Traveling salesman problem
This web page is a duplicate of https://optimization.mccormick.northwestern.edu/index.php/Traveling_salesman_problems
Author: Jessica Yu (ChE 345 Spring 2014)
Steward: Dajun Yue, Fengqi You
The traveling salesman problem (TSP) is a widely studied combinatorial optimization problem, which, given a set of cities and a cost to travel from one city to another, seeks to identify the tour that will allow a salesman to visit each city only once, starting and ending in the same city, at the minimum cost. 1
- 2.1 Graph Theory
- 2.2 Classifications of the TSP
- 2.3 Variations of the TSP
- 3.1 aTSP ILP Formulation
- 3.2 sTSP ILP Formulation
- 4.1 Exact algorithms
- 4.2.1 Tour construction procedures
- 4.2.2 Tour improvement procedures
- 5 Applications
- 7 References
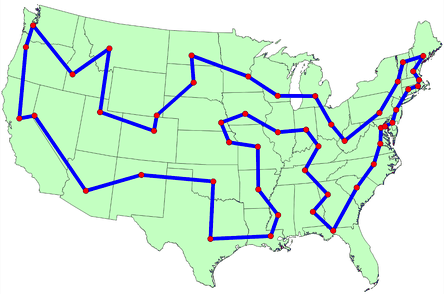
The origins of the traveling salesman problem are obscure; it is mentioned in an 1832 manual for traveling salesman, which included example tours of 45 German cities but gave no mathematical consideration. 2 W. R. Hamilton and Thomas Kirkman devised mathematical formulations of the problem in the 1800s. 2
It is believed that the general form was first studied by Karl Menger in Vienna and Harvard in the 1930s. 2,3
Hassler Whitney, who was working on his Ph.D. research at Harvard when Menger was a visiting lecturer, is believed to have posed the problem of finding the shortest route between the 48 states of the United States during either his 1931-1932 or 1934 seminar talks. 2 There is also uncertainty surrounding the individual who coined the name “traveling salesman problem” for Whitney’s problem. 2
The problem became increasingly popular in the 1950s and 1960s. Notably, George Dantzig, Delber R. Fulkerson, and Selmer M. Johnson at the RAND Corporation in Santa Monica, California solved the 48 state problem by formulating it as a linear programming problem. 2 The methods described in the paper set the foundation for future work in combinatorial optimization, especially highlighting the importance of cutting planes. 2,4
In the early 1970s, the concept of P vs. NP problems created buzz in the theoretical computer science community. In 1972, Richard Karp demonstrated that the Hamiltonian cycle problem was NP-complete, implying that the traveling salesman problem was NP-hard. 4
Increasingly sophisticated codes led to rapid increases in the sizes of the traveling salesman problems solved. Dantzig, Fulkerson, and Johnson had solved a 48 city instance of the problem in 1954. 5 Martin Grötechel more than doubled this 23 years later, solving a 120 city instance in 1977. 5 Enoch Crowder and Manfred W. Padberg again more than doubled this in just 3 years, with a 318 city solution. 5
In 1987, rapid improvements were made, culminating in a 2,392 city solution by Padberg and Giovanni Rinaldi. In the following two decades, David L. Appelgate, Robert E. Bixby, Vasek Chvátal, & William J. Cook led the cutting edge, solving a 7,397 city instance in 1994 up to the current largest solved problem of 24,978 cities in 2004. 5

Description
Graph theory.
In the context of the traveling salesman problem, the verticies correspond to cities and the edges correspond to the path between those cities. When modeled as a complete graph, paths that do not exist between cities can be modeled as edges of very large cost without loss of generality. 6 Minimizing the sum of the costs for Hamiltonian cycle is equivalent to identifying the shortest path in which each city is visiting only once.
Classifications of the TSP
The TRP can be divided into two classes depending on the nature of the cost matrix. 3,6
- Applies when the distance between cities is the same in both directions
- Applies when there are differences in distances (e.g. one-way streets)
An ATSP can be formulated as an STSP by doubling the number of nodes. 6
Variations of the TSP
Formulation
The objective function is then given by
To ensure that the result is a valid tour, several contraints must be added. 1,3
There are several other formulations for the subtour elimnation contraint, including circuit packing contraints, MTZ constraints, and network flow constraints.
aTSP ILP Formulation
The integer linear programming formulation for an aTSP is given by
sTSP ILP Formulation
The symmetric case is a special case of the asymmetric case and the above formulation is valid. 3, 6 The integer linear programming formulation for an sTSP is given by
Exact algorithms
Branch-and-bound algorithms are commonly used to find solutions for TSPs. 7 The ILP is first relaxed and solved as an LP using the Simplex method, then feasibility is regained by enumeration of the integer variables. 7
Other exact solution methods include the cutting plane method and branch-and-cut. 8
Heuristic algorithms
Given that the TSP is an NP-hard problem, heuristic algorithms are commonly used to give a approximate solutions that are good, though not necessarily optimal. The algorithms do not guarantee an optimal solution, but gives near-optimal solutions in reasonable computational time. 3 The Held-Karp lower bound can be calculated and used to judge the performance of a heuristic algorithm. 3
There are two general heuristic classifications 7 :
- Tour construction procedures where a solution is gradually built by adding a new vertex at each step
- Tour improvement procedures where a feasbile solution is improved upon by performing various exchanges
The best methods tend to be composite algorithms that combine these features. 7
Tour construction procedures
Tour improvement procedures
Applications
The importance of the traveling salesman problem is two fold. First its ubiquity as a platform for the study of general methods than can then be applied to a variety of other discrete optimization problems. 5 Second is its diverse range of applications, in fields including mathematics, computer science, genetics, and engineering. 5,6
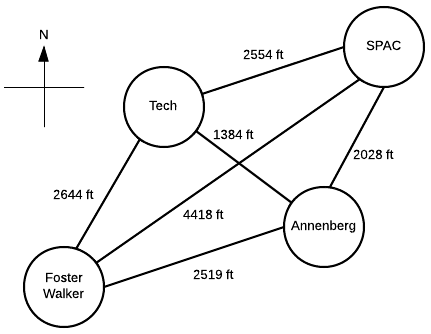
Suppose a Northwestern student, who lives in Foster-Walker , has to accomplish the following tasks:
- Drop off a homework set at Tech
- Work out a SPAC
- Complete a group project at Annenberg
Distances between buildings can be found using Google Maps. Note that there is particularly strong western wind and walking east takes 1.5 times as long.
It is the middle of winter and the student wants to spend the least possible time walking. Determine the path the student should take in order to minimize walking time, starting and ending at Foster-Walker.
Start with the cost matrix (with altered distances taken into account):
Method 1: Complete Enumeration
All possible paths are considered and the path of least cost is the optimal solution. Note that this method is only feasible given the small size of the problem.
From inspection, we see that Path 4 is the shortest. So, the student should walk 2.28 miles in the following order: Foster-Walker → Annenberg → SPAC → Tech → Foster-Walker
Method 2: Nearest neighbor
Starting from Foster-Walker, the next building is simply the closest building that has not yet been visited. With only four nodes, this can be done by inspection:
- Smallest distance is from Foster-Walker is to Annenberg
- Smallest distance from Annenberg is to Tech
- Smallest distance from Tech is to Annenberg ( creates a subtour, therefore skip )
- Next smallest distance from Tech is to Foster-Walker ( creates a subtour, therefore skip )
- Next smallest distance from Tech is to SPAC
- Smallest distance from SPAC is to Annenberg ( creates a subtour, therefore skip )
- Next smallest distance from SPAC is to Tech ( creates a subtour, therefore skip )
- Next smallest distance from SPAC is to Foster-Walker
So, the student would walk 2.54 miles in the following order: Foster-Walker → Annenberg → Tech → SPAC → Foster-Walker
Method 3: Greedy
With this method, the shortest paths that do not create a subtour are selected until a complete tour is created.
- Smallest distance is Annenberg → Tech
- Next smallest is SPAC → Annenberg
- Next smallest is Tech → Annenberg ( creates a subtour, therefore skip )
- Next smallest is Anneberg → Foster-Walker ( creates a subtour, therefore skip )
- Next smallest is SPAC → Tech ( creates a subtour, therefore skip )
- Next smallest is Tech → Foster-Walker
- Next smallest is Annenberg → SPAC ( creates a subtour, therefore skip )
- Next smallest is Foster-Walker → Annenberg ( creates a subtour, therefore skip )
- Next smallest is Tech → SPAC ( creates a subtour, therefore skip )
- Next smallest is Foster-Walker → Tech ( creates a subtour, therefore skip )
- Next smallest is SPAC → Foster-Walker ( creates a subtour, therefore skip )
- Next smallest is Foster-Walker → SPAC
So, the student would walk 2.40 miles in the following order: Foster-Walker → SPAC → Annenberg → Tech → Foster-Walker
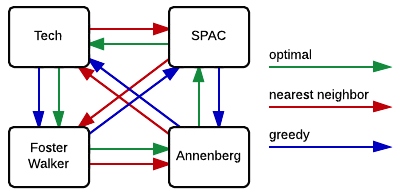
As we can see in the figure to the right, the heuristic methods did not give the optimal solution. That is not to say that heuristics can never give the optimal solution, just that it is not guaranteed.
Both the optimal and the nearest neighbor algorithms suggest that Annenberg is the optimal first building to visit. However, the optimal solution then goes to SPAC, while both heuristic methods suggest Tech. This is in part due to the large cost of SPAC → Foster-Walker. The heuristic algorithms cannot take this future cost into account, and therefore fall into that local optimum.
We note that the nearest neighbor and greedy algorithms give solutions that are 11.4% and 5.3%, respectively, above the optimal solution. In the scale of this problem, this corresponds to fractions of a mile. We also note that neither heuristic gave the worst case result, Foster-Walker → SPAC → Tech → Annenberg → Foster-Walker.
Only tour building heuristics were used. Combined with a tour improvement algorithm (such as 2-opt or simulated annealing), we imagine that we may be able to locate solutions that are closer to the optimum.
The exact algorithm used was complete enumeration, but we note that this is impractical even for 7 nodes (6! or 720 different possibilities). Commonly, the problem would be formulated and solved as an ILP to obtain exact solutions.
- Vanderbei, R. J. (2001). Linear programming: Foundations and extensions (2nd ed.). Boston: Kluwer Academic.
- Schrijver, A. (n.d.). On the history of combinatorial optimization (till 1960).
- Matai, R., Singh, S., & Lal, M. (2010). Traveling salesman problem: An overview of applications, formulations, and solution approaches. In D. Davendra (Ed.), Traveling Salesman Problem, Theory and Applications . InTech.
- Junger, M., Liebling, T., Naddef, D., Nemhauser, G., Pulleyblank, W., Reinelt, G., Rinaldi, G., & Wolsey, L. (Eds.). (2009). 50 years of integer programming, 1958-2008: The early years and state-of-the-art surveys . Heidelberg: Springer.
- Cook, W. (2007). History of the TSP. The Traveling Salesman Problem . Retrieved from http://www.math.uwaterloo.ca/tsp/history/index.htm
- Punnen, A. P. (2002). The traveling salesman problem: Applications, formulations and variations. In G. Gutin & A. P. Punnen (Eds.), The Traveling Salesman Problem and its Variations . Netherlands: Kluwer Academic Publishers.
- Laporte, G. (1992). The traveling salesman problem: An overview of exact and approximate algorithms. European Journal of Operational Research, 59 (2), 231–247.
- Goyal, S. (n.d.). A suvey on travlling salesman problem.
Navigation menu
Traveling Salesman Problem
- Reference work entry
- pp 3935–3944
- Cite this reference work entry
- Gregory Gutin 3
653 Accesses
3 Citations
Article Outline
Keywords and Phrases
Introduction
Basic Definitions and Notation
Computational Complexity
Formulations
Applications
Exact Algorithms
This is a preview of subscription content, log in via an institution to check access.
Access this chapter
- Available as PDF
- Read on any device
- Instant download
- Own it forever
- Durable hardcover edition
- Dispatched in 3 to 5 business days
- Free shipping worldwide - see info
Tax calculation will be finalised at checkout
Purchases are for personal use only
Institutional subscriptions
Arora S (1998) Polynomial-time approximation schemes for Euclidean TSP and other geometric problems. J ACM 45:753–782
Article MATH MathSciNet Google Scholar
Arora S (2002) Approximation algorithms for geometric TSP. In: Gutin G, Punnen AP (eds) The Traveling Salesman Problem and its Variations. Kluwer, Dordrecht, pp 207–221
Google Scholar
Bang-Jensen J, Gutin G (2000) Digraphs: Theory, Algorithms and Applications. Springer, London
Blaeser M, Manthey B, Sgall J (2006) An Improved Approximation Algorithm for the Asymmetric TSP with Strengthened Triangle Inequality. J Discret Algorithms 4:623–632
Article MATH Google Scholar
Burkard RE, Deineko VG, van Dal R, van der Veen JAA, Woeginger GJ (1998) Well-solvable special cases of the traveling salesman problem: a survey. SIAM Rev 40:496–546
Baum EB (1986) Iterated descent: A better algorithm for local search in combinatorial optimization problems. unpublished manuscript
Chekuri C, Goldberg A, Karger D, Levine M, Stein C (1997) Experimental Study of Minimum Cut Algorithms. In: Proc. 8th ACM-SIAM Symp. Discrete Algorithms (SODA'97). ACM/SIAM, pp 324–333
Christofides N (1976) Worst-case analysis of a new heuristic for the traveling salesman problem. Technical Report CS-93–13, Carnegie Mellon University
Clarke G, Wright JW (1964) Scheduling of vehicles from a central depot to a number of delivery points. Oper Res 12:568–581
Dantzig GB, Fulkerson DR, Johnson SM (1954) Solution of large scale traveling salesman problem. Oper Res 2:393–410
MathSciNet Google Scholar
Fischetti M, Lodi A, Toth P (2002) Exact Methods for the Asymmetric Traveling Salesman Problem. In: Gutin G, Punnen AP (eds) The Traveling Salesman Problem and its Variations. Kluwer, Dordrecht, pp 169–205
Garey MR, Graham RL, Johnson DS (1976) Some NP-complete geometric problems. In: Proc. 8th ACM Symp. Theory Comput. ACM, pp 10–22
Glover F, Gutin G, Yeo A, Zverovich A (2001) Construction heuristics for the asymmetric TSP. Eur J Oper Res 129:555–568
Golden BL, Stewart WR (1985) Empirical Analysis of Heuristics. In: Lawler EL, Lenstra JK, Rinnooy Kan AHG, Shmoys DB (eds) The Traveling Salesman Problem: A Guided Tour of Combinatorial Optimization. Wiley, New York, pp 207–249
Gutin G, Jakubowicz H, Ronen S, Zverovitch A (2005) Seismic vessel problem. Commun DQM 8:13–20
Gutin G, Koller A, Yeo A (2006) Note on Upper Bounds for TSP Domination Number. Algorithmic Oper Res 1:52–54
MATH MathSciNet Google Scholar
Gutin G, Yeo A, Zverovich A (2002) Traveling salesman should not be greedy: domination analysis of greedy-type heuristics for the TSP. Discrete Appl Math 117:81–86
Hassin R, Khuller S (2001) z‑Approximations. J Algorithms 41:429–442
Held M, Karp RM (1962) A dynamic programming approach to sequencing problems. J Soc Ind Appl Math 10:196–210
Hoffman AJ, Wolfe P (1985) History. In: Lawler EL, Lenstra JK, Rinnooy Kan AHG, Shmoys DB (eds) The Traveling Salesman Problem: A Guided Tour of Combinatorial Optimization. Wiley, New York, pp 1–16
Johnson DS, Gutin G, McGeoch LA, Yeo A, Zhang W, Zverovitch A (2002) Experimental Analysis of Heuristics for ATSP. In: Gutin G, Punnen AP (eds) The Traveling Salesman Problem and its Variations. Kluwer, Dordrecht
Johnson DS, McGeoch LA (2002) Experimental Analysis of Heuristics for STSP. In: Gutin G, Punnen AP (eds) The Traveling Salesman Problem and its Variations. Kluwer, Dordrecht, pp 369–443
Johnson DS, Papadimitriou CH (1985) Performance guarantees for heuristics. In: Lawler EL, Lenstra JK, Rinnooy Kan AHG, Shmoys DB (eds) The Traveling Salesman Problem: A Guided Tour of Combinatorial Optimization. Wiley, New York, pp 145–180
Jonker R, Volgenant T (1983) Transforming asymmetric into symmetric traveling salesman problems. Oper Res Lett 2:161–163
Jünger M, Rinaldi G, Thienel S (2000) Practical Performance of efficient minimum Cut Algorithms. Algorithmica 26:172–195
Kabadi SN (2002) Polynomially solvable cases of the TSP. In: Gutin G, Punnen AP (eds) The Traveling Salesman Problem and its Variations. Kluwer, Dordrecht, pp 489–583
Kaplan H, Lewenstein M, Shafrir N, Sviridenko M (2005) Approximation Algorithms for the Asymmetric TSP by Decomposing Regular Multigraphs. J ACM 52:602–626
Article MathSciNet Google Scholar
Karp RM (1972) Reducibility among combinatorial problems. In: Miller RE, Thatcher JW (eds) Complexity of Computer Computations. Plenum Press, New York, pp 85–103
Karp RM, Steele JM (1985) Probabilistic analysis of heuristics. In: Lawler EL, Lenstra JK, Rinnooy Kan AHG, Shmoys DB (eds) The Traveling Salesman Problem: A Guided Tour of Combinatorial Optimization. Wiley, New York, pp 181–205
Lodi A, Punnen AP (2002) TSP Software. In: Gutin G, Punnen AP (eds) The Traveling Salesman Problem and its Variations. Kluwer, Dordrecht, pp 737–749
Menger K (1932) Das Botenproblem. Ergebnisse eines Mathematischen Kolloquiums 2:11–12
Miller CE, Tucker AW, Zemlin RA (1960) Integer Programming formulations and traveling salesman problems. J Assoc Comput Mach 7:326–329
Mitchell JCB (1999) Guillotine subdivisions approximate polygonal subdivisions: A simple polynomial time approximation scheme for geometric TSP, k-MST, and related problem. SIAM J Comput 28:1298–1309
Nagata Y (2006) New EAX crossover for large TSP instances. Lecture Notes Comp Sci 4193:372–381
Article Google Scholar
Nagata Y, Kobayashi S (1997) Edge Assembly Crossover: A High-power Genetic Algorithm for the Traveling Salesman Problem. In: Proc. of the 7th Int. Conference on Genetic Algorithms, pp 450–457
Naddef D (2002) Polyhedral Theory and Branch-and-Cut Algorithms for the Symmetric TSP. In: Gutin G, Punnen AP (eds) The Traveling Salesman Problem and its Variations. Kluwer, Dordrecht, pp 29–116
Padberg MW, Sung TY (1991) An analytical comparison of different formulations of the traveling salesman problem. Math Program Ser A 52:315–357
Papadimitriou CH (1977) The Euclidean traveling salesman problem is NP-complete. Theoret Comput Sci 4:237–244
Papadimitriou CH, Steiglitz K (1982) Combinatorial Optimization. Prentice-Hall, New Jersey
MATH Google Scholar
Punnen AP (2002) The Traveling Salesman Problem: Aplications, Formulations and Variations. In: Gutin G, Punnen AP (eds) The Traveling Salesman Problem and its Variations. Kluwer, Dordrecht, pp 1–28
Punnen AP, Margot F, Kabadi S (2003) TSP heuristics: domination analysis and complexity. Algorithmica 35:111–127
Rao S, Smith W (1998) Approximating geometric graphs via “spanners” and “banyans”. Proc. 30th Ann. ACM Symp. Theory Comput. ACM, pp 540–550
Rego C, Glover F (2002) Local Search and Metaheuristics. In: Gutin G, Punnen AP (eds) The Traveling Salesman Problem and its Variations. Kluwer, Dordrecht, pp 309–368
Rego C, Glover F, Gamboa D, Osterman C: A Doubly-Rooted Stem-and-Cycle Ejection Chain Algorithm for Asymmetric Traveling Salesman Problems. submitted
Trevisan L (1997) When Hamming meets Euclid: the approximability of geometric TSP and MST. In: Proc. 29th ACM Symp. Theory Comput. ACM, pp 21–39
Zemel E (1981) Measuring the quality of approximate solutions to zero-one programming problems. Math Oper Res 6:319–332
Download references
Author information
Authors and affiliations.
Department of Computer Science, Royal Holloway, University of London, Egham, UK
Gregory Gutin
You can also search for this author in PubMed Google Scholar
Editor information
Editors and affiliations.
Department of Chemical Engineering, Princeton University, Princeton, NJ, 08544-5263, USA
Christodoulos A. Floudas
Center for Applied Optimization, Department of Industrial and Systems Engineering, University of Florida, Gainesville, FL, 32611-6595, USA
Panos M. Pardalos
Rights and permissions
Reprints and permissions
Copyright information
© 2008 Springer-Verlag
About this entry
Cite this entry.
Gutin, G. (2008). Traveling Salesman Problem . In: Floudas, C., Pardalos, P. (eds) Encyclopedia of Optimization. Springer, Boston, MA. https://doi.org/10.1007/978-0-387-74759-0_687
Download citation
DOI : https://doi.org/10.1007/978-0-387-74759-0_687
Publisher Name : Springer, Boston, MA
Print ISBN : 978-0-387-74758-3
Online ISBN : 978-0-387-74759-0
eBook Packages : Mathematics and Statistics Reference Module Computer Science and Engineering
Share this entry
Anyone you share the following link with will be able to read this content:
Sorry, a shareable link is not currently available for this article.
Provided by the Springer Nature SharedIt content-sharing initiative
- Publish with us
Policies and ethics
- Find a journal
- Track your research
12.9 Traveling Salesperson Problem
Learning objectives.
After completing this section, you should be able to:
- Distinguish between brute force algorithms and greedy algorithms.
- List all distinct Hamilton cycles of a complete graph.
- Apply brute force method to solve traveling salesperson applications.
- Apply nearest neighbor method to solve traveling salesperson applications.
We looked at Hamilton cycles and paths in the previous sections Hamilton Cycles and Hamilton Paths . In this section, we will analyze Hamilton cycles in complete weighted graphs to find the shortest route to visit a number of locations and return to the starting point. Besides the many routing applications in which we need the shortest distance, there are also applications in which we search for the route that is least expensive or takes the least time. Here are a few less common applications that you can read about on a website set up by the mathematics department at the University of Waterloo in Ontario, Canada:
- Design of fiber optic networks
- Minimizing fuel expenses for repositioning satellites
- Development of semi-conductors for microchips
- A technique for mapping mammalian chromosomes in genome sequencing
Before we look at approaches to solving applications like these, let's discuss the two types of algorithms we will use.
Brute Force and Greedy Algorithms
An algorithm is a sequence of steps that can be used to solve a particular problem. We have solved many problems in this chapter, and the procedures that we used were different types of algorithms. In this section, we will use two common types of algorithms, a brute force algorithm and a greedy algorithm . A brute force algorithm begins by listing every possible solution and applying each one until the best solution is found. A greedy algorithm approaches a problem in stages, making the apparent best choice at each stage, then linking the choices together into an overall solution which may or may not be the best solution.
To understand the difference between these two algorithms, consider the tree diagram in Figure 12.187 . Suppose we want to find the path from left to right with the largest total sum. For example, branch A in the tree diagram has a sum of 10 + 2 + 11 + 13 = 36 10 + 2 + 11 + 13 = 36 .
To be certain that you pick the branch with greatest sum, you could list each sum from each of the different branches:
A : 10 + 2 + 11 + 13 = 36 10 + 2 + 11 + 13 = 36
B : 10 + 2 + 11 + 8 = 31 10 + 2 + 11 + 8 = 31
C : 10 + 2 + 15 + 1 = 28 10 + 2 + 15 + 1 = 28
D : 10 + 2 + 15 + 6 = 33 10 + 2 + 15 + 6 = 33
E : 10 + 7 + 3 + 20 = 40 10 + 7 + 3 + 20 = 40
F : 10 + 7 + 3 + 14 = 34 10 + 7 + 3 + 14 = 34
G : 10 + 7 + 4 + 11 = 32 10 + 7 + 4 + 11 = 32
H : 10 + 7 + 4 + 5 = 26 10 + 7 + 4 + 5 = 26
Then we know with certainty that branch E has the greatest sum.
Now suppose that you wanted to find the branch with the highest value, but you only were shown the tree diagram in phases, one step at a time.
After phase 1, you would have chosen the branch with 10 and 7. So far, you are following the same branch. Let’s look at the next phase.
After phase 2, based on the information you have, you will choose the branch with 10, 7 and 4. Now, you are following a different branch than before, but it is the best choice based on the information you have. Let’s look at the last phase.
After phase 3, you will choose branch G which has a sum of 32.
The process of adding the values on each branch and selecting the highest sum is an example of a brute force algorithm because all options were explored in detail. The process of choosing the branch in phases, based on the best choice at each phase is a greedy algorithm. Although a brute force algorithm gives us the ideal solution, it can take a very long time to implement. Imagine a tree diagram with thousands or even millions of branches. It might not be possible to check all the sums. A greedy algorithm, on the other hand, can be completed in a relatively short time, and generally leads to good solutions, but not necessarily the ideal solution.
Example 12.42
Distinguishing between brute force and greedy algorithms.
A cashier rings up a sale for $4.63 cents in U.S. currency. The customer pays with a $5 bill. The cashier would like to give the customer $0.37 in change using the fewest coins possible. The coins that can be used are quarters ($0.25), dimes ($0.10), nickels ($0.05), and pennies ($0.01). The cashier starts by selecting the coin of highest value less than or equal to $0.37, which is a quarter. This leaves $ 0.37 − $ 0.25 = $ 0.12 $ 0.37 − $ 0.25 = $ 0.12 . The cashier selects the coin of highest value less than or equal to $0.12, which is a dime. This leaves $ 0.12 − $ 0.10 = $ 0.02 $ 0.12 − $ 0.10 = $ 0.02 . The cashier selects the coin of highest value less than or equal to $0.02, which is a penny. This leaves $ 0.02 − $ 0.01 = $ 0.01 $ 0.02 − $ 0.01 = $ 0.01 . The cashier selects the coin of highest value less than or equal to $0.01, which is a penny. This leaves no remainder. The cashier used one quarter, one dime, and two pennies, which is four coins. Use this information to answer the following questions.
- Is the cashier’s approach an example of a greedy algorithm or a brute force algorithm? Explain how you know.
- The cashier’s solution is the best solution. In other words, four is the fewest number of coins possible. Is this consistent with the results of an algorithm of this kind? Explain your reasoning.
- The approach the cashier used is an example of a greedy algorithm, because the problem was approached in phases and the best choice was made at each phase. Also, it is not a brute force algorithm, because the cashier did not attempt to list out all possible combinations of coins to reach this conclusion.
- Yes, it is consistent. A greedy algorithm does not always yield the best result, but sometimes it does.
Your Turn 12.42
The traveling salesperson problem.
Now let’s focus our attention on the graph theory application known as the traveling salesperson problem (TSP) in which we must find the shortest route to visit a number of locations and return to the starting point.
Recall from Hamilton Cycles , the officer in the U.S. Air Force who is stationed at Vandenberg Air Force base and must drive to visit three other California Air Force bases before returning to Vandenberg. The officer needed to visit each base once. We looked at the weighted graph in Figure 12.192 representing the four U.S. Air Force bases: Vandenberg, Edwards, Los Angeles, and Beal and the distances between them.
Any route that visits each base and returns to the start would be a Hamilton cycle on the graph. If the officer wants to travel the shortest distance, this will correspond to a Hamilton cycle of lowest weight. We saw in Table 12.11 that there are six distinct Hamilton cycles (directed cycles) in a complete graph with four vertices, but some lie on the same cycle (undirected cycle) in the graph.
Since the distance between bases is the same in either direction, it does not matter if the officer travels clockwise or counterclockwise. So, there are really only three possible distances as shown in Figure 12.193 .
The possible distances are:
So, a Hamilton cycle of least weight is V → B → E → L → V (or the reverse direction). The officer should travel from Vandenberg to Beal to Edwards, to Los Angeles, and back to Vandenberg.
Finding Weights of All Hamilton Cycles in Complete Graphs
Notice that we listed all of the Hamilton cycles and found their weights when we solved the TSP about the officer from Vandenberg. This is a skill you will need to practice. To make sure you don't miss any, you can calculate the number of possible Hamilton cycles in a complete graph. It is also helpful to know that half of the directed cycles in a complete graph are the same cycle in reverse direction, so, you only have to calculate half the number of possible weights, and the rest are duplicates.
In a complete graph with n n vertices,
- The number of distinct Hamilton cycles is ( n − 1 ) ! ( n − 1 ) ! .
- There are at most ( n − 1 ) ! 2 ( n − 1 ) ! 2 different weights of Hamilton cycles.
TIP! When listing all the distinct Hamilton cycles in a complete graph, you can start them all at any vertex you choose. Remember, the cycle a → b → c → a is the same cycle as b → c → a → b so there is no need to list both.
Example 12.43
Calculating possible weights of hamilton cycles.
Suppose you have a complete weighted graph with vertices N, M, O , and P .
- Use the formula ( n − 1 ) ! ( n − 1 ) ! to calculate the number of distinct Hamilton cycles in the graph.
- Use the formula ( n − 1 ) ! 2 ( n − 1 ) ! 2 to calculate the greatest number of different weights possible for the Hamilton cycles.
- Are all of the distinct Hamilton cycles listed here? How do you know? Cycle 1: N → M → O → P → N Cycle 2: N → M → P → O → N Cycle 3: N → O → M → P → N Cycle 4: N → O → P → M → N Cycle 5: N → P → M → O → N Cycle 6: N → P → O → M → N
- Which pairs of cycles must have the same weights? How do you know?
- There are 4 vertices; so, n = 4 n = 4 . This means there are ( n − 1 ) ! = ( 4 − 1 ) ! = 3 ⋅ 2 ⋅ 1 = 6 ( n − 1 ) ! = ( 4 − 1 ) ! = 3 ⋅ 2 ⋅ 1 = 6 distinct Hamilton cycles beginning at any given vertex.
- Since n = 4 n = 4 , there are ( n − 1 ) ! 2 = ( 4 − 1 ) ! 2 = 6 2 = 3 ( n − 1 ) ! 2 = ( 4 − 1 ) ! 2 = 6 2 = 3 possible weights.
- Yes, they are all distinct cycles and there are 6 of them.
- Cycles 1 and 6 have the same weight, Cycles 2 and 4 have the same weight, and Cycles 3 and 5 have the same weight, because these pairs follow the same route through the graph but in reverse.
TIP! When listing the possible cycles, ignore the vertex where the cycle begins and ends and focus on the ways to arrange the letters that represent the vertices in the middle. Using a systematic approach is best; for example, if you must arrange the letters M, O, and P, first list all those arrangements beginning with M, then beginning with O, and then beginning with P, as we did in Example 12.42.
Your Turn 12.43
The brute force method.
The method we have been using to find a Hamilton cycle of least weight in a complete graph is a brute force algorithm, so it is called the brute force method . The steps in the brute force method are:
Step 1: Calculate the number of distinct Hamilton cycles and the number of possible weights.
Step 2: List all possible Hamilton cycles.
Step 3: Find the weight of each cycle.
Step 4: Identify the Hamilton cycle of lowest weight.
Example 12.44
Applying the brute force method.
On the next assignment, the air force officer must leave from Travis Air Force base, visit Beal, Edwards, and Vandenberg Air Force bases each exactly once and return to Travis Air Force base. There is no need to visit Los Angeles Air Force base. Use Figure 12.194 to find the shortest route.
Step 1: Since there are 4 vertices, there will be ( 4 − 1 ) ! = 3 ! = 6 ( 4 − 1 ) ! = 3 ! = 6 cycles, but half of them will be the reverse of the others; so, there will be ( 4 − 1 ) ! 2 = 6 2 = 3 ( 4 − 1 ) ! 2 = 6 2 = 3 possible distances.
Step 2: List all the Hamilton cycles in the subgraph of the graph in Figure 12.195 .
To find the 6 cycles, focus on the three vertices in the middle, B, E, and V . The arrangements of these vertices are BEV, BVE, EBV, EVB, VBE , and VEB . These would correspond to the 6 cycles:
1: T → B → E → V → T
2: T → B → V → E → T
3: T → E → B → V → T
4: T → E → V → B → T
5: T → V → B → E → T
6: T → V → E → B → T
Step 3: Find the weight of each path. You can reduce your work by observing the cycles that are reverses of each other.
1: 84 + 410 + 207 + 396 = 1097 84 + 410 + 207 + 396 = 1097
2: 84 + 396 + 207 + 370 = 1071 84 + 396 + 207 + 370 = 1071
3: 370 + 410 + 396 + 396 = 1572 370 + 410 + 396 + 396 = 1572
4: Reverse of cycle 2, 1071
5: Reverse of cycle 3, 1572
6: Reverse of cycle 1, 1097
Step 4: Identify a Hamilton cycle of least weight.
The second path, T → B → V → E → T , and its reverse, T → E → V → B → T , have the least weight. The solution is that the officer should travel from Travis Air Force base to Beal Air Force Base, to Vandenberg Air Force base, to Edwards Air Force base, and return to Travis Air Force base, or the same route in reverse.
Your Turn 12.44
Now suppose that the officer needed a cycle that visited all 5 of the Air Force bases in Figure 12.194 . There would be ( 5 − 1 ) ! = 4 ! = 4 × 3 × 2 × 1 = 24 ( 5 − 1 ) ! = 4 ! = 4 × 3 × 2 × 1 = 24 different arrangements of vertices and ( 5 − 1 ) ! 2 = 4 ! 2 = 24 2 = 12 ( 5 − 1 ) ! 2 = 4 ! 2 = 24 2 = 12 distances to compare using the brute force method. If you consider 10 Air Force bases, there would be ( 10 − 1 ) ! = 9 ! = 9 ⋅ 8 ⋅ 7 ⋅ 6 ⋅ 5 ⋅ 4 ⋅ 3 ⋅ 2 ⋅ 1 = 362 , 880 ( 10 − 1 ) ! = 9 ! = 9 ⋅ 8 ⋅ 7 ⋅ 6 ⋅ 5 ⋅ 4 ⋅ 3 ⋅ 2 ⋅ 1 = 362 , 880 different arrangements and ( 10 − 1 ) ! 2 = 9 ! 2 = 9 ⋅ 8 ⋅ 7 ⋅ 6 ⋅ 5 ⋅ 4 ⋅ 3 ⋅ 2 ⋅ 1 2 = 181 , 440 ( 10 − 1 ) ! 2 = 9 ! 2 = 9 ⋅ 8 ⋅ 7 ⋅ 6 ⋅ 5 ⋅ 4 ⋅ 3 ⋅ 2 ⋅ 1 2 = 181 , 440 distances to consider. There must be another way!
The Nearest Neighbor Method
When the brute force method is impractical for solving a traveling salesperson problem, an alternative is a greedy algorithm known as the nearest neighbor method , which always visit the closest or least costly place first. This method finds a Hamilton cycle of relatively low weight in a complete graph in which, at each phase, the next vertex is chosen by comparing the edges between the current vertex and the remaining vertices to find the lowest weight. Since the nearest neighbor method is a greedy algorithm, it usually doesn’t give the best solution, but it usually gives a solution that is "good enough." Most importantly, the number of steps will be the number of vertices. That’s right! A problem with 10 vertices requires 10 steps, not 362,880. Let’s look at an example to see how it works.
Suppose that a candidate for governor wants to hold rallies around the state. They plan to leave their home in city A , visit cities B, C, D, E , and F each once, and return home. The airfare between cities is indicated in the graph in Figure 12.196 .
Let’s help the candidate keep costs of travel down by applying the nearest neighbor method to find a Hamilton cycle that has a reasonably low weight. Begin by marking starting vertex as V 1 V 1 for "visited 1st." Then to compare the weights of the edges between A and vertices adjacent to A : $250, $210, $300, $200, and $100 as shown in Figure 12.197 . The lowest of these is $100, which is the edge between A and F .
Mark F as V 2 V 2 for "visited 2nd" then compare the weights of the edges between F and the remaining vertices adjacent to F : $170, $330, $150 and $350 as shown in Figure 12.198 . The lowest of these is $150, which is the edge between F and D .
Mark D as V 3 V 3 for "visited 3rd." Next, compare the weights of the edges between D and the remaining vertices adjacent to D : $120, $310, and $270 as shown in Figure 12.199 . The lowest of these is $120, which is the edge between D and B .
So, mark B as V 4 V 4 for "visited 4th." Finally, compare the weights of the edges between B and the remaining vertices adjacent to B : $160 and $220 as shown in Figure 12.200 . The lower amount is $160, which is the edge between B and E .
Now you can mark E as V 5 V 5 and mark the only remaining vertex, which is C , as V 6 V 6 . This is shown in Figure 12.201 . Make a note of the weight of the edge from E to C , which is $180, and from C back to A , which is $210.
The Hamilton cycle we found is A → F → D → B → E → C → A . The weight of the circuit is $ 100 + $ 150 + $ 120 + $ 160 + $ 180 + $ 210 = $ 920 $ 100 + $ 150 + $ 120 + $ 160 + $ 180 + $ 210 = $ 920 . This may or may not be the route with the lowest cost, but there is a good chance it is very close since the weights are most of the lowest weights on the graph and we found it in six steps instead of finding 120 different Hamilton cycles and calculating 60 weights. Let’s summarize the procedure that we used.
Step 1: Select the starting vertex and label V 1 V 1 for "visited 1st." Identify the edge of lowest weight between V 1 V 1 and the remaining vertices.
Step 2: Label the vertex at the end of the edge of lowest weight that you found in previous step as V n V n where the subscript n indicates the order the vertex is visited. Identify the edge of lowest weight between V n V n and the vertices that remain to be visited.
Step 3: If vertices remain that have not been visited, repeat Step 2. Otherwise, a Hamilton cycle of low weight is V 1 → V 2 → ⋯ → V n → V 1 V 1 → V 2 → ⋯ → V n → V 1 .
Example 12.45
Using the nearest neighbor method.
Suppose that the candidate for governor wants to hold rallies around the state but time before the election is very limited. They would like to leave their home in city A , visit cities B , C , D , E , and F each once, and return home. The airfare between cities is not as important as the time of travel, which is indicated in Figure 12.202 . Use the nearest neighbor method to find a route with relatively low travel time. What is the total travel time of the route that you found?
Step 1: Label vertex A as V 1 V 1 . The edge of lowest weight between A and the remaining vertices is 85 min between A and D .
Step 2: Label vertex D as V 2 V 2 . The edge of lowest weight between D and the vertices that remain to be visited, B, C, E , and F , is 70 min between D and F .
Repeat Step 2: Label vertex F as V 3 V 3 . The edge of lowest weight between F and the vertices that remain to be visited, B, C, and E , is 75 min between F and C .
Repeat Step 2: Label vertex C as V 4 V 4 . The edge of lowest weight between C and the vertices that remain to be visited, B and E , is 100 min between C and B .
Repeat Step 2: Label vertex B as V 5 V 5 . The only vertex that remains to be visited is E . The weight of the edge between B and E is 95 min.
Step 3: A Hamilton cycle of low weight is A → D → F → C → B → E → A . So, a route of relatively low travel time is A to D to F to C to B to E and back to A . The total travel time of this route is: 85 min + 70 min + 75 min + 100 min + 95 min + 90 min = 515 min or 8 hrs 35 min 85 min + 70 min + 75 min + 100 min + 95 min + 90 min = 515 min or 8 hrs 35 min
Your Turn 12.45
Check your understanding, section 12.9 exercises.
As an Amazon Associate we earn from qualifying purchases.
This book may not be used in the training of large language models or otherwise be ingested into large language models or generative AI offerings without OpenStax's permission.
Want to cite, share, or modify this book? This book uses the Creative Commons Attribution License and you must attribute OpenStax.
Access for free at https://openstax.org/books/contemporary-mathematics/pages/1-introduction
- Authors: Donna Kirk
- Publisher/website: OpenStax
- Book title: Contemporary Mathematics
- Publication date: Mar 22, 2023
- Location: Houston, Texas
- Book URL: https://openstax.org/books/contemporary-mathematics/pages/1-introduction
- Section URL: https://openstax.org/books/contemporary-mathematics/pages/12-9-traveling-salesperson-problem
© Dec 21, 2023 OpenStax. Textbook content produced by OpenStax is licensed under a Creative Commons Attribution License . The OpenStax name, OpenStax logo, OpenStax book covers, OpenStax CNX name, and OpenStax CNX logo are not subject to the Creative Commons license and may not be reproduced without the prior and express written consent of Rice University.
Open Access is an initiative that aims to make scientific research freely available to all. To date our community has made over 100 million downloads. It’s based on principles of collaboration, unobstructed discovery, and, most importantly, scientific progression. As PhD students, we found it difficult to access the research we needed, so we decided to create a new Open Access publisher that levels the playing field for scientists across the world. How? By making research easy to access, and puts the academic needs of the researchers before the business interests of publishers.
We are a community of more than 103,000 authors and editors from 3,291 institutions spanning 160 countries, including Nobel Prize winners and some of the world’s most-cited researchers. Publishing on IntechOpen allows authors to earn citations and find new collaborators, meaning more people see your work not only from your own field of study, but from other related fields too.
Brief introduction to this section that descibes Open Access especially from an IntechOpen perspective
Want to get in touch? Contact our London head office or media team here
Our team is growing all the time, so we’re always on the lookout for smart people who want to help us reshape the world of scientific publishing.
Home > Books > Theory of Complexity - Definitions, Models, and Applications
How to Solve the Traveling Salesman Problem
Submitted: 23 September 2020 Reviewed: 20 January 2021 Published: 09 February 2021
DOI: 10.5772/intechopen.96129
Cite this chapter
There are two ways to cite this chapter:
From the Edited Volume
Theory of Complexity - Definitions, Models, and Applications
Edited by Ricardo López-Ruiz
To purchase hard copies of this book, please contact the representative in India: CBS Publishers & Distributors Pvt. Ltd. www.cbspd.com | [email protected]
Chapter metrics overview
809 Chapter Downloads
Impact of this chapter
Total Chapter Downloads on intechopen.com

Total Chapter Views on intechopen.com
The Traveling Salesman Problem (TSP) is believed to be an intractable problem and have no practically efficient algorithm to solve it. The intrinsic difficulty of the TSP is associated with the combinatorial explosion of potential solutions in the solution space. When a TSP instance is large, the number of possible solutions in the solution space is so large as to forbid an exhaustive search for the optimal solutions. The seemingly “limitless” increase of computational power will not resolve its genuine intractability. Do we need to explore all the possibilities in the solution space to find the optimal solutions? This chapter offers a novel perspective trying to overcome the combinatorial complexity of the TSP. When we design an algorithm to solve an optimization problem, we usually ask the critical question: “How can we find all exact optimal solutions and how do we know that they are optimal in the solution space?” This chapter introduces the Attractor-Based Search System (ABSS) that is specifically designed for the TSP. This chapter explains how the ABSS answer this critical question. The computing complexity of the ABSS is also discussed.
- combinatorial optimization
- global optimization
- heuristic local search
- computational complexity
- traveling salesman problem
- multimodal optimization
- dynamical systems
Author Information
- School of Management, University of Michigan-Flint, Flint, USA
*Address all correspondence to: [email protected]
1. Introduction
The TSP is one of the most intensively investigated optimization problems and often treated as the prototypical combinatorial optimization problem that has provided much motivation for design of new search algorithms, development of complexity theory, and analysis of solution space and search space [ 1 , 2 ]. The TSP is defined as a complete graph Q = V E C , where V = v i : i = 1 2 … n is a set of n nodes, E = e i j : i j = 1 2 … n i ≠ j n × n is an edge matrix containing the set of edges that connects the n nodes, and C = c i j : i j = 1 2 … n i ≠ j n × n is a cost matrix holding a set of traveling costs associated with the set of edges. The solution space S contains a finite set of all feasible tours that a salesman may traverse. A tour s ∈ S is a closed route that visits every node exactly once and returns to the starting node at the end. Like many real-world optimization problems, the TSP is inherently multimodal; that is, it may contain multiple optimal tours in its solution space. We assume that a TSP instance Q contains h ≥ 1 optimal tours in S . We denote f ( s ) as the objective function, s ∗ = min s ∈ S f s as an optimal tour and S ∗ as the set of h optimal tours. The objective of the TSP is to find all h optimal tours in the solution space, that is, S ∗ ⊂ S . Therefore, the argument is
Under this definition, the salesman wants to know what all best alternative tours are available. Finding all optimal solutions is the essential requirement for an optimization search algorithm. In practice, knowledge of multiple optimal solutions is extremely helpful, providing the decision-maker with multiple options, especially when the sensitivity of the objective function to small changes in its variables may be different at the alternative optimal points. Obviously, this TSP definition is elegantly simple but full of challenge to the optimization researchers and practitioners.
Optimization has been a fundamental tool in all scientific and engineering areas. The goal of optimization is to find the best set of the admissible conditions to achieve our objective in our decision-making process. Therefore, the fundamental requirement for an optimization search algorithm is to find all optimal solutions within a reasonable amount of computing time . The focus of computational complexity theory is to analyze the intrinsic difficulty of an optimization problem and the asymptotic property of a search algorithm to solve it. The complexity theory attempts to address this question: “How efficient is a search algorithm for a particular optimization problem, as the number of variables gets large?”
The TSP is known to be NP-hard [ 2 , 3 ]. The problems in NP-hard class are said to be intractable because these problems have no asymptotically efficient algorithm, even the seemingly “limitless” increase of computational power will not resolve their genuine intractability. The intrinsic difficulty of the TSP is that the solution space increases exponentially as the problem size increases, which makes the exhaustive search infeasible. When a TSP instance is large, the number of possible tours in the solution space is so large to forbid an exhaustive search for the optimal tours. A feasible search algorithm for the TSP is one that comes with a guarantee to find all best tours in time at most proportional to n k for some power k .
Do we need to explore all the possibilities in the solution space to find the optimal solutions? Imagine that searching for the optimal solution in the solution space is like treasure hunting. We are trying to hunt for a hidden treasure in the whole world. If we are “blindfolded” without any guidance, it is a silly idea to search every single square inch of the extremely large space. We may have to perform a random search process, which is usually not effective. However, if we are able to use various clues to locate the small village where the treasure was placed, we will then directly go to that village and search every corner of the village to find the hidden treasure. The philosophy behind this treasure-hunting case for optimization is that: if we do not know where the optimal point is in the solution space, we can try to identify the small region that contains the optimal point and then search that small region thoroughly to find that optimal point.
Optimization researchers have developed many optimization algorithms to solve the TSP. Deterministic approaches such as exhaustive enumeration and branch-and-bound can find exact optimal solutions, but they are very expensive from the computational point of view. Stochastic optimization algorithms, such as simple heuristic local search, Evolutionary Algorithms, Particle Swarm Optimization and many other metaheuristics, can find hopefully a good solution to the TSP [ 1 , 4 , 5 , 6 , 7 ]. The stochastic search algorithms trade in guaranteed correctness of the optimal solution for a shorter computing time. In practice, most stochastic search algorithms are based on the heuristic local search technique [ 8 ]. Heuristics are functions that help us decide which one of a set of possible solutions is to be selected next [ 9 ]. A local search algorithm iteratively explores the neighborhoods of solutions trying to improve the current solution by a local change. However, the scope of local search is limited by the neighborhood definition. Therefore, heuristic local search algorithms are locally convergent. The final solution may deviate from the optimal solution. Such a final solution is called a locally optimal solution , denoted as s ′ in this chapter. To distinguish from locally optimal solutions, the optimal solution s ∗ in the solution space is usually called the globally optimal solution .
This chapter studies the TSP from a novel perspective and presents a new search algorithm for the TSP. This chapter is organized in the following sections. Section 2 presents the ABSS algorithm for the TSP. Section 3 describes the important data structure that is a critical player in solving the TSP. Section 4 discusses the nature of heuristic local search algorithm and introduces the concept of solution attractor. Section 5 describes the global optimization features of the ABSS. Section 6 discusses the computational complexity of the ABSS. Section 7 concludes this chapter.
2. The attractor-based search system for the TSP
Figure 1 presents the Attractor-Based Search System (ABSS) for the TSP. In this algorithm, Q is a TSP instance with the edge matrix E and cost matrix C . At beginning of search, the matrix E is initialized by assigning zeros to all elements of E . The function InitialTour() constructs an initial tour s i using any tour-construction technique. The function LocalSearch() takes s i as an input, performs local search using any type of local search technique, and returns a locally optimal tour s j . The function UpdateE() updates the matrix E by recording the edge configuration of tour s j into the matrix. K is the number of search trajectories. After the edge configurations of K locally optimal tours are stored in the matrix E , the function ExhaustedSearch() searches E completely using the depth-first tree search technique, which is a simple recursive search method that traverses a directed graph starting from a node and then searches adjacent nodes recursively. Finally, the ABSS outputs a set of all best tours S ∗ found in the edge configuration of E . The search strategy in the ABSS is straightforward: generating K locally optimal tours, storing their edge configurations in the matrix E , and then identifying the best tours by evaluating all tours represented by the edge configuration of E . The ABSS is a simple and efficient computer program that can solve the TSP effectively. This search algorithm shows strong features of effectiveness, flexibility, adaptability, scalability and efficiency. The computational model of the ABSS is inherently parallel, facilitating implementation on concurrent processors. It can be implemented in many different ways: series, parallel, distributed, or hybrid.

The ABSS algorithm for the TSP.
Figure 2 uses a 10-node instance as an example to illustrate how the ABSS works. We randomly generate K = 6 n = 60 initial tours, which edge configurations hit all elements of the matrix E (marked as black color), as shown in Figure 2(a) . It means that these 60 random tours hit all 45 edges that represent all 181440 tours in the solution space. We let each of the search trajectories run 5000 iterations and obtain 60 locally optimal tours. However, due to the small size of the instance, most locally optimal tours have identical edge configurations. Among the 60 locally optimal tours, we find only four distinct locally optimal tours as shown in Figure 2(b) . Figure 2(c) shows the union of the edge configurations of the 60 locally optimal tours, in which 18 edges are hit. Then we use the depth-first tree search, as illustrated in Figure 2(d) , to identify all five tours in the edge configuration of E , which are listed in Figure 2(e) . In fact, one of the five tours is the globally optimal tour. This simple example indicates that (1) local search trajectories converge to small set of edges, and (2) the union of the edge configurations of K locally optimal tours is not just a countable union of the edge configurations of the these tours, but also include the edge configurations of other locally optimal tours. The ABSS consists of two search phases: local search phase and exhaustive search phase. The task of the local search phase is to identify the region that globally optimal tour is located (i.e. the village hiding the treasure), and the task of the exhaustive search phase is to find the globally optimal tour (i.e. find the hidden treasure). The remaining sections will briefly explain the features of the ABSS.

A simple example of the ABSS algorithm. (a) Union of the edge configurations of 60 random initial tours, (b) four distinct locally optimal tours, (c) union of the edge configurations of the 60 locally optimal tours, (d) the depth-first tree search on the edge configuration of E , and (e) five tours found in E .
In all experiments mentioned in the chapter, we generate symmetric TSP instances with n nodes. The element c i j of the cost matrix C is assigned a random integer independently drawn from a uniform distribution of the range [1, 1000]. The triangle inequality c i j + c j k ≥ c i k is not assumed in the instances. Although this type of problem instances is application-free, it is mathematically significant. A TSP instance without triangle inequality cannot be approximated within any constant factor. A heuristic local search algorithm usually performs much worse for this type of TSP instances, which offers a strikingly challenge to solving them [ 2 , 3 , 6 , 10 , 11 ]. We use the 2-opt local search technique in the local search phase. The 2-opt neighborhood can be characterized as the neighborhood that induces the greatest correlation between function values of neighboring tours, because neighboring tours differ in the minimum possible four edges. Along the same reasoning line, the 2-opt may have the smallest expected number of locally optimal points [ 12 ]. The local search process randomly selects a solution in the neighborhood of the current solution. A move that gives the first improvement is chosen. The great advantage of the first-improvement pivoting rule is to produce randomized locally optimal points. The software program written for the experiments use several different programming languages and are run in PCs with different versions of Window operating system.
3. The edge matrix E
Usually the edge matrix E is not necessary to be included in the TSP definition because the TSP is a complete graph. However, the edge matrix E is an effective data structure that can help us understand the search behavior of a local search system. General local search algorithm may not require much problem-specific knowledge in order to generate good solutions. However, it may be unreasonable to expect a search algorithm to be able to solve any problem without taking into account the data structure and properties of the problem at hand.
To solve a problem, the first step is to create a manipulatable description of the problem itself. For many problems, the choice of data structure for representing a solution plays a critical role in the analysis of search behavior and design of new search algorithm. For the TSP, a tour can be represented by an ordered list of nodes or an edge configuration of a tour in the edge matrix E , as illustrated in Figure 3 . The improvement of the current tour represents the change in the order of the nodes or the edge configuration of a tour.

Two representations of a tour: an ordered list of nodes and an edge configuration of a tour.
Observing the behavior of search trajectories in a local search system can be quite challenging. The edge matrix E is a natural data structure that can help us trace the search trajectories and understand the dynamics of a local search system. An edge e i j is the most basic element of a tour, but contains a piece of information about each of n − 2 ! tours that go through it. Essentially, the nature of local search for the TSP is an edge-selection process: preservation of good edges and rejection of bad edges according to the objective function f s . Each edge has an implicit probability to be selected by a locally optimal tour. A better edge has higher probability to be included in a locally optimal tour. Therefore, the edges in E can be divided into three groups: globally superior edges, G -edges, and bad edges. A globally superior edge is the edge that occurs in many or all locally optimal tours. Although each of these locally optimal tours selects this edge based on its own search trajectory, the edge is globally superior since the edge is selected by these individual tours from different search trajectories going through different search regions. The globally superior edges have higher probability to be selected by a locally optimal tour. A G -edge is the edge that is included in a globally optimal tour. All G -edges are globally superior edges and can be treated as a special subset of the globally superior edges. The edges that are discarded by all search trajectories or selected by only few locally optimal tours are bad edges. A bad edge is impossible to be included in a globally optimal tour. A locally optimal tour usually consists of some G -edges, some globally superior edges and a few bad edges.
The changes of the edge configuration of the matrix E represent the transformations of the search trajectories in a local search system. When all search trajectories reach their end points, the final edge configuration of E represents the final state of the local search system. For a tour s k , we define an element e i j of E as
Then the hit-frequency value e ij in the element e i j is defined as the number of occurrence of the element in K tours, that is
When K search trajectories reach their end points, the value e ij + e ji / K can represent the probability of the edge e i j being hit by a locally optimal tour. We can use graphical technique to observe the convergent behavior of the search trajectories through the matrix E . The hit-frequency value e ij can be easily converted into a unit of half-tone information in a computer, a value that we interpret as a number H ij somewhere between 0 and 1. The value 1 corresponds to black color, 0 to white color, and any value in between to a gray level. Let K be the number of search trajectories, the half-tone information H ij on a computer screen can be represented by the hit-frequency e ij in the element e i j of E :
Figure 4 illustrates a simple example of visualization showing the convergent behavior of 100 search trajectories for a 50-node instance. Figure 4(a) shows the image of the edge configurations of 100 random initial tours. Since each element of E has equal chance to be hit by these initial tours, almost all elements are hit by these initial tours, and all elements have very low H ij values, ranging from 0.00 to 0.02. When the local search system starts searching, the search trajectories constantly change their edge configurations, and therefore the colors in the elements of E are changed accordingly. As the search continues, more and more elements become white (i.e. they are discarded by all search trajectories) and other elements become darker (i.e. they are selected by more search trajectories). When all search trajectories reach their end points, the colored elements represent the final edge configuration of the search system. Figure 4(b) and (c) show the images of edge configuration of E when all search trajectories completed 2000 iterations and 5000 iterations, respectively. At 5000th iteration, the range of H ij values in the elements of E is from 0.00 to 0.42. The value 0.42 means that 42% of the search trajectories select this element. Majority of the elements of E become white color.

Visualization of the convergent dynamics of local search system. (a) the image of the edge configurations of 100 initial tours, (b) and (c) the images of edge configurations when the search trajectories are at 2000th and 5000th iteration, respectively.
This simple example has great explanatory power about the global dynamics of the local search system for the TSP. As search trajectories continue searching, the number of edges hit by them becomes smaller and smaller, and better edges are hit by more and more search trajectories. This edge-convergence phenomenon means that all search trajectories are moving closer and closer to each other, and their edge configurations become increasingly similar. This phenomenon describes the globally asymptotic behavior of the local search system.
It is easily verified that under certain conditons, a local search system is able to find the set of the globally optimal tours S ∗ when the number of search trajectories is unlimited, i.e.
However, the required search effort may be very huge – equivalent to enumerating all tours in the solution space. Now one question for the ABSS is “How many search trajectories in the search system do we need to find all globally optimal tours?” The matrix E consists of n n − 1 elements (excluding the diagonal elements). When we randomly construct a tour and record its edge configuration in E , n elements of E will be hit by this tour. If we construct more random tours and record their edge configurations in E , more elements will be hit. We define K as the number of randomly-constructed initial tours, whose edge configurations together will hit all elements of E . We know that all elements of E represent all combinatorial possibilities in the solution space. Therefore, K is the number of search trajectories such that the union of edge configurations of ther initial tours covers the entire solution space. In our experiments, we found that the edge configurations of at most 6 n randomly-constructed tours can guarantee to hit all elements of E . From the tour perspective, K = 6 n random tours represent only a small set of the tours in the solution space. However, from the view of edge-configuration, the union of the edge configurations of 6 n random tours represents the edge configurations of all tours in the solution space. It reveals an amazing fact: the union of the edge configurations of only 6 n random tours contains the edge configurations of all n n − 1 ! / 2 tours in the solution space. It reflects the combinatorial nature of the TSP: the tours in the solution space are formed by different combinations of the edges. The union of the edge configurations of a set of tours contains information about many other tours because one tour shares its edges with many other tours. One fundamental theory that can help us explain this phenomenon is the information theory [ 13 ]. According to the information theory, each solution point contains some information about its neighboring solutions that can be modeled as a function, called information function or influence function . The influence function of the i th solution point in the solution space S is defined as a function Ω i : S → R , such that Ω i is a decreasing function of the distance from a solution point to the i th solution point. The notion of influence function has been extensively used in datamining, data clustering, and pattern recognition.
4. The nature of heuristic local search
Heuristic local search is based on the concept of neighborhood search. A neighborhood of a solution s i , denoted as N s i , is a set of solutions that are in some sense close to s i . For the TSP, a neighborhood of a tour s i is defined as a set of tours that can be reached from s i in one single transition. From edge-configuration perspective, all tours in N s i are very similar because they share significant number of edges with s i . The basic operation of local search is iterative improvement, which starts with an initial tour and searches the neighborhood of the current tour for a better tour. If such a tour is found, it replaces the current tour and the search continues until no improvement can be made. The local search algorithm returns a locally optimal tour.
The behavior of a local search trajectory can be understood as a process of iterating a search function g s . We denote s 0 as an initial point of search and g t s as the t th iteration of the search function g s . A search trajectory s 0 , g s 0 , g 2 s 0 , … , g t s 0 , … converges to a locally optimal point s ′ as its limit, that is,
Therefore, a search trajectory will reach an end point (a locally optimal point) and will stays at this point forever.
In a heuristic local search algorithm, there is a great variety of ways to construct initial tour, choose candidate moves, and define criteria for accepting candidate moves. Most heuristic local search algorithms are based on randomization. In this sense, a heuristic local search algoorithm is a randomized system. There are no two search trajectories that are exactly alike in such a search system. Different search trajectories explore different regions of the solution space and stop at different final points. Therefore, local optimality depends on the initial points, the neighborhood function, randomness in the search process, and time spent on search process. On the other hand, however, a local search algorithm essentially is deterministic and not random in nature. If we observe the motion of all search trajectories, we will see that the search trajectories go towards the same direction, move closer to each other, and eventually converge into a small region in the solution space.
Heuristic local search algorithms are essentially in the domain of dynamical systems. A heuristic local search algorithm is a discrete dynamical system, which has a solution space S (the state space), a set of times T (search iterations), and a search function g : S × T → S that gives the consequents to a solution s ∈ S in the form of s t + 1 = g s t . A search trajectory is the sequence of states of a single search process at successive time-steps, which represents the part of the solution space searched by this search trajectory. The questions about the behavior of a local search system over time are actually the questions about its search trajectories. The most basic question about the search trajectories is “Where do they go in the solution space and what do they do when they get there?”
The attractor theory of dynamical systems is a natural paradigm that can be used to describe the search behavior of a heuristic local search system. The theory of dynamical systems is an extremely broad area of study. A dynamical system is a model of describing the temporal evolution of a system in its state space. The goal of dynamical system analysis is to capture the distinctive properties of certain points or regions in the state space of a given dynamical system. The theory of dynamical systems has discovered that many dynamical systems exhibt attracting behavior in the state space [ 14 , 15 , 16 , 17 , 18 , 19 , 20 , 21 , 22 ]. In such a system, all initial states tend to evolve towards a single final point or a set of points. The term attractor is used to describe this single point or the set of points in the state space. The attractor theory of dynamical systems describes the asymptotic behavior of typical trajectories in the dynamical system. Therefore, the attractor theory provides the theoretical foundation to study the search behavior of a heuristic lcoal search system.
In a local search system for the TSP, no matter where we start a search trajectory in the solution space, all search trajectories will converge to a small region in the solution space for a unimodal TSP instance or h small regions for a h -model TSP. We call this small region a solution attractor of the local search system for a given TSP instance, denoted as A . Therefore, the solution attractor of a local search system for the TSP can be defined as an invariant set A ⊂ S consisting of all locally optimal tours and the globally optimal tours. A single search trajectory typically converges to either one of the points in the solution attractor. A search trajectory that is in the solution attractor will remain within the solution attractor forward in time. Because a globally optimal tour s ∗ is a special case of locally optimal tours, it is undoubtedly embodied in the solutioin attractor, that is, s ∗ ∈ A . For a h -modal TSP instance, a local search system will generate h solution attractors A 1 A 2 … A h that attract all search trajectories. Each of the solution attractors has its own set of locally optimal tours, surrounding a globally optimal tour s i ∗ i = 1 2 … h . A particular search trajectory will converge into one of the h solution attractors. All locally optimal tours will be distributed to these solution attractors. According to dynamical systems theory [ 20 ], the closure of an arbitrary union of attractors is still an attractor. Therefore, the solution attractor A of a local search system for a h -modal TSP is a complete collection of h solution attractors A = A 1 ∪ A 2 ∪ … ∪ A h .
Convexity , i.e. ∀ s i ∈ S , g t s i ∈ A for sufficient long t ;
Centrality , i.e. the globally optimal tour s i ∗ is located centrally with respect to the other locally optimal tours in A i i = 1 2 … h ;
Invariance , i.e. ∀ s ′ ∈ A , g t s ′ = s ′ and g t A = A for all time t ;
Inreducibility , i.e. the solution attractor A contains a limit number of invariant locally optimal tours.

Illustration of the concepts of serch trajectories and solution attractors in a local search system for a multimodal optimization problem.
A search trajectory in a local search system changes its edge configuration during the search according to the objective function f s and its neighborhood structure. The matrix E can follow the “footprints” of search trajectories to capture the dynamics of the local search system. When all search trajectories reach their end points – the locally optimal tours, the edge configuration of the matrix E will become fixed, which is the edge configuration of the solution attractor A . This fixed edge configuration contains two groups of edges: the edges that are not hit by any of locally optimal tours (non-hit edges) and the edges that are hit by at least one of the locally optimal tours (hit edges). Figure 6 shows the edge grouping in the edge configuration of E when all search trajectories stop at their final points.

The grouping of the edges in E when all search trajectories reach their end points.
In the ABSS, we use K search trajectories in the local search phase. Different sets of K search trajectories will generate different final edge configuration of E . Suppose that, we start the local search from a set of K initial points and obtain a edge configuration M a in E when the local search phase is terminated. Then we start the local search process again from a different set of K initial points and obtains a little different edge configuration M b in E . Which edge configuration truly describes the edge configuration of the real solution attractor? Actually, M a and M b are structurally equivalent because they are different only in the set of bad edges, thus M a precisely replicates the dynamical properties of M b . The final edge configuration of the constructed solution attractor generated from K search trajectories is not sensitive to the selection of K search trajectories. This property indicates that a heuristic local search system actually is a deterministic system: although a single search trajectory appears stochastic, all search trajectories from differeitn initial points will be always trapped into the same small region in the solution space and the final edge configuration of E will always converge to the same set of the globally optimal edges.
The convergence of the search trajectories can be measured by the change in the edge configuration of the matrix E . In the local search process, search trajectories collect all available topology information about the quality of the edges from their search experience and record such information in the matrix E . The changes in the edge configuration of E fully reflects the real search evolution of the search system. A state of convergence is achieved once no any more local search trajectory can change the edge configuration of E . For a set of search trajectories to be converging, they must be getting closer and closer to each other, that is, their edge configurations become increasingly similar. As a result, the edge configurations of the search trajectories converge to a small set of edges that contains all globally superior edges and some bad edges. Let W denote total number of edges in E , α t the number of the edges that are hit by all search trajectories at time t , β t the number of the edges that are hit by one or some of the search trajectories, and γ t the number of edges that have no hit at all, then at any time t , we have
For a given TSP instance, W is a constant value W = n n − 1 / 2 for a symmetric instance or W = n n − 1 for an asymmetric instance. During the local search process, the values for α t and γ t will increase and the value for β t will decrease. However, these values cannot increase or decrease foreover. At certain point of time, they will become constant values, that is,
Our experiments confirmed this inference about α t , β t and γ t . Figure 7 illustrates the patterns of α t , β t and γ t curves generated in our experiments. Our experiments also found that, for unimodal TSP instances, the ratio γ t / W could approach to 0.70 quickly for different sizes of TSP instances. For multimodal TSP instances, this ratio depends on the number of the globally optimal points. However, the set of hit edges is still very small.

The α t , β t and γ t curves with search iterations.
It contains all locally optimal tours;
It contains a complete collection of solution attractors, i.e. A = A 1 ∪ A 2 ∪ … ∪ A h ;
It contains a complete collection of G -edges, i.e. G = G 1 ∪ G 2 ∪ … ∪ G h .
From this analysis, we can see that the edge matrix E is an extremely useful data structure that not only collcets the information about search trajectories, but also convert local search behavor of individual search trajectories into global search behavor of the search system. The global convergence and deterministic property of the search trajectories make the local search system always converge to the same solution attractors and the edge configurations of the search trajectories always converge to the same set of globally superior edges. The matrix E shows us clearly where the search trajectories go and where all locally optimal points are located. We found the village! However, it is still difficult to identify all G -edges among the globally superior edges. The ABSS uses the exhaustive search phase to find all tours in the solution attractor. Since the local search phase has significantly reduced the size of the search space for the exhaustive search phase, the complete search in the solution attractor becomes feasible.
5. Global optimization feature of the ABSS
The search system should be globally convergent.
The search system should be deterministic and have a rigorous guarantee for finding all globally optimal solutions without excessive computational burden.
The optimality criterion in the system must be based on information on the global behavior of the search system.
The ABSS combines beautifully two crucial aspects in search: exploration and exploitation. In the local search phase, K search trajectories explore the full solution space to identify the globally superior edges, which form the edge configuration of the solution attractor. These K search trajectories are independently and invidually executed, and therefore they create and maintain diversity from beginning to the end. The local search phase is a randomized process due to randomization in the local search function g s . In this sense, the K search trajectories actually perform the Monte Carlo simulation to sample locally optimal tours. The essential idea of Monte Carlo method is using randomness to solve problems that might be deterministic in principle [ 26 ]. In the ABSS, K search trajectories start a sample of initial points from a uniform distribution over the solution space S , and, through the randomized local search process, generate a sample of locally optimal points uniformly distributed in the solution attractor A . The edge configuration of E is actually constructed through this Monte Carlo sampling process.
Each of the K search trajectories passes through many neighborhoods on its way to the final point. For any tour s i , the size of N s i is greater than n 2 ! [ 12 ]. Let N s i ′ denote the neighborhood of the final point s i ′ of the i th search trajectory and Ω N s tran i as the union of the neighborhoods of all transition points of the search trajectory, then we can believe that the search space covered by K search trajectories is
That is, the solution attractor A is formed through the entire solution space S . The solution attractor A contains h unique minimal “convex” sets A i i = 1 2 … h . Each A i has a unique best tour s i ∗ surrounded by a set of locally optimal tours. The tour s i ∗ in A i satisfies f s i ∗ < f s for all s ∈ A i and f s 1 ∗ = f s 2 ∗ = … = f s h ∗ .
We see that the matrix E plays a critical role to transform local search process of the individual search trajectories into a collective global search process of the system. Each time when a local search trajectory finds a better tour and updates the edge configuraton of E , the conditional distribution on the edges are updated. More values are attached to the globally superior edges, and bad edges are discarded. Let W be the complete set of the edges in E and W A the set of edges in the edge configuration of the solution attractor A such that g W is contained in the interior of W . Then the intersection W A of the nested sequence of sets is
and lim t → ∞ g t W A = W A . As a result, the edge configurations of K search trajectories converge to a small set of edges.
∀ s ∈ A i , f s i ∗ < f s
f s 1 ∗ = f s 2 ∗ = … = f s h ∗
min s ∈ A f s = min s ∈ S f s
Therefore the global convergence and deterministic property of the search trajectories in the local search phase make the ABSS always find the same set of globally optimal tours. We conducted several experiments to confirm this argument empirically. In our experiments, for a given TSP instance, the ABSS performed the same search process on the instance several times, each time using a different set of K search trajectories. The ABSS outputed the same set of the best tours in all trials.
Table 1 shows the results of two experiments. One experiment generated n = 1000 instance Q 1000 , the other generated n = 10000 instance Q 10000 . We conducted 10 trials on each of the instances respectively. In each trial, the ABSS used K = 6 n search trajectories. Each search trajectory stopped when no improvement was made during 10 n iterations. The matrix E stored the edge configurations of the K final tours and then was searched completely using the depth-first tree search process. Table 1 lists the number of tours found in the constructed solution attractor A , the cost range of these tours, and the number of the best tours found in the constructed solution attractor. For instance, in trial 1 for Q 1000 , the ABSS found 6475824 tours with the cost range [3241, 4136] in the constructed solution attractor. There was a single best tour in the solution attractor. The ABSS found the same best tour in all 10 trials. For the instance Q 10000 , the ABSS found the same set of four best tours in all 10 trials. These four best tours have the same cost value, but with different edge configurations. If any trial had generated a different set of the best tours, we could immediately make a conclusion that the best tours in the constructed solution attractor may not be the globally optimal tours. From practical perspective, the fact that the same set of the best tours was detected in all trials provides an empirical evidence of the global optimality of these tours. The fact also indicates that the ABSS converges in solution. Convergence in solution means that the search system can identify all optimal solutions repeatedly. Always finding the same set of optimal solutions actually is the fundamental requirement for global optimization systems.
Tours in constructed solution attractor A for Q 1000 and Q 10000 .
6. Computing complexity of the ABSS
With current search technology, the TSP is an infeasible problem because it is not solvable in a reasonable amount of time. Faster computers will not help. A feasible search algorithm for the TSP is one that comes with a guarantee to find all best tours in time at most proportional to n k for some power k . The ABSS can guarantee to find all globally optimal tours for the TSP. Now the question is how efficient it is?
The core idea of the ABSS is that, if we have to use exhaustive search to confirm the globally optimal points, we should first find a way to quickly reduce the effective search space for the exhaustive search. When a local search trajectory finds a better tour, we can say that the local search trajectory finds some better edges. It is an inclusive view. We also can say that the local search trajectory discards some bad edges. It is an exclusive view. The ABSS uses the exclusive strategy to conquer the TSP. The local search phase in the ABSS quickly prunes out large number of edges that cannot possibly be included in any of the globally optimal tours. Thus, a large useless area of the solution space is excluded. When the first edge is discarded by all K search trajectories, n − 2 ! tours that go through that edge are removed from the search space for the exhaustive search phase. Each time when an edge is removed, large number of tours are removed from the search space. Although the complexity of finding a true locally optimal tour is still open, and we even do not know any nontrivial upper bounds on the number of iterations that may be needed to reach local optimality [ 27 , 28 ], decades of empirical evidence and practical research have found that heuristic local search converges quickly, within low order polynomial time [ 1 , 8 , 27 , 29 ]. In practice, we are rarely able to find perfect locally optimal tour because we simply do not allow the local search process to run enough long time. Usually we let a local search process run a predefined number of iterations, accept whatever tour it generates, and treat it as a locally optimal tour. Therefore, the size of the constructed solution attractor depends not only on the problem structure and the neighborhood function, but also on the amount of search time invested in the local search process. As we increase local search time, we will constructe a smaller and stronger solution attractor. The local search phase in the ABSS can significantly reduce the search space for the exhaustive search phase by excluding a large number of edges. Usually the local search phase can remove about 60% of edges of the matrix E in O n 2 .
Now an essential question is naturally raised: What is the relationship between the size of the constructed solution attractor and the size of the problem instance? Unfortunately, there is no theoretical analysis tool available in the literature that can be used to answer this question. We have to depend on empirical results to lend some insights. We conducted several experiments to observe the relationship between the size of the constructed solution attractor and the TSP instance size. Figures 8 – 10 show the results of one of our experiments. All other similar experiments reveal the same pattern. In this experiment, we generated 10 unimodal TSP instances in the size from 1000 to 10000 nodes with 1000-node increment. For each instance, the ABSS generated K = 6 n search trajectories. We first let each search trajectory stop when no tour improvement was made during 10000 iterations regardless of the size of the instance (named “fixed search time”). Then we did the same search procedures on these instances again. This time we made each search trajectory stop when no improvement was made during 10 n iterations (named “varied search time 1”) and 100 n iterations (named “varied search time 2”) respectively. Figure 8 shows the number of the edges that were discarded at the end of local search phase. Figure 9 shows the number of tours in the constructed solution attractor for each instance, and Figure 10 shows the effective branching factors in the exhaustive search phase.

The number of discarded edges at the end of local search phase.

Relationship between the size of the constructed solution attractor and instance size.

The b ∗ values for different instance size n in our experiment.
In Figure 8 , we can see that the search trajectories can quickly converge to a small set of edges. In the fixed-search-time case, about 60% of the edges were discarded by search trajectories for the 1000-node instance, but this percentage decreases as instance size increases. For the 10000-node instance, only about 46% of the edges are discarded. However, if we increase the local search time linearly when the instance size increases, we can keep the same percentage of discarded-edge for all instance sizes. In the varied-search-time-1 case, about 60% of the edges are abandoned for all different instance sizes. In the varied-search-time-2 case, this percentage increases to 68% for all instances. Higher percentage of abandoned edges means that majority of the branches are removed from the search tree.
Figure 9 shows the number of tours exist in the constructed solution attractor for these instances. All curves in the chart appear to be linear relationship between the size of constructed solution attractor and the size of the problem instance, and the varied-search-time curves have much flatter slope because longer local search time makes a smaller constructed solution attractor. Figures 8 and 9 indicate that the search trajectories in the local search phase can effectively and efficiently reduce the search space for the exhaustive search, and the size of the solution attractor increases linearly as the size of the problem instance increases. Therefore, the local search phase in the ABSS is an efficiently asymptotical search process that produces an extremely small search space for further exhaustive search.
The completely searching of the constructed solution attractor is delegated to the exhaustive search phase. This phase may still need to examine tens or hundreds of millions of tours but nothing a computer processor cannot handle, as opposed to the huge number of total possibilities in the solution space. The exhaustive search phase can find the exact globally optimal tours for the problem instance after a limited number of search steps.
The exhaustive search phase can use any enumerative technique. However, the edge configuration of E can be easily searched by the depth-first tree search algorithm. One of the advantages of depth-first tree search is less memory requirement since only the nodes on the current path are stored. When using tree-search algorithm, we usually use branching factor, average branching factor, or effective branching factor to measure the computing complexity of the algorithm [ 30 , 31 , 32 , 33 ]. In the data structure of search tree, the branching factor is the number of successors generated by a given node. If this value is not uniform, an average branching factor can be calculated. An effective branching factor b ∗ is the number of sucessors generated by a typical node for a given tree-search problem. We use the following definition to calculate effective brancing factor b ∗ for the exhaustive search phase:
where n is the size of the TSP instance, representing the depth of the tree, and N is total number of nodes generated in the tree from the origin node. In our experiments, the tree-search process always starts from node 1 (the first row of E ). N is total number of nodes that are processed to construct all valid tours and incomplete (therefore abandoned) tours in E . N does not count the node 1 (the origin node), but includes the node 1 as the end node of a valid tour. We use Figure 2(d) as an example. The depth-first search process searches the edge configuration of E and will generate N = 58 nodes. Therefore, b ∗ ≈ 1.3080 , that is, 58 ≈ 1.3080 + 1.3080 2 + … + 1.3080 10 . Figure 10 shows the effective branching factor b ∗ in our experiment. The low values of b ∗ indicates that the edge configuration of the solution attractor represents a tree with extremely sparse branches, and the degree of sparseness does not changes as the problem size increase if we linearly increase local search time in the local search phase for a large instance. The search time in the exhaustive search phase is probably in O n 2 since the size of the constructed solution attractor might be linearly increased with the problem size n and the number of edges in E is polynomially increased with the problem size. Our experiments shows that the ABSS can significantly reduce the computational complexity for the TSP and solve the TSP efficiently with global optimality guarantee.
Therefore, the ABSS is a simple algorithm that increases in computational difficulty polynomially with the size of the TSP. In the ABSS, the objective pursued by the local search phase is “quickly eliminating unnecessary search space as much as possible.” It can provide an answer to the question “In which small region of the solution space is the optimal solution located?” in time of O n 2 . The objective of the exhaustive search phase is “identifying the best tour in the remaining search space.” It can provide an anwer to the question “Which is the best tour in this small region?” in time of O n 2 . All together, the ABSS can answer the question “Is this tour the best tour in the solution space?” in time of O n 2 . Therefore, the ABSS is probably with computing complexity of O n 2 and memory space requirement of O n 2 . This suggests that the TSP might not be as complex as we might have expected.
7. Conclusion
Advances in computational techniques on the determination of the global optimum for an optimization problem can have great impact on many scientific and engineering fields. Although both the TSP and heuristic local search algorithms have huge literature, there is still a variety of open problems. Numerous experts have made huge advance on the TSP research, but two fundamental questions of the TSP remain essentially open: “How can we find the optimal tours in the solution space, and how do we know they are optimal?”
The P-vs-NP problem is about how fast we can search through a huge number of solutions in the solution space [ 34 ]. Do we ever need to explore all the possibilities of the problem to find the optimal one? Actually, the P-vs-NP problem asks whether, in general, we can find a method that completely searches only the region where the optimal points are located [ 34 , 35 , 36 ]. Most people believe P ≠ NP because we have made little fundamental progress in the area of exhaustive search. Modern computers can greatly speed up the search, but the extremely large solution space would still require geologic search time to find the exact optimal solution on the fastest machines imaginable. A new point of view is needed to improve our capacity to tackle these difficulty problems. This paper describe a new idea: using efficient local search process to effectively reduce the search space for exhaustive search. The concept of solution attractor in heuristic local search systems may change the way we think about both local search and exhaustive search. Heuristic local search is an efficient search system, while exhaustive search is an effective search system. The key is how we combines these two systems into one system beautifully to conquer the fundamental issues of the hard optimization problems. In the TSP case, the edge matrix E , a problem-specific data structure, plays a critical role of reducing the search space and transforming local search to global search.
The ABSS is designed for the TSP. However, the concepts and formulation behind the search algorithm can be used for any combinatorial optimization problem requiring the search of a node permutation in a graph.
- 1. Applegate DL, Bixby RE, Chaátal V, Cook WJ. The Traveling Salesman Problem: A Computational Study. Princeton: Princeton University Press; 2006
- 2. Papadimitriou CH, Steiglitz K. Combinatorial Optimization: Algorithms and Complexity. New York: Dover Publications; 1998
- 3. Papadimitriou CH, Steiglitz K. On the complexity of local search for the traveling salesman problem. SIAM Journal on Computing. 1977:6:76–83
- 4. Gomey J. Stochastic global optimization algorithms: a systematic formal approach. Information Science. 2019:472:53–76
- 5. Korte B, Vygen J. Combinatorial Optimization: Theory and Algorithms. New York: Springer; 2007
- 6. Rego C, Gamboa D, Glover F, Osterman C. Traveling salesman problem heuristics: leading methods, implementations and latest advances. European Journal of Operational Research. 2011:211:427–411
- 7. Zhigliavsky A, Zillinakas A. Stochastic Global Optimization. New York: Springer; 2008
- 8. Aart E, Lenstra JK. Local Search in Combinatorial Optimization. Princeton: Princeton University Press; 2003
- 9. Michalewicz Z, Fogel DB. How to Solve It: Modern Heuristics. Berlin: Springer; 2002
- 10. Sahni S, Gonzales T. P-complete approximation problem. Journal of the ACM. 1976:23:555–565
- 11. Sourlas N. Statistical mechanics and the traveling salesman problem. Europhysics Letters. 1986:2:919–923
- 12. Savage SL. Some theoretical implications of local optimality. Mathematical Programming. 1976:10:354–366
- 13. Shammon CE. A mathematical theory of communication. Bell System Technical Journal. 1948:27:379–423&623–656
- 14. Alligood KT, Sauer TD, York JA. Chaos: Introduction to Dynamical System. New York: Springer; 1997
- 15. Auslander J, Bhatia NP, Seibert P. Attractors in dynamical systems. NASA Technical Report NASA-CR-59858; 1964
- 16. Brin M, Stuck G. Introduction to Dynamical Systems. Cambridge: Cambridge University Press
- 17. Brown R. A Modern Introduction to Dynamical Systems. New York: Oxford University Press
- 18. Denes A, Makey G. Attractors and basis of dynamical systems. Electronic Journal of Qualitative Theory of Differential Equations. 2011:20(20):1–11
- 19. Fogedby H. On the phase space approach to complexity. Journal of Statistical Physics. 1992:69:411–425
- 20. Milnor J. On the concept of attractor. Communications in Mathematical Physics. 1985:99(2):177–195
- 21. Milnor J. Collected Papers of John Milnor VI: Dynamical Systems (1953–2000). American Mathematical Society; 2010
- 22. Ruelle D. Small random perturbations of dynamical systems and the definition of attractor. Communications in Mathematical Physics. 1981:82:137–151
- 23. Li W. Dynamics of local search trajectory in traveling salesman problem. Journal of Heuristics. 2005:11:507–524
- 24. Li W, Feng M. Solution attractor of local search in traveling salesman problem: concepts, construction and application. International Journal of Metaheuristics. 2013:2(3):201–233
- 25. Li W, Li X. Solution attractor of local search in traveling salesman problem: computational study. International Journal of Metaheuristics. 2019:7(2):93–126
- 26. Kroese DP, Taimre T, Botev ZI. Handbook of Monte Carlo Methods. New York: John Wiley & Sons; 2011
- 27. Fischer ST. A note on the complexity of local search problems. Information Processing Letters. 1995:53(2):69–75
- 28. Chandra B, Karloff HJ, Tovey CA. New results on the old-opt algorithm for the traveling salesman problem. SIAM Journal on Computing. 1999:28(6):1998–2029
- 29. Grover, LK. Local search and the local structure of NP-complete problems. Operations Research Letters. 1992:12(4):235–243
- 30. Baudet GM. On the branching factor of the alpha-beta pruning algorithm. Artificial Intelligence. 1978:10(23):173–199
- 31. Edelkamp S, Korf RE. The branching factor of regular search space. Proceedings of the 15 th National Conference on Artificial Intelligence. 1998:292–304
- 32. Korf RE. Depth-first iterative deepening: an optimal admissible tree search. Artificial Intelligence. 1985:27:97–109
- 33. Pearl J. The solution for the branching factor of the alpha-beta pruning algorithm and its optimality. Communication of the ACM. 1982:25(8):559–564
- 34. Fortnow L. The Golden Ticket – P, NP, and the Search for the Impossible. Princeton: Princeton University Press; 2013
- 35. Fortnow L. The status of the P versus NP problem. Communication of the ACM. 2009:52(9):78–86
- 36. Sipser M. The history of status of the P verses NP question. Proceedings of 24 th Annual ACM Symposium on Theory of Computing. 1992:603–618
© 2021 The Author(s). Licensee IntechOpen. This chapter is distributed under the terms of the Creative Commons Attribution 3.0 License , which permits unrestricted use, distribution, and reproduction in any medium, provided the original work is properly cited.
Continue reading from the same book
Theory of complexity.
Published: 30 June 2021
By Rafael Prieto Curiel
432 downloads
By Michael J. Droboniku, Heidi Kloos, Dieter Vanderel...
369 downloads
By Sérgio Henrique Vannucchi Leme de Mattos, Luiz Edu...
553 downloads
DSA Tutorial
Linked lists, stacks & queues, hash tables, shortest path, minimum spanning tree, maximum flow, time complexity, dsa reference, dsa examples, dsa the traveling salesman problem.
The Traveling Salesman Problem
The Traveling Salesman Problem states that you are a salesperson and you must visit a number of cities or towns.
Rules : Visit every city only once, then return back to the city you started in.
Goal : Find the shortest possible route.
Except for the Held-Karp algorithm (which is quite advanced and time consuming, (\(O(2^n n^2)\)), and will not be described here), there is no other way to find the shortest route than to check all possible routes.
This means that the time complexity for solving this problem is \(O(n!)\), which means 720 routes needs to be checked for 6 cities, 40,320 routes must be checked for 8 cities, and if you have 10 cities to visit, more than 3.6 million routes must be checked!
Note: "!", or "factorial", is a mathematical operation used in combinatorics to find out how many possible ways something can be done. If there are 4 cities, each city is connected to every other city, and we must visit every city exactly once, there are \(4!= 4 \cdot 3 \cdot 2 \cdot 1 = 24\) different routes we can take to visit those cities.
The Traveling Salesman Problem (TSP) is a problem that is interesting to study because it is very practical, but so time consuming to solve, that it becomes nearly impossible to find the shortest route, even in a graph with just 20-30 vertices.
If we had an effective algorithm for solving The Traveling Salesman Problem, the consequences would be very big in many sectors, like for example chip design, vehicle routing, telecommunications, and urban planning.
Checking All Routes to Solve The Traveling Salesman Problem
To find the optimal solution to The Traveling Salesman Problem, we will check all possible routes, and every time we find a shorter route, we will store it, so that in the end we will have the shortest route.
Good: Finds the overall shortest route.
Bad: Requires an awful lot of calculation, especially for a large amount of cities, which means it is very time consuming.
How it works:
- Check the length of every possible route, one route at a time.
- Is the current route shorter than the shortest route found so far? If so, store the new shortest route.
- After checking all routes, the stored route is the shortest one.
Such a way of finding the solution to a problem is called brute force .
Brute force is not really an algorithm, it just means finding the solution by checking all possibilities, usually because of a lack of a better way to do it.
Speed: {{ inpVal }}
Finding the shortest route in The Traveling Salesman Problem by checking all routes (brute force).
Progress: {{progress}}%
n = {{vertices}} cities
{{vertices}}!={{posRoutes}} possible routes
Show every route: {{showCompares}}
The reason why the brute force approach of finding the shortest route (as shown above) is so time consuming is that we are checking all routes, and the number of possible routes increases really fast when the number of cities increases.
Finding the optimal solution to the Traveling Salesman Problem by checking all possible routes (brute force):
Using A Greedy Algorithm to Solve The Traveling Salesman Problem
Since checking every possible route to solve the Traveling Salesman Problem (like we did above) is so incredibly time consuming, we can instead find a short route by just going to the nearest unvisited city in each step, which is much faster.
Good: Finds a solution to the Traveling Salesman Problem much faster than by checking all routes.
Bad: Does not find the overall shortest route, it just finds a route that is much shorter than an average random route.
- Visit every city.
- The next city to visit is always the nearest of the unvisited cities from the city you are currently in.
- After visiting all cities, go back to the city you started in.
This way of finding an approximation to the shortest route in the Traveling Salesman Problem, by just going to the nearest unvisited city in each step, is called a greedy algorithm .
Finding an approximation to the shortest route in The Traveling Salesman Problem by always going to the nearest unvisited neighbor (greedy algorithm).
As you can see by running this simulation a few times, the routes that are found are not completely unreasonable. Except for a few times when the lines cross perhaps, especially towards the end of the algorithm, the resulting route is a lot shorter than we would get by choosing the next city at random.
Finding a near-optimal solution to the Traveling Salesman Problem using the nearest-neighbor algorithm (greedy):
Other Algorithms That Find Near-Optimal Solutions to The Traveling Salesman Problem
In addition to using a greedy algorithm to solve the Traveling Salesman Problem, there are also other algorithms that can find approximations to the shortest route.
These algorithms are popular because they are much more effective than to actually check all possible solutions, but as with the greedy algorithm above, they do not find the overall shortest route.
Algorithms used to find a near-optimal solution to the Traveling Salesman Problem include:
- 2-opt Heuristic: An algorithm that improves the solution step-by-step, in each step removing two edges and reconnecting the two paths in a different way to reduce the total path length.
- Genetic Algorithm: This is a type of algorithm inspired by the process of natural selection and use techniques such as selection, mutation, and crossover to evolve solutions to problems, including the TSP.
- Simulated Annealing: This method is inspired by the process of annealing in metallurgy. It involves heating and then slowly cooling a material to decrease defects. In the context of TSP, it's used to find a near-optimal solution by exploring the solution space in a way that allows for occasional moves to worse solutions, which helps to avoid getting stuck in local minima.
- Ant Colony Optimization: This algorithm is inspired by the behavior of ants in finding paths from the colony to food sources. It's a more complex probabilistic technique for solving computational problems which can be mapped to finding good paths through graphs.
Time Complexity for Solving The Traveling Salesman Problem
To get a near-optimal solution fast, we can use a greedy algorithm that just goes to the nearest unvisited city in each step, like in the second simulation on this page.
Solving The Traveling Salesman Problem in a greedy way like that, means that at each step, the distances from the current city to all other unvisited cities are compared, and that gives us a time complexity of \(O(n^2) \).
But finding the shortest route of them all requires a lot more operations, and the time complexity for that is \(O(n!)\), like mentioned earlier, which means that for 4 cities, there are 4! possible routes, which is the same as \(4 \cdot 3 \cdot 2 \cdot 1 = 24\). And for just 12 cities for example, there are \(12! = 12 \cdot 11 \cdot 10 \cdot \; ... \; \cdot 2 \cdot 1 = 479,001,600\) possible routes!
See the time complexity for the greedy algorithm \(O(n^2)\), versus the time complexity for finding the shortest route by comparing all routes \(O(n!)\), in the image below.

But there are two things we can do to reduce the number of routes we need to check.
In the Traveling Salesman Problem, the route starts and ends in the same place, which makes a cycle. This means that the length of the shortest route will be the same no matter which city we start in. That is why we have chosen a fixed city to start in for the simulation above, and that reduces the number of possible routes from \(n!\) to \((n-1)!\).
Also, because these routes go in cycles, a route has the same distance if we go in one direction or the other, so we actually just need to check the distance of half of the routes, because the other half will just be the same routes in the opposite direction, so the number of routes we need to check is actually \( \frac{(n-1)!}{2}\).
But even if we can reduce the number of routes we need to check to \( \frac{(n-1)!}{2}\), the time complexity is still \( O(n!)\), because for very big \(n\), reducing \(n\) by one and dividing by 2 does not make a significant change in how the time complexity grows when \(n\) is increased.
To better understand how time complexity works, go to this page .
Actual Traveling Salesman Problems Are More Complex
The edge weight in a graph in this context of The Traveling Salesman Problem tells us how hard it is to go from one point to another, and it is the total edge weight of a route we want to minimize.
So far on this page, the edge weight has been the distance in a straight line between two points. And that makes it much easier to explain the Traveling Salesman Problem, and to display it.
But in the real world there are many other things that affects the edge weight:
- Obstacles: When moving from one place to another, we normally try to avoid obstacles like trees, rivers, houses for example. This means it is longer and takes more time to go from A to B, and the edge weight value needs to be increased to factor that in, because it is not a straight line anymore.
- Transportation Networks: We usually follow a road or use public transport systems when traveling, and that also affects how hard it is to go (or send a package) from one place to another.
- Traffic Conditions: Travel congestion also affects the travel time, so that should also be reflected in the edge weight value.
- Legal and Political Boundaries: Crossing border for example, might make one route harder to choose than another, which means the shortest straight line route might be slower, or more costly.
- Economic Factors: Using fuel, using the time of employees, maintaining vehicles, all these things cost money and should also be factored into the edge weights.
As you can see, just using the straight line distances as the edge weights, might be too simple compared to the real problem. And solving the Traveling Salesman Problem for such a simplified problem model would probably give us a solution that is not optimal in a practical sense.
It is not easy to visualize a Traveling Salesman Problem when the edge length is not just the straight line distance between two points anymore, but the computer handles that very well.

COLOR PICKER

Contact Sales
If you want to use W3Schools services as an educational institution, team or enterprise, send us an e-mail: [email protected]
Report Error
If you want to report an error, or if you want to make a suggestion, send us an e-mail: [email protected]
Top Tutorials
Top references, top examples, get certified.
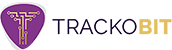
What is a Travelling Salesman Problem (TSP)? Explained!
- Author: Diksha Bhandari
- Read Time: 9 min
- Published: September 14, 2023
- Last Update: November 8th, 2023
Table of Contents
- Asset Tracking
- Dispatch Management
- Driver Behaviour
- Electric Vehicle
- Employee Management
- Field Sales And Service
- Fleet Management
- Fuel Management
- GPS Software
- GPS Trackers and Hardware
- Last Mile Delivery
- Lead Management
- Leave And Attendance
- Roster Management
- Route Optimisation
- Route Planning
- Sensor Integration
- Task and Workflow
- Tech and Beyond
- TrackoBit Industries
- TrackoField
- TrackoField Industries
- TrackoMile Industries
- Vehicle Tracking
- Video telematics
- What's New
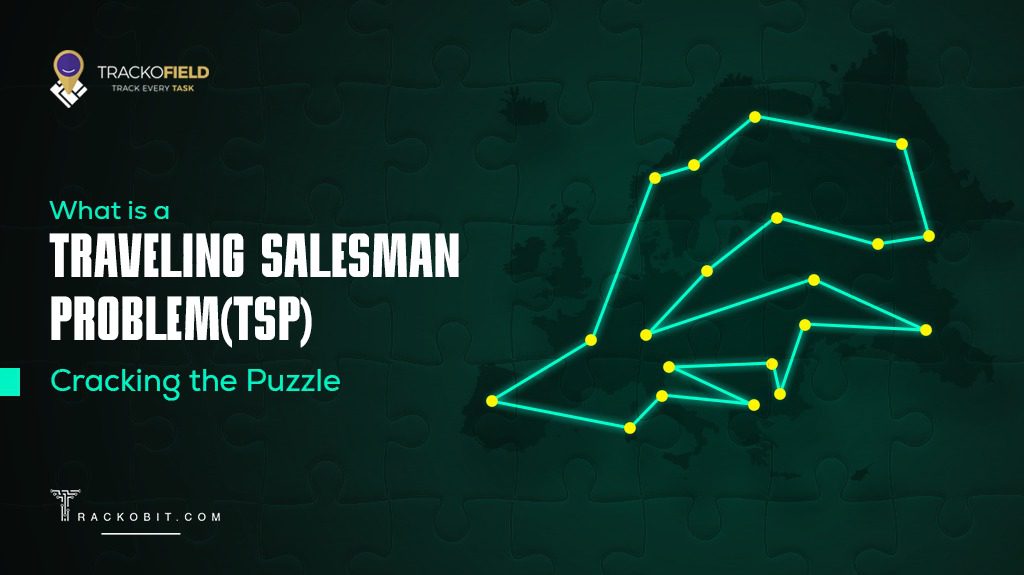
Want to know what a travelling salesman problem (TSP) is? Need solutions to real-life TSP challenges. Learn here.
Do you also look for the shortest route on Google Maps before embarking on a trip?
I am sure, you know multiple routes to reach the office, the mall, or your desired location, but checking on the internet before leaving home has become a ritual. It becomes all the more important to refer to maps when you have to pick up friends or colleagues along the way.
‘ADD STOPS’
Yes, you are right! 💯
That’s what solving the TSP challenge using software means!
What is a Travelling Salesman Problem (TSP)?
The traveling salesman problem is the popular combinatorial optimisation challenge in mathematics and computer science. The prime objective of the problem is to determine the shortest possible route a salesperson must take to cover a set of locations in one go and then return to the starting point.
Addressing travelling salesman challenges and their optimisation are more relevant in this time and age, especially in the supply chain, logistics and delivery space.
TSP may result in delayed deliveries and slimming profits as it’s not easy for delivery agents to choose the most viable and cost-effective route in real-time.
What are Traveling Salesman Problem Challenges to Solve?
When a salesperson is in the field hopping from one client site to another, finding out the best and the shortest route is an added pressure on the agent. In today’s day and age, distance isn’t the only factor that defines efficiency. There are several factors, such as time, fuel consumption, capacity, etc. that together define efficiency.
However, addressing the travelling salesman challenges involves mitigating a few unavoidable challenges along the way that field agents face themselves.
1. Time Constraints
Sales agents often have a tight schedule with multiple deliveries to make with a short TAT. Similarly, in TSP, optimising routes to minimise travel time is a fundamental challenge.
2. Last-minute Changes
Eleventh-hour changes are not a new concept for salespeople. They encounter urgent visits and last-minute cancellations a lot. Similarly, TSP solutions must be adaptable to handle dynamic scenarios and route modifications.
3. Resource Efficiency
Just as salespersons aim at reducing fuel costs and ensuring on-time deliveries, TSP solutions such as TrackoMile must strive for resource optimisation by reducing travel distances and delivery TAT.
4. Objective Diversification
While solving the travelling salesman problem (TSP) , optimising multiple objectives such as cost, time, and environmental factors adds complexity as solutions need to balance conflicting goals.
5. Combinatorial Complexity
TSP is a combinatorial optimisation problem, which means it involves complicated mathematical calculations with numerous variables. Sometimes, complex scenarios get further intricate as multiple variables are involved.
6. Adaptability and Scalability
Similarly, how sales agents adjust to the routes on the fly, the route algorithm must be flexible and responsive to real-time changes such as spiking volumes, vehicle breakdown or traffic slow down. A TSP solution must have a good appetite to handle large datasets and complex networks.
Also Read 4 Key Solutions for Fuel Management System 2023
Top 5 Solutions to The Travelling Salesman Problem
The traveling salesman problem solutions offer various trade-offs between computational intricacies and the quality of the resolution, allowing practitioners to choose the best-suited approach based on their needs and problems.
Here are the Top 5 solutions to the Traveling Salesman Problem (TSP) :
1. Brute Force Algorithm
The Brute Force algorithm is a straight approach to solving the Traveling Salesman Problem (TSP). It systematically explores all possible routes to identify the shortest one among them all. While it guarantees an optimal solution, its downside lies in its major time complexity, making it practical only for small TSP challenges.
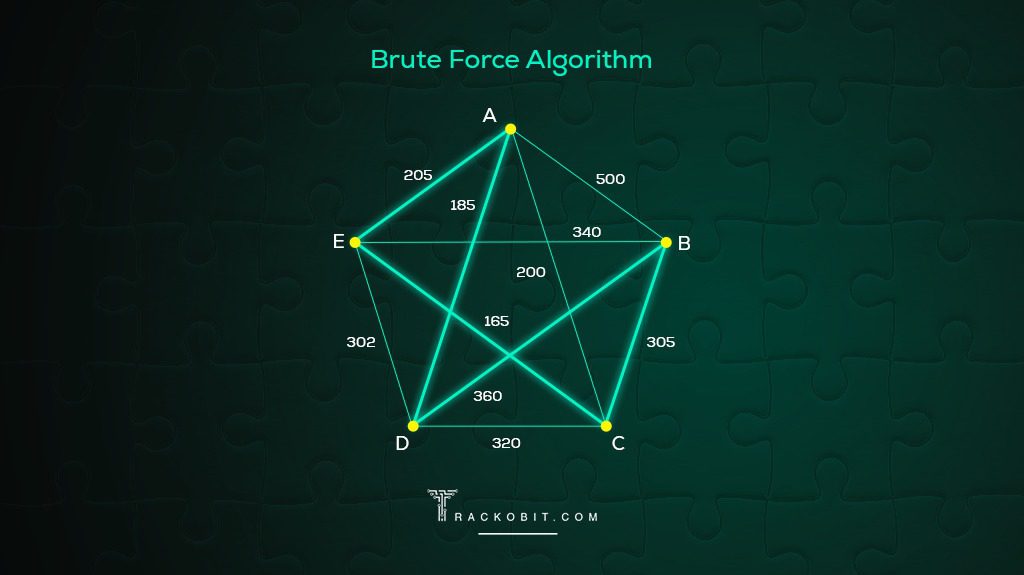
2. Nearest Neighbour Algorithm
The Nearest Neighbour method is the simplest heuristic for the TSP. It starts from the first location and repeatedly selects the closest unvisited location to form a tour. Although it is quick to implement this method, it may always yield the optimal solution for it prioritises proximity over other factors.
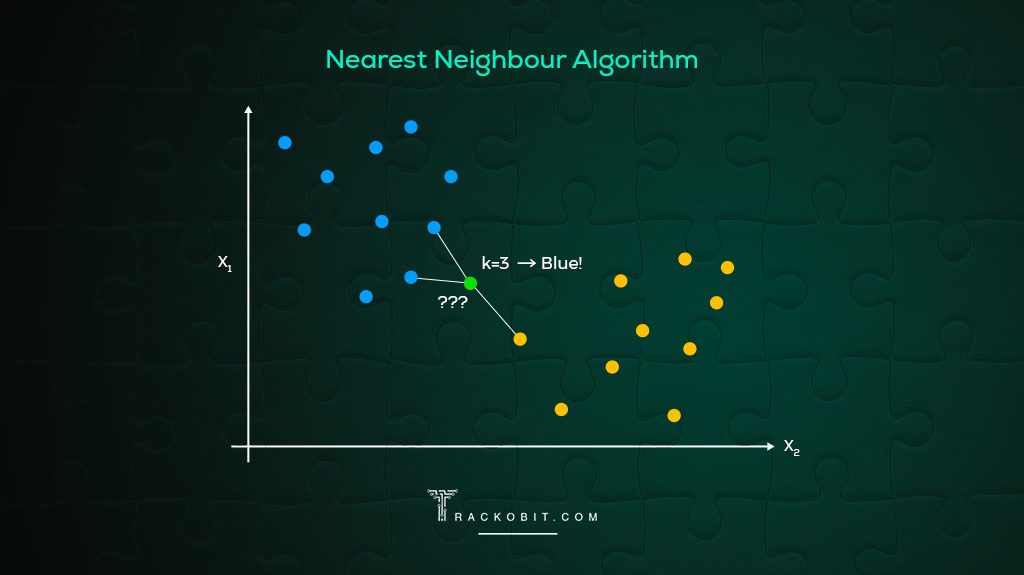
3. Genetic Algorithm
This technique or method draws inspiration from nature itself. They evolve TSP solutions through selection, crossovers and mutation. They pick the best routes and mix them up. This creates new routes that might be even better. Then, they keep the best ones and repeat the mixing and picking process. Survival of the fittest in the true sense.
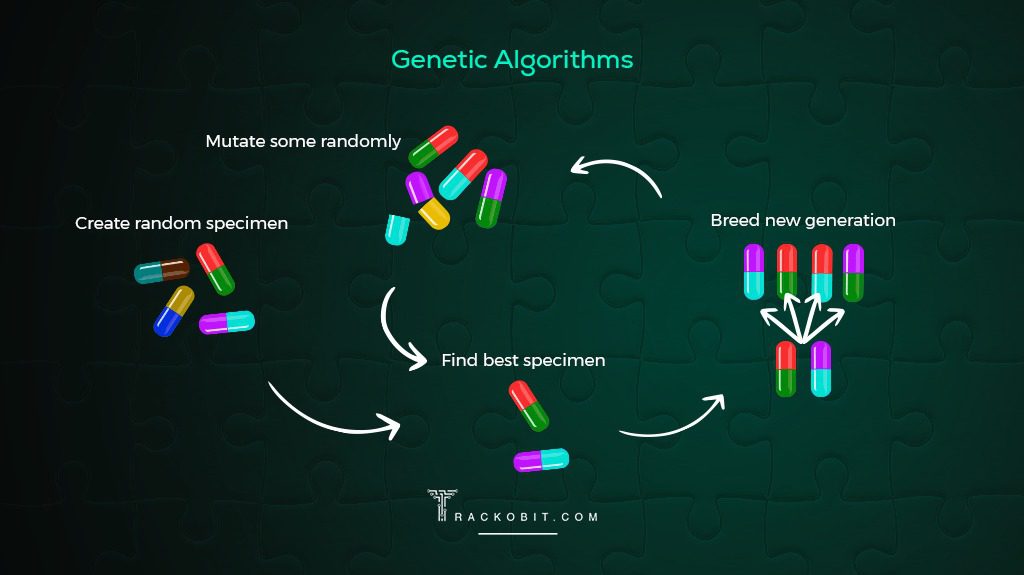
4. Ant Colony Optimisation (ACO)
Ants have a tendency to leave pheromones on the shorter routes they find, calling fellow ants on the same route. They keep leaving more pheromones on the shorter routes they find. Over time, the collective behaviour of the ants causes them to converge on the shortest route. Inspired by the nature of ants, ACO finds the shortest route by analysing the trails of data left by artificial ants based on the strength of these data trails.
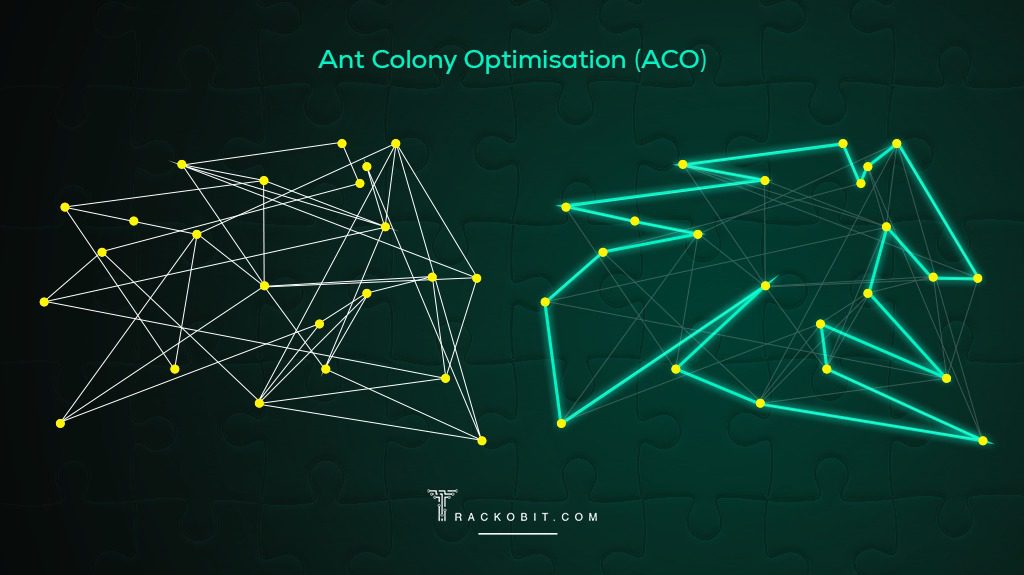

5. Dynamic Programming
Dynamic Programming is like solving a puzzle, step-by-step, by breaking it into smaller pieces. In TSP challenges, it finds the best route to visit all locations. It begins with figuring out the shortest route between two locations; then it builds on that to find ways to more locations. It’s a smart TSP solution for small scenarios but may require significant memory resources for larger and more complex problems.
What Are Real-world Travelling Salesman Problem Applications?
The Traveling Salesman Problem (TSP) has a wide array of applications across various domains due to its relevance in optimising routes and sequences. Here are several crucial real-word TSP applications and implementations in the real world.
1. TSP implementation in Logistics and Delivery Services
The logistics and supply chain sectors have the widest TSP applications.
- Courier, Express & Parcel : Companies like FedEx, UPS, and DHL rely on TSP algorithms to optimise delivery routes for their fleet of delivery trucks. By finding the most efficient sequence of stops, they minimise fuel consumption , reduce delivery TAT, and save on operational overheads too.
- On-demand Delivery : Food delivery companies, instant grocery delivery apps and at-home appointment platforms like Swiggy, BlinkIt and UrbanCompany, respectively, leverage TSP solutions to ensure timely delivery. Enhancing the customer experience and increasing the number of deliveries each rider can make.
2. TSP Applications in Transportation and Urban Planning Waste collection routes, Traffic light synchronisation, optic cable installation, etc. are some areas where TSP Solutions works like a knight in shining armour. Other real-world TSP applications include
- Public Transport : City planners and public transport agencies use TSP principles to design bus, tram and train routes that reduce travel for passengers.
- Emergency Service Dispatch : Ambulance services, Police PCR vans employ TSP algorithms to dispatch vehicles quickly and efficiently in response to emergency calls. Finding the shortest route to reach the incident location can save lives.
- Urban Mobility Solution : In the era of ride-sharing and on-demand mobility apps like Uber, Ola, Lyft, etc., real-world TSP applications become prominent. TSP solutions optimise the route to destinations, ensuring quick and cost-effective transportation.
Other significant real-life applications of the Travelling Salesman Problem are
- TSP in Healthcare and Medical Research – for DNA sequencing and understanding genetic patterns and diseases.
- TSP in Manufacturing and Production – In circuit board manufacturing and job scheduling of technicians.
- TSP in Robotics and Autonomous Vehicles -Self-driving cars and drones use TSP-like algorithms for efficient navigation.
Solving the Travelling Salesman Problem – Last Mile Delivery Route Optimisation
Route optimisation is the key to efficient last-mile delivery . In order to attain flawless route optimisation, the software must solve the traveling salesman problem every step of the way.
Why it’s essential to solve TSP for Last Mile Delivery?
In simple and minimal words, solving TSP problems helps in many ways:
- Saves Time : It makes deliveries faster, so your customers get orders sooner.
- Customer Satisfaction : Fast deliveries give you an edge over the competition and enhance customer experience too.
- Saves Money : It reduces fuel wastage and vehicle wear, making deliveries cheaper.
- Environment Friendly : It lowers pollution by using fewer vehicles and shorter routes.
- Happy Staff: Drivers and dispatchers have less stress and can finish their work faster.
How do we solve the travelling salesman problem for last-mile delivery?
Solving TSP challenges for Last-mile delivery is like solving a big jigsaw puzzle. There are a hundred thousand addresses to visit daily. The software must find the shortest and most optimised route to them and come back to the starting point at the end.
- Our route optimisation software , TrackoMile, leverages capacity management , routing algorithms and robust rule engines to present the most optimal combination of delivery addresses. Thereby giving the most optimally planned routes or trips.
- All delivery managers have to do is upload the CSV file of the addresses or integrate TrackoMile to their CRM to fetch the delivery addresses. Now trip allocation, route optimisation, dispatch and everything else happen in a few clicks.
- ETA when the delivery is en route, POD when the order is delivered successfully, and trip analysis, are added features to simplify overall operations.
The Vehicle Routing Problem is very similar to TSP, with wide applications in logistics, delivery services and transportation. While TSP focuses on finding the shortest route for a single traveller visiting various locations, VRP deals with multiple vehicles serving multiple customers, considering added constraints like vehicle capacity, TATs and more.
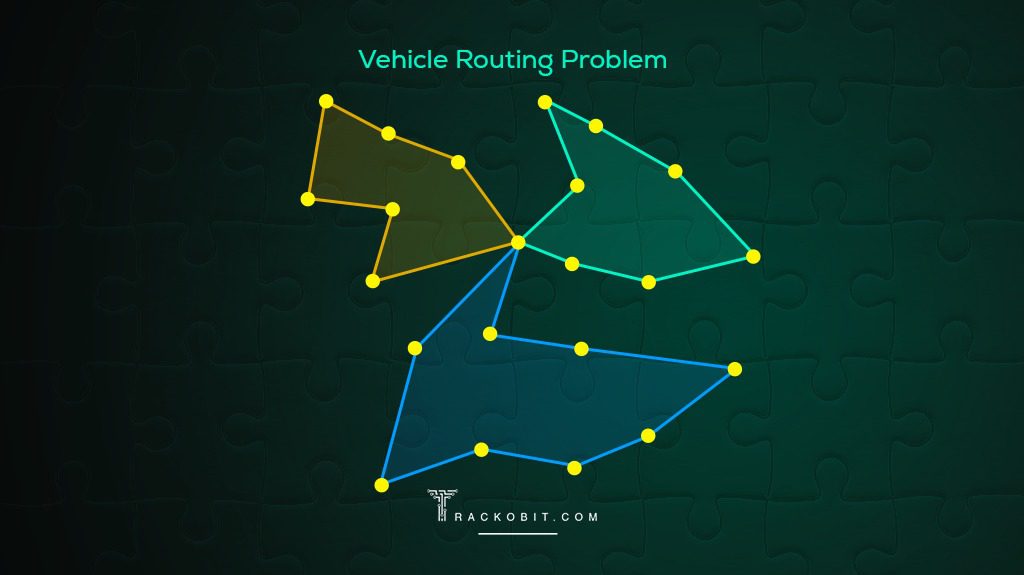
How Can AI Help in Solving Traveling Salesman Problem (TSP)?
AI or Artificial Intelligence are becoming the driving force for business growth across various industrial sectors. AI particularly aids in solving the Traveling Salesman Problem(TSP) in the logistics and delivery sector by employing advanced algorithms and techniques. What are a few tricks up AI’s sleeves that help in automating TSP resolution? Let’s find out!
1. Advanced Algorithms
AI algorithms such as Genetic Algorithms, ACO, simulated annealing and a few others mentioned above, tackle complex Travelling Salesman Problem scenarios.
2. Machine Learning
Gathering information from historical data and optimising routes based on real-time insights is what AI is best for. Machine learning models are trained to adapt to changing conditions, like traffic, weather and delivery constraints, to provide a more accurate plan of action.
3. Parallel Computing
AIi enables the use of a parallel computing process, which means solving multiple segments of TSP simultaneously. This accelerates the problem-solving process for large-scale challenges.
4. Heuristic Improvement
TSP Heuristics powered by AI can groom initial solutions, gradually improving their results over time. These heuristics can be applied iteratively by AI to reach better results.
5. Hybrid Approaches
Applying hybrid algorithms is not a new technique to refine techniques and produce more accurate results. AI on top of it singles out data-oriented combinations that work the best in varied use cases.
Wrapping Up!
The travelling salesman problem’s importance lies in its real-world applications. Whether optimising delivery routes, planning manufacturing processes or organising circuit board drilling, finding the most efficient way to cover multiple locations is crucial to minimise costs and save time.
The TSP problems have evolved over the years, and so have TSP algorithms, heuristics and solutions. With the advent of advanced technologies such as GPS and machine learning, TSP continues to adapt and find new applications in emerging fields, cementing its status as a fundamental problem in optimization theory and a valuable tool for various industries. Mobility automation software like Trackobit, TrackoMile and TrackoField resort to TSP heuristics to solve challenges along the way.
Read Blog – Best Delivery Route Planner Apps for 2023
Traveling Salesman Problem FAQs
What is tsp.
TSP is an abbreviation for Traveling Salesman Problem. It’s the routing problem that deals with finding the shortest route to travel to a combination of locations in the most optimal manner.
Is Travelling Salesman Problem Solvable?
Yes, the Traveling Salesman Problem (TSP) is solvable, but the time to find the solution can grow proportionately with an increase in the number of locations. With the evolution of travelling salesman problem applications, various TSP algorithms and heuristics, their hybrids have also emerged.
Wh at is the objective of TSP?
The objective of the Traveling Salesman Problem (TSP) is to find the shortest possible route that covers all given locations or waypoints and comes back to the starting point with the least resource utilisation.
Diksha Bhandari
Currently creating SaaSy content strategies for TrackoBit and TrackoField, this content professional has dedicated a decade of her life to enriching her portfolio and continues to do so. In addition to playing with words and spilling SaaS, she has a passion for traveling to the mountains and sipping on adrak wali chai.
- Author Showcase
- All Blog Post
Never Miss a Beat
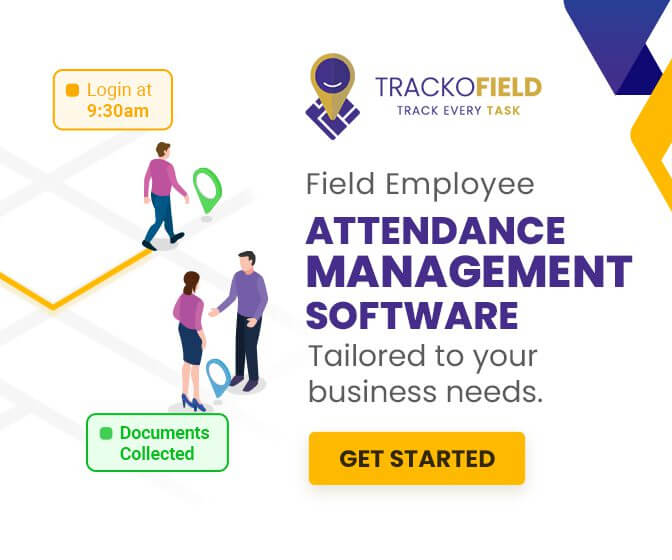
Related Blog
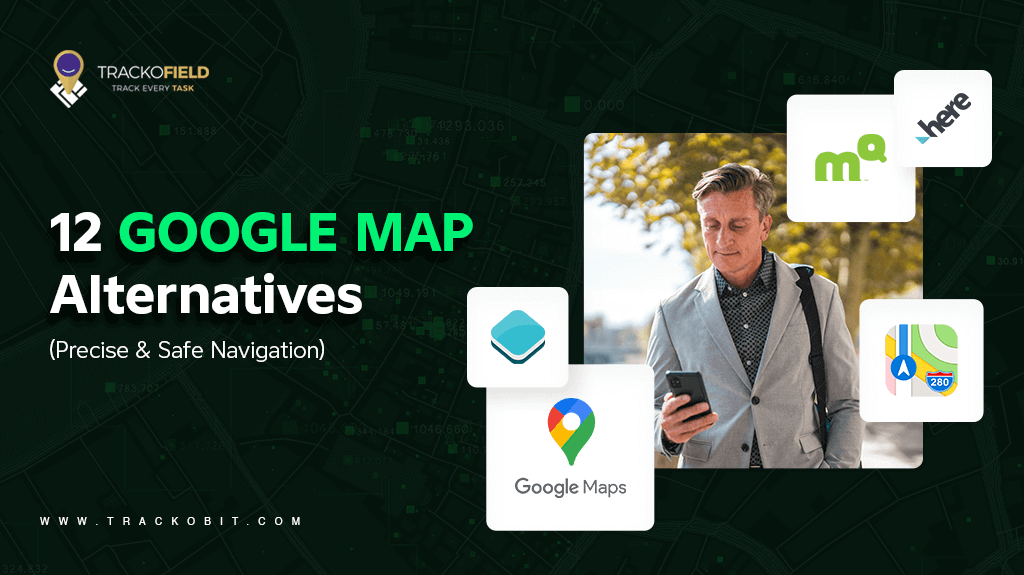
Top 12 Google Map Alternatives – Offering Precise Navigation
Explore our best picks for 12 free Google map alternatives that offer accurate and secure location and navigational solutions.
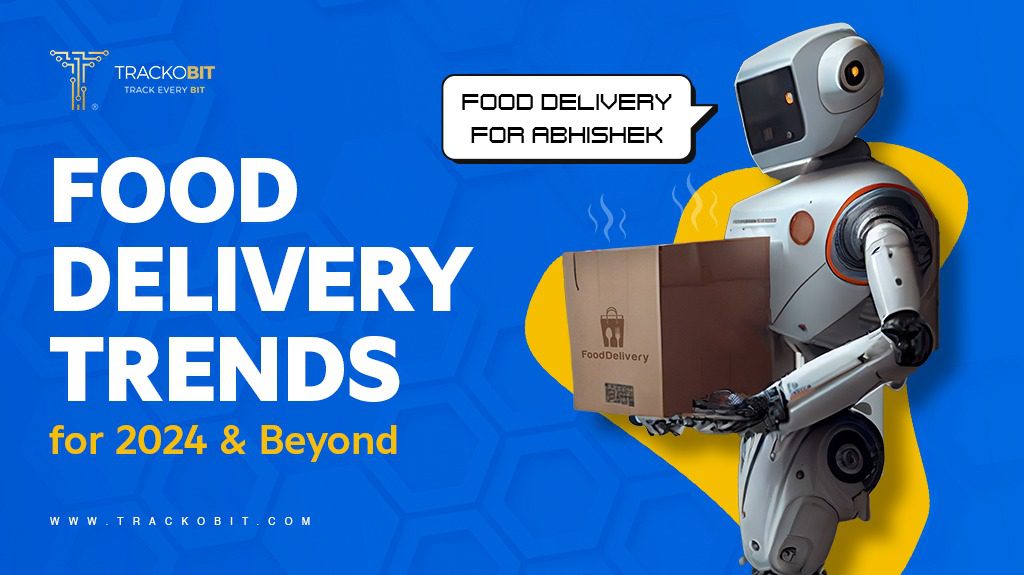
Food Delivery Trends to Watch in 2024 & Beyond
Food and technology are both revolving along with consumer demands. Here are some of the food delivery trends to watch in 2024.
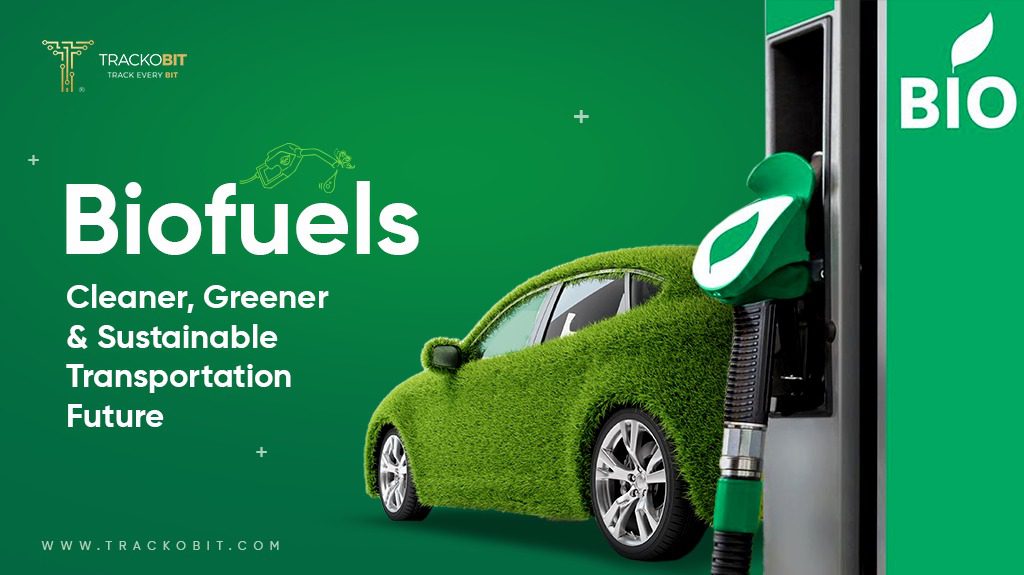
Biofuel – An Alternative to Fossil Fuels in Transportation | G20
Transportation industries are moving towards a greener future through the sustainable use of biofuels like Ethanol — discussed in the G20 2023 summit.
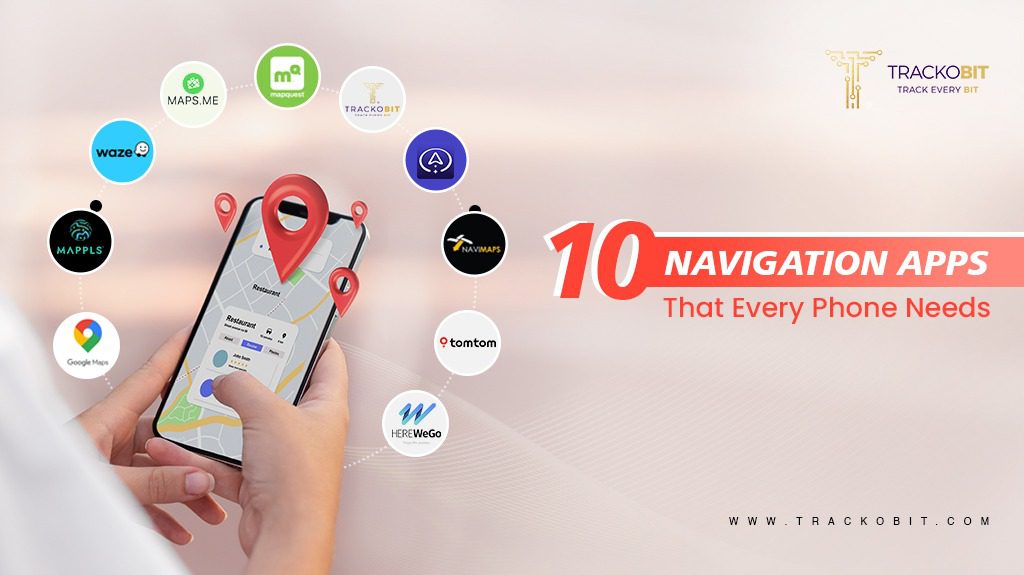
Top 10 Navigation Apps for Android and iOS | 2024
Discover the 10 best navigation apps in 2024 that offer personalised navigation just like Sherpas (experienced guides of Himalayan terrain) do.
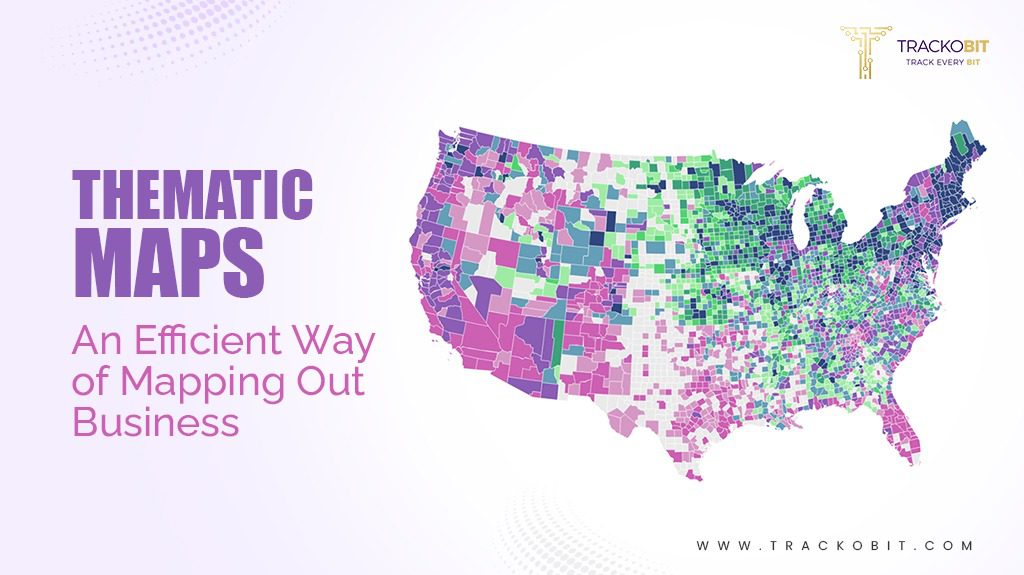
What are Thematic Maps? Types, Applications And Advantages
Maps have come a long way, and their usage has gone beyond just navigating distances. Thematic maps are now being used to interpret data, gain information, etc.
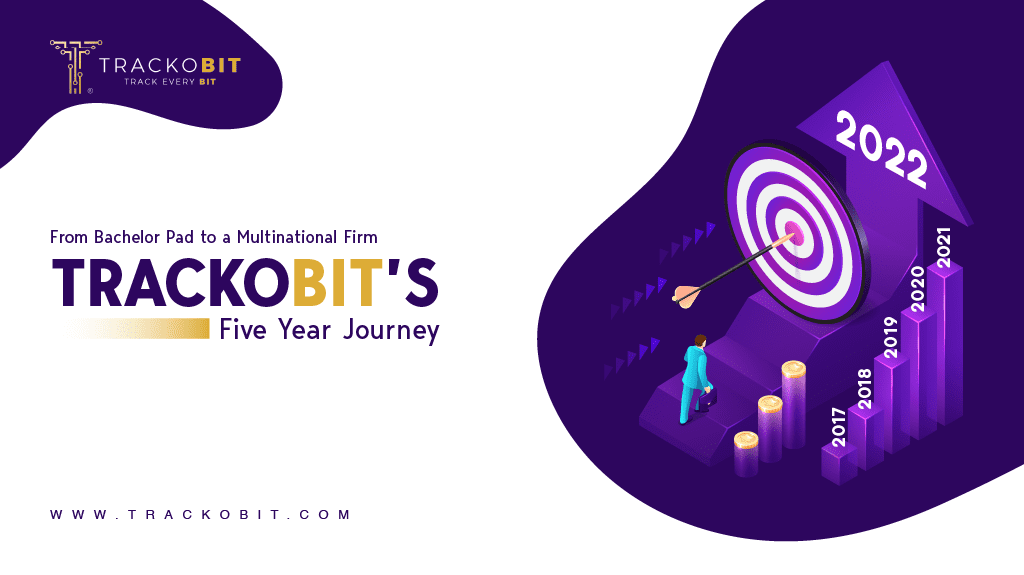
From a Bachelor Pad to a Multinational Firm: Tracking TrackoBit’s 5-year Journey
“It’s when ordinary people rise above the expectations and seize the opportunity that milestones truly are reached.” – Mike Huckabee
Stay Updated on tech, telematics and mobility. Don't miss out on the latest in the industry.

Thank you for reaching out! We'll speak to you soon.
In the meantime, why not find out more about us, explore our products, or visit our blog?
Cookie Consent
We use cookies to enhance and personalize your browsing experience. By continuing to use our website, you agree to our Privacy Policy.
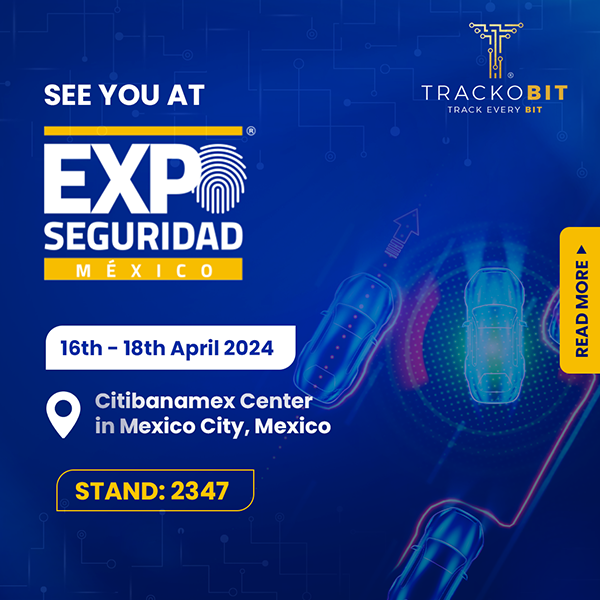
Traveling Salesman Problem with Python: Greedy and Brute Force

Just imagine yourself as a delivery courier. You have to deliver multiple parcels to many different spots. You also aim to minimize the fuel costs and time of traveling to maximize profit. This generally creates confusion about where to deliver parcels first and which routes to take.
In this article, we will learn about the famous problem of Travelling Salesman. We will solve this issue using Python programming language. We will also try to plot the best route to be taken and calculate the minimum distance to be traveled.
Recommended: Connected Health: Exploring IoT Solutions for Healthcare Transformation
Recommended: Delivery Route Optimization using Python: A Step-by-Step Guide
Solving the Traveling Salesman Problem with Python
The problem statement is that a salesman has to travel to the Indian cities of Mumbai, Delhi, Bangalore, Hyderabad, Ahmedabad, Chennai, Kolkata, Surat, Pune, and Jaipur to sell some products.
The objective of the problem is to minimize the total distance travelled by the salesman.
This describes our problem statement. Now let’s move on to how to solve this problem using Python programming language.
We will look at two ways of solving the problem – using the Greedy algorithm and the Brute Force method ( this method is the most preferred one as it provides more optimal solutions ).
Example Usage and Visualization of the Greedy Algorithm
Let us look at the code of the Simple Greedy algorithm.
In the above example, we randomly input some coordinates and calculated the best route. Let us look at the output of this method.
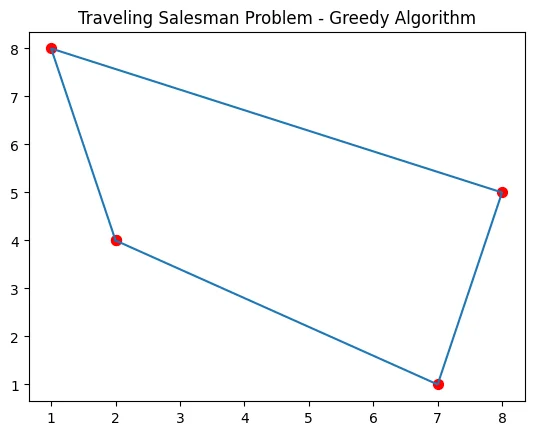
Thus, according to the Greedy algorithm, we will travel to city 1 first, and cities 1,3,2 after that.
Brute Force Approach to the Traveling Salesman Problem
Let us look at the code of the Brute Force Method.
Let us look at the output of the Brute Force method.

Thus, according to the Brute force method, the minimum distance is approximately 230 units.
Greedy Algorithm vs. Brute Force Method
The difference between the Greedy algorithm and the Brute Force method is that the Greedy algorithm builds up the solution step by step whereas in the Brute Force method, all the permutations of the solution are found and then the solution with minimum distance is selected.
Here you go! Now you know the Travelling Salesman Problem and how to solve it using Python. In this article, you learned about two methods of approach i.e. Greedy algorithm and Travelling Salesman problem.
Hope you enjoyed reading it!
Recommended: Backtracking Line Search Algorithm for Unconstrained Optimization
Recommended: Large integer handling in Python (optimization)

- Interview Q
DAA Tutorial
Asymptotic analysis, analysis of sorting, divide and conquer, lower bound theory, sorting in linear time, binary search trees, red black tree, dynamic programming, greedy algorithm, backtracking, shortest path, all-pairs shortest paths, maximum flow, sorting networks, complexity theory, approximation algo, string matching.
Interview Questions
- Send your Feedback to [email protected]
Help Others, Please Share

Learn Latest Tutorials

Transact-SQL

Reinforcement Learning

R Programming

React Native

Python Design Patterns

Python Pillow

Python Turtle

Preparation

Verbal Ability

Company Questions
Trending Technologies

Artificial Intelligence

Cloud Computing

Data Science

Machine Learning

B.Tech / MCA

Data Structures

Operating System

Computer Network

Compiler Design

Computer Organization

Discrete Mathematics

Ethical Hacking

Computer Graphics

Software Engineering

Web Technology

Cyber Security

C Programming

Control System

Data Mining

Data Warehouse


- Data Structures & Algorithms
- DSA - Overview
- DSA - Environment Setup
- DSA - Algorithms Basics
- DSA - Asymptotic Analysis
- Data Structures
- DSA - Data Structure Basics
- DSA - Data Structures and Types
- DSA - Array Data Structure
- Linked Lists
- DSA - Linked List Data Structure
- DSA - Doubly Linked List Data Structure
- DSA - Circular Linked List Data Structure
- Stack & Queue
- DSA - Stack Data Structure
- DSA - Expression Parsing
- DSA - Queue Data Structure
- Searching Algorithms
- DSA - Searching Algorithms
- DSA - Linear Search Algorithm
- DSA - Binary Search Algorithm
- DSA - Interpolation Search
- DSA - Jump Search Algorithm
- DSA - Exponential Search
- DSA - Fibonacci Search
- DSA - Sublist Search
- DSA - Hash Table
- Sorting Algorithms
- DSA - Sorting Algorithms
- DSA - Bubble Sort Algorithm
- DSA - Insertion Sort Algorithm
- DSA - Selection Sort Algorithm
- DSA - Merge Sort Algorithm
- DSA - Shell Sort Algorithm
- DSA - Heap Sort
- DSA - Bucket Sort Algorithm
- DSA - Counting Sort Algorithm
- DSA - Radix Sort Algorithm
- DSA - Quick Sort Algorithm
- Graph Data Structure
- DSA - Graph Data Structure
- DSA - Depth First Traversal
- DSA - Breadth First Traversal
- DSA - Spanning Tree
- Tree Data Structure
- DSA - Tree Data Structure
- DSA - Tree Traversal
- DSA - Binary Search Tree
- DSA - AVL Tree
- DSA - Red Black Trees
- DSA - B Trees
- DSA - B+ Trees
- DSA - Splay Trees
- DSA - Tries
- DSA - Heap Data Structure
- DSA - Recursion Algorithms
- DSA - Tower of Hanoi Using Recursion
- DSA - Fibonacci Series Using Recursion
- Divide and Conquer
- DSA - Divide and Conquer
- DSA - Max-Min Problem
- DSA - Strassen's Matrix Multiplication
- DSA - Karatsuba Algorithm
- Greedy Algorithms
- DSA - Greedy Algorithms
- DSA - Travelling Salesman Problem (Greedy Approach)
- DSA - Prim's Minimal Spanning Tree
- DSA - Kruskal's Minimal Spanning Tree
- DSA - Dijkstra's Shortest Path Algorithm
- DSA - Map Colouring Algorithm
- DSA - Fractional Knapsack Problem
- DSA - Job Sequencing with Deadline
- DSA - Optimal Merge Pattern Algorithm
- Dynamic Programming
- DSA - Dynamic Programming
- DSA - Matrix Chain Multiplication
- DSA - Floyd Warshall Algorithm
- DSA - 0-1 Knapsack Problem
- DSA - Longest Common Subsequence Algorithm
- DSA - Travelling Salesman Problem (Dynamic Approach)
- Approximation Algorithms
- DSA - Approximation Algorithms
- DSA - Vertex Cover Algorithm
- DSA - Set Cover Problem
- DSA - Travelling Salesman Problem (Approximation Approach)
- Randomized Algorithms
- DSA - Randomized Algorithms
- DSA - Randomized Quick Sort Algorithm
- DSA - Karger’s Minimum Cut Algorithm
- DSA - Fisher-Yates Shuffle Algorithm
- DSA Useful Resources
- DSA - Questions and Answers
- DSA - Quick Guide
- DSA - Useful Resources
- DSA - Discussion
- Selected Reading
- UPSC IAS Exams Notes
- Developer's Best Practices
- Questions and Answers
- Effective Resume Writing
- HR Interview Questions
- Computer Glossary
Travelling Salesman Problem (Greedy Approach)
Travelling salesperson algorithm.
The travelling salesman problem is a graph computational problem where the salesman needs to visit all cities (represented using nodes in a graph) in a list just once and the distances (represented using edges in the graph) between all these cities are known. The solution that is needed to be found for this problem is the shortest possible route in which the salesman visits all the cities and returns to the origin city.
If you look at the graph below, considering that the salesman starts from the vertex ‘a’, they need to travel through all the remaining vertices b, c, d, e, f and get back to ‘a’ while making sure that the cost taken is minimum.
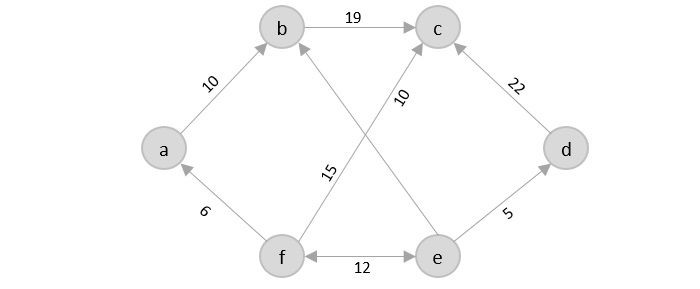
There are various approaches to find the solution to the travelling salesman problem: naive approach, greedy approach, dynamic programming approach, etc. In this tutorial we will be learning about solving travelling salesman problem using greedy approach.
As the definition for greedy approach states, we need to find the best optimal solution locally to figure out the global optimal solution. The inputs taken by the algorithm are the graph G {V, E}, where V is the set of vertices and E is the set of edges. The shortest path of graph G starting from one vertex returning to the same vertex is obtained as the output.
Travelling salesman problem takes a graph G {V, E} as an input and declare another graph as the output (say G’) which will record the path the salesman is going to take from one node to another.
The algorithm begins by sorting all the edges in the input graph G from the least distance to the largest distance.
The first edge selected is the edge with least distance, and one of the two vertices (say A and B) being the origin node (say A).
Then among the adjacent edges of the node other than the origin node (B), find the least cost edge and add it onto the output graph.
Continue the process with further nodes making sure there are no cycles in the output graph and the path reaches back to the origin node A.
However, if the origin is mentioned in the given problem, then the solution must always start from that node only. Let us look at some example problems to understand this better.
Consider the following graph with six cities and the distances between them −
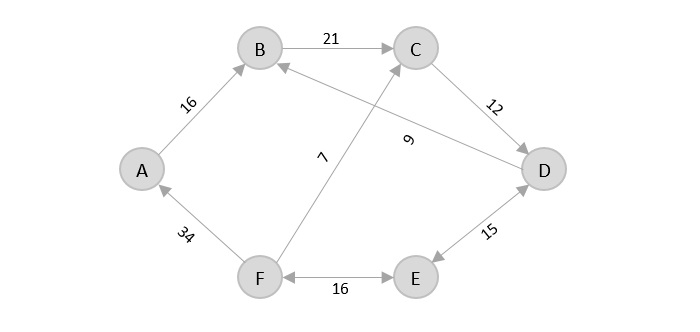
From the given graph, since the origin is already mentioned, the solution must always start from that node. Among the edges leading from A, A → B has the shortest distance.
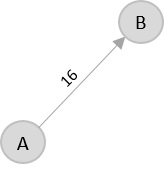
Then, B → C has the shortest and only edge between, therefore it is included in the output graph.
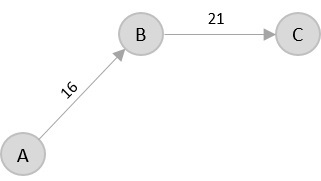
There’s only one edge between C → D, therefore it is added to the output graph.
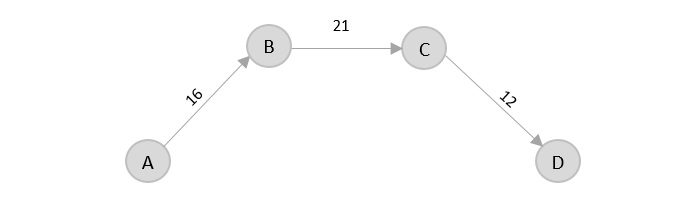
There’s two outward edges from D. Even though, D → B has lower distance than D → E, B is already visited once and it would form a cycle if added to the output graph. Therefore, D → E is added into the output graph.
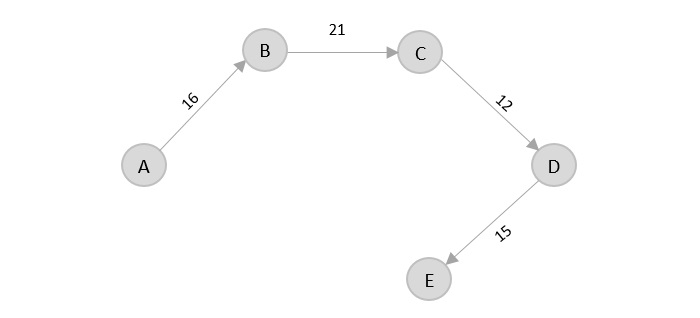
There’s only one edge from e, that is E → F. Therefore, it is added into the output graph.
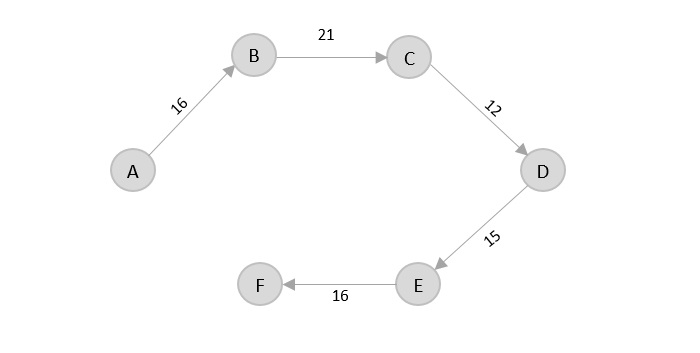
Again, even though F → C has lower distance than F → A, F → A is added into the output graph in order to avoid the cycle that would form and C is already visited once.
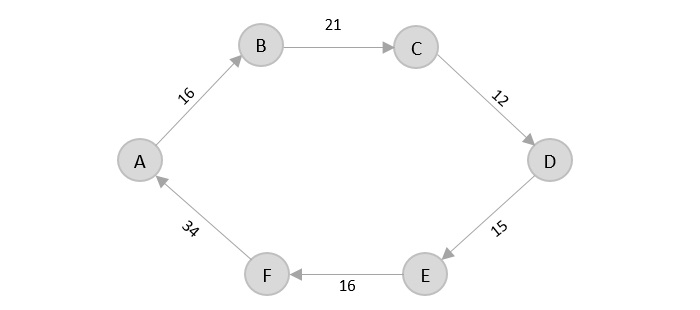
The shortest path that originates and ends at A is A → B → C → D → E → F → A
The cost of the path is: 16 + 21 + 12 + 15 + 16 + 34 = 114.
Even though, the cost of path could be decreased if it originates from other nodes but the question is not raised with respect to that.
The complete implementation of Travelling Salesman Problem using Greedy Approach is given below −
- Data Structures
- Linked List
- Binary Tree
- Binary Search Tree
- Segment Tree
- Disjoint Set Union
- Fenwick Tree
- Red-Black Tree
- Advanced Data Structures
Travelling Salesman Problem (TSP) using Reduced Matrix Method
- Travelling Salesman Problem using Hungarian method
- Travelling Salesman Problem implementation using BackTracking
- Traveling Salesman Problem using Genetic Algorithm
- Traveling Salesman Problem (TSP) Implementation
- Traveling Salesman Problem using Branch And Bound
- Travelling Salesman Problem using Dynamic Programming
- Approximate solution for Travelling Salesman Problem using MST
- Bitonic Travelling Salesman Problem
- Travelling Salesman Problem | Greedy Approach
- Proof that traveling salesman problem is NP Hard
- Bitmasking and Dynamic Programming | Travelling Salesman Problem
- Transportation Problem | Set 4 (Vogel's Approximation Method)
- Transportation Problem | Set 6 (MODI Method - UV Method)
- Transportation Problem | Set 3 (Least Cost Cell Method)
- Transportation Problem Set 8 | Transshipment Model-1
- Implement a parallel programming task using graphs
- Transportation Problem | Set 2 (NorthWest Corner Method)
- Transportation Problem | Set 1 (Introduction)
- Java Program to Solve Travelling Salesman Problem Using Incremental Insertion Method
- Introduction to Backtracking - Data Structure and Algorithm Tutorials
- 8 puzzle Problem using Branch And Bound
- 0/1 Knapsack using Branch and Bound
- Job Assignment Problem using Branch And Bound
- Difference between Backtracking and Branch-N-Bound technique
- Implementation of 0/1 Knapsack using Branch and Bound
- N Queen Problem using Branch And Bound
- 0/1 Knapsack using Least Cost Branch and Bound
- Introduction to Branch and Bound - Data Structures and Algorithms Tutorial
Given a set of cities and the distance between every pair of cities, the problem is to find the shortest possible route that visits every city exactly once and returns to the starting point.
Input: Example of connections of cities Output: 80 Explanation: An optimal path is 1 – 2 – 4 – 3 – 1.
Dynamic Programming Approach: This approach is already discussed in Set-1 of this article.
Branch and Bound Approach: The branch and bound approach is already discussed in this article .
Reduced Matrix: This approach is similar to the Branch and Bound approach. The difference here is that the cost of the path and the bound is decided based on the method of matrix reduction. The following are the assumptions for a reduced matrix:
- A row or column of the cost adjacency matrix is said to be reduced if and only if it contains at least one zero element and all remaining entries in that row or column ≥ 0.
- If all rows and columns are reduced then only the matrix is reduced matrix.
- Tour length (new) = Tour length (old) – Total value reduced.
- We first rewrite the original cost adjacency matrix by replacing all diagonal elements from 0 to Infinity
The basic idea behind solving the problem is:
The cost to reduce the matrix initially is the minimum possible cost for the travelling salesman problem. Now in each step, we need to decide the minimum possible cost if that path is taken i.e., a path from vertex u to v is followed. We can do that by replacing uth row and vth column cost by infinity and then further reducing the matrix and adding the extra cost for reduction and cost of edge (u, v) with the already calculated minimum path cost. Once at least one path cost is found, that is then used as upper bound of cost to apply the branch and bound method on the other paths and the upper bound is updated accordingly when a path with lower cost is found.
Following are the steps to implement the above procedure:
- Step1: Create a class (Node) that can store the reduced matrix, cost, current city number, level (number of cities visited so far), and path visited till now.
- Step2: Create a priority queue to store the live nodes with the minimum cost at the top.
- Row reduction – Find the min value for each row and store it. After finding the min element from each row, subtract it from all the elements in that specific row.
- Column reduction – Find the min value for each column and store it. After finding the min element from each column, subtract it from all the elements in that specific column. Now the matrix is reduced.
- Now add all the minimum elements in the row and column found earlier to get the cost.
- Step4: Push the element with all information required by Node into the Priority Queue.
- Pop the element with the min value from the priority queue.
- For each pop operation check whether the level of the current node is equal to the number of nodes/cities or not.
- If yes then print the path and return the minimum cost.
- The cost of a reduced Matrix can be calculated by converting all the values of its rows and column to infinity and also making the index Matrix[Col][row] = infinity.
- Then again push the current node into the priority queue.
- Step6: Repeat Step5 till we don’t reach the level = Number of nodes – 1.
Follow the illustration below for a better understanding.
Illustration:
Consider the connections as shown in the graph: Example of connections Initially the cost matrix looks like: row/col no 1 2 3 4 1 – 10 15 20 2 10 – 35 25 3 15 35 – 30 4 20 25 30 – After row and column reduction the matrix will be: row/col no 1 2 3 4 1 – 0 5 10 2 0 – 25 15 3 0 20 – 15 4 0 5 10 – and row minimums are 10, 10, 15 and 20. row/col no 1 2 3 4 1 – 0 0 0 2 0 – 20 5 3 0 20 – 5 4 0 5 5 – and the column minimums are 0, 0, 5 and 10. So the cost reduction of the matrix is (10 + 10 + 15 + 20 + 5 + 10) = 70 Now let us consider movement from 1 to 2: Initially after substituting the 1st row and 2nd column to infinity, the matrix will be: row/col no 1 2 3 4 1 – – – – 2 – – 20 5 3 0 – – 5 4 0 – 5 – After the matrix is reduced the row minimums will be 5, 0, 0 row/col no 1 2 3 4 1 – – – – 2 – – 15 0 3 0 – – 5 4 0 – 5 – and the column minimum will be 0, 5, 0 row/col no 1 2 3 4 1 – – – – 2 – – 10 0 3 0 – – 5 4 0 – 0 – So the cost will be 70 + cost (1, 2) + 5 + 5 = 70 + 0 + 5 + 5 = 80. Continue this process till the traversal is complete and find the minimum cost. Given below the structure of the recursion tree along with the bounds: The recursion diagram with bounds
Below is the implementation of the above approach.
Time Complexity: O(2 N * N 2 ) where N = number of node/ cities. Space Complexity: O(N 2 )
Please Login to comment...
Similar reads.
- Branch and Bound
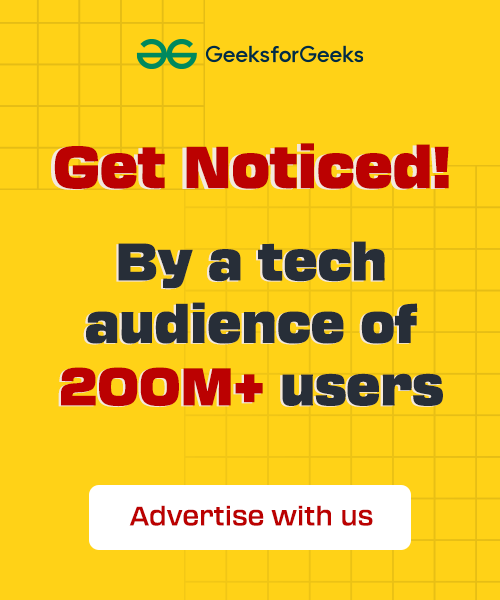
Improve your Coding Skills with Practice
What kind of Experience do you want to share?
Thank you for visiting nature.com. You are using a browser version with limited support for CSS. To obtain the best experience, we recommend you use a more up to date browser (or turn off compatibility mode in Internet Explorer). In the meantime, to ensure continued support, we are displaying the site without styles and JavaScript.
- View all journals
- My Account Login
- Explore content
- About the journal
- Publish with us
- Sign up for alerts
- Open access
- Published: 24 April 2024
Energy-efficient superparamagnetic Ising machine and its application to traveling salesman problems
- Jia Si ORCID: orcid.org/0000-0003-0737-4905 1 , 2 ,
- Shuhan Yang 1 ,
- Yunuo Cen 1 ,
- Jiaer Chen 1 ,
- Yingna Huang 1 ,
- Zhaoyang Yao 1 ,
- Dong-Jun Kim 1 ,
- Kaiming Cai 1 ,
- Jerald Yoo ORCID: orcid.org/0000-0002-3150-1727 1 ,
- Xuanyao Fong ORCID: orcid.org/0000-0001-5939-7389 1 &
- Hyunsoo Yang ORCID: orcid.org/0000-0003-0907-2898 1
Nature Communications volume 15 , Article number: 3457 ( 2024 ) Cite this article
1297 Accesses
3 Altmetric
Metrics details
- Electrical and electronic engineering
- Magnetic devices
The growth of artificial intelligence leads to a computational burden in solving non-deterministic polynomial-time (NP)-hard problems. The Ising computer, which aims to solve NP-hard problems faces challenges such as high power consumption and limited scalability. Here, we experimentally present an Ising annealing computer based on 80 superparamagnetic tunnel junctions (SMTJs) with all-to-all connections, which solves a 70-city traveling salesman problem (TSP, 4761-node Ising problem). By taking advantage of the intrinsic randomness of SMTJs, implementing global annealing scheme, and using efficient algorithm, our SMTJ-based Ising annealer outperforms other Ising schemes in terms of power consumption and energy efficiency. Additionally, our approach provides a promising way to solve complex problems with limited hardware resources. Moreover, we propose a cross-bar array architecture for scalable integration using conventional magnetic random-access memories. Our results demonstrate that the SMTJ-based Ising computer with high energy efficiency, speed, and scalability is a strong candidate for future unconventional computing schemes.
Similar content being viewed by others
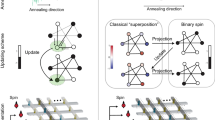
Efficient combinatorial optimization by quantum-inspired parallel annealing in analogue memristor crossbar
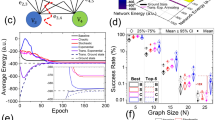
Combinatorial optimization by weight annealing in memristive hopfield networks
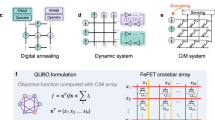
Ferroelectric compute-in-memory annealer for combinatorial optimization problems
Introduction.
The demands for future data-intensive and energy-efficient computing tasks overwhelm the computational power of conventional von Neumann architectures 1 . For example, NP-hard problems are often encountered in combinatorial optimizations 2 , resource allocation 3 , cryptography 4 , finance 5 , image processing 6 , tour planning 7 , and job sequencing 8 , and their computational time and hardware resources increase exponentially with the problem size, which makes them very difficult or impossible to be solved by conventional computers in a finite time. These problems can be mapped to the Ising model, a mathematical model to characterize interactions between magnetic spins 9 . The dynamics of the model is algorithm- based, i.e. by constructing a proper coupling matrix and allowing the system to evolve utilizing an intrinsic convergence property of the Ising model, the ground state could be obtained as a solution to the corresponding problems. However, as the system might be trapped in many local minima, the annealing process has usually been adopted in Ising computers to address such limitations. It is commonly agreed that adding fluctuations prevents the Ising computer from being stuck at the local minima.
Efficient algorithms and hardware systems for finding an optimal or near-optimal solution of an Ising model at a fast speed and low power have been sought. Adiabatic quantum computing (AQC) 10 , 11 and quantum computing 12 , 13 , 14 , 15 based on superconducting qubits are capable of converging the Ising model by tunneling out of local minima to the global minima. A 100-node Maxcut problem was solved using a quantum computer of 2048 spins with huge power consumption 16 . Besides the high cost and complexity of cryogenic temperature, this proof-of-concept system was limited by the sparse connections only between the nearest neighbors, which leads to sub-optimal outcomes 17 . Simulated annealing based on CMOS implementations was exploited for parallel Ising computing, including central processing units (CPU) 18 , 19 , graphics processing units (GPU) 20 , and field-programmable gate array (FPGA) 21 , 22 . These hardware have reported as large as 16,384 spins, however, it requires huge hardware resources for generating random numbers to introduce stochasticity to escape from the local minima 4 , 18 , 23 , 24 . Coherent Ising machine (CIM) is an optical scheme with competitive energy efficiency. However, it requires a long fiber ring cavity and relies on external FPGA for implementing coupling 25 , 26 . The temporal multiplexing process is also time-consuming and hard to expand to large systems. Recently, experiments and simulation works have investigated various devices to emulate the behavior of Ising spins by taking advantage of their intrinsic physics. An 8-spin asynchronous probabilistic computer based on superparamagnetic tunnel junctions for solving integer factorization tasks of values up to 945 was demonstrated 4 . SPICE simulations of 16-city TSP using simulated annealing method were presented 27 . Other works such as 8-spin phase-transition nano-oscillators 28 , multiferroic oxide devices with a high thermal stability 29 , and magnetoresistive random access memory (MRAM) 30 , 31 have also conceptually proved that spin-based devices are suitable for representing Ising units. However, these works have encountered challenges in either partially-connected Ising spins or small scalability which limit the Ising computer from solving practical problems.
TSP discussed in this paper is a well-known problem which is much beyond the limitation of locally connected Ising models. Other combinatorial optimization problems, such as knapsack problems, coloring problems, and number partitioning, need all-to-all connection to satisfy specific constraints 9 . In practice, an additional graph embedding process is often required when mapping to 2-dimensional CMOS circuitry which only considered the coupling between adjacent spins 32 , 33 , 34 . Since the embedding increases the required number of auxiliary spins and causes spin connections to change, the annealing accuracy is degraded significantly, especially when the problem size is large. This means that supporting a fully connected Ising model is highly recommended for dealing with a wide range of problems. Another problem is the rapidly increasing connectivity when considering large-scale systems, which usually results in huge energy consumption and latency. Since the number of spins that a particular annealing processor can handle limit the scale of the problem that can be solved, how to solve complex problems with limited hardware in an energy-efficient way has also drawn significant attention.
In this work, we experimentally report a scalable Ising computer based on 80 SMTJs with all-to-all connections and successfully solve the 4761-node TSP problem. The intrinsic stochasticity in SMTJ enables ultra-fast and low-power Ising annealing without using extra resources for random number generation and Metropolis determining process 7 . By combining global annealing with intrinsic annealing in SMTJ, the convergence of the Ising problem is guaranteed especially in large-scale Ising problems. The method to determine parameters of global annealing is discussed. With an all-to-all connection among Ising spins, the combinatorial optimization of 9-city TSP is solved with the optimal solution. We further develop the algorithm for constrained TSP (CTSP) with no extra auxiliary Ising bits both in algorithm and hardware, indicating the superiority and flexibility of this Ising computer. Furthermore, we propose an optimization strategy based on graph partitioning (GP) and CTSP and experimentally solved a 70-city TSP, which typically needs 4761 nodes, on our 80-node Ising computer with a near-optimal solution. The system can obtain the lowest power consumption of 0.64 mW as well as high energy efficiency of 39 solutions per second per watt among state-of-art Ising annealers. We have experimentally demonstrated that large-scale Ising problems can be solved by small-scale hardware in an energy-efficient way.
SMTJ-based artificial Ising spin
Various NP-hard problems can be solved by constructing corresponding Ising models and observing the ground states during evolution processes. Figure 1a shows an all-to-all connected Ising model, whose Ising Hamiltonian can be written as
where \(H\) is the total energy of the system, \(N\) is the total number of spins, \({s}_{i}\) is the \(\,i\) -th spin with one of two states; “+1” (Ising spin up) or “−1” (Ising spin down), \({J}_{i,j}\) is the coefficient of coupling between the \(i\) -th and the \(j\) -th spins, and \({h}_{i}\) is the external field of the \(\,i\) -th spin. For a fixed configuration of other spins than \({s}_{k}\) , the probability of \({s}_{k}\) staying in the down-state is given by
where \(\Lambda=\frac{\partial H}{\partial {s}_{k}}\) (see Supplementary Note 1 ).
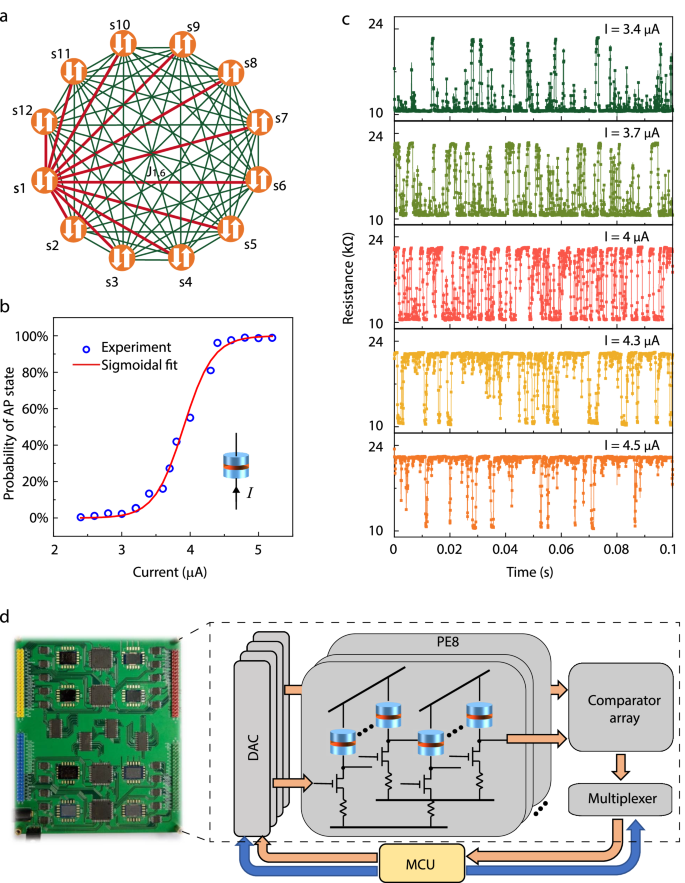
a All-to-all connected 12-spin Ising model with s represents the spin and J 1,6 represents the coupling between s1 and s6. b Sigmoidal fit of probability of AP state ( \({p}_{{AP}}\) ) of an SMTJ under different input currents ( I ). \({p}_{{{{{{\rm{AP}}}}}}}=\frac{1}{1+{e}^{-4.672\times (I-3.905{{{{{\rm{\mu }}}}}}{{{{{\rm{A}}}}}})}}\) . Inset: diagram of an SMTJ. A tunneling barrier layer is sandwiched by a reference layer and a free layer. c Time-dependent resistance of an SMTJ under different input currents ( I ). d Photograph and schematic diagram of SMTJ-based Ising computer. The system contains 8 processing elements (PEs), 4 digital-to-analog converters (DACs), a comparator array, a multiplexer and a microcontroller unit (MCU). Each PE has 10 SMTJ computing units. Each computing unit includes a transistor and a resistor to adjust the property into stochastic. Blue lines and orange arrows represent the control and data flow, respectively.
One natural implementation of this Ising spin is based on a stochastic nanomagnet. The inset of Fig. 1b shows the sketch of an SMTJ, consisting of a tunneling barrier sandwiched by a reference layer and a free layer (see Methods section). Because of the small device diameter (~50 nm), the energy barrier of the free layer between the anti-parallel (AP) and parallel (P) states is low that the retention time of either state is in the range of μs to ms, similar to previous studies 4 , 35 . The SMTJ resistance, measured as a function of time in Fig. 1c , shows preferred AP states at high currents and P states at low currents. When the current ( I ) is ~4 μA, SMTJ shows an equal chance of AP and P states. The probability of the AP state under different input currents over 0.1 s is fitted in Fig. 1b by a sigmoid function:
where \({{{{{\rm{a}}}}}}=4.67 \, {{{{{\rm{and\; b}}}}}}=3.9 \, {{{{{\rm{\mu }}}}}}{{{{{\rm{A}}}}}}.\) In order to emulate Ising spin \({s}_{k}\) with our SMTJ device, we only need to make the probability of the down-state of \({s}_{k}\) to be equal to that for the AP state of SMTJ, namely \({p}{\_}{{{{{{\rm{\_}}}}}}{{{{{\rm{AP}}}}}}}={p}{\_}{{{{{{\rm{\_}}}}}}\downarrow }\) , with two calibration coefficients. Thus, we can derive the form of the current \({{I\_}}_{k}\) injected to SMTJ as (see Supplementary Note 1 ):
where \(c=1/{kT}\) is the effective inverse temperature which can be conducted for global annealing.
Intrinsic annealing in SMTJs-based Ising computer
By integrating 80 SMTJs with a peripheral circuit and a microcontroller unit (MCU), we build an 80-node Ising computer (see Supplementary Note 2 ). Each Ising spin in Eq. ( 1 ) is emulated by an SMTJ with intrinsic randomness, where P (AP) state represents spin-up (down). Figure 1d shows the photograph of the printed circuit board (PCB) and the diagram of the system (see Methods section). The system contains 8 processing elements (PEs); each PE has 10 SMTJ computing units. Each SMTJ computing unit includes a transistor and a resistor to adjust the state of SMTJ into stochastic. During the computing process, an MCU examines the states of all SMTJs by reading the output of comparator arrays through multiplexers and generates new input voltages for digital-to-analog converters (DACs) according to the updating rule in Eq. ( 4 ) (see Supplementary Note 3 for calibration of 80 SMTJ computing units).
During the evolution process, an Ising solver could be easily trapped in a local minimum state. To avoid this non-optimal solution, annealing algorithms such as simulated annealing (SA) or quantum annealing (QA) were developed. The general idea of SA is to make the system evolve from a high temperature to a low temperature gradually 7 . The convergence and relaxation of SA can be mathematically provable 36 . During each iteration, a random number is generated for stochasticity and introduced to determine whether the result in this iteration should be accepted or not. In QA, quantum fluctuations cause quantum tunneling between states 17 . In both SA and QA, stochasticity needs to be introduced into the annealing process. In contrast, our Ising system utilizes the intrinsic stochastic behaviors of SMTJ to perform the Metropolis process of standard SA in hardware, which greatly saves the solution time and hardware resources for generating randomness (see Supplementary Note 4 ). Besides, our Ising computer has an all-to-all connection which has wider application scenarios, as well as a better capability of escaping from local minima.
Ising mapping of N-city TSP and CTSP
We have applied our Ising computer to the TSP problem, one of the combinatorial optimization problems, which applies to various sectors, such as vehicle routing, logistics, planning, and scheduling. The goal is to find the shortest route that visits all listed cities once and only once given distances between the cities in the list. In order to solve this problem, we first map N -city-TSP to an \({N}^{2}\) -spin Ising model, or \({(N-1)}^{2}\) -spin model assuming a fixed starting city. Figure 2a shows the coordinates of 9 cities and Fig. 2b shows the 81-spin Ising model, whose rows indicate the cities and columns indicate the visiting order. We define the binary spin, s , as \({s}_{i,j}\) = 1 if city i is visited as j -th city or \({s}_{i,j}\) = −1 otherwise. The total Hamiltonian of TSP is expressed by 9
where the first term is a constraint that represents only one city is visited at the j -th visit, and the second term represents one city is visited only one time. \(w\) is a constant small enough ( \(0 \, < \, w \, < \, 1\) ) not to violate the two constraints of the TSP cycle. \({d}_{i,{i{{\hbox{'}}}}}\) is the distance between city \(i\) and city \({i{{\hbox{'}}}}\) . According to Eqs. ( 1 ) and ( 5 ), coupling matrix \(J\) of 81 spins could be obtained, as shown in Fig. 2c (see Supplementary Note 5 ). It shows that spins in the same row or column have strong coupling, as indicated by the first two terms in Eq. ( 5 ).
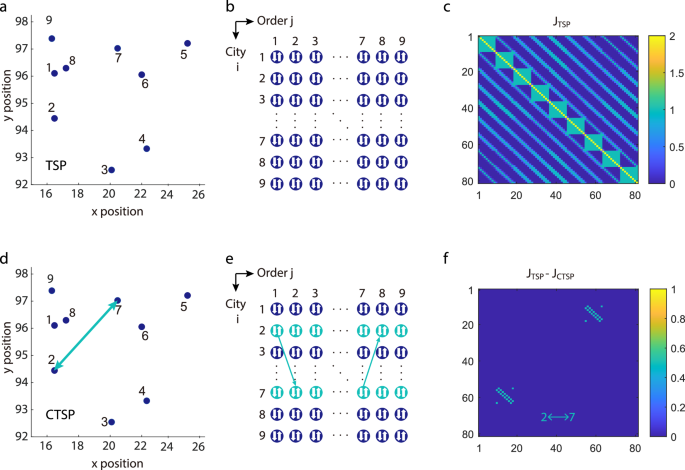
a Coordinates of all 9 cities used in this problem which are the first 9 cities in the dataset Burma14 from TSPLIB. b Ising spin representation for 9-city TSP (81 spins). Rows indicate names of cities and columns indicate the visiting order. Each spin can be 1 (visited) or −1 (not visited) in each iteration. c Color map of the coupling matrix J TSP of 9-city TSP, and the color bar represents an effective energy with the unit of kT . Here, k is the Boltzmann constant and T is the temperature. d Constrained TSP (CTSP) with a fixed vising sequence from city 2 to city 7 or from city 7 to city 2. The arrows represent the visiting sequence. e The Ising spin representation for CTSP with the fixed visiting sequence in d . Arrows represent possible vising sequences. f Color map of the difference of coupling matrix between TSP (J TSP ) in a and CTSP (J CTSP ) in d . Arrows represent the fixed vising sequences from city 2 to city 7 or from city 7 to 2.
We define CTSP as the visiting orders of some cities are enforced during the traveling. This is quite useful in real-life scenarios. For example, a delivery man collects food and drinks at shop A and must deliver hot drinks to B first even though the total cost is higher than optimal. We propose an algorithm for solving CTSP by adding negative “distance” to the Hamiltonian. For example, suppose that city A and city B are required to be connected in the CTSP as city 2 and city 7 shown in Fig. 2d , and then we add the term
such that the energy of a path, where city A and city B are connected, is always lowered by \(\theta .\) When \(\theta\) is sufficiently large, the optimal path must have city 2 and city 7 connected. Thus, the total Hamiltonian of the CTSP is expressed by
Constructing an Ising model for CTSP is exactly the same as TSP except for extra allowed visiting sequences, as shown in Fig. 2e . This would lead to a modification of the coupling matrix of \(J\) according to Eq. ( 7 ) (see the deduction of \({J}_{{CTSP}}\) in Supplementary Note 6 ). From Fig. 2f we can clearly see the differences between \({J}_{{CTSP}}\) and \({J}_{{TSP}}\) . This algorithm of CTSP fits for arbitrary constraints of visiting sequences as well as their combinations.
Experimental demonstration of 9-city TSP
We first run a 9-city TSP in the 80 SMTJ-based Ising computer at a relatively low but non-zero effective temperature to examine the intrinsic annealing in SMTJ. The iteration time is set comparable to the longest retention time of SMTJs to avoid reading previous spin states. In our experiments, we set the iteration time as 0.1 ms. As shown in Fig. 3a , as the effective inverse temperature ( c ) is increased quickly to 0.5, the system converges rapidly to a low energy state within 50 iterations and reaches the ground state after 4000 iterations. It should be noted that the intrinsic stochasticity in SMTJs helps the system escape from local minima without an extra annealing process, as shown in the right inset of Fig. 3a . Figure 3b illustrates the evolution of 9 spins out of 81 spins. The evolution of all 81 spins can be found in Supplementary Note 7 .
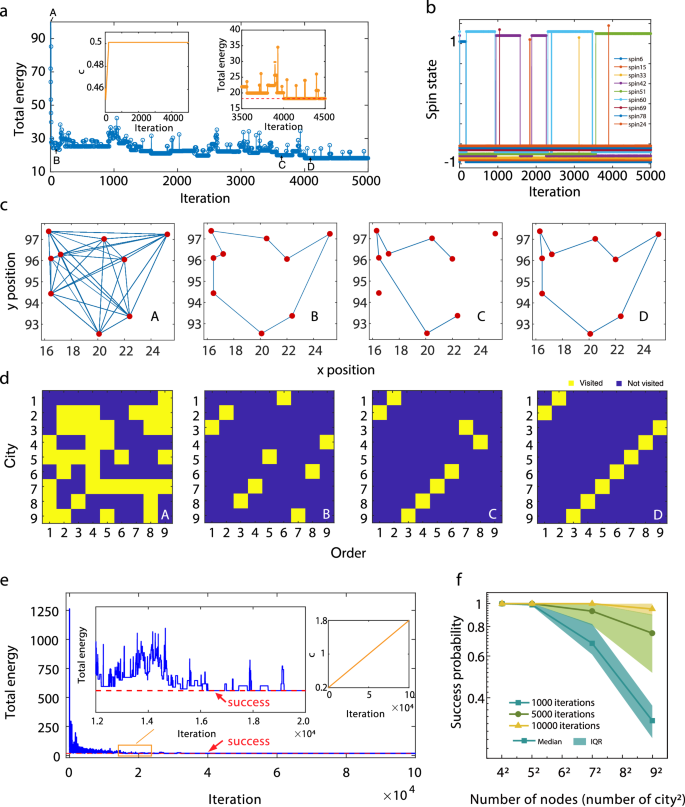
a Total energy transition of 9-city TSP with 5000 iterations (the optimal solution with the energy of 18.23 corresponds to the dashed horizontal line). Insets: effective inverse temperature ( c ) and total energy within 3500–4500 iterations. b Evolution of 9 representative SMTJ states in 5000 iterations. An offset is used in the y -axis to show each SMTJ clearly. c Visiting routes of state A, B, C, and D in a . d Corresponding Ising spins of state A, B, C, and D in a . The yellow squares represent ‘visited ( \({s}_{i,j}=1\) )’ and the purple squares represent ‘not visited ( \({s}_{i,j}=-1\) )’. e Total energy transition with increasing c from 0.2 to 1.8. Left inset: zoom-in view of total energy transition with increasing c from 0.392 to 0.52. Right inset: transition of c with iterations. The red dashed line represents the optimal path (success). f Success probability of solving TSP with varying the node size. The data points and shadows represent the median value and the interquartile range (IQR), respectively.
We choose four states in Fig. 3a to inspect the traveling path in Fig. 3c and their Ising spins, namely \({s}_{i,j}\) , as shown in Fig. 3d . The yellow square in Fig. 3d represents \({s}_{i,j}=1\) (visited) and the blue square represents \({s}_{i,j}=-1\) (not visited). In an initial state A, the spin states are randomly set and then converge to a relatively low energy at state B. State C is an intermediate solution during the annealing process. State D is the optimal solution satisfying two constraints of the TSP. Because we anneal the system to a relatively low but non-zero temperature so that the convergence to a sub-optimal state could be guaranteed, and at the same time, the intrinsic randomness in SMTJ helps the system to escape from local minima and find a ground state quickly. We test 10 different random initial states each with 5000 iterations and find that in all cases the system can obtain a relatively small energy, as shown in Supplementary Note 8 . However, there is a probability that the system jumps out of the ground state because of the non-zero temperature. If we continue to observe the evolution in a large timescale, the system would move back to the global minimum state. In some cases, where the speed and near-optimal solution matter but the accurate optimal solution is not, the number of iterations can be chosen to be small.
Further global annealing of the system to a lower effective temperature may guarantee the convergence of the computation. Here we use linear annealing as an example to examine the convergence of this algorithm in a very large-iteration limit. The initial temperature should be chosen sufficiently high to ensure that the thermal energy exceeds any energy barrier ( \(\Delta H{=H}_{\max }-{H}_{\min }\) ) within the system, while still adhering to the fundamental constraints of the specific Ising model. For a given N-city TSP, \({H}_{\max }\) in Eq. ( 5 ) can be estimated as \(w\times N\times \bar{d}\) , assuming that the distance between any two cities is the same as the average distance \(\bar{d}\) . Similarly, \({H}_{\min }\) can be estimated as \(w\times N\times {d}_{\min }\) . Therefore, the initial \(c\) of 9-city TSP in our experiment can be estimated as \({c}_{{{{{{\rm{initial}}}}}}}\, \sim 1/\Delta H=0.07\) , where \(w=0.5\) , \(N=9\) for a total of 9 cities, \(\bar{d}=4\) and \({d}_{\min }=0.8\) for the average and shortest distance of each two cities, respectively in Fig. 3c . We then choose \({c}_{{{{{{\rm{initial}}}}}}}\) = 0.2 which is sufficiently safe for annealing. As the temperature linearly decreases, the dynamical system gradually stabilizes. The final temperature should be low enough i.e., \({c}_{{{{{{\rm{final}}}}}}} \, \gg \, 1/\Delta H\) , to freeze all possible fluctuations. Here we set \({c}_{{{{{{\rm{final}}}}}}}=1.8\) which is at least one order larger than \(1/\Delta H\) . This can also be verified by observing randomly generated states under \({c}_{{{{{{\rm{final}}}}}}}\) for long iterations. Regarding the annealing speed, if several changes in the spin configuration are observed under each value of c , then this annealing speed is valid. Plenty trials are required to find the proper annealing speed (details in Supplementary Note 8 ).
In Fig. 3e we can find the first global minimum energy appears after 16,500 iterations, and converge to the ground state after 40,000 iterations. Temperature schedules can be optimized to reduce iteration numbers, e.g. increase the effective temperature in the first few time steps, and then decrease gradually, or learned by the reinforcement learning method 37 . In practice, we use one memory to store the minimum energy state during the computation, and another memory to record the final energy state. We take the minimum value of these two results as the solution. Figure 3f shows the success probability (defined as finding the optimal path) of TSP with various node sizes. The success probability of 9-city TSP reaches 95% after 10 4 iterations. The success probability with the parameter \(w\) in Eq. ( 5 ) which determines the relative strength of the constrain term and distance term is also discussed. If the \(w\) is too large, then the probabilities of violations, namely the invalid path, would increase, as shown in Supplementary Note 8 . If \(w\) is too small, then the effect of the distance term is small, which results in a slower convergence to the ground state.
The advantages of this annealer are threefold: (1) Selective working modes by using different temperature schemes. One is the probabilistic sampling mode working at a constant temperature, which is similar to an asynchronous probabilistic computer 4 ; the other is the annealing mode conducted by reducing the effective temperature. (2) Fast speed and low power consumption to find the ground state because of the intrinsic annealing properties in SMTJ. (3) Global annealing outperforms probabilistic sampling in achieving efficient convergence, especially for large-scale problems.
We have implemented a synchronous design with a lower requirement on the speed of peripheral circuits. This design also effectively mitigates issues such as leakage, sneak currents, and parasitic resistances which might encountered in asynchronous hardware with a memristive (or resistive) crossbar array.
Compressing 70-city TSP to 80-node Ising computer
Generally, the number of spins required for an N -city TSP is ( N -1) 2 , which limits the scalability of TSP on state-of-the-art computing systems. Here, we propose a graph Ising compressing algorithm based on CTSP that can significantly reduce the number of spins and interactions for solving a TSP. Figure 4a is an example of how we apply this algorithm to our 80-node SMTJ Ising computer for solving a 70-city TSP (4761 nodes, st70 data set from TSPLIB 38 ). The major steps of this algorithm can be described as follows: (a) divide the cities into several smaller groups until the number of cities in each group is less than 10 by GP method; (b) solve TSP within each group separately; (c) integrate neighboring groups to obtain an initial path of the whole group; and (d) optimize the path in (c) by a CTSP window sliding over the whole map.
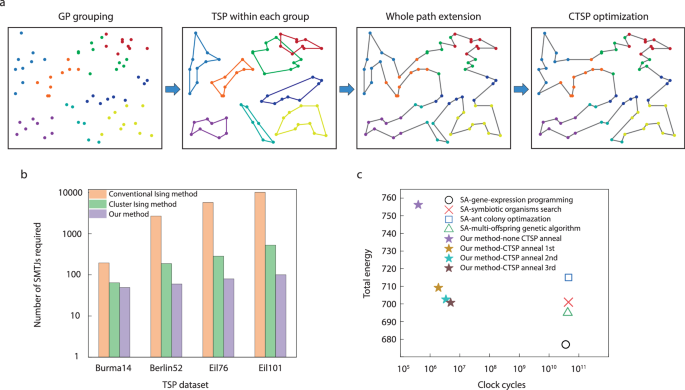
a Optimization algorithm for 70-city TSP. b Number of required SMTJs for various problems using different methods. Burma14, berlin52, eil76, and eil101 are TSP of 14, 52, 76, and 101 cities, respectively. c Comparison of total Ising energy (path) and total clock cycles for final solution with different SA-based algorithms, including symbiotic organisms search 40 , ant colony optimazation 41 , multi-offspring genetic algorithm 42 , and gene-expression programming 7 . Our method is tested on our Ising system and others are tested on Intel Core-i7 PC. In this comparison, our system runs at a main frequency of 10 kHz.
It is worth mentioning that GP is also an Ising problem. When converting a global TSP into local TSPs, using GP would be more hardware-friendly for our Ising computer compared to other clustering algorithms. It is based on the idea that the original graph can be separated into multiple sub-graphs depending on the Euclidean distance. The number of spins required for solving GP is ~ N and thus, GP is quite efficient for local TSPs since the problem size can be reduced to ~ \({\left(N-1\right)}^{2}/a\) , where \(a\) is the number of groups, and each TSP can be optimized independently (see GP mapping in Supplementary Note 9 ).
The final step (d) is based on CTSP, where a rectangular window slides over the path and cuts it into several disconnected lines, among which the two longest lines are chosen and the edge cities are connected as a circular path (Supplementary Note 10 ). The CTSP is solved within each window for sub-area optimization without changing the visiting order of edge cities. After this, the two lines at the edge cities are opened and CTSP is carried out again after sliding to the next window. GP-CTSP-based optimization algorithm provides an efficient way of finding near-optimal solutions for large-scale TSP on limited hardware resources.
Figure 4b shows the comparison of numbers of spins for different TSPs by a conventional Ising method 9 , cluster Ising method 39 , and our method. The required number of spins in our method is relatively unchanged for various TSPs, while that of other methods increases substantially with the scale of the problem. Figure 4c shows the total path of 70-city TSP as a function of iteration number using different SA-based algorithms, including symbiotic organisms search 40 , ant colony optimization 41 , multi-offspring genetic algorithm 42 , and gene-expression programming 7 . Finally, we obtain the near-optimal path with a total energy of 700.71, which is slightly higher than the optimal solution of 675. However, the iteration number for an optimized solution is 4.9 \(\times\) 10 6 by our method, which is two to three orders lower than that of SA-based algorithms running on Intel Core-i7 CPU 7 with the main frequency of 3 GHz, as shown in Fig. 4c .
Ising computer scaling and cross-bar architecture
The above experimental demonstration shows our Ising computer with 80 SMTJs is capable of finding a near-optimal solution to a medium-scale NP-hard problem. We then explore the performance with increasing from 70 to 200 cities. The simulation of complete TSP task is carried out using MATLAB, incorporating a stochastic model of the SMTJ employed in our experiment (details in Supplementary Note 11 ). The solution quality is defined as
Figure 5a illustrates the solution quality of the best results obtained for each TSP task (Supplementary Note 12 for the best solutions). Notably, as the number of SMTJ (M) increases, higher quality solutions can be attained. It is worth emphasizing that the shortest path obtained for the 101-city TSP is 640.9755 in our study, surpassing the optimal path of 642.3095 provided by TSPLIB (Eil101.opt.tour). This outcome serves as evidence of the superiority of our method. The utilization of more SMTJs solving TSP per sliding window leads to improved optimization of CTSP annealing, resulting in an enhanced solution quality, as depicted in Fig. 5b . Consequently, the time to convergence s would also increase with the use of more SMTJS. When dealing with a fixed hardware capacity, an appropriate number of SMTJs for CTSP optimization can be assigned, taking into account both the solution quality and convergence speed. Figure 5c showcases the success rate (defined as achieving 95% solution quality) as the problem size increases. The success probability of 200-city TSP, whose complexity is ~40,000 nodes, can reach as high as 90%, demonstrating the scalability of our method compared to typical TSP (without GP and CTSP) 9 .
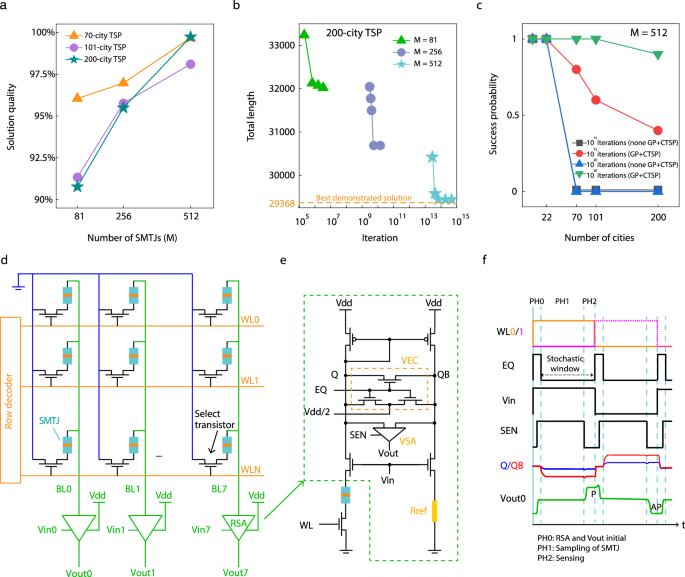
a Solution quality of various problems using different number of SMTJs (M) in the array. The datasets used are St70, Eil101 and KroA200, for 70, 101 and 200 cities, respectively. b Total length of KroA200 TSP at different convergence speeds using different number of SMTJs. The dashed line represents the best demonstrated solution. c Success probability of different TSP algorithm (without/with GP and CTSP) as the number of cities increases after running for 50 times. A total of 512 SMTJs are used. Here we define the success as achieving the solution quality of 95%. d SMTJ cross-bar array which contains row decoder, SMTJ, select transistor and read sense amplifier (RSA). BL represents bit line, WL represents word line, Vin, Vout and Vdd represent the input voltage, output voltage and supply voltage of RSA. e Circuit of one RSA which contains a current mirror, voltage equalization circuit (VEC, with a control signal of EQ which initializes the voltages in Q and QB points, under a reference voltage of Vdd/2), voltage sense amplifier (VSA, with a control signal of SEN), reference resistance ( \({{{{{\rm{Rref}}}}}}=\frac{1}{2}({{{{{\rm{Rap}}}}}}+{{{{{\rm{Rp}}}}}})\) , Rap and Rp represent SMTJ’s resistance in AP and P state respectively), and control transistors. f Signals of writing/reading two adjacent SMTJ cells in one BL, selected by WL0 and WL1 in sequence. All signals are defined in e and f .
We also propose a cross-bar architecture for large-scale Ising computer implementation, which can be integrated by using modern MRAM and CMOS technologies. The core part of this architecture consists of SMTJ bit cells organized as a cross-bar array, integrated with row decoders and read sense amplifiers (RSA), as shown in Fig. 5d . Each SMTJ bit cell contains one select transistor and one SMTJ (1T1SMTJ), whereas the gate of the select transistor is driven by word lines (WL), and the source of all bit cells are connected to the ground. Each bit line is assigned with an RSA. The current flows through SMTJ can be continuously adjusted by Vin of RSA, and the state of SMTJ can be read by RSA at the same time. Figure 5e illustrated the circuit of RSA, in which two clamp transistors control the current flow through the bit cell path and reference path by the gate voltage (Vin), and a current mirror is used to guarantee the same current of the above two paths. Then different voltages would show in the Q and QB point when the resistance of SMTJ is higher or lower than the reference resistor (Rref). By utilizing an enabled voltage sense amplifier (VSA), the voltages at the Q and QB points are sensed, allowing the SMTJ state to be determined as either Vdd (P state) or 0 V (AP state). Particularly, a voltage equalization circuit (VEC) is designed for initializing VSA to avoid incorrect readout. Electrical coupling through a resistance change 43 is evaluated to have neglectable effects (details in Supplementary Note 11 ). Figure 5f shows the signals to control and read bit cells. In phase 0 (PH0), one row of SMTJs is selected by WL, and Vin prepared by peripheral circuit is applied to the corresponding RSA. EQ is set high to initialize Q, QB and Vout as Vdd/2. In phase 1 (PH1), the SMTJ fluctuates from the falling edge to next rising edge of EQ. Finally, in phase 2 (PH2), RSAs read the data of one row in parallel at the falling edge of SEN. After the first row has been retrieved, the partial sum starts to be computed. Meanwhile, the same process for the second row can be started, so and so forth. To avoid reading the previous state, the duration of PH1 is preferred to be comparable with the retention time of SMTJ, which limits the main frequency of the system (see details in Supplementary Note 11 ).
We compare our system with other state-of-art Ising solvers, including CMOS annealer (Intel Core i7 processor) 7 , quantum annealer (D-Wave 2000Q) 16 , 17 , CIM with FPGA 26 , memristor Hopfield neural networks (mem-HNN) 44 , and phase-transition nano-oscillators (PTNO) 28 in solving 4761-node TSP70, as shown in Table 1 . We use the experimental data for benchmarking from literature, and two kinds of SMTJs for comparison. One is our perpendicular anisotropy SMTJ device and the other is assuming recently reported in-plane anisotropy SMTJ with a retention time of 8 ns 45 , 46 . The major attributes are the main frequency (defined as 1/iteration time), power, time-to-solution as well as energy efficiency (defined as solutions per second per watt). As quantum computers, CIM, mem-HNN, and PTNO only demonstrated ~100-node max-cut problems, we estimate the time-to-solution for solving TSP70 by assuming that the algorithm and the total number of spins to find a near-optimal solution is the same as our work (details in Supplementary Note 13 ). Here, we set 80-spin Ising computer as a standard and fix the number of iterations of 400,000 for a good solution to TSP70. Only Ising computing parts are calculated for power consumption.
In Table 1 , although the main frequency of CPU is the highest among all candidates, the energy efficiency is lower than our SMTJ-based approach. This is due to the redundant logic and data transfer delay between the memory and PEs in a conventional von-Neumann architecture. The SMTJ-based approach currently outperforms the quantum annealer both in the power consumption as well as time to solution. The power of quantum annealer is huge which needs to be optimized further for real applications. CIM is another promising architecture with a fast speed and acceptable power consumption. Current CIM systems are proof-of-concept systems which are not at present optimized for energy efficiency. Mem-HNN has a relatively fast speed assuming the 180-nm CMOS technology. However, the required number of devices is large, which limits the integrated density. The PTNO approach uses capacitors or resistors to mimic spin coupling, whose main frequency would be limited by the system scale and parasitic effects. It is reported that the ideal main frequency would decrease from 500 to 87 MHz when the system scale increases from 8-node to 100-node 28 . Our SMTJ-based Ising computer outperforms other approaches with low power consumption with 0.64 mW (details in Supplementary Note 13 ).
We experimentally demonstrate perpendicular MTJs with a retention time of ~0.1 ms and solve TSP70 Ising problems at an energy efficiency of 39 solutions per second per watt. Furthermore, we simulate an Ising computer with 4 Kb SMTJs using 40 nm commercial CMOS technology. The simulated energy efficiency for solving TSP70 by using the same SMTJ can reach 68 solutions per second per watt. By using reported in-plane SMTJ 45 and advanced CMOS, the system could obtain the highest energy efficiency of \(5.4\times {10}^{3}\) , which shows several orders of magnitude improvement over other approaches. This result suggests that an SMTJ-based Ising computer can be a good candidate for solving dense Ising problems in a highly energy-efficient and fast way.
In summary, we have experimentally demonstrated an intrinsic all-to-all Ising computer based on 80 SMTJs, and solved 9-city TSP with the optimal solution. Furthermore, a compressing strategy based on CTSP and GP is proposed to experimentally solve 4761-node 70-city TSP on an 80-node system with a near-optimum solution as well as ultra-low energy consumption. A cross-bar architecture is then proposed for large-scale Ising computers and the 200 city TSP task is simulated. Our system provides a feasible solution to fast, energy-efficient, and scalable Ising computing schemes to solve NP-hard problems.
Sample growth and device fabrication
Thin film samples of substrate/[W (3)/Ru (10)] 2 /W (3)/Pt (3)/Co (0.25)/Pt (0.2)/[Co (0.25)/Pt (0.5)] 5 /Co (0.6)/Ru (0.85)/Co (0.6)/Pt (0.2)/Co (0.3)/Pt (0.2)/Co (0.5)/W (0.3)/CoFeB (0.9)/MgO (1.1)/CoFeB (1.5)/Ta (3)/Ru (7)/Ta (5) were deposited via DC (metallic layers) and RF magnetron (MgO layer) sputtering on the Si substrates with thermal oxide of 300 nm with a base pressure of less than \(2\times {10}^{-8}\) Torr at room temperature. The numbers in parentheses are thicknesses in nanometers. To fabricate the superparamagnetic tunnel junctions, bottom electrode structures with a width of 10 µm were firstly patterned via photolithography and Ar ion milling. MTJ pillar structures with a diameter of ~50 nm for the superparamagnetic behavior were patterned by using e-beam lithography. The encapsulation layer of Si 3 N 4 was in-situ deposited after ion milling without breaking vacuum by using RF magnetron sputtering, and top electrode structures with a width of 10 µm were patterned via photolithography and top electrodes of Ta (5 nm)/Cu (40 nm) were deposited by using DC magnetron sputtering.
MTJ characterization by probe station
The setup includes a source meter (Keithley 2400) for supplying DC bias currents and a data acquisition card (NI-DAQmx USB-6363) for the read operation. A single SMTJ operation cycle comprises two steps (i.e. bias and read). A small DC input current with an amplitude of 1–20 μA is applied to SMTJ. Simultaneously, the DAQ card reads the voltage signal across the SMTJ at a maximum sampling rate of 2 MHz. The MTJ switching probability varies in accordance with the amplitude of applied currents. The retention time of MTJ is determined from random telegraph noise measurements over 250 ms. The expectation values of event time τ is determined by fitting an exponential function to the experimental results.
80 SMTJ arrays and peripheral circuits are integrated on a 12 cm × 15 cm PCB, controlled by an MCU (Arduino Mega 2560 Rev3). Four 12-bit rail-to-rail DACs (AD5381) with 160 output channels in total are used to generate analog DC inputs for PE and comparator arrays. Half of the DAC output channels are used to provide stimulation to the gate terminal of NMOSs (2N7002DW-G), and others are used to provide reference voltages to comparators (AD8694). The drain voltages of NMOS are compared with reference voltages and generate outputs in parallel. Outputs of comparator arrays are read by MCU through four multiplexers (FST16233) and then are calculated to obtain new inputs for DACs. The supply voltage of the PCB board and SMTJs is 5 V and 0.8 V, respectively. The value of resistors in each computing unit can be designed to adjust the center of sigmoidal curves.
Data availability
The data generated during this study are available within the article and the Supplementary Information file. Source data are provided with this paper.
Code availability
The codes that support this study can be available from the corresponding author upon request.
Theis, T. N. & Wong, H. S. P. The End of Moore’s Law: A New Beginning for Information Technology. Comput. Sci. Eng. 19 , 41–50 (2017).
Article Google Scholar
Shim, Y., Jaiswal, A. & Roy, K. Ising computation based combinatorial optimization using spin-Hall effect (SHE) induced stochastic magnetization reversal. J. Appl. Phys. 121 , 193902 (2017).
Article ADS Google Scholar
Tindell, K. W., Burns, A. & Wellings, A. J. Allocating hard real-time tasks: An NP-Hard problem made easy. J. Real.-Time Syst. 4 , 145–165 (1992).
Borders, W. A. et al. Integer factorization using stochastic magnetic tunnel junctions. Nature 573 , 390–393 (2019).
Article ADS CAS PubMed Google Scholar
Tatsumura, K., Hidaka, R., Yamasaki, M., Sakai, Y. & Goto, H. A Currency Arbitrage Machine Based on the Simulated Bifurcation Algorithm for Ultrafast Detection of Optimal Opportunity. in 2020 IEEE International Symposium on Circuits and Systems (ISCAS) 1–5 (IEEE, 2020). https://doi.org/10.1109/ISCAS45731.2020.9181114 .
Cohen, E., Carmi, M., Heiman, R., Hadar, O. & Cohen, A. Image restoration via ising theory and automatic noise estimation. In 2013 IEEE International Symposium on Broadband Multimedia Systems and Broadcasting (BMSB) 1–5 (IEEE, 2013). https://doi.org/10.1109/BMSB.2013.6621708 .
Zhou, A.-H. et al. Traveling-Salesman-Problem Algorithm Based on Simulated Annealing and Gene-Expression Programming. Information 10 , 7 (2018).
Garza-Santisteban, F. et al. A Simulated Annealing Hyper-heuristic for Job Shop Scheduling Problems. In 2019 IEEE Congress on Evolutionary Computation (CEC) 57–64 (IEEE, 2019). https://doi.org/10.1109/CEC.2019.8790296 .
Lucas, A. Ising formulations of many NP problems. Front. Physics 2 , 5 (2014).
Albash, T. & Lidar, D. A. Adiabatic quantum computation. Rev. Mod. Phys. 90 , 015002 (2018).
Article ADS MathSciNet Google Scholar
Dickson, N. G. & Amin, M. H. S. Does Adiabatic Quantum Optimization Fail for NP-Complete Problems? Phys. Rev. Lett. 106 , 050502 (2011).
Article ADS PubMed Google Scholar
Heim, B., Ronnow, T. F., Isakov, S. V. & Troyer, M. Quantum versus classical annealing of Ising spin glasses. Science 348 , 215–217 (2015).
Article ADS MathSciNet CAS PubMed Google Scholar
Kadowaki, T. & Nishimori, H. Quantum annealing in the transverse Ising model. Phys. Rev. E 58 , 5355–5363 (1998).
Article ADS CAS Google Scholar
Martoňák, R., Santoro, G. E. & Tosatti, E. Quantum annealing of the traveling-salesman problem. Phys. Rev. E 70 , 057701 (2004).
Okuyama, T., Hayashi, M. & Yamaoka, M. An Ising Computer Based on Simulated Quantum Annealing by Path Integral Monte Carlo Method. In 2017 IEEE International Conference on Rebooting Computing (ICRC) 1–6 (IEEE, 2017). https://doi.org/10.1109/ICRC.2017.8123652 .
Hamerly, R. et al. Experimental investigation of performance differences between Coherent Ising Machines and a quantum annealer. Sci. Adv. 5 , eaau0823 (2019).
Article ADS PubMed PubMed Central Google Scholar
Johnson, M. W. et al. Quantum annealing with manufactured spins. Nature 473 , 194–198 (2011).
Yamaoka, M. et al. A 20k-Spin Ising Chip to Solve Combinatorial Optimization Problems With CMOS Annealing. IEEE J. Solid State Circuits 51 , 303–309 (2016).
Yamaoka, M. et al. 24.3 20k-spin Ising chip for combinational optimization problem with CMOS annealing. In 2015 IEEE International Solid-State Circuits Conference - (ISSCC) Digest of Technical Papers 1–3 (IEEE, 2015). https://doi.org/10.1109/ISSCC.2015.7063111 .
Davendra, D., Metlicka, M. & Bialic-Davendra, M. CUDA Accelerated 2-OPT Local Search for the Traveling Salesman Problem. In Novel Trends in the Traveling Salesman Problem (eds. Davendra, D. & Bialic-Davendra, M.) (IntechOpen, 2020). https://doi.org/10.5772/intechopen.93125 .
Tatsumura, K., Dixon, A. R. & Goto, H. FPGA-Based Simulated Bifurcation Machine. In 2019 29th International Conference on Field Programmable Logic and Applications (FPL) 59–66 (IEEE, 2019). https://doi.org/10.1109/FPL.2019.00019 .
Tatsumura, K., Yamasaki, M. & Goto, H. Scaling out Ising machines using a multi-chip architecture for simulated bifurcation. Nat. Electron 4 , 208–217 (2021).
Mathew, S. K. et al. μ RNG: A 300–950 mV, 323 Gbps/W All-Digital Full-Entropy True Random Number Generator in 14 nm FinFET CMOS. IEEE J. Solid-State Circuits 51 , 1695–1704 (2016).
Pervaiz, A. Z., Sutton, B. M., Ghantasala, L. A. & Camsari, K. Y. Weighted p-Bits for FPGA Implementation of Probabilistic Circuits. IEEE Trans. Neural Netw. Learn. Syst. 30 , 1920–1926 (2019).
Article PubMed Google Scholar
McMahon, P. L. et al. A fully programmable 100-spin coherent Ising machine with all-to-all connections. Science 354 , 614–617 (2016).
Inagaki, T. et al. A coherent Ising machine for 2000-node optimization problems. Science 354 , 603–606 (2016).
Sutton, B., Camsari, K. Y., Behin-Aein, B. & Datta, S. Intrinsic optimization using stochastic nanomagnets. Sci. Rep. 7 , 44370 (2017).
Dutta, S. et al. An Ising Hamiltonian solver based on coupled stochastic phase-transition nano-oscillators. Nat. Electron 4 , 502–512 (2021).
Sharmin, S., Shim, Y. & Roy, K. Magnetoelectric oxide based stochastic spin device towards solving combinatorial optimization problems. Sci. Rep. 7 , 11276 (2017).
Faria, R., Camsari, K. Y. & Datta, S. Implementing Bayesian networks with embedded stochastic MRAM. AIP Adv. 8 , 045101 (2018).
Zand, R., Camsari, K. Y., Datta, S. & DeMara, R. F. Composable Probabilistic Inference Networks Using MRAM-based Stochastic Neurons. J. Emerg. Technol. Comput. Syst. 15 , 1–22 (2019).
Choi, V. Minor-embedding in adiabatic quantum computation: I. The parameter setting problem. Quantum Inf. Process 7 , 193–209 (2008).
Article MathSciNet Google Scholar
Sugie, Y. et al. Minor-embedding heuristics for large-scale annealing processors with sparse hardware graphs of up to 102,400 nodes. Soft Comput 25 , 1731–1749 (2021).
Cai, J., Macready, W. G. & Roy, A. A practical heuristic for finding graph minors. Preprint at http://arxiv.org/abs/1406.2741 (2014).
Chaves-O’Flynn, G. D., Wolf, G., Sun, J. Z. & Kent, A. D. Thermal Stability of Magnetic States in Circular Thin-Film Nanomagnets with Large Perpendicular Magnetic Anisotropy. Phys. Rev. Appl. 4 , 024010 (2015).
Mitra, D., Romeo, F. & Sangiovanni-Vincentelli, A. Convergence and finite-time behavior of simulated annealing. Adv. Appl. Probab. 18 , 747–771 (1986).
Mills, K., Ronagh, P. & Tamblyn, I. Finding the ground state of spin Hamiltonians with reinforcement learning. Nat. Mach. Intell. 2 , 509–517 (2020).
MP-TESTDATA - The TSPLIB Symmetric Traveling Salesman Problem Instances. http://comopt.ifi.uni-heidelberg.de/software/TSPLIB95/tsp/ (2013).
Dan, A., Shimizu, R., Nishikawa, T., Bian, S. & Sato, T. Clustering Approach for Solving Traveling Salesman Problems via Ising Model Based Solver. In 2020 57th ACM/IEEE Design Automation Conference (DAC) 1–6 (IEEE, 2020). https://doi.org/10.1109/DAC18072.2020.9218695 .
Ezugwu, A. E.-S., Adewumi, A. O. & Frîncu, M. E. Simulated annealing based symbiotic organisms search optimization algorithm for traveling salesman problem. Expert Syst. Appl. 77 , 189–210 (2017).
Mohsen, A. M. Annealing Ant Colony Optimization with Mutation Operator for Solving TSP. Comput. Intell. Neurosci. 2016 , 1–13 (2016).
Wang, J., Ersoy, O. K., He, M. & Wang, F. Multi-offspring genetic algorithm and its application to the traveling salesman problem. Appl. Soft Comput. 43 , 415–423 (2016).
Talatchian, P. et al. Mutual control of stochastic switching for two electrically coupled superparamagnetic tunnel junctions. Phys. Rev. B 104 , 054427 (2021).
Cai, F. et al. Power-efficient combinatorial optimization using intrinsic noise in memristor Hopfield neural networks. Nat. Electron 3 , 409–418 (2020).
Hayakawa, K. et al. Nanosecond Random Telegraph Noise in In-Plane Magnetic Tunnel Junctions. Phys. Rev. Lett. 126 , 117202 (2021).
Safranski, C. et al. Demonstration of Nanosecond Operation in Stochastic Magnetic Tunnel Junctions. Nano Lett. 21 , 2040–2045 (2021).
Download references
Acknowledgements
This work was supported by National Research Foundation (NRF), Prime Minister’s Office, Singapore, under its Competitive Research Programme (NRF-000214-00 to H.Y.), Advanced Research and Technology Innovation Center (ARTIC to H.Y.), the National University of Singapore under Grant (project number: A-0005947-19-00 to H.Y.), and Ministry of Education, Singapore, under Tier 2 (T2EP50123-0025 to H.Y.). We thank Yuqi Su, and Chne-Wuen Tsai from National University of Singapore and Zhi-Da Song from Peking University for useful discussions.
Author information
Authors and affiliations.
Department of Electrical and Computer Engineering, National University of Singapore, Singapore, Singapore
Jia Si, Shuhan Yang, Yunuo Cen, Jiaer Chen, Yingna Huang, Zhaoyang Yao, Dong-Jun Kim, Kaiming Cai, Jerald Yoo, Xuanyao Fong & Hyunsoo Yang
Key Laboratory for the Physics and Chemistry of Nanodevices and Center for Carbon-based Electronics, School of Electronics, Peking University, Beijing, China
You can also search for this author in PubMed Google Scholar
Contributions
J.S. and H.Y. conceived and designed the experiments. J.S. designed, fabricated, and coded the hardware system. D.K., and S.Y. fabricated the devices. J.S., S.Y., and K.C. performed device measurements. Z.Y. bonded the components on PCB. J.S. designed SMTJ-based Ising system. J.S., J.C., Y.C., Y.H. and X.F. developed the optimization algorithm and performed simulations. J.S., S.Y., Y.C., J.Y., X.F. and H.Y. analyzed the data. J.S. and H.Y. wrote the manuscript. H.Y. proposed and supervised this work. All authors discussed the results and revised the manuscript.
Corresponding author
Correspondence to Hyunsoo Yang .
Ethics declarations
Competing interests.
The authors declare no competing interests.
Peer review
Peer review information.
Nature Communications thanks the anonymous reviewers for their contribution to the peer review of this work.
Additional information
Publisher’s note Springer Nature remains neutral with regard to jurisdictional claims in published maps and institutional affiliations.
Supplementary information
Supplementary information, source data, source data, rights and permissions.
Open Access This article is licensed under a Creative Commons Attribution 4.0 International License, which permits use, sharing, adaptation, distribution and reproduction in any medium or format, as long as you give appropriate credit to the original author(s) and the source, provide a link to the Creative Commons licence, and indicate if changes were made. The images or other third party material in this article are included in the article’s Creative Commons licence, unless indicated otherwise in a credit line to the material. If material is not included in the article’s Creative Commons licence and your intended use is not permitted by statutory regulation or exceeds the permitted use, you will need to obtain permission directly from the copyright holder. To view a copy of this licence, visit http://creativecommons.org/licenses/by/4.0/ .
Reprints and permissions
About this article
Cite this article.
Si, J., Yang, S., Cen, Y. et al. Energy-efficient superparamagnetic Ising machine and its application to traveling salesman problems. Nat Commun 15 , 3457 (2024). https://doi.org/10.1038/s41467-024-47818-z
Download citation
Received : 16 June 2022
Accepted : 11 April 2024
Published : 24 April 2024
DOI : https://doi.org/10.1038/s41467-024-47818-z
Share this article
Anyone you share the following link with will be able to read this content:
Sorry, a shareable link is not currently available for this article.
Provided by the Springer Nature SharedIt content-sharing initiative
By submitting a comment you agree to abide by our Terms and Community Guidelines . If you find something abusive or that does not comply with our terms or guidelines please flag it as inappropriate.
Quick links
- Explore articles by subject
- Guide to authors
- Editorial policies
Sign up for the Nature Briefing newsletter — what matters in science, free to your inbox daily.

What a lovely hat
Is it made out of tin foil , paper 2024/626, exponential quantum speedup for the traveling salesman problem.
The traveling salesman problem is the problem of finding out the shortest route in a network of cities, that a salesman needs to travel to cover all the cities, without visiting the same city more than once. This problem is known to be $NP$-hard with a brute-force complexity of $O(N^N)$ or $O(N^{2N})$ for $N$ number of cities. This problem is equivalent to finding out the shortest Hamiltonian cycle in a given graph, if at least one Hamiltonian cycle exists in it. Quantum algorithms for this problem typically provide with a quadratic speedup only, using Grover's search, thereby having a complexity of $O(N^{N/2})$ or $O(N^N)$. We present a bounded-error quantum polynomial-time (BQP) algorithm for solving the problem, providing with an exponential speedup. The overall complexity of our algorithm is $O(N^3\log(N)\kappa/\epsilon + 1/\epsilon^3)$, where the errors $\epsilon$ are $O(1/{\rm poly}(N))$, and $\kappa$ is the not-too-large condition number of the matrix encoding all Hamiltonian cycles.
Note: 6 pages, 2 figures, comments welcome.
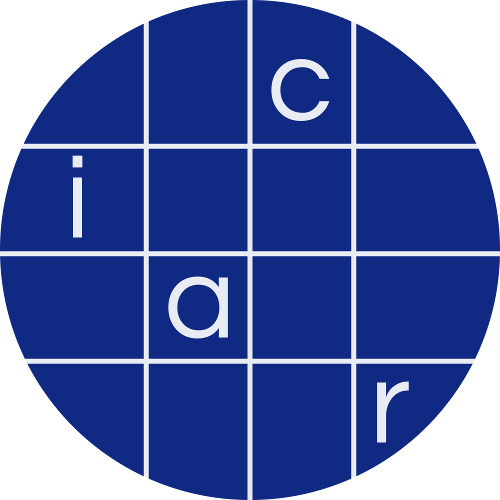

IMAGES
VIDEO
COMMENTS
A TSP tour in the graph is 1-2-4-3-1. The cost of the tour is 10+25+30+15 which is 80. The problem is a famous NP-hard problem. There is no polynomial-time known solution for this problem. Examples: Output of Given Graph: minimum weight Hamiltonian Cycle : 10 + 25 + 30 + 15 := 80.
It is used as a benchmark for many optimization methods. Even though the problem is computationally difficult, ... In March 2005, the travelling salesman problem of visiting all 33,810 points in a circuit board was solved using Concorde TSP Solver: a tour of length 66,048,945 units was found, and it was proven that no shorter tour exists. The ...
The Traveling Salesman Problem (TSP) is a well-known challenge in computer science, mathematical optimization, and operations research that aims to locate the most efficient route for visiting a group of cities and returning to the initial city.TSP is an extensively researched topic in the realm of combinatorial optimization.It has practical uses in various other optimization problems ...
The traveling salesman problem (TSP) is a widely studied combinatorial optimization problem, which, given a set of cities and a cost to travel from one city to another, seeks to identify the tour that will allow a salesman to visit each city only once, starting and ending in the same city, at the minimum cost. 1
1. Introduction. The traveling salesman problem (TSP) is undoubtedly the most extensively studied problem in combinatorial optimization. In popular language, the TSP can be described as the problem of finding a minimum distance tour of n cities, starting and ending at the same city and visiting each other city exactly once. In spite of the simplicity of its problem statement, the TSP is ...
The traveling salesman problem is a classic problem in combinatorial optimization. This problem is finding the shortest path a salesman should take to traverse a list of cities and return to the origin city. The list of cities and the distance between each pair are provided. TSP is beneficial in various real-life applications such as planning ...
The traveling salesman problem (TSP) involves finding the shortest path that visits n specified locations, starting and ending at the same place and visiting the other n-1 destinations exactly ...
2.2 Traditional TSP Algorithms. The traditional methods for solving the Traveling Salesman Problem can be categorized into two types: exact algorithms and heuristic and Approximation Methods. Exact algorithms can directly compute the optimal solution, particularly for smaller instances, through methods like iteration.
2.7 Traveling Salesman Problem. The traveling salesman problem (TSP) is a popular and well-studied combinatorial problem in operations research. Scientists have been paying serious attention to this topic for many decades. Several exact, heuristic, and metaheuristic TSP methods have been proposed.
The Traveling Salesman Problem (TSP) is perhaps the most studied discrete optimization problem. Its popularity is due to the facts that TSP is easy to formulate, difficult to solve, and has a large number of applications. It appears that K. Menger [ 31] was the first researcher to consider the Traveling Salesman Problem (TSP).
For the following exercises, use your solutions to the indicated exercises to compare the results of the brute force method to the results of the nearest neighbor method for each traveling salesman problem of finding a reasonably short route to leave from city U, visit each of the other cities listed and return to city U. Indicate whether the ...
The Traveling Salesman Problem (TSP) is the challenge of finding the shortest path or shortest possible route for a salesperson to take, given a starting point, a number of cities (nodes), and optionally an ending point. ... For larger real-world travelling salesman problems, when manual methods such as Google Maps Route Planner or Excel route ...
The Traveling Salesman Problem (TSP) is believed to be an intractable problem and have no practically efficient algorithm to solve it. The intrinsic difficulty of the TSP is associated with the combinatorial explosion of potential solutions in the solution space. When a TSP instance is large, the number of possible solutions in the solution space is so large as to forbid an exhaustive search ...
The Traveling Salesman Problem (TSP) is a problem that is interesting to study because it is very practical, but so time consuming to solve, that it becomes nearly impossible to find the shortest route, even in a graph with just 20-30 vertices. ... This is a type of algorithm inspired by the process of natural selection and use techniques such ...
Here are the Top 5 solutions to the Traveling Salesman Problem (TSP): 1. Brute Force Algorithm. The Brute Force algorithm is a straight approach to solving the Traveling Salesman Problem (TSP). It systematically explores all possible routes to identify the shortest one among them all.
Traveling Salesman Problem (TSP) is a main attention issue at present. Neural network can be used to solve combinatorial optimization problems. In recent years, there have existed many neural network methods for solving TSP, which has made a big step forward for solving combinatorial optimization problems. This paper reviews the neural network ...
Let us look at the output of this method. Visualization of Greedy Algorithm Output Travelling Salesman Greedy Algorithm Output. Thus, according to the Greedy algorithm, we will travel to city 1 first, and cities 1,3,2 after that. Brute Force Approach to the Traveling Salesman Problem. Let us look at the code of the Brute Force Method.
The Travelling Salesman Problem (also known as the Travelling Salesperson Problem or TSP) is an NP-hard graph computational problem where the salesman must visit all cities (denoted using vertices in a graph) given in a set just once. The distances (denoted using edges in the graph) between all these cities are known.
The Traveling Salesman Problem is to find the circuit that visits every vertex (at least once) and minimizes the total weight of its edges. The Traveling Salesman Problem could also be called the UPS Deliveryman Problem. There is a weight (or cost) to each edge of the graph. The weight could be expressed as. Distance - Find the shortest circuit.
Travelling salesman problem takes a graph G {V, E} as an input and declare another graph as the output (say G') which will record the path the salesman is going to take from one node to another. The algorithm begins by sorting all the edges in the input graph G from the least distance to the largest distance. The first edge selected is the ...
Step1: Create a class (Node) that can store the reduced matrix, cost, current city number, level (number of cities visited so far), and path visited till now. Step2: Create a priority queue to store the live nodes with the minimum cost at the top. Step3: Initialize the start index with level = 0 and reduce the matrix.
Conclusion. This paper proposed an effective method based on NSGA-II to solve the multiple traveling salesman problem. The total travel distance and the difference between the longest subtour and the shortest one are the two conflict objectives. A novel crossover operator is designed to generate new offspring, and two mutation operators are ...
The method involves two learning phases: initially, a model-free reinforcement learning approach leverages privileged information to distill knowledge from expert trajectories generated by the LinKernighan heuristic (LKH) algorithm. ... Dubins traveling salesman problem with neighborhoods: A graph-based approach. Algorithms, 6(1):84-99, 2013 ...
The traveling-salesman problem is a generalized form of the simple problem to find the smallest closed loop that connects a number of points in a plane. Efforts in the past to find an efficient method for solving it have met with only partial success. The present paper describes a method of solution that has the following properties ( a) It is ...
Traveling Salesman Problems(DTSP) with Neighborhood (DTSPN) to quickly produce a tour of a non-holonomic vehicle passing through neighborhoods of given task points. The method involves two learn-ing phases: initially, a model-free reinforcement learning approach leverages privileged information to distill knowledge from expert tra-
Here, we experimentally present an Ising annealing computer based on 80 superparamagnetic tunnel junctions (SMTJs) with all-to-all connections, which solves a 70-city traveling salesman problem ...
the traveling-salesman problem for bounded-degree graphs," Physical Review A, vol. 95, no. 3, p. 032323, March 2017. [18]C. Tszyunsi and I. I. Beterov, "A quantum algorithm for solving the travelling salesman problem by quantum phase estimation and quantum search," Journal of Experimental and Theoretical Physics, vol. 137, pp.
Traveling Salesperson Problem Hamiltonian Cycle Problem Quantum Algorithm Exponential Speedup Contact author(s) anantsharma2410 @ gmail com rajeshdeshpande @ students iisertirupati ac in sanchita ghosh14 @ gmail com sreetama das @ ino cnr it roy shibdas @ gmail com History 2024-04-26: approved 2024-04-23: received See all versions Short URL
The application of Traveling Salesman Problem can be seen in various areas of life including the problem of determining the shortest route in the distribution of rice. The problem of choosing the optimal route for rice distribution in this study was taken using data on rice refineries in the Simalungun area. So many agents have become customers of the rice refinery. Currently, these agents are ...