversky ( 1979 ) introduced the ProspectTheory 5 to study the impacts of biases. Prospect Theory is a descriptive theory with three main components: First, people are more sensitive to the sure things (e.g., the probability between 0.9 and 1.0, or between 0.0 and 0.1 ), while being indifferent to the middle range (e.g., from 0.45 to 0.55). Second, people care more about the change of overall proportion than the absolute values regardless of gains or losses. Third, people make choice based on a reference point, rather than the overall situation or worth. Economist also extend the theory of expected utility maximization to Behavioral Economics by address the influence of psychology on human behavior.
- Regret Theory
Regret Theory introduces the notions of risk or uncertainty in decisions ( Loomes and Sugden 1982 ) . Psychological studies found that individuals will not only try to maximize the utility but to minimize the anticipation of regret. The fear of regret could affect people’s rational behavior. For example, A high risk of congestion in peak hours could encourage a commuter to choose transit mode. Likewise, a good reputation for punctuality can give traveler confidence in the rail system.
In addition to the traditional utility framework, a regret term is added to address the uncertainty resolution. The utility function on the best alternative outcome will be smaller after subtracting the regret term, which is an increasing, continuous and non-negative function.
- Cognitive Bias
Another psychological factor, cognitive bias can result in judgement errors. For example, people treat potential gains and losses differently, that is called Loss Aversion. Loss Aversion suggests that the negative feeling about losses is greater than the positive response to gains ( Tversky and Kahneman 1992 ) . As a result, individual’s decisions may not be consistent with evidence and tend to pay additional costs to avoid losses.
2.2 Human Mobility (Travel as an object)
2.2.1 trip distribution laws.
There is a long history of human mobility studies. In Physics and Geography, travel distance and pattern are treated as an objective phenomenon. The related theories try to use some statistical expressions to fit the aggregated trip distributions.
Gravity Law is a dominant theory in this field. Scholars have developed some more delicate forms of Gravity Law and found some mathematical relationship to other famous distribution laws. Some theories from different perspectives, like intervening opportunities also show strong ability for explaining travel patterns and regularities.
2.2.1.1 Distance Based Theories
- Law of Migration
An early theory called Law of Migration by Ravenstein ( 1885 ) tried to explain the regional migration patterns. This found is based on observation rather than quantitative analysis. But it capture the fact that the direction of migration is toward the regional center with great commerce and industry. It also pointed out that distance is a primary factor for migrant. This theory inspired many studies on population movement consequently. Even today, socio-economic factors and distance-constraints are the essential parts in the relevant models and frameworks.
Zip’s law is also called discrete Pareto distribution . It is found in linguistics to explain the inverse relationship between the frequency and rank of a word. The charm is that this rank-frequency distribution disclosed a universal law in many realms of society and physics, such as urban size, corporation sizes, cells’ transcriptomes and so on. Zipf interpreted the two competing factors as force of diversification and unification . The former produces larger amount of cases and the later tries to upgrade the rank. An equilibrium of the rank-frequency balance is controlled through a parameter \(\alpha\) in the exponent. For example, a city’s population size \(m\) has a negative power relationship to its rank \(r\) as below. 6
\[ m \sim 1 / r^{\alpha} \]
Zipf ( 1946 ) extended this expression to describe the traffic in both directions between two cities:
\[ t_{ij}\propto \frac{m_i m_j} {d_{ij}} \]
where \(t_{ij}\) represent the traffic flow of goods between two centers \(i\) and \(j\) with population sizes \(m_i\) and \(m_j\) . \(d_{ij}\) is the distance from \(i\) to \(j\) . Because Zipf’s formula has a same form with Newtonian mechanics ( Newton 1848 ) , people call this expression as Gravity Law.
- Gravity Law
As the most influential theory, Gravity Law asserts that the amount of traffic flow between two centers is proportional to the product of their mass and inverse to their distance. The mass is often measured by population size.
\[ p_{ij}\propto m_i m_j f(d_{ij}), \qquad i\ne j \]
where \(p_{ij}\) is the probability of commuting between origin \(i\) and destination \(j\) , satisfying \(\sum_{i,j=1}^n p_{ij}=1\) . \(m_i\) and \(m_j\) are the population of two census units. The travel cost between the two places is represented as a distance decay function of \(d_{ij}\) .
Exponential and power are the two forms of the distance decay function with a parameter \(\lambda\) showed as below:
\[ f(d_{ij})=\exp(-\lambda d_{ij}) \] and
\[ f(d_{ij})={d_{ij}}^{-\lambda} \] The function implies that the movements between the origin and destination decays with their distance. In transportation modeling, a common form of gravity model is :
\[ T_{ij}= \alpha_i O_i \cdot \beta_j D_j \cdot f(d_{ij}) \]
where \(T_{ij}\) is the flow between \(i\) and \(j\) . the two population are replaced by total tirp generation of origin \(O_i\) and total trip attraction of destination \(D_i\) . \(\alpha_i\) and \(\beta_j\) are two constraining parameters to satisfy \(\sum_{i}^{n_i}T_{ij} = D_j\) and \(\sum_{j}^{n_j}T_{ij} = O_i\) . It means that \(\alpha_i = [\sum_{j}^{n_j} \beta_j D_j \cdot f(d_{ij})]^{-1}\) and \(\beta_j = [\sum_{i}^{n_i} \alpha_i O_i \cdot f(d_{ij})]^{-1}\) . Thus, this model is called as doubly constrained gravity model.
If it relieves the two constrains. this model will be simplified to single-constrained and unconstrained gravity model. By assuming \(\alpha\beta\) is an adjustment parameter irrelevant to locations \(i\) and \(j\) for controlling the total flows, this model will not guarantee that the attraction of a destination equals the sum of flow from all origins, and the generation of a origin equals the sum of flow to all destinations.
Broadly speaking, Zipf’s law and Gravity Law have a common essence of power law, or scaling pattern. The Zipfian distribution is one of a family of power-law probability distributions. The power-law distribution also holds in many realms: urban size, population density, street blocks, building heights, etc.
The state-of-the-art studies of human mobility agree that travel behavior follows a power-law distribution at the population level ( Barbosa et al. 2018 ) . An example is Brockmann, Hufnagel, and Geisel ( 2006 ) use dollar bills to track travel habits and confirm this theory. It reflects the fact that both trip and land use, as two geographic variables, follow some Paretian-like distribution. Apparently, it conflicts with Gaussian thinking, the foundation frame of linear models based on the location and scale parameters. 7
Meanwhile, the log-normal distribution may be asymptotically equivalent to a special case of Zipf’s law, which could support the logarithm transform in current VMT-density models ( Saichev, Malevergne, and Sornette 2010 ) .
2.2.1.2 Opportunity Based Theories
- Law of Intervening Opportunities
Law of Intervening Opportunities by Stouffer ( 1940 ) developed the migration theory in a different direction. Stouffer proposed that “the number of people going a given distance is directly proportional to the number of opportunities at that distance and inversely proportional to the number of intervening opportunities.”
Comparing with gravity law, the number of intervening opportunities \(s_{ij}\) replaces the distance between origin and destination. For example, a resident living in location \(i\) is attracted to location \(j\) with \(s_{ij}\) job opportunities in between.
\[ p_{ij}\propto m_i \frac{P(1|m_i,m_j,s_{ij})}{\sum_{k=1}^n P(1|m_i,m_j,s_{ij})}, \qquad i\ne j \]
where the conditional probability \(P(1|m_i,m_j,s_{ij})\) can be expressed by Schneider (1959) as:
\[ P(1|m_i,m_j,s_{ij})=\exp[-\gamma s_{ij}] - \exp[-\gamma (m_j + s_{ij})] \]
- Radiation Law
Simini et al. ( 2012 ) propose a radiation model express the probability of the destination \(j\) absorbing a person living in location \(i\) as below:
\[ P(1|m_i,m_j,s_{ij})= \frac{m_i m_j}{(m_i + s_{ij})(m_i + m_j + s_{ij})} \]
Or in transportation model it is expressed as:
\[ T_{ij}= O_i\cdot\frac{m_i m_j}{(m_i + s_{ij})(m_i + m_j + s_{ij})} \] To approximating the number of opportunities, \(s_{ij}\) is from the population within a circle centered at origin. The radius is the distance between \(i\) and \(j\) . Then \(m_i + m_j + s_{ij}\) represents the total population within the circle, and \(m_i + s_{ij}\) is the total population within the circle but excluding \(j\) , that is:
\[ T_{ij}= O_i\cdot\frac{m_i }{m_i + s_{ij}}\cdot\frac{m_j}{m_i + m_j + s_{ij}} \] The part of fraction converts to the product of two weights, the weights of origin and destination in the whole region. Although distance \(d_{ij}\) doesn’t appear in the expression of radiation model, it is still a determinant as in gravity model.
- Distance Decay (hazard models)
Using the survival analysis framework, Yang et al. ( 2014 ) further extended this model by assuming a trip from origin to destination as a time-to-event process. Here time variable is replaced by the number of opportunities.
The survival function \(S(t)=Pr(T>t)\) represents the cumulative probability of the event not happened within a certain amount of opportunities. Choosing Weibull distribution as the survival function, \(S(t)=\exp[-\lambda t^\alpha]\) with scale parameter \(\lambda \in (0, +\infty)\) . By assuming \(f(\lambda)=\exp[-\lambda]\) and integral on \(\lambda\) , the derivation is:
By replacing \(t\) with \(m_i+s_{ij}\) , the conditional probability is:
\[ \begin{aligned} P(1|m_i,m_j,s_{ij})= &\frac{P(T>m_i+s_{ij})-P(T> m_i+s_{ij}+m_j)}{P(T>m_i)} \\ =&\frac{[(m_i + s_{ij} + m_j)^{\alpha}-(m_i + s_{ij})^{\alpha}](m_i^{\alpha}+1)}{[(m_i + s_{ij} + m_j)^{\alpha}+1][(m_i + s_{ij})^{\alpha}+1]}\\ \end{aligned} \]
where \(\alpha\) is a parameter adjusting the effect of the number of job opportunities between origins and destinations.
A similar method can be found in Ding, Mishra, et al. ( 2017 ) ’s study. They use a multilevel hazard model to examine the effects of TAZ level and individual level factors with respect to commuting distance using the data of Washington metropolitan area.
Based on commuting data from six countries, Lenormand, Bassolas, and Ramasco ( 2016 ) found gravity law performs better than the intervening opportunities law. The reasons could be the circle with radius \(d_{ij}\) can not accurately represent the real influencing area, and the different between population and opportunities is not captured in this way.
2.2.2 Time Geography
In contrast to overall trip distribution, the movements of individuals are always research interest in geography. Hägerstraand ( 1970 ) proposed some concepts and tools in space and time to measure and understand the individual trajectories. This branch is called time geography. The famous “space-time aquarium/prism” is a 3D cube by adding temporal scales on the geographic space. It can capture the detailed structure and behavior of traveler.
A daily travel could include multiple trips and form a travel chain. The traveler may switch the sequence or adjust the routes to optimize the chain and minimize the travel costs. The daily total travel distance is the summation of every trip distances. The number of trips denotes as trip count. It exists but not so common that driving itself is the travel purpose, especially in daily life.
At individual level, time geography borrows some physical and mathematical concept and methods such as random walk, Brownian motion, and Levy flight
Along with the wide usage of Global Positioning System (GPS), high performance computer, and sophisticated algorithms, the high-resolution data being collected. The relevant studies also have a dramatic increase after 2005.
2.3 Discussion
The theories of travel behavior follow a positivism tradition for a long time. Economics and geography give some strong explanations for both macro and micro travel patterns. In order to remove the limitation of ideal rationality, more sociological and psychological theories and methods are introduced into this field. Gradually, people realized the normative concept is not sufficient for real world applications. More descriptive and narrative arguments appear in transportation and land use planning. An example is the shift from mobility to accessibility.
A primary trend in urban and transportation fields recently is the transition from techno-centric to socio-centric (Lanzini & Stochetti, 2020) 8 The socio-centric methods claim that accessibility is the key concept for evaluating urban sustainable mobility. This trend emphasizes the interpretations of travel behavior are context dependent and avoids generalizations.
Research in human mobility insist the positivism methodology and has some significant contributions because the individuals differences are confounded at the macro level. Under this framework, geographic distance always plays a prominent role in all human mobility theories. In adding to travel distance and Origin-Destination Matrices, Some primary metrics such as Mean Square Displacement and Radius of gyration are defined to quantitatively describe travel behaviors
A vital insight is that human behavior has two mobility roles: explorers and returners. It might be an inherent property of society, the instinct of exploring more territory and keeping together for division of labor. The explorers’ behavior is consistent with the theory of utility maximization. People are always looking for more benefit. The concept of habit also match the behavior of ‘preferential return,’ which means people are natural or nurtured likely to return to frequently visited locations or recently-visited locations.
Both gravity and opportunities theory choose population size as the source of travel demand. This is a rough assumption and is not enough to get more accurate predictions. One solution is to use empirical observed demand to calibrate the model case by case. Another way is to find more suitable variables such as residential, employment, or activity size to improve the model.
When area of interest is intra-urban, the O-D matrix records the trip connections among all paired locations. The matrix contain plenty of information including urban spatial structure, opportunities, activities and other socio-economic characteristics. The theories imply that O-D matrix have some strong connections to travel behavior in some ways. The first challenge is how to mine the information and extract some explainable elements. A limitation is that the empirical O-D matrix may only reflect the particular characteristics in that city and can not be applied to others. The second challenge is how to get a generalized interpretation,
Once choosing the individual perspective, current theories and methods are still insufficient. For example, the physical transportation network is only a part of travel decisions. Social networks with a ‘hub-and-spoke structure’ play a prominent role in finding a job. Using social media data, some studies provide valuable insight but still have a gap to form new theories.
An interdisciplinary perspective could provide a theoretical explanation for model selection. Existing mobility theories can play an anchor to identify the key variables’ property and confirm the additive and linear relation among the factors.
CMDT=Cognitive moral development theory (Kohlberg, 1984),
ITB=Ipsative theory of behavior (Frey, 1988),
NAM=Norm activation model (Schwartz, 1977,Schwartz and Howard, 1981),
SDT=Self-determination theory(Deci & Ryan, 1985),
TAM=Technology acceptance model(Davis, 1989),
TDM=Travel demand management measures,
TNC=Theory of normative conduct (Cialdini et al., 1990,Cialdini et al., 1991),
TPB=Theory of planned behavior(Ajzen, 1985,Ajzen, 1991),
VBN=Value-belief-norm (Stern, 2000,Stern et al., 1999),
MGB=Model of goal-directed behavior(Perugini & Bagozzi, 2001), ↩︎
“Prospect Theory - an Overview ScienceDirect Topics ” ( n.d. ) ↩︎
Visser ( 2013 ) ; Jiang, Yin, and Liu ( 2015 ) ; Rozenfeld et al. ( 2011 ) ; Gomez-Lievano, Youn, and Bettencourt ( 2012 ) ; Hackmann and Klarl ( 2020 ) ↩︎
Jiang and Jia ( 2011 ) ; Chen and Jiang ( 2018 ) ; Jiang ( 2018a ) ; Jiang ( 2018b ) ↩︎
Lanzini P., Stochetti A.. From Techno-Centrism toSocio-Centrism: The Evolution of Principles for Urban Sustainable Mobility [J].International Journal of Sustainable Transportation, 2020, in Press. ↩︎

An official website of the United States government
The .gov means it’s official. Federal government websites often end in .gov or .mil. Before sharing sensitive information, make sure you’re on a federal government site.
The site is secure. The https:// ensures that you are connecting to the official website and that any information you provide is encrypted and transmitted securely.
- Publications
- Account settings
The PMC website is updating on October 15, 2024. Learn More or Try it out now .
- Advanced Search
- Journal List
- Front Psychol
How Tourists’ Perception Affects Travel Intention: Mechanism Pathways and Boundary Conditions
Xiufang jiang.
1 College of Economics, Southwest Minzu University, Chengdu, China
Jianxiong Qin
2 College of Historical Culture & Tourism, Southwest Minzu University, Chengdu, China
Jianguo Gao
3 College of Earth Sciences, Chengdu University of Technology, Chengdu, China
Mollie G. Gossage
4 Department of Anthropology, University of Wisconsin-Madison, Madison, AL, United States
Associated Data
The original contributions presented in this study are included in the article/supplementary material, further inquiries can be directed to the corresponding authors.
Tourist subjectivities have an important effect on behavioral intentions. Under the background of normalization, tourism decision-making manifests primarily in tourists’ individual preferences, which has led much research to ignore the importance of other subjective factors, as well as objective environmental factors. In the COVID-19 era, tourism behavior’s social attributes have become more prominent; the effect of important others or organizations’ attitudes toward tourism behavior, as well as personal knowledge, ability, and experience in preventing and controlling tourism risks, are evident. This study integrates knowledge-attitude-behavior (KAB), Theory of Perceived Risk (TPR), Social Identity Theory (SIT), and Theory of Planned Behavior (TPB), along with a comprehensive framework method, to construct an integrated model exploring the impact of knowledge, identity, and perceived risk on travel intention, to analyze its pathways and effects, to resolve the issue of mechanism, to analyze the moderating effect of past travel experience, and to answer the problem of boundary conditions. It finds that knowledge, perceived risk, and identity have a significant positive impact on travel intention; travel attitudes, subjective norms, and perceived control mediate the influence of knowledge, perceived risk, and identity on travel intention; these mechanism pathways do not always exist. The positive adjustment of past travel experiences shows that repeat visitors have a greater impact than newcomers and potential tourists.
Introduction
When assuming normalized circumstances, travel intention primarily arises from a combination of tourists’ personal preferences, expectations, motivations, and satisfaction, as well as destination marketing and other factors. However, existing research has tended to ignore other important influences, including subjective factors and objective environmental factors. Meanwhile, a variety and repetition of crisis events over many years have only underlined the social attributes of travel behavior. That is, whether a person engages in travel—and where to—depends not only on personal preferences, but also their knowledge regarding and ability to prevent and control tourism risks, their past travel experience, and the attitudes of other key persons or organizations regarding travel behavior at the time of decision-making. Following the initial outbreaks and rapid global spread of COVID-19, domestic and international tourism have stagnated; hospitality and other tourism-related industries have had to suspend work accordingly; no part of the industry has been unaffected ( Figure 1 ). Despite explosive growth in the global tourism industry over the past 40 years, establishing it as one of the major engines driving global economic development, employment, and industrial transformation, the COVID-19 pandemic has hit the brakes and stalled the engines. The enormity of the pandemic’s impact on global tourism is still coming to light. This reflects not only the tight bonds within tourism production and consumption networks in the age of globalization but also the vulnerability of the industry at large. At this moment there is an urgent need for tourism scholars and industry experts to examine the relationship between tourism and the global public health crisis from a variety of perspectives. Thus far scholars have measured the impact of relevant cognitive factors on travel intention in terms of knowledge, perceived risk ( Zhu and Deng, 2020 ), and psychological distance ( Li et al., 2020 ). However, the literature mostly approaches behavioral intention from the perspective of individual tourists rather than groups and tends to employ one or two different theories to account for cognitive factors’ influence on travel intention. Lee and Jan (2018) believe that an integrated framework provides researchers and managers with critical insights and a more accurate grasp of the factors influencing ecotourism behavior. Thus, they construct an integrated model combining multiple theories and previously neglected factors to survey complex relations within structures, explain working mechanisms, and enhance explanatory and predictive power.

China’s annual domestic tourist volume, 2012 to 2021.
To this end, this research deepens the study of tourists’ travel intention in two aspects: integrating key factors and concepts and testing these against a moderating variable. For the former, the three factors are knowledge (as in knowledgeattitude-behavior, or KAB theory), identity (as in social identity theory, SIT), and perceived risk (as in theory of perceived risk, TPR). These are integrated to build a more comprehensive model of travel intention factors, to explore the path and effect of tourists’ action, and to answer the question of mechanism pathways. As for the latter, past travel experience is used as a moderating variable to test for changes in the relations of the abovementioned variables with varying levels of past travel experience. When considering the impact of past travel experience on current travel intention, previous papers have mainly employed binary variables to compare whether travel intention was affected by past travel experience (potential tourists and actual tourists; first-time visitors and repeat visitors) for their assessments, but the current study increases precision by dividing tourists into three graded categories—potential tourists, first-time visitors, and re-visitors—to assess the impact of past travel experience on the path of this mechanism and solve the problem of boundary conditions.
Theoretical Background and Hypothetical Development
Theory of planned behavior.
The TPB is typically used to explain the relationship between attitudes and planned, intentional behavior when people have enough time to think about their attitudes. According to this theory, behavioral attitudes, subjective norms, and perceived behavioral control are the three key factors that affect behavioral intentions. Behavioral attitude is an individual’s personal evaluation of a behavior; subjective norms refer to the perception of whether important others agree with the behavior or not. These “important others” may be members of families, private networks, community organizations, work units, party organizations, and so on ( Duan and Jiang, 2008 ). Perceived behavioral control is the sense of control an individual believes he or she has over behavior as well as the perceived difficulty of performing that behavior. Generally, tourists with better professional knowledge and who are secure in their time and monetary resources have greater perceived control and thus stronger travel intention ( Zhu and Deng, 2020 ).
Theory of planned behavior and its extensions are often used to illustrate the mechanism behind tourists’ behavior intentions, and exhibit good explanatory and predictive power. Boguszewicz-Kreft et al. (2020) have verified the TPB model’s applicability to medical tourism and compared the differential willingness among consumers of different nationalities to use medical tourism services. Hu et al. (2021) have found that attitudes, perceived behavioral control, environmental awareness, and perceived moral obligations are significantly and positively correlated with young people’s intent for low-carbon travel behavior, while subjective norms are not. Chen et al. (2019) have applied TPB to a study of pro-environmental tourism behavior among urban residents, finding that intentions and habits are the key influential factors, while attitudes have the most significant impact on behavioral intention. Joo et al. (2020) , meanwhile, have found that perceived behavioral control and subjective norms have a significant positive impact on rural tourism intention; among these two, subjective norms have a greater effect, while attitudes have no significant effect on travel intention.
Knowledge-Attitude-Behavior
The KAB model divides human behavior into three processes: acquiring knowledge, generating belief, and forming behavior. According to this theory, attitude is the best predictor of behavior, knowledge is the basis of changes in attitude, and the degree of knowledge mastery affects the consistency of attitude and behavior. Thus tourism knowledge is the key to the development of attitudes and travel behavior, but this “knowledge” is different from knowledge in the objective sense; rather, it is an abstracted perception of knowledge that directly affects tourists’ psychology and decision-making practices, as through the arousal of confidence and willingness to act ( Quintal et al., 2010 ; Sharifpour et al., 2014 ). Zhu and Deng (2020) define such knowledge perception as a tourist’s mental assessment of his or her ability to identify and understand the risks of tourism and COVID-19, the danger these pose to humans and the tourism industry, as well as countermeasures and other related issues. Psychologically, having more knowledge can increase an individual’s personal control over uncertain scenarios ( Zhang et al., 2021 ). When travelers think that they have more knowledge than others, and thus a greater ability to prevent and control risks, they are more likely to participate in tourism activities ( Tassiello and Tillotson, 2020 ). Therefore, considering the above analysis, this study puts forth the following hypotheses:
- H1: Knowledge (a: knowledge of tourism; b: knowledge of COVID-19) has a direct and indirect positive impact on travel intention through attitudes.
- H2: Knowledge has a direct and indirect positive impact on travel intention through subjective norms.
- H3: Knowledge has a direct and indirect positive impact on travel intention through perceived behavioral control.
Social Identity Theory
Social identity theory focuses on individual behavior in group settings. It suggests that group belonging (regardless of that group’s size or distribution) is, to a large extent, an individual mental state, but one that is totally distinct from that person’s independent mental state outside of the group setting. Belonging to a group gives one social identity, or a shared collective answer to the question “who am I?”, along with a set of behaviors appropriate to that identity ( Abrams and Hogg, 2006 ). According to SIT, “identity” includes both self-identity, social identity, etc.; while self-identity is an individual’s perception of self-consistency and continuity ( Erikson, 1968 ); social identity is an individual’s awareness of belonging to a specific social group ( Tajfel, 1982 ). Such identification can work to depersonalize an individual’s self-perception and social actions.
In their research on the relationship between identity and past travel experience, Gieling and Ong (2016) find that attitudes and behaviors are affected by important social relationships. Likewise, Forsyth et al. (2015) suggest that a sense of community can influence individual behavior. Canovi (2019) , meanwhile, finds that winemakers’ identities are moderated by the local community, which ultimately affects their attitudes toward diversified tourism development. It is clear that travel intention is inseparable from larger social contexts. Researchers must move beyond understandings of individual intention and formulate their studies to account for communities’ collective consciousnesses, individual respect for collective interests, and the association of individual behavior with important social members or organizations. In light of this, the following hypotheses are put forward:
- H4: Tourism self-identity has a direct and indirect positive impact on travel intention through attitudes.
- H5: Tourism self-identity has a direct and indirect positive impact on travel intention through subjective norms.
- H6: Tourism self-identity has a direct and indirect positive impact on travel intention through perceived behavioral control.
Theory of Perceived Risk
The basic principles of TPR are based on the theory of bounded rationality and satisfaction. Bauer (1960) believes that when consumers make decisions, they do not seek to “maximize utility” as economists call it, but to minimize the associated risk. According to TPR, perceived risk is one’s expectation that he or she may suffer losses. This subjective take is important because if a tourist does not perceive risk, it may not affect his or her travel decisions; conversely, even in the absence of objective risk, a tourist’s perception of its presence may affect decision-making nevertheless ( Khan et al., 2019 ).
Initial research on perceived risk tended to suggest that the greater tourists’ perceived risk—in terms of time, economy, physical and mental health, etc.—the more likely they are to lower their travel intention in avoidance of said risk ( Fischer et al., 1991 ; Roehl and Fesenmaier, 1992 ; Zhu and Deng, 2020 ). As research has deepened, perceived risk’s positive effect on behavioral intention has received much attention. Scholars have found that perceived risk can enhance public attention to risk, crisis awareness, risk recognition and understanding, the ability to interpret risk information in a calm manner, to participate in discussions on risk, and to form reasonable perceptions and attitudes in relation to risk—important outcomes beneficial to the reduction of perceived risk and formation of positive behavioral attitudes ( Cui et al., 2016 ). And, as novel phenomena inevitably inspire some people’s curiosity, a certain level of perceived risk may actually inspire more adventurous attitudes and a willingness to face challenges. Reisinger and Mavondo (2005) find that backpackers have higher risk tolerance than group tourists and will seek out moderately high levels of risk to increase the excitement of travel. Vespestad et al. (2019) , meanwhile, propose that higher risk perception in adventure tourism is a point of attraction for its potential consumers. Thus, considering that perceived risk can also stimulate a person’s intent to travel, this study puts forth the following hypotheses:
- H7: Perceived risk has a direct and indirect positive impact on travel intention through attitudes.
- H8: Perceived risk has a direct and indirect positive impact on travel intention through subjective norms.
- H9: Perceived risk has a direct and indirect positive impact on travel intention through perceived behavioral control.
Moderating Role of Past Travel Experience
Past travel experience refers to individuals’ prior instances of personal participation in tourism activities. Such experience can increase willingness to revisit ( Roehl and Fesenmaier, 1992 ; Sönmez and Graefe, 1998 ; Vespestad et al., 2019 ). When it comes to behavioral intention, past travel experience has greater explanatory power than other variables in TPB, and when it comes to tourism behavior, in particular, past travel experience is considered a key determinant ( Ajzen, 2002 ). Tourists with different past travel experiences will differ significantly in cognitive levels and emotional attitudes, so past travel experience is often regarded as an important moderating factor ( Hammond et al., 1998 ; Ajzen, 2002 ). Deutsch and Krauss (1965) find that compared to indirect experience, personal experience can affect more consistency in attitude and behavior. For a more specific illustration, Beldona et al. (2005) find that earlier (i.e., more experienced) users of online travel sites are more likely to purchase online travel products than later users. Murodjon et al. (2021) also found that past personal experience had a significant impact on tourists’ behavioral intention in their study of Uzbekistan’s Silk Road tourism. Kim and Chen (2021) , when comparing two groups of interviewees, find that those with firsthand experience expressed higher destination loyalty and stronger behavioral intention. In view of these findings, this study puts forward the following hypotheses:
- H10: Past travel experience moderates the impact of (a) knowledge, (b) tourism self-identity, (c) perceived risk, (d) attitude, (e) subjective norms, and (f) perceived behavioral control on travel intention.
- H11: Past travel experience moderates the impact of (a) knowledge, (b) tourism self-identity, and (c) perceived risk on attitude.
- H12: Past travel experience moderates the impact of (a) knowledge, (b) tourism self-identity, and (c) perceived risk on subjective norms.
- H13: Past travel experience moderates the impact of (a) knowledge, (b) tourism self-identity, and (c) perceived risk on perceived behavioral control.
Lepp and Gibson (2003) indicate past travel experience as an important factor affecting tourists’ perceived risk. Likewise, Fuchs and Reichel (2011) find differences in risk perception between first-time and repeat visitors. As repeat visitors generally have more experience preventing and controlling tourism risks, their risk perception is lowered, and it is easier for them to form positive travel attitudes. At the same time, repeat visitors’ perceived control has a more pronounced effect on their travel intention; experienced tourists will even ignore the risks involved. They are also more familiar with the variety of travel activities and applicable precautions, more inclined to support the unified management of communities and destinations, and feel more in control of their behavior during travel. Accordingly, it may be deduced that the mediating role of attitudes, subjective norms, and perceived behavioral control on knowledge, tourism self-identity, and perceived risk’s impact on travel intention may be yet further regulated by past travel experience. Therefore, it is proposed that:
- H14: Past travel experience moderates the mediation of (a) attitudes, (b) subjective norms, and (c) perceived behavioral control between knowledge and travel intention.
- H15: Past travel experience moderates the mediation of (a) attitudes, (b) subjective norms, and (c) perceived behavioral control between tourism self-identity and travel intention.
- H16: Past travel experience moderates the mediation of (a) attitudes, (b) subjective norms, and (c) perceived behavioral control between perceived risk and travel intention.
By combining these hypotheses, this study proposes a comprehensive theoretical model ( Figure 2 ).

Conceptual diagram of a final integrated model.
Materials and Methods
Questionnaire design and variable measurement.
The main body of the questionnaire utilizes the 5-point Likert scale (1 = strongly disagree, 5 = strongly agree). To ensure the reliability and validity of results, its design draws upon well-established scales, with adjustments to fit the specific context and goals of the study. With reference to Zhu and Deng (2020) , knowledge is measured in two aspects—knowledge of tourism, and knowledge of COVID-19—across eight items, while the perceived risk is measured across 10 items and in four dimensions: physical, cost, performance, and equipment risk. Tourism self-identity, subjective norms, and perceived behavioral control draw on the well-established scales of Lee and Jan (2018) and are measured by four to five items each, while travel intention is measured by three items. As categorical variables cannot accurately measure the degree of impact the environment exerts during a certain experience, this study selects graded variables to measure past travel experience, dividing the respondents into three categories—potential tourists, first-time visitors, and repeat visitors—to analyze the moderating effect of past travel experience. A pre-test of reliability finds that after deleting the item “(KP1) I know the original cause of COVID-19”, the reliability coefficient improves significantly; this item was thus deleted during the revision of the questionnaire ( Table 1 ).
Results of exploratory factor analysis (EFA).
PR, perceived risk; SN, subjective norms; KC, knowledge of COVID-19; KT, knowledge of tourism; ATT, attitudes; TID, tourism self-identity; PBC, perceived behavioral control; TI, travel intention. Bold values indicates the absolute value of factor loading is greater than the threshold.
Survey Sampling and Data Collection
The sample size formula designed by Yamane (1967) is as below.
Where n represents sample size, N stands for the population, and e represents the precision level. Usually, e was at a 95% confidence level. In this study, in accordance with the figure 3.25 billion domestic person-trips for China in 2021, the formula yielded an n of 399.9 as the minimum acceptable sample size.
This study, using convenience sampling, collected data using the professional survey application ‘‘Questionnaire Star’’, 1 whose paying customers to cover more than 30,000 companies and 90% of universities in China. The official questionnaire was open from 26 December 2020 to 26 January 2021. After applying the “Questionnaire Recommendation Service” through Questionnaire Star, the online system randomly invited people from its 2.6-million-sample database to fill in their responses. Beyond that, members of the research team and the surrounding community were invited to respond to the survey as well—via weblink, QR code, or WeChat message.
The average time for each questionnaire is 487 s. The questionnaires with an answering time of less than 1 min or a missing proportion of more than 70% are considered invalid questionnaires. After the invalid questionnaires were deleted, a total of 405 valid questionnaires were obtained. Among the valid questionnaires, 69.8% of respondents are men and 30.2% are women; in terms of age structure, the largest group is 18–25 years (67.57%), followed by 26–30 years (18.81%) and 31–40 years (10.64%); as for educational attainment, the most common level is college or post-secondary professional schooling (55.56%), followed by high school or vocational schooling (25.68%), then masters or doctoral study (15.8%). Respondents come from 89 cities in 27 provinces and cover 15 occupations in 24 industries. Potential tourists, first-time visitors, and repeat visitors account for 20.15, 13.18, and 66.67% of respondents, respectively. In the first year of COVID (23 January 2020 to 26 January 2021), 80.3% of tourists did not participate in tourism, while 19.7% of tourists did participate in tourism activities.
Analysis Methods
For data analyses, the SPSSAU data scientific analysis platform ( https://spssau.com/ ) developed by Changsha Ranxing Information Technology Co., Ltd., location in Changsha, China, was used to run reliability and validity tests, descriptive statistics, and correlation coefficient checks. Building on that, hierarchical regression analysis was employed to test the main effect, mediated effect, and moderating effect, while a bias-corrected non-parametric percentile method (bootstrapping) was used to test for mediating and moderated mediating effects.
Reliability and Validity Analysis
First is index classification analysis. Thirty-six items were enriched through exploratory factor analysis (EFA) and rotated with maximum variance rotation. During factor analysis, KMO values were 0.88 > 0.6, indicating that the data can be used for factor analysis ( Kaiser, 1974 ). The final condensation into eight factors is shown in Table 1 . Cumulative variance is 78.41%, meaning that these eight factors are able to extract 78.41% of information from the total 36 items; variance (rotated)—that is, the amount of information extracted—for the eight factors following rotation are 15.91, 11.59, 9.68, 9.39, 9.38, 8.37, 8.2, and 5.9, respectively. This distribution is sufficiently uniform to demonstrate the comprehensive soundness of factor analysis results. Moreover, the 36 items fit well with professional expectations. Integrating the congruence between factors and analysis items, the eight condensed factors are ultimately named: “perceived risk,” “subjective norms,” “knowledge of COVID-19,” “knowledge of tourism,” “attitudes,” “tourism self-identity,” “perceived behavioral control,” and “travel intention.”
The second is the reliability test, which employs reliability analysis. The data involves eight dimensions: perceived risk, subjective norms, knowledge of COVID-19, knowledge of tourism, attitudes, tourism self-identity, perceived behavioral control, and travel intention. Cronbach’s alpha (α) measures the quality of data reliability. If the value of α is higher than 0.8, the data is highly reliable; if α is between 0.7 and 0.8, reliability is good; a value between 0.6 and 0.7 indicates acceptable reliability, and a value of 0.6 or below indicates poor reliability ( Eisinga et al., 2013 ). As shown in Table 2 , the questionnaire’s total score is 0.921 and all eight dimensions have a Cronbach’s alpha value higher than 0.8, with the lowest dimension scoring 0.831. Data reliability is thus of high quality, and the data is considered credible and true.
Results of reliability analysis.
The third is the convergent validity analysis. Confirmatory factor analysis (CFA) is conducted for a total of eight factors and 36 analysis items, as shown in Table 2 . The effective sample size is 405—more than ten times the number of analysis items, and within the moderate range ( Everitt, 1975 ). The study finds that all measurement items are significant to the.001 level ( p < 0.001). The scale of the study is represented by eight condensed factors. As shown in Table 3 , the factors’ average variance extracted (AVE) values are all greater than 0.6, with a minimum value of 0.634, significantly exceeding the standard of 0.5, while composite reliability (CR) values are all greater than.8, significantly exceeding the respective standard of 0.7. The study scale thus demonstrates excellent convergent validity ( Gim Chung Ruth et al., 2004 ). Moreover, the standardized factor loading coefficients for all 36 items corresponding to the eight factors, given in Table 4 , are greater than or equal to 0.7, comprehensively indicating the excellent convergent validity of scale data in this study.
Results of confirmatory factor analysis.
Standardized factor loading.
The fourth is discriminant validity testing. Discriminant validity is measured by comparing the square root of AVE—representing that factor’s convergence—with factors’ correlation coefficients, which express the degree of correlation. If a factor’s convergence is very strong (significantly stronger than the “correlation coefficient between this factor and other factors”), then it is considered to have discriminant validity. In this study, Pearson correlation analysis is performed first to determine the interfactor correlation coefficients. Next, the square root of each factor’s AVE value is compared against the interfactor correlation coefficients. The results of this analysis are given in Table 5 . The square roots of AVE for “knowledge of COVID-19” is 0.891, which is greater than the correlation coefficients between “knowledge of COVID-19” and all seven other factors (the highest is 0.547); similarly, the square roots of AVE for “knowledge of tourism” is 0.887, which is greater than all its correlation coefficients with other factors (the highest is 0.627); indeed, all eight factors have AVE root values that are higher than their interfactor correlation coefficients. Therefore, the study’s scale data has good discriminant validity ( Gim Chung Ruth et al., 2004 ).
Means, standard deviations, and correlation coefficients for study variables.
KT, knowledge of tourism; KC, knowledge of COVID-19; TID, tourism self-identity; PR, perceived risk; ATT, attitudes; SN, subjective norms; PBC, perceived behavioral control; TI, travel intention. Bold numbers are the square roots of average variance extracted values.
**p < 0.01.
***p < 0.001.
Multicollinearity, Autocorrelation, and Normality Tests
Linear regression analysis is used to assess how perceived risk, subjective norms, knowledge of COVID-19, knowledge of tourism, attitudes, tourism self-identity, and perceived behavioral control relate to “travel intention”. The study finds that the model passes the F -test ( p < 0.001); in other words, the model is meaningful, and at least one of the seven factors will have an impact on travel intention. As the model’s R 2 value is 0.79, these seven factors should explain 79% of the variation in travel intention. In addition, the model’s multicollinearity test results in a maximum VIF value of 1.79, which means that all factors’ VIF values are less than 5, indicating that there is no collinearity, and the model is satisfactory ( Hauke and Kossowski, 2011 ).
The Kolmogorov-Smirnov test is used to analyze the data’s distribution normality. It finds the skewness coefficient’s absolute value to fall between 0.12 and 1.05 and the kurtosis coefficient’s absolute value to fall between 0.12 and 2.15—well below the critical values of 3 and 8, respectively. The sample thus passes the normality test ( Drezner et al., 2010 ).
Descriptive Statistical Analysis
Pearson correlation analysis is used to verify correlation and the strength of the relationships between variables. The study finds a significant positive correlation between the following factors ( Table 5 ): knowledge of tourism and attitudes ( r = 0.39, p < 0.001), knowledge of COVID-19 and attitudes ( r = 0.55, p < 0.001), knowledge of tourism and subjective norms ( r = 0.38, p < 0.001), knowledge of COVID-19 and subjective norms ( r = 0.13, p < 0.01), knowledge of tourism and perceived behavioral control ( r = 0.49, p < 0.001), knowledge of COVID-19 and perceived behavioral control ( r = 0.18, p < 0.001), tourism self-identity and attitudes ( r = 0.46, p < 0.001), tourism self-identity and subjective norms ( r = 0.5, p < 0.001), tourism self-identity and perceived behavioral control ( r = 0.49, p < 0.001), perceived risk and attitudes ( r = 0.32, p < 0.001), perceived risk and perceived behavioral control ( r = 0.48, p < 0.001), knowledge of tourism and travel intention ( r = 0.43, p < 0.001), knowledge of COVID-19 and travel intention ( r = 0.15, p < 0.01), tourism self-identity and travel intention ( r = 0.56, p < 0.001), perceived risk and travel intention ( r = 0.31, p < 0.001), attitudes and travel intention ( r = 0.45, p < 0.001), subjective norms and travel intention ( r = 0.71, p < 0.001), perceived behavioral control and travel intention ( r = 0.51, p < 0.001). These results provide preliminary evidence for subsequent hypothesis testing.
Model Comparison Analysis
The study selects the most commonly used fitting indexes such as GFI, NNFI, CFI, RMR, RMSEA, etc. in order to analyze the fit of the model. As shown in Table 6 , compared with the KAB, SIT and TPB models, the integrated model has better fitting conditions and more reliable results.
The model indices of the four theoretical models.
IM, integrated model.
According to Jöreskog and Sörbom (1996) , χ 2 can test whether there is a statistically significant difference between the two competing models in their ability to explain covariance. Therefore, the study compares three competitive models (TPB model, SIT model, and KAB model) to determine the optimal model (integrated model). The fitting indexes of the four theoretical models in Table 6 show that the integrated model is better. The explanatory power of the integrated model and the competitive model showed a significant difference ( p < 0.001).
Hypothesis Testing
Main effect test.
After controlling for demographic and travel behavior characteristics—such as gender, age, marital status, educational attainment, industry, occupation, tourist origin, etc., regression analysis is used to verify the influence of independent variables on dependent variables. The study finds significant positive influences on travel intention for the following factors: knowledge (second-order) (β = 0.32, p < 0.001), knowledge of tourism (β = 0.35, p < 0.001), knowledge of COVID-19 (β = 0.11, p < 0.05), perceived risk (β = 0.24, p < 0.001), tourism self-identity (β = 0.58, p < 0.001). This means that knowledge, perceived risk, and tourism self-identity can increase travel intention.
Mediation Effect Test
After controlling for demographic and behavioral characteristics, the Bootstrap sampling method is used to test the mediating effect. The Bootstrap test for the sampling method refers to whether the 95% CI for the regression coefficient a*b contains the number 0; if it does not include the number 0, it means a mediating effect is present; if it does include the number 0, then there is no mediating effect. The results after sampling 5,000 times are shown in Table 7 . In total there are five independent variables, three mediator variables, and 15 mediation paths—among these, nine paths are fully mediated; four paths are partially mediated, and two paths are not significantly mediated.
Results of mediation analysis.
K, knowledge; KT, knowledge of tourism; KC, knowledge of COVID-19; TID, tourism self-identity; PR, perceived risk; ATT, attitudes; SN, subjective norms; PBC, perceived behavioral control; TI, travel intention.
*p < 0.05.
The 95% CIs corresponding to the mediating effect (on travel intention) of attitudes, subjective norms, and perceived behavioral control in knowledge (second-order), knowledge of tourism, and knowledge of COVID-19 do not contain 0, indicating a significant mediation path, and the effect sizes were all 100%. This means that the effect of knowledge on travel intention works through attitudes, subjective norms, and perceived behavioral control. That is to say, the findings support Hypotheses 1-3: knowledge can both directly influence travel intention and indirectly promote travel intention when mediated by attitudes, subjective norms, and perceived behavioral control.
The 95% CIs respectively corresponding to the mediating effects of subjective norms and perceived behavioral control in tourism self-identity’s effect on travel intention are [0.16,0.31] and [0.01,0.1], not containing 0, indicating a significant mediating effect. This validates Hypotheses 5 and 6: tourism self-identity can both directly influence travel intention and indirectly promote travel intention through the mediation of subjective norms and perceived behavioral control. In the two groups, the indirect effects of subjective norms and perceived behavioral control are 41.17 and 8.78%, respectively.
The 95% CIs respectively corresponding to the mediating effect of attitudes and perceived behavioral control in perceived risk’s effect on travel intention are [0.00,0.05] and [0.01,0.11], not containing 0, indicating a significant mediating effect. Thus Hypotheses 7 and 9 hold: that perceived risk can both directly influence travel intention and indirectly promote travel intention through the mediation of attitudes and perceived behavioral control. In the two groups, the indirect effects of attitudes and perceived behavioral control are 8.96 and 17.94%, respectively.
As for the two remaining paths, however, (TI←ATT←TID and TI←SN←PR), the 95% CIs are [−0.00,0.07] and [−0.04,0.13], and contain the number 0, meaning that the mediating effect is not significant. Thus perceived risk does not affect travel intention indirectly via subjective norms, and tourism self-identity does not affect travel intention indirectly via attitudes; Hypotheses 4 and 8 do not hold.
Test of the Moderating Effect
After centering the independent and moderator variables and controlling for demographic and travel behavior characteristics, the moderating effect is tested. This analyzes the effect of independent variables on dependent variables and whether or not the moderator comes into play—that is, when the level of past travel experience varies, whether there is a significant difference in the magnitude of influence.
The study finds that when the level of past travel experience varies, the impact of knowledge, knowledge of COVID-19, tourism self-identity, perceived risk, attitude, and subjective norms on travel intention are the same ( p > 0.05). Therefore, H10b–H10e do not hold, the effects of knowledge, knowledge of COVID-19, tourism self-identity, perceived risk, attitude, and subjective norms on travel intention were completely consistent regardless of the subject’s status as a first-time visitor, repeat visitor, or potential tourist. Generally speaking, the influence that knowledge exerts over travel intention is unperturbed by the factor of past travel experience; however, this is not always the case. While past travel experience does not interfere with the effect of knowledge of COVID-19 on travel intention, it does interfere with the effect of knowledge of tourism on travel intention ( p = 0.001). The strength of interference varies as follows: repeat visitors > first-time visitors > potential tourists ( Figure 3A ). Therefore, H10a holds in part. As shown in Figure 3B , the effect of perceived behavioral control on travel intention is positively moderated by the interference of past travel experience ( p = 0.036). The more travel experience, the greater the effect. That is, repeat visitors > first-time visitors > and potential tourists. H10f is therefore supported.

(A,B) The moderating effect of past travel experience on travel intention. (C–F) The moderating effect of past travel experience on attitudes. (G–I) The moderating effect of past travel experience on subjective norms. (J–L) The moderating effect of past travel experience on perceived behavioral control.
As shown in Figures 3C–F , the impact of knowledge (second-order) ( p = 0.013), knowledge of tourism ( p = 0.000), knowledge of COVID-19 ( p = 0.022), and perceived risk ( p = 0.002) on attitude are significantly different, indicating past travel experience regulates the impact of these factors on attitude. The richer the past travel experience, the more positive the attitude. H11a and H11c thus hold. When the level of past travel experience varies, the impact of tourism self-identity on attitude is the same ( p > 0.05). H11b is not supported.
As shown in Figures 3G–I , the impact of knowledge (second-order), knowledge of tourism, and tourism self-identity on subjective norms vary with the level of past travel experience. When past travel experience is richer, the respective impact of the three abovementioned factors on subjective norms is greater. H12b thus holds while H12c is not supported. H12a partially holds. Past travel experience tends to interfere with the effect of knowledge on subjective norms, but not invariably; it moderates the effect of knowledge of tourism (as shown in Figure 3H : repeat visitors > first-time visitors > potential tourists) but does not moderate the effect of knowledge of COVID-19 on subjective norms ( p > 0.05).
As shown in Figures 3J–L , the impact of knowledge (second-order), knowledge of tourism, and tourism self-identity on perceived behavioral control vary with the level of past travel experience. When past travel experience is richer, the impact of the three abovementioned factors on perceived behavioral control is greater. H13b thus holds while H13c is not supported. H13a holds in part. Past travel experience generally does moderate the effect of knowledge on perceived behavioral control, but not always; while the effect of knowledge of tourism is affected by past travel experience (as shown in Figure 3K : repeat visitors > first-time visitors > potential tourists), the effect of knowledge of COVID-19 on behavioral control is not thus affected ( p > 0.05).
Moderated Mediation Model Test
In the moderated mediation model, the independent variable affects the dependent variable through a moderator variable such that the process of mediation is moderated by it. the moderated mediation model is used to analyze whether there are significant differences in the mediating effect with varying levels of a moderator variable. The test results for this study are given in Table 8 . When there is a high level of past travel experience, the mediating effect of knowledge (second-order) and knowledge of COVID-19 on travel intention through attitudes is not significant (i.e., BootCI contains the number 0), but when there is a low or average level of past travel experience, the mediating effect is significant (i.e., BootCI does not contain the number 0). When there is either a low or high level of past travel experience, the mediating effect of knowledge of tourism on travel intention through attitudes is not significant (BootCI contains 0), but where there is an average level of past travel experience, the mediating effect becomes significant (BootCI does not contain 0). This suggests a lack of consistency regarding the mediating impact of attitudes across the three levels and that a conditional mediating effect exists for the three paths TI←ATT←K, TI←ATT←KT, and TI←ATT←KC. Therefore, H14a holds: past travel experience moderates the mediation of attitudes between knowledge and travel intention.
Results of a moderated mediation analysis.
When there is a low level of past travel experience, then the mediating effects of knowledge, knowledge of tourism, and knowledge of COVID-19 on travel intention through subjective norms and perceived behavioral control, respectively, are not significant (BootCI contains 0); however, at average or high levels of past travel experience, the mediating effect becomes significant (BootCI does not contain 0). This indicates that subjective norms and perceived behavioral control each have inconsistent mediating effects across the three levels. Therefore, for the six pathways TI←SN←K, TI←SN←KT, TI←SN←KC, TI←PBC←K, TI←PBC←KT, and TI←PBC←KC, conditional mediation exists; H14b and H14c hold.
For the mediation path TI←ATT←TID, the mediating effect of attitude is not significant regardless of the level of past travel experience (BootCI contains 0); H15a does not hold. In contrast, subjective norms do significantly mediate (BootCI does not contain 0) for the TI←SN←TID path, regardless of the level of past travel experience. At all three levels, subjective norms play a mediating role, and the size of the effect is always greater than 0. This indicates no moderation because the mediating effect is consistently the same. Thus, H15b does not hold. As for the TI←PBC←TID path, when past travel experience is at a low level, perceived behavioral control does not play a mediating role (BootCI contains 0), but it does play a mediating role at average or high levels (BootCI does not contain 0). This inconsistency in mediation across the three levels suggests conditional mediation, such that H15c holds.
For the path TI←ATT←PR, the mediating effect of attitude is not significant when past travel experience is at either a low or high level (BootCI contains 0). Here, the difference is that the effect value is negative (−0.003) at a low level and positive (0.016) at a high level. The mediating effect of attitude is, however, significant when past travel experience is at an average level (BootCI does not contain 0). Evidentially, the mediating effect of attitudes is inconsistent across the three levels; thus conditional mediation is present and H16a holds. As for TI←SN←PR, subjective norms do not play a mediating role regardless of the level of past travel experience (BootCI contains 0). There is no significant conditional mediation, and H16b does not hold. Regarding path TI←PBC←PR, perceived behavioral control does not mediate at a low level of past travel experience (BootCI contains 0) but does mediate at average or high levels (BootCI does not contain 0). This inconsistency points to perceived behavioral control’s conditional mediating role, effectively supporting H16c.
Perhaps no event in modern tourism has had (and continues to have) a more significant impact on travel desire, perceived travel risk, and the hospitality industry at large than the 2020 outbreak and global spread of COVID-19. As tourism destinations and management continue to grapple with the threat of outbreaks or case surges, control, and prevention measures, changing policies and various closures, the quality of knowledge on tourist subjectivities and behavior will only become more valuable. Although this study is not specifically focused on the impact of COVID-19 on risk perception (cf. Nazneen et al., 2020 ; Sánchez-Cañizares et al., 2021 ; Chi et al., 2022 ; Jiang et al., 2022 ), the pandemic is still a crucial element and inseparable background to its findings. Until the virus is defeated on the global scale, the subjective and objective effects of COVID-19 must be integrated into tourist psychology research to some degree. At the same time, it is important not to set the scope of research too narrowly and over-focus on individual reactions in the present moment, as new information is always filtered through past experiences, and potential behavior is mentally screened against the anticipated opinions and reactions of key social others. This study provides a comprehensive framework for understanding travel intention outcomes that are both adequately unique to “post-COVID” reality and sufficiently holistic for wide application.
Consistent with the conclusions of Quintal et al. (2010) and Sharifpour et al. (2014) , this study finds that knowledge has a significant positive impact on travel intention. If travelers suspect that their knowledge is insufficient, they may mitigate uncertainty by abstaining from tourism activities. This, however, does not mean that the acquisition of knowledge can immediately change attitudes and affect behavioral intentions. In practice, if 40% of people exhibit a behavior, then 60% of people must have a positive attitude toward engaging in the behavior; 80% of people believe in a kind of behavior, and more than 90% must have the necessary knowledge in order for the behavior to change ( Wang and Cheng, 2018 ). Related research has also found that more knowledge is not necessarily better, because there are negative effects associated with “information overload”. Some of the main reasons that tourists visit where they do are heterogeneity and curiosity, so when they become too familiar with a destination, curiosity weakens, as does that destination’s attractive force ( Park and Jang, 2013 ; Hadar and Sood, 2014 ; Hu and Krishen, 2019 ). Zhu and Deng (2020) find that the greater tourists’ knowledge of tourism, the higher they will evaluate their own abilities in tourism risk management, and the stronger their travel intention will be. However, this article proposes that due to the characteristics of COVID-19—high transmissibility, a long incubation period, rapid mutation, and novelty (that is, a lack of previous experience in its prevention and control)—individual tourists will not have significantly improved perceived behavioral control with greater knowledge of COVID-19.
It is typically assumed that perceived risk undermines confidence and reduces perceived control over a situation. Conversely, this study conforms with Reisinger and Mavondo (2005) ; Hajibaba et al. (2017) , and Vespestad et al. (2019) , finding that perceived risk has a significant positive impact on travel intention. There are three possible explanations for this result: (1) Perceived risk allows individuals to understand potential threats and thus appropriately determine the level of risk, take scientific precautions, actively respond, and lessen adverse effects. (2) The situation with COVID-19-related risks is somewhat different but to a similar end. COVID-19 has yet to be completely eliminated, and threats of new outbreaks are ongoing. Even the cancellation of tourism activities may not be enough to avoid the risk of infection. Still, due to new structures or feelings of social isolation, people are eager to release psychological pressure through tourism behavior, including “getting close to nature”. Thus, the pandemic has amplified travel desires. (3) Moderate risks can actually increase the excitement of travel. In moderation, risks stimulate tourists’ adventurous spirit and drive to face challenges, which will stimulate their desire to travel as well. Some tourists will even seek out a highly volatile destination for travel ( Fuchs and Reichel, 2011 ). The effect of perceived risk on travel intention is thus two-sided, in some contexts actually enhancing the intention to travel. This study finds that perceived risk negatively affects tourism attitudes among potential tourists, but positively affects the travel attitudes of first-time and repeat visitors. Perhaps this is because with an increase in past travel experience, tourists’ knowledge and ability to prevent and control tourism risks have been continuously enhanced, and tolerance of tourism risks has increased accordingly as well. These conclusions enrich the systematic understanding of how perceived risk affects travel attitudes and travel intention.
The study has added important concepts to the model—such as tourism self-identity and subjective norms—and put the study of individual behavioral intention into its social context, exploring how group psychological states affect an individual’s social perception, social attitude, and social behavior. Similar to Lee and Jan (2018) , in this study tourism self-identity has a significant positive influence on travel intention. Compared with other influencing factors, tourism self-identity and subjective norms have a greater impact on travel intention. Therefore, tourism marketing after COVID-19 must not only focus on tourists, but also on the attitudes of potential tourists, important others, and important groups.
The study divides tourists into three categories—potential tourists, first-time visitors, and repeat visitors—to verify the moderating effect of past travel experiences. Consistent with previous findings ( Sönmez and Graefe, 1998 ; Ajzen, 2002 ; Tassiello and Tillotson, 2020 ), when tourists have more past travel experience, there is positive moderation of the indirect effects on travel intention—through travel attitudes, subjective norms, and perceived control, perceived risk through travel attitudes, and tourism self-identity through subjective norms and perceived control. Generally speaking, the more past travel experience, the greater the effects. Similar to Ramkissoon and Mavondo (2015) , which identify gender as a strong moderator of tourists’ place satisfaction and pro-environmental behavioral intention, this study’s introduction of past travel experience substantially improves explanatory power as well as the multi-dimensionality and holism of tourism behavioral modeling. Tourist psychology has much more to do with social roles and ties formed prior to or outside of the defined tourism context than is generally appreciated, and understanding the interplay of those forces requires the application of more nuanced moderated mediation models.
Conclusion and Limitations
This study integrates TPR, TPB, SIT, and KAB models to simultaneously investigate multiple influences on travel intention, including cognitive factors and environmental factors. It builds a comprehensive model for analyzing the mechanisms by which multiple cognitive factors affect travel intention, explores the associated boundary conditions, and uses past travel experience as the moderating variable. In accounting for travel intention, the comprehensive model’s explanatory power comes to 79%, thus outperforming the TPR, TPB, SIT, and KAB models, respectively. This work verifies the combined influence of internal subjective and external objective factors on travel intention, which extends and strengthens the overall psychological-sociological framework for researching travel intention. One key finding is that subjective norms—i.e., the approval of important others—have a greater impact on travel intention than more strictly personal factors such as perceived risk and perceived behavioral control. This points to the importance of contextualizing individual tourists’ intentions within their social relations, an approach that has previously been neglected. The study also finds that, overall, knowledge can have a direct influence on travel intention as well as an indirect influence through attitudes, subjective norms, or perceived behavioral control. Identity, meanwhile, can have a direct influence on travel intention, but indirectly only works through subjective norms or perceived behavioral control, and not through attitudes. As for perceived risk, it can have a direct influence on travel intention, but only influences it indirectly through attitudes and perceived behavioral control, and not through subjective norms.
Study findings indicate that past travel experience moderates the following twelve effects: knowledge of tourism and perceived behavioral control on travel intention; knowledge, knowledge of tourism, knowledge of COVID-19 and perceived risk on attitudes; knowledge, knowledge of tourism, and identity on subjective norms; and of knowledge, knowledge of tourism, and identity on perceived behavioral control. In addition, it also positively moderates the mediating effects of attitudes, subjective norms, and perceived behavioral control. The richer a tourist’s past travel experience, the greater the effect (repeat visitors > first-time visitors > potential tourists). In that sense, this study tests the influence of practical knowledge on tourists’ perceptions and travel decisions. Compared with indirect experience (knowledge of tourism and knowledge of COVID-19), the direct, practical experience of past travel has a greater impact on tourists’ perceptions and travel decisions. This shows that the source of knowledge is important, leading to different cognitive and behavioral outcomes. Therefore, research on the relationship between tourists’ cognitive factors and tourism attitudes or behavioral decisions will benefit greatly from the introduction of the independent variable “past travel experience.”
This study’s comprehensive model lays the groundwork for better decision-making in tourism management. Finding that subjective norms are the most important factor influencing travel intention, implies that post-COVID tourism marketing must focus not only on the tourists themselves but on the attitudes of potential tourists, important others, and important groups as well. Analysis of subjective norms’ antecedent variables finds that knowledge and self-identity significantly improve tourists’ evaluation of important others’ travel behavior approval, whereas perceived risk does not lead to an improvement in subjective norms. Therefore, tourism destinations can improve tourists’ evaluation of subjective norms by various routes including promoting knowledge of tourism risks and enhancing tourism self-identity. At the same time, these methods can improve tourists’ sense of perceived behavioral control, which in turn reinforces travel intention. Because perceived risk positively affects travel intention, the development of abundant and stimulating experiential tourism products is crucial to improving travel intention. In comparing the specific dimensions of perceived risk, this study finds that potential tourists are most concerned about cost risks; therefore, tourist receiving locations should actively mitigate the risks of economic and time costs that tourists face—by setting reasonable prices, by better-publicizing traffic, tour route and tourism product information and by improving the quality of tourism services. Moreover, in light of the current epidemic situation and tourists’ concomitant psychological fluctuations, destinations must implement normalized epidemic prevention measures to create a safe and comfortable tourism environment.
This study does have its limitations. First, although the predictive power of the comprehensive model exceeds that of competing models, it still falls short of completely explaining travel intention, indicating that there are other factors at play. This study only selected typical cognitive factors to verify their influence on travel intention. In reality, tourists’ behavioral decisions may also be affected by emotional factors (e.g., worry) and other cognitive factors (perceived value, satisfaction, etc.). Follow-up studies may construct a more complete theoretical model. Second, the study finds that knowledge has a significant positive impact on travel intention, but it is known that information overload reduces the attractiveness of destinations to tourists, in turn reducing their travel intention ( Hadar and Sood, 2014 ; Hu and Krishen, 2019 ). Resolution of this apparent paradox will require follow-up studies to compare the effect boundaries for different levels of knowledge affecting behavioral intentions in greater detail, thereby identifying the thresholds at which knowledge changes from a positive to a negative factor for travel intention.
Data Availability Statement
Ethics statement.
The studies involving human participants were reviewed and approved by College of Economics, Southwest Minzu University. Written informed consent to participate in this study was provided by the participants’ legal guardian/next of kin.
Author Contributions
XJ conceived and designed the concept, collected the data, and wrote the manuscript. JQ provided technical support and supervision. JG was responsible for investigation, data collection, and validation. MG reviewed and edited the manuscript. All authors have read and agreed to the published version of the manuscript.
Conflict of Interest
The authors declare that the research was conducted in the absence of any commercial or financial relationships that could be construed as a potential conflict of interest.
Publisher’s Note
All claims expressed in this article are solely those of the authors and do not necessarily represent those of their affiliated organizations, or those of the publisher, the editors and the reviewers. Any product that may be evaluated in this article, or claim that may be made by its manufacturer, is not guaranteed or endorsed by the publisher.
1 https://www.wjx.cn/
This research was funded by “Structural adjustment strategy in the tourism endowment industry: a supply-side reform perspective”, grant number 17BJY157 and supported by “the Innovative research project of Southwest Minzu University”, grant number CX2020BS20.
- Abrams D., Hogg M. A. (2006). Social Identifications: A Social Psychology of Intergroup Relations and Group Processes. New York: Routledge. [ Google Scholar ]
- Ajzen I. (2002). Residual effects of past on later behavior: habituation and reasoned action perspectives. Personal. Social Psychology Review 6 107–122. 10.1207/S15327957PSPR0602_02 [ CrossRef ] [ Google Scholar ]
- Bauer R. A. (1960). Consumer Behavior as Risk Taking. London: Harvard university press. [ Google Scholar ]
- Beldona S., Kline S. F., Morrison A. M. (2005). Utilitarian value in the Internet: differences between broadband and narrowband users. J. Travel Touris. Market. 17 63–77. 10.1300/J073v17n02-06 [ CrossRef ] [ Google Scholar ]
- Boguszewicz-Kreft M., Kuczamer-Kłopotowska S., Kozłowski A. (2020). The theory of planned behaviour in medical tourism: international comparison in the young consumer segment. Int. J. Environ. Res. Public Health 17 : 1626 . 10.3390/ijerph17051626 [ PMC free article ] [ PubMed ] [ CrossRef ] [ Google Scholar ]
- Canovi M. (2019). Resistance to agritourism diversification: an analysis of winery owners’ identities. Tourism Manage. Perspect. 32 : 100566 . 10.1016/j.tmp.2019.100566 [ CrossRef ] [ Google Scholar ]
- Chen W., Cao C., Fang X. (2019). Expanding the theory of planned behaviour to reveal urban residents’ pro-environment travel behaviour. Atmosphere 10 : 467 . 10.3390/atmos10080467 [ CrossRef ] [ Google Scholar ]
- Chi C. G., Ekinci Y., Ramkissoon H. (2022). ) Evolving effects of COVID-19 safety precaution expectations, risk avoidance, and socio-demographics factors on customer hesitation toward patronizing restaurants and hotels. J. Hospital. Market. Manage. 31 396–412. [ Google Scholar ]
- Cui F., Liu Y., Chang Y. (2016). An overview of tourism risk perception. J. Int. Soc. Prevent. Mitigat. Nat. Hazards 82 643–658. 10.1007/s11069-016-2208-1 [ CrossRef ] [ Google Scholar ]
- Deutsch M., Krauss R. M. (1965). Social Psychology. New York: Basic Books. [ Google Scholar ]
- Drezner Z., Turel O., Zerom D. (2010). A modified Kolmogorov-Smirnov test for normality. Commun. Stat. Simul. Comput. 39 693–704. [ Google Scholar ]
- Duan W. T., Jiang G. R. (2008). A Review of the Theory of Planned Behavior. Adv. Psychol. Sci. 16 315–320. [ Google Scholar ]
- Eisinga R., Te Grotenhuis M., Pelzer B. (2013). The reliability of a two-item scale: pearson, Cronbach, or Spearman-Brown? Int. J. Public Health 58 637–642. 10.1007/s00038-012-0416-3 [ PubMed ] [ CrossRef ] [ Google Scholar ]
- Erikson E. H. (1968). Identity: Youth and Crisis. New York: Norton. [ Google Scholar ]
- Everitt B. S. (1975). Multivariate analysis: the need for data, and other problems. Brit. J. Psychiatr. 126 237–240. 10.1192/bjp.126.3.237 [ PubMed ] [ CrossRef ] [ Google Scholar ]
- Fischer G. W., Morgan M. G., Fischhoff B., et al. (1991). What risks are people concerned about. Risk Anal. 11 303–314. 10.1111/j.1539-6924.1991.tb00606.x [ CrossRef ] [ Google Scholar ]
- Forsyth D. R., van Vugt M., Schlein G. (2015). Identity and sustainability: localized sense of community increases environmental engagement. Anal. Soc. Iss. Public Policy 15 233–252. [ Google Scholar ]
- Fuchs G., Reichel A. (2011). An exploratory inquiry into destination risk perceptions and risk reduction strategies of first time vs. repeat visitors to a highly volatile destination. Touris. Manage. 32 266–276. 10.1016/j.tourman.2010.01.012 [ CrossRef ] [ Google Scholar ]
- Gieling J., Ong C. E. (2016). Warfare past travel experiences and national identity: the case of Airborne Museum ‘Hartenstein’in Oosterbeek, the Netherlands. Touris. Manage. 57 45–55. 10.1016/j.tourman.2016.05.017 [ CrossRef ] [ Google Scholar ]
- Gim Chung Ruth H., Bryan S. K., Kim Jose M., et al. (2004). Asian American multidimensional acculturation scale: development, factor analysis, reliability, and validity. Cult. Divers. Ethn. Minor. Psychol. 10 66–80. 10.1037/1099-9809.10.1.66 [ PubMed ] [ CrossRef ] [ Google Scholar ]
- Hadar L., Sood S. (2014). When knowledge is demotivating: subjective knowledge and choice overload. Psychol. Sci. 25 1739–1747. 10.1177/0956797614539165 [ PubMed ] [ CrossRef ] [ Google Scholar ]
- Hajibaba H., Karlsson L., Dolnicar S. (2017). Residents open their homes to tourists when disaster strikes. J. Travel Res. 56 1065–1078. [ Google Scholar ]
- Hammond K., McWilliam G., Diaz A. N. (1998). Fun and work on the web: differences in attitudes between novices and experienced users. ACR North Am. Adv. 25 372–378. [ Google Scholar ]
- Hauke J., Kossowski T. (2011). Comparison of values of Pearson’s and Spearman’s correlation coefficient on the same sets of data. Quaestiones Geographicae 30 87–93. 10.2478/v10117-011-0021-1 [ CrossRef ] [ Google Scholar ]
- Hu H. F., Krishen A. S. (2019). When is enough, enough? Investigating product reviews and information overload from a consumer empowerment perspective. J. Bus. Res. 100 27–37. 10.1016/j.jbusres.2019.03.011 [ CrossRef ] [ Google Scholar ]
- Hu X., Wu N., Chen N. (2021). Young people’s behavioral intentions towards low-carbon travel: extending the theory of planned behavior. Int. J. Environ. Res. Public Health 18 : 2327 . 10.3390/ijerph18052327 [ PMC free article ] [ PubMed ] [ CrossRef ] [ Google Scholar ]
- Jiang X., Qin J., Gao J. (2022). The mediation of perceived risk’s impact on destination image and travel intention: an empirical study of Chengdu, China during COVID-19. PLoS One 17 : e0261851 . 10.1371/journal.pone.0261851 [ PMC free article ] [ PubMed ] [ CrossRef ] [ Google Scholar ]
- Joo Y., Seok H., Nam Y. (2020). The moderating effect of social media use on sustainable rural tourism: a theory of planned behavior model. Sustainability 12 : 4095 . 10.3390/su12104095 [ CrossRef ] [ Google Scholar ]
- Jöreskog K. G., Sörbom D. (1996). LISREL 8: User’s Reference Guide. Chicago: Scientific Software International. [ Google Scholar ]
- Kaiser H. F. (1974). An index of factorial simplicity. Psychometrika 39 31–36. 10.1007/BF02291575 [ CrossRef ] [ Google Scholar ]
- Khan M. J., Chelliah S., Ahmed S. (2019). Intention to visit India among potential travelers: role of travel motivation, perceived travel risks, and travel constraints. Touris. Hospital. Res. 19 351–367. [ Google Scholar ]
- Kim H., Chen J. S. (2021). Memorable past travel experiences: recollection vs belief. Touris. Recreat. Res. 46 124–131. [ Google Scholar ]
- Lee T. H., Jan F. H. (2018). Ecotourism behavior of nature-based tourists: an integrative framework. J. Travel Res. 57 792–810. [ Google Scholar ]
- Lepp A., Gibson H. (2003). Tourist roles, perceived risk and international tourism. Ann. Touris. Res. 30 606–624. 10.1016/S0160-7383(03)00024-0 [ CrossRef ] [ Google Scholar ]
- Li Z., Zhang S., Liu X. (2020). Seeing the invisible hand: underlying effects of COVID-19 on tourists’ behavioral patterns. J. Destinat. Market. Manage. 18 : 100502 . 10.1016/j.jdmm.2020.100502 [ CrossRef ] [ Google Scholar ]
- Murodjon R., Husanjon J., Youngjoo A. (2021). Destination attractiveness and memorable past travel experiences in silk road tourism in Uzbekistan. Sustainability 13 : 2252 . 10.3390/su13042252 [ CrossRef ] [ Google Scholar ]
- Nazneen S., Hong X., Ud Din N. (2020). COVID-19 Crises and Tourist Travel Risk Perceptions. Rochester: Social Science Electronic Publishing, 10.2139/ssrn.3592321 [ CrossRef ] [ Google Scholar ]
- Park J. Y., Jang S. C. S. (2013). Confused by too many choices? Choice overload in tourism. Touris. Manage. 35 1–12. 10.1016/j.tourman.2012.05.004 [ CrossRef ] [ Google Scholar ]
- Quintal V. A., Lee J. A., Soutar G. N. (2010). Risk, uncertainty and the theory of planned behavior: a tourism example. Touris. Manage. 31 797–805. 10.1016/j.tourman.2009.08.006 [ CrossRef ] [ Google Scholar ]
- Ramkissoon H., Mavondo F. T. (2015). The satisfaction–place attachment relationship: potential mediators and moderators. J. Bus. Res. 68 2593–2602. 10.1016/j.jbusres.2015.05.002 [ CrossRef ] [ Google Scholar ]
- Reisinger Y., Mavondo F. (2005). Travel anxiety and intentions to travel internationally: implications of travel risk perception. J. Travel Res. 43 212–225. 10.1177/0047287504272017 [ CrossRef ] [ Google Scholar ]
- Roehl W. S., Fesenmaier D. R. (1992). Risk perceptions and pleasure travel: an exploratory analysis. J. Travel Res. 30 17–26. 10.1177/004728759203000403 [ CrossRef ] [ Google Scholar ]
- Sánchez-Cañizares S. M., Cabeza-Ramírez L. J., Muñoz-Fernández G. (2021). Impact of the perceived risk from Covid-19 on intention to travel. Curr. Iss. Touris. 24 970–984. 10.1080/13683500.2020.1829571 [ CrossRef ] [ Google Scholar ]
- Sharifpour M., Walters G., Ritchie B. W. (2014). Risk perception, prior knowledge, and willingness to travel: investigating the Australian tourist market’s risk perceptions towards the Middle East. J. Vacat. Market. 20 111–123. 10.1177/1356766713502486 [ CrossRef ] [ Google Scholar ]
- Sönmez S. F., Graefe A. R. (1998). Determining future travel behavior from past travel experience and perceptions of risk and safety. J. Travel Res. 37 171–177. 10.1177/004728759803700209 [ CrossRef ] [ Google Scholar ]
- Tajfel H. (1982). Social psychology of intergroup relations. Ann. Rev. Psychol. 33 1–39. 10.1146/annurev.ps.33.020182.000245 [ CrossRef ] [ Google Scholar ]
- Tassiello V., Tillotson J. S. (2020). How subjective knowledge influences intention to travel. Ann. Touris. Res. 80 : 102851 . 10.1016/j.annals.2019.102851 [ CrossRef ] [ Google Scholar ]
- Vespestad M. K., Lindberg F., Mossberg L. (2019). Value in tourist experiences: how nature-based experiential styles influence value in climbing. Tourist. Stud. 19 453–474. 10.1177/1468797619837966 [ CrossRef ] [ Google Scholar ]
- Wang X. Y., Cheng X. Y. (2018). The application of knowledge-belief-action theory in the improvement of medical humanistic literacy of doctors. Manage. Observ. 08 164–165. [ Google Scholar ]
- Yamane T. (1967). Statistics: An Introductory Analysis (2nd ed.). New York, NY: Harper and Row. [ Google Scholar ]
- Zhang C. X., Wang L., Rickly J. M. (2021). Non-interaction and identity change in Covid-19 tourism. Ann. Tourism Res. 89 : 103211 . 10.1016/j.annals.2021.103211 [ PMC free article ] [ PubMed ] [ CrossRef ] [ Google Scholar ]
- Zhu H., Deng F. (2020). How to influence rural tourism intention by risk knowledge during COVID-19 containment in China: mediating role of risk perception and attitude. Int. J. Environ. Res. Public Health 17 : 3514 . 10.3390/ijerph17103514 [ PMC free article ] [ PubMed ] [ CrossRef ] [ Google Scholar ]
Academia.edu no longer supports Internet Explorer.
To browse Academia.edu and the wider internet faster and more securely, please take a few seconds to upgrade your browser .
Enter the email address you signed up with and we'll email you a reset link.
- We're Hiring!
- Help Center
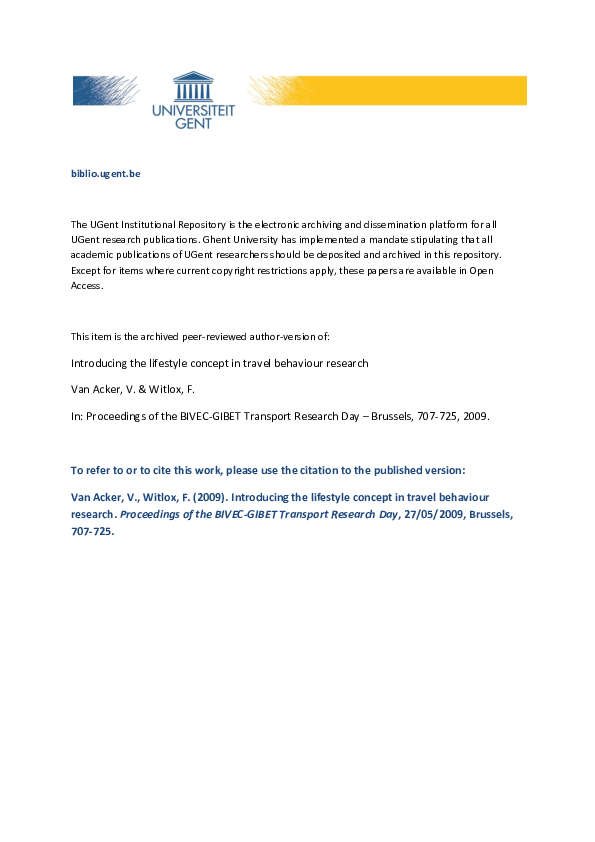
Introducing the lifestyle concept in travel behaviour research
This paper considers the complex relationships between different lifestyles, the built environment, stage in life, car availability and travel behaviour by means of structural equation modelling. The analyses are based on 2007 data from an Internet survey in Flanders, Belgium. The sample is overrepresented by highly-educated respondents, but different travel patterns can still be found within this homogenous group. This is (partly) due to lifestyles. While controlling for residential selfselection and mediating variables such as car availability, the results indicate that lifestyles significantly influence modal choice for shopping trips, social visits and leisure trips. The built environment also has the expected effect on modal choice: car use is lower among respondents living in neighbourhoods closely located to a local or regional centre, with high density and good local accessibility. The influence of lifestyle on modal choice is, however, not always that strong compared to the...
Related Papers
Transport Policy
Patricia L Mokhtarian
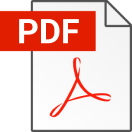
Martin Dijst
International Journal of Sustainable Transportation
Veronique Van Acker
Transportation Research Record: Journal of the Transportation Research Board
Edward Waygood
Urban Studies
Many countries now have policies to reduce distances travelled by private car and to favour the use of public transport, cycling and walking. The development of compact urban forms and the design of urban communities which favour walking are seen as particularly effective strategies for reducing car dependency. The factors which determine travel behaviour are not fully understood, so that effective policies influencing travel patterns are difficult to formulate. Apart from urban form and design, personal attributes and circumstances have an impact on modal choice and distances travelled. People with higher incomes are more likely to own and use a private car than low-income households. Families with children use cars more often than one-person households. The purpose of a trip-work, shopping and leisure—also influences travel mode and distance. We used the Netherlands National Travel Survey (OVG) to explore some of these relationships in more depth. The relative importance of person...
The concept of lifestyle adds a behavioural component to travel models that used to be dominated by engineering and econometric traditions. This paper presents an overview of how lifestyle is defined and measured in transport studies, and how travel behaviour is influenced by lifestyles. Lifestyles are often are often used pragmatically rather than theoretically in the behaviour studies. Nevertheless, some important theoretical contributions have been made, especially in sociology by scholars such as Weber, Bourdieu, Ganzeboom and Schulz who agree on the communicative character of lifestyles: individuals express their social position through specific patterns of behaviour, consumption and leisure. These behavioural patterns are shaped by underlying opinions and orientations, including beliefs, interests and attitudes. Thus, travel behaviour is not simply determined by price, speed and comfort but is also related to attitudes, status and preferences. Because lifestyle has many differ...
Studies that model the effects of the built environ me t on travel behavior are well represented in the literature. Usually, these models are controlled for socio-economic differences among respondents, and sometimes take i nto account personality traits (such as perceptions, attitudes and lifestyles). However, fa r less is know about the conceptual relationship that exists between spatial, socio-eco nomic and personality characteristics on the one hand and travel behavior on the other. Answerin g this query involves combining and linking theories stemming from transport geography (e.g., utility-maximizing theory, activitybased approach) and social psychology (e.g., theory of planned behavior, theory of repeated behavior). Using key-variables from these theories, this paper aims to develop a new conceptual model for travel behavior. This conceptu al model brings together concepts such as ‘perceptions’, ‘attitudes’, ‘preferences’ and ‘life style’. Furthermore, travel behavior is cons...
Transportation Research Record
Martin Lanzendorf
... availability of number of cars in house-hold, subscription to public transport) and workday use of trans-port modes (car use on last workday, public transport use on last workday); urban form elements (residential neighborhood, garden ownership, allotment, second home ...
European Journal of Transport and Infrastructure Research
Xiong Yubing
RELATED PAPERS
Luciano Floridi
Antonio De Santiago Perez
Wayan Rentanu
Cristina Soraci , Andoni Llamazares , Werner Eck , Mela Albana
Há espaço para as músicas indígenas em um Brasil multicultural? - a inserção do repertório indígena na educação musical
Magda D . Pucci
IJCIRAS Research Publication
Palabra Clave
José Octavio Islas Carmona
azimah abdullah
Nile Magazine 14
Traugott Huber
Filip Świerczyński
Linguistic Typology at the Crossroads
Guglielmo Inglese
JERITH ALEXANDRA CRUZ ARDILA
FLOR ALMARAZ
Molecular Psychiatry
Kuan-pin Su
Acta scientific dental sciences
Shruti Dabi
Contact Dermatitis
Fatima Pereira
Tạp chí Y học Việt Nam
Hoàng Minh Đức
sellertintaprinter
seller tintaprinter5
原版复制英国斯旺西大学 swansea毕业证硕士学历托业成绩单原版一模一样
Perceptual and Motor Skills
Christina Paul
hgkgf ehyrfg
Biochemistry
John R Coggins
International Journal of Engineering …
usman hamza
RELATED TOPICS
- We're Hiring!
- Help Center
- Find new research papers in:
- Health Sciences
- Earth Sciences
- Cognitive Science
- Mathematics
- Computer Science
- Academia ©2024

IMAGES
VIDEO
COMMENTS
Travel behavior refers to the allocation of resources, such as time and money, to activities and movements from one place to another. It encompasses the combination of activity participation and travel, which are influenced by motivations, emotions, and beliefs. Understanding travel behavior involves studying the evolution and adaptation of ...
The family influence on buying behavior extends to travel decisions as well, often shaping our preferences from a young age. The World Around Us: Environmental and Situational Influences. As much as our internal factors drive our travel behavior, the external world plays an equally important role.
The m ain scope of travel behaviour research is the measurement, analysis, modelling. and forecasting of the travellers' schedules, that is, the volume, structure and characteristics of. the ...
By considering behaviour as not just an outcome of other constructs, but also as an important predictor, a cyclical process emerges. In the following section, we introduce such a process - inspired by the above theories - including attitude, desire, intention, behaviour, and satisfaction. 4. The travel mode choice cycle.
Travel behavior is a multidimensional concept in which choice. of mode of transport is often given most prominence, but it. also includes the numbers and frequency of trips made, desti-
Purpose of Review This paper reviews the use of conceptual frameworks in research on active travel, such as walking and cycling. Generic framework features and a wide range of contents are identified and synthesized into a comprehensive framework of active travel behavior, as part of the Physical Activity through Sustainable Transport Approaches project (PASTA). PASTA is a European ...
The existing body of research on travel behaviour can therefore best be seen as fragmented due to: (1) individual studies replicating one or a few CB concepts borrowed from marketing and general management fields and applying them to tourism; (2) many studies investigate the same effect (e.g. satisfaction → loyalty), but the results cannot be ...
Travel behaviour change is a significantly growing field of research which has resulted in a diverse range of theories, behaviours and tools being studied. Consequently, a wide-angled review is critical for synthesising knowledge in this field, to develop evidence for this approach and proposing future research directions. 1.1.
Concepts of Travel Behavior Research. In the first part this chapter proposes a conceptual framework for travel behavior research through a definition of the scope of the research topic, essentially human activity schedules, and a conceptualization of the traveler as a network actor negotiating infrastructure and human networks and dealing with the social content of the activities undertaken.
Concepts of Travel Behaviour Research. Kay W. Axhausen (Institute of Transport Planning, Swiss Federal Institute of Technology, Zürich, Switzerland) Threats from Car Traffic to the Quality of Urban Life. ISBN: 978--08-044853-4, eISBN: 978--08-048144-9. Publication date: 12 April 2007. Acknowledgements ...
Travel behavior is hypothesized to be influenced significantly by both self-congruity and functional congruity. Self-congruity is the match between the destination visitor image and tourists' self-concept (actual, ideal, social, and ideal social self-image).
This finding reveals that sustainable tourist behavior is a dynamic concept requiring an innovative and adaptive approach in future research. Over 50 theories and models have been used by scholars to investigate sustainable tourist behavior, along with over 150 variables influencing sustainable tourist behavior directly or indirectly.
Chapter 2 Travel Behavior Theories. Two different perspectives, individual and collective, can explain travel behavior. When people contextualizing travel as a personal choice or decision-making, the traveler as a subject make mode choices, driving or not. ... While 'decision' is a broader concept. A decision could be a schedule with a ...
theoretically arises as a natural evolution of research on human behavior, in general, and travel behavior, in particular (McNally, 2000b). This paper offers a brief review of the concept of travel behavior analysis in order to provide a better understanding and forecasting of travel behavior. The article further
A complementary psychological concept to the TPB is that of habit. It assumes that instead of consciously planning journeys, the commuter has developed a routine, for example habitually choosing to use the car parked outside the house every day without reflection. ... Moreover, travel behaviour may be influenced by the interplay of ...
such reviews in the broader context of travel or tourist behaviour. For example, concepts, influences and research contexts can be studied for a specific travel stage (pre-visit, on-site and post-visit) in the visitation process (e.g. Frias, Rodriguez, & Castaneda, 2008, on pre-visit factors in the formation of destination image).
H13: Past travel experience moderates the impact of (a) knowledge, (b) tourism self-identity, and (c) perceived risk on perceived behavioral control. indicate past travel experience as an important factor affecting tourists' perceived risk. Likewise, find differences in risk perception between first-time and repeat visitors.
Despite the wide use of travel motivation as a concept in tourist behaviour studies, the development of travel motivation theories has been limited (Hsu & Huang, 2008). Nonetheless, the socio ...
The concept of lifestyle adds a behavioral component to travel models that used to be dominated by engineering and econometric traditions. This article presents an overview of how lifestyle is defined and measured in transport studies, and how travel behavior is influenced by lifestyles.
The concept of lifestyle adds a behavioural component to travel models that used to be dominated by engineering and econometric traditions. This paper presents an overview of how lifestyle is defined and measured in transport studies, and how travel behaviour is influenced by lifestyles.
The concept of constraints can also serve as a device to assist in perceiving new connections to overcoming these constraints (Jackson & Scott, 1999). Research on leisure constraints has existed as a distinct subfield of investigation within leisure studies for a little over two decades. ... The strongest predictor of travel behavior was ...
Kevin Manaugh, Ahmed M. El-Geneidy, What makes travel 'local', Journal of Transport and Land Use, Vol. 5, No. 3 (2012), pp. 15-27
The concept of the pre-stressed stayed columns in civil engineering application are used in this work to solve the instability problem of the slender lattice [6, 7]. It was shown that by introducing stays, the ultimate load of the structure can be significantly increased.
1. Introduction. The study of ways to improve quality of life is of keen interest to researchers and policymakers across various disciplines, including travel, tourism, health, sociology, and psychology (Zhang, 2017).Both travel behavior (De Vos, 2019) and tourism (Smith & Diekmann, 2017) literature acknowledge the respective roles of daily travel or commute experiences and tourism activities ...