Pupillary correlates of lapses of sustained attention
- Published: 01 April 2016
- Volume 16 , pages 601–615, ( 2016 )

Cite this article
- Nash Unsworth 1 &
- Matthew K. Robison 1
8370 Accesses
145 Citations
13 Altmetric
Explore all metrics
The current study examined the extent to which pupillary responses (both pretrial baseline and phasic responses) would accurately track lapses of attention as predicted by theories of locus coeruleus norepinephrine (LC-NE) functioning. Participants performed a sustained attention task while pupil responses were continuously recorded. Periodically during the task, participants were presented with thought probes to determine if they were on or off task. The results suggested the pupillary responses accurately distinguished on from off-task states. Importantly, pretrial baseline pupil responses distinguished different types of lapses of attention, with inattentive and mind-wandering states being associated with small pretrial baseline pupil diameters on average and distracted states being associated with larger pretrial baseline pupil diameters on average compared to focused states. These results support the notion that pupil diameter is sensitive to different types of lapses of attention which may be associated with different LC-NE modes.
Similar content being viewed by others
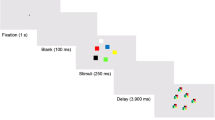
Pupillometry tracks fluctuations in working memory performance
Matthew K. Robison & Nash Unsworth
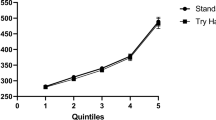
Effort Mobilization and Lapses of Sustained Attention
Nash Unsworth, Ashley L. Miller & Shadee Aghel
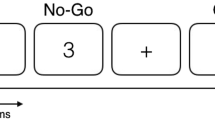
Influence of content and intensity of thought on behavioral and pupil changes during active mind-wandering, off-focus, and on-task states
Esperanza Jubera-García, Wim Gevers & Filip Van Opstal
Avoid common mistakes on your manuscript.
The ability to focus and sustain attention on task-relevant information is a critically important skill that is needed in a host of everyday activities. Despite the importance of focusing and sustaining attention on goal-relevant information, sometimes the attention system falters, leading to lapses. Understanding these lapses, whereby attention is disengaged from the current task and focused on other external distracting stimuli or internal thoughts (daydreaming), is important for understanding how and when attentional processes falter in both the laboratory and in real-world situations (Reason, 1984 ). For example, the ability to focus attention is needed in a host of activities where any lapses of attention could result in unwanted outcomes, such driving accidents, lower academic performance, and failures to spot weapons during baggage screening (Reason, 1990 ; Unsworth, McMillan, Brewer, & Spillers, 2012 ). Gaining a better understanding of fluctuations in attention is important for understanding the attentional system more broadly and for predicting when and for whom attention failures are most likely.
Regulating Attentional State
Theoretically, cognitive control processes are needed to ensure active goal maintenance leading to task-appropriate behaviors. Prominent theories of cognitive control suggest that cognitive control is implemented by the prefrontal cortex in situations where task goals need to be actively maintained and dynamically updated (Miller & Cohen, 2001 ). Accordingly, top-down attention control processes are needed to maintain task goals and bias responding so that the appropriate behavior is executed. In many situations, participants will have to actively maintain a novel task goal that is in direct opposition to prepotent response tendencies (Roberts & Pennington, 1996 ). If there is a failure of active goal maintenance, then it is likely that prepotent response tendencies will guide behavior, leading to goal neglect and the execution of the incorrect response (Duncan, 1995 ). In situations when attention is tightly focused on the task goal, performance will be both fast and accurate. However, if attention is not tightly focused on the task goal, goal neglect can occur. Thus, any lapses in attention will result in prepotent responses guiding behavior and hence the occurrence of fast reflexive errors (Unsworth, Schrock, & Engle, 2004 ) or much slower than normal response times (Unsworth, Redick, Lakey, & Young, 2010 ).
Recent research suggests that the locus coeruleus norepinephrine system (LC-NE) may be particularly important in modulating prefrontal cortex representations based on attentional control demands (Cohen, Aston-Jones, & Gilzenrat, 2004 ). The LC is a brainstem neuromodulatory nucleus that is responsible for most of the NE released in the brain and it has widespread projections throughout the neocortex (Berridge & Waterhouse, 2003 ). Generally the LC-NE system has been associated with general functions such as the sleep-wake cycle and overall arousal levels. More recently neurophysiological and computational modeling studies (Aston-Jones & Cohen, 2005 ; Aston-Jones, Iba, Clayton, Rajkowski, & Cohen, 2007 ; Usher, Cohen, Servan-Schreiber, Rajkowski, & Aston-Jones, 1999 ) have suggested that the LC-NE system is important for regulating the balance between exploitation (maintaining attention on the current task) and exploration (task disengagement). A great deal of recent research (see Aston-Jones & Cohen, 2005 ; Berridge & Waterhouse, 2003 , for reviews) suggests that there is an inverted-U relationship between LC tonic (baseline) activity and performance on various cognitive tasks consistent with the Yerkes–Dodson curve (Yerkes & Dodson, 1908 ). Specifically, it is assumed that when tonic LC activity is low (hypoactive mode), individuals are inattentive and nonalert, leading to poor behavioral performance and little to no phasic LC activity in response to task-relevant stimuli. As tonic LC activity increases to an intermediate range (phasic mode), attention becomes more focused, LC phasic activity increases for target stimuli, and behavioral performance is optimal. However, as tonic LC activity increases further, the individual experiences a more distractible attentional state, leading to task disengagement, lowered LC phasic activity, and a reduction in behavioral performance. Intracranial recordings in rats and monkeys and psychopharmacological studies in animals and humans provide evidence in support of the notion of an inverted-U relationship between the LC-NE system and behavioral performance (Aston-Jones & Cohen, 2005 ). Collectively this research suggests that the LC exhibits fluctuations between these various modes/states during simple attentional tasks, and these fluctuations are linked to fluctuations in behavioral performance. As such, prior research suggests that LC-NE system is important in regulating the current attentional state and that fluctuations in attentional state correspond to fluctuations in tonic LC activity.
Pupil Diameter and the LC-NE System
A potential candidate as a psychophysiological marker for changes in attentional state is pupil diameter. Much prior research has shown that the pupil dilates in response to the cognitive demands of a task (Beatty, 1982a ). For example, Hess and Polt ( 1964 ) demonstrated that the pupils dilated as a function of problem difficulty in a mental multiplication task with higher peak dilations for the hardest problems. Similarly, Kahneman and Beatty ( 1966 ) demonstrated that pupillary dilation increased as more items were required for recall in a standard short-term memory task (see also Unsworth & Robison, 2015 ). These effects reflect task-evoked pupillary responses (TEPRs) in which the pupil dilates relative to baseline levels due to increases in cognitive processing load. A number of studies have demonstrated similar TEPRs in a variety of tasks (see Beatty & Lucero-Wagoner, 2000 , for a review). These and other results led Kahneman ( 1973 ) and Beatty ( 1982a ) to suggest that TEPRs are a reliable and valid psychophysiological marker of cognitive effort or processing load.
More recently, research has suggested that pupil dilations are indirectly related to the functioning of the LC-NE system (Aston-Jones & Cohen, 2005 ; Gilzenrat et al., 2010 ; Joshi Cohen, Servan-Schreiber, Rajkowski, & Aston-Jones, 2016 ). Specifically, Aston-Jones and Cohen ( 2005 ; based on results from Rajkowski, Kubiak, & Aston-Jones, 1993 ) demonstrated a modest correlation between baseline pupil diameter and baseline firing rate of an LC neuron in a monkey (see also recent work by Joshi et al., 2016 ). According to Aston-Jones and Cohen ( 2005 ; see also Gilzenrat et al., 2010 ; Jepma & Nieuwenhuis, 2011 ; Joshi et al., 2016 ), pupil diameter provides an indirect index of the LC modes such that in the hypoactive mode overall baseline pupil diameter is small. In the tonic LC mode, overall baseline pupil diameter is relatively large. Finally, in the LC phasic mode, overall baseline pupil diameter tends to be at intermediate levels. Collectively, this work suggests that baseline pupil diameter should provide an indirect index of LC-NE functioning. For example, prior research has demonstrated that drugs such as modafinil increase central NE, leading to increases in subjective alertness and increases in baseline pupil diameter (Hou, Freeman, Langley, Szabadi, & Bradshaw, 2005 ). Drugs such as clonidine decrease central NE, leading to lowered subjective alertness and lower baseline pupil diameter (Hou et al., 2005 ). Furthermore, recent neuroimaging work has shown that activity in the LC is correlated with changes in pupil diameter (Alnaes et al., 2014 ; Murphy, O’Connell, O’Sullivan, Robertson, & Balsters, 2014 ). Thus, there is a correlation between baseline pupil diameter and baseline firing rate of LC neurons, and drugs that increase or decrease central NE levels lead to increases or decreases in baseline pupil diameter. This work suggests that baseline pupil diameter can be used as an indirect reporter variable of LC-NE functioning.
Although prior work is suggestive of a link between LC-NE functioning and pupil diameter, it should be acknowledged that this relation is correlational in nature and it could be due to the fact that both the LC and the sympathetic nervous system are linked via a third system. Specifically, Nieuwenhuis, de Geus, and Aston-Jones ( 2011 ) suggested that the LC–pupil link is not due to a direct causal pathway but rather is due to the fact that both the LC and the sympathetic nervous system are controlled by the nucleus paragigantocellularis. The influence of NE pharmacological manipulations on baseline pupil diameter could thus be due to the influence of lower medullary NE cell groups rather than the LC directly. It is also possible that pupillary changes reflect drug effects on the peripheral (rather than the central) nervous system, where NE is an important neurotransmitter. More definitive work is needed to establish the relationship between the LC-NE and pupil diameter. For now, pupil diameter can be used as an indirect measure of LC-NE functioning, while acknowledging that the relation could be due to a third variable (the nucleus paragigantocellularis).
Several recent studies have begun to explore whether pupil diameter provides an index of LC-NE functioning and changes in control state. For example, using a similar auditory detection task as that used by Rajkowski et al. ( 1993 ) to examine the relation between pupil diameter and LC tonic activity, Gilzenrat et al. ( 2010 ) found that trials that were preceded by large baseline pupil diameters were associated with poorer performance indicative of lapses of engagement corresponding to the LC tonic mode. Trials that were preceded by smaller baseline pupil were associated with better task performance indicative of task engagement corresponding to the LC phasic mode. Corroborative results were found in their additional experiments, suggesting that pupil diameter tracks changes in control state related to LC activity. Furthermore, Murphy et al. ( 2011 ) found an inverted-U relationship between baseline pupil size and performance on an auditory oddball task such that performance (in particular, variability in reaction time) was worse when baseline pupil was very small or very large, but performance was best at intermediate baseline levels. Murphy et al. suggested that baseline pupil diameter not only provides an index of the tonic and phasic modes (the upper and intermediate ends of the LC-NE curve) but also provides an index of low levels of alertness and arousal (the lower end of the curve) and suggested that when examining links between pupil diameter and LC activity, the entire curve must be taken into account. This is consistent with prior research, which has consistently shown that under conditions of fatigue or low levels of alertness, baseline pupil diameter is smaller and more variable than when alert (Hou et al., 2005 ; Morad, Lemberg, Yofe, Dagan, 2000 ). Likewise, Kristjansson et al. ( 2009 ) found that baseline pupil diameter was much smaller on trials preceding very slow reaction times (indicative of lapses of attention) on a vigilance task compared to trials where reaction time was close to the mean. Kristjansson et al. suggested that fluctuations in alertness resulted in variable reaction times and that baseline pupil diameter provides an index of changes in alertness. Collectively, these results suggest that baseline pupil diameter provides an index of LC-NE functioning and can be used to track fluctuations in attentional control state.
Thought Probing and Attentional State
The aforementioned work suggests the importance of maintaining goal-relevant information in an active state to ensure accurate responding and further suggests that periodic lapses in attention or task engagement can lead to deficits in behavioral performance. Furthermore, prior research has suggested that baseline pupil diameter may provide an index of changes in attentional state. Another way of examining changes in attentional state is to simply ask participants what their current attention state is. Prior research has suggested that participants’ self-reports of their attentional state provides important and useful information for understanding changes in attentional state (Smallwood & Schooler, 2006 ). Specifically, a number of studies have utilized thought-probe techniques in which, periodically, during a prolonged attention task, participants are probed and are required to report whether their attention was currently focused on task or whether they were thinking of things unrelated to the task (i.e., mind-wandering). This research has consistently found that not only do participants report extensive off-task thoughts during attentional tasks, but also these self-reports of off-task thoughts are correlated with actual performance, such that self-reports of off-task thoughts are associated with lower levels of performance (McVay & Kane, 2012b ; Schooler, Reichle, & Halpern, 2005 ). Additionally, rates of off-task thoughts have been shown to be associated with very slow reaction times, which have long been seen as indicators of lapses of attention (McVay & Kane, 2012a ; Unsworth et al., 2010 ). Prior work suggests that the probe techniques for examining variation in attentional state have been shown to be both reliable and valid and have demonstrated the importance of examining fluctuations in attentional state during a number of tasks and situations.
Most prior research that has used thought probe techniques has focused on whether participants are focused on the current task or whether they are mind-wandering. However, when participant’s attention is not on the current task it is not always because they are mind-wandering. Rather, it is possible that sometimes participants engage in off-task thoughts about external distractors as well as internal distractors (i.e., mind-wandering). Stawarczyk, Majerus, Maj, Van der Linden, and D’Argembeau ( 2011 ) introduced a novel experience sampling method to distinguish the different varieties of off task thoughts. Specifically, Stawarczyk et al. used a thought-probe technique in which participants were not simply instructed to indicate if they were on or off task, but rather participants had to indicate if they were on task, if they were experiencing task-related interference (interfering thoughts related to the appraisal of the current task such as worrying about performance), if they were distracted by external stimuli, or if they were mind-wandering. Implementing this technique in a sustained attention task, Stawarczyk et al. ( 2011 ) found that roughly 20 % of the responses to the thought probes were external distractions and roughly 21 % were mind-wandering. Furthermore, when participants reported that they experienced either external distraction or mind-wandering, performance was worse than when participants reported that they were focused on the task. In another recent study, we (Unsworth & McMillan, 2014a ) had participants perform a variety of attention control tasks, and participants responded to the same thought probes as used Stawarczyk et al. ( 2011 ). We found that participants who reported more lapses of attention (i.e., more mind-wandering and more external distraction) also tended to have more variable reaction times and more slow reaction times (see also McVay & Kane, 2012a ). Thus, off-task thinking can be directed towards internal thoughts and concerns (mind-wandering) or toward external stimuli unrelated to the task at hand (external distraction), and both of these can lead to lapses in performance. Finally, it is also likely that when participants report that they are not focused on the current task at hand, that sometimes they are simply inattentive (nonalert) and are not directing their thoughts to internal concerns or to external stimuli, but rather they are simply zoning out or experiencing mind-blanking (Ward & Wegner, 2013 ), leading to lower behavioral performance (Unsworth & McMillan, 2014b ). Thus, thought-probe techniques can be used to examine fluctuations in attentional state and be used to classify various attentional states (i.e., focused, inattentive, mind-wandering, and/or distracted).
The Current Study
Despite initial research examining fluctuations in attention and control state with pupillary responses, more research is needed to better determine the extent to which fluctuations in various attentional states can be tracked accurately with changes in pupillary dilations and the extent to which these fluctuations correspond to subjective changes in attentional state. To our knowledge, only three prior studies have examined a potential link between changes in baseline pupil diameter and subjective attentional state by examining differences between on- and off-task states while simultaneously measuring baseline pupil diameter (Franklin, Broadway, Mrazek, Smallwood, & Schooler, 2013 ; Grandchamp, Braboszcz, & Delorme, 2014 ; Mittner et al., 2014 ). In one of these studies (Franklin et al., 2013 ) the authors found that off-task reports (thought to reflect mind-wandering) were associated with larger baseline pupil diameters than were on-task reports. However, both Grandchamp et al. ( 2014 ) and Mittner et al. ( 2014 ) found that off-task reports were associated with smaller baseline pupil diameters than were on-task reports. Thus, there are clear conflicting results in the literature. One limitation with this prior research is that participants were simply asked if their current thoughts were on or off task. As noted previously, this simple dichotomous response ignores the many varieties of attentional lapses (e.g., inattentive, mind-wandering, distracted) and thus, it is unclear how pupil diameter is related to different attentional states.
It should be noted that other studies have examined the link between pupil diameter and attentional state, but these studies did not simultaneously measure pupil diameter and attentional state at the same time (Kang, Huffer, & Wheatley, 2014 ; Smallwood, Brown, Tipper, et al., 2011 ; Smallwood, Brown, Baird, et al., 2012 ). Specifically, Smallwood et al. ( 2011 ) and Kang et al. ( 2014 ) demonstrated larger baseline pupil diameters in a choice reaction time task than in a working memory task and suggested that the larger baseline pupil diameters reflected more mind-wandering. In a separate experiment, Smallwood et al. ( 2011 ) demonstrated that more mind-wandering occurred in the choice reaction-time task than in the working memory task, but unfortunately they did not simultaneously measure both pupil diameter and attentional state in the same experiment, so it is unclear whether baseline pupil diameter is larger or smaller during a mind-wandering episode. In a similar vein, Smallwood et al. ( 2012 ) measured pupillary responses during a vigilance task but measured mind-wandering after the task with a questionnaire. Smallwood et al. found that individuals who reported more mind-wandering had larger pupils than individuals who reported less mind-wandering. Although there was a positive relation between pupil diameter and mind-wandering, again, pupil diameter and attentional state were not measured at the same time, and there is no indication for what the relation is within subjects (rather than between subjects). Thus, the more evidence is needed to examine the relation between pupillary responses and attentional state.
To examine whether baseline pupil diameter accurately tracks different attentional states, in the current study we had participants perform a sustained attention task (the psychomotor vigilance task; Dinges & Powell, 1985 ). This task has long been used as a measure of sustained attention to examine how changes in alertness affect vigilant attention (Dorrian, Rogers, & Dinges, 2005 ). Additionally, factors such as sleep deprivation tend to amplify these effects (Dorrian et al., 2005 ) and the slowest RTs in this task have been found to be linked to greater activity in the default-mode network (Drummond et al., 2005 ). Recent work also suggests that performance on this task correlates with performance on other attentional control tasks, including the Stroop task, flankers, antisaccade, and the sustained attention to response task (e.g., Unsworth & McMillan, 2014a ). Thus, there is good evidence that the slowest reaction times in this task provide an index of lapses of attention. During the psychomotor vigilance task, participants were periodically presented with thought probes asking whether they were on task, thinking about their performance on the task, distracted by external information, mind-wandering, or simply inattentive and not alert. Throughout the task participants’ pupils were continuously measured. Building on prior research, it is assumed that baseline pupil diameter should provide an index of when individuals are focused on a task and when they are experiencing different types of lapses of attention. Specifically, Lenartowicz, Simpson, and Cohen ( 2013 ) suggested a landscape of attentional lapses wherein when arousal is low and attention is focused internally, mind-wandering occurs. When arousal is low and attention is focused externally, attentional capture from bottom-up sources is expected to occur. When arousal is high and attention is focused internally, this can lead to internal distraction in the form of ruminations and racing thoughts. When arousal is high and attention is focused externally, this can lead to external distraction, resulting in oversensitivity to external stimuli. Thus, Lenartowicz et al. ( 2013 ) suggest that the type of lapse of attention is determined by arousal levels from the LC-NE system and by whether attention is directed to external stimuli or to internal thoughts.
In terms of pupil diameter, this suggests that baseline pupil diameter should provide a means to track different types of lapses of attention. When baseline pupil diameter is small participants should report being inattentive/nonalert (corresponding to low tonic LC activity and low arousal), resulting in lower task performance and more lapses of attention. At intermediate baseline pupil diameters, participants should be focused on the task at hand (corresponding to the LC phasic mode and optimal arousal levels), leading to good task performance. Finally, when baseline pupil diameter is relatively large, participants should report that they are distracted and disengaged from the task (corresponding to the LC tonic mode and over arousal) leading to lower task performance and increases in lapses of attention. Furthermore, we examined time on task effects to determine how pupil diameter changes as a function of time on task and whether this is related to corresponding changes in performance and attentional state. Specifically, as time on task increases, we expect that performance should worsen (i.e., the vigilance decrement), and participants should report being unfocused and off task more (i.e., more mind-wandering and inattentiveness), and pupil diameter should correspond to changes in attentiveness. Thus, it is predicted that there should be a link not only between pupil diameter and performance but also between pupil diameter and different attentional state reports.
Participants
Participants were 39 undergraduate students recruited from the subject pool at the University of Oregon. Based on prior pupillometry work, which suggested a medium to large effect size (Franklin et al., 2013 ; Grandchamp et al., 2014 ; Mittner et al., 2014 ) we determined that a minimum sample size of 35 participants would be sufficient to find a medium effect size, with power of .80 and alpha set at .05 (two tailed). Participants were between the ages of 18 and 35 and received course credit for their participation. Data from two participants were excluded from analyses because of data collection problems with the eye tracker, leaving a final sample of 37 participants.
Participants were tested individually in a dimly lit room. Pupil diameter was continuously recorded binocularly at 120 Hz using a Tobii T120 eye tracker. Participants were seated approximately 60 cm from the monitor. After providing informed consent and after calibrating the eye tracker, participants performed a variant of the psychomotor vigilance task (Dinges & Powell, 1985 ). Stimuli were presented on the Tobii T120 eye tracker 17-in. monitor with a 1,024 × 768 screen resolution. As shown in Figure 1 , in this task participants were first presented with a row of five black fixation crosses in the middle of the screen on a gray background for 2,000 ms. Participants were then presented with a row of zeros in blue Arial 24-point font (visual angle 1.21°) in the center of the screen, and after a variable wait time (equally distributed from 2 to 10 s in in 500 ms increments) the zeros began to count up in 17-ms intervals from 0 ms. The participants’ task was to press the spacebar as quickly as possible once the numbers started counting up. After pressing the spacebar, the RT was left on screen in red for 1 s to provide feedback to the participants (shown in bold in Figure 1 ). Following feedback ,a 500 ms blank screen was presented and then either the next trial started or participants were presented with a thought probe. Participants performed 160 trials, and the experiment lasted approximately 30 min. Thirty thought probes were randomly presented after roughly 19 % of the trials equally distributed across blocks.
Schematic of the experimental task with a thought-probe after the trial
Thought Probes
During the task, participants were periodically presented with thought probes asking them to classify their immediately preceding thoughts. The thought probes asked participants to press one of five keys to indicate what they were thinking just prior to the appearance of the probe. Specifically, participants saw the following:
Please characterize your current conscious experience.
I am totally focused on the current task.
I am thinking about my performance on the task or how long it is taking.
I am distracted by sights/sounds/temperature or by physical sensations (hungry/thirsty).
I am daydreaming/my mind is wandering about things unrelated to the task.
I am not very alert/my mind is blank or I’m drowsy.
These thought probes were based on those used by Stawarczyk et al. ( 2011 ) and Unsworth and McMillan ( 2014a ). Participants were given specific instructions regarding the different categories based on prior research (McVay & Kane, 2012b ; Stawarczyk et al., 2011 ; Unsworth & McMillan, 2014a ). Similar to prior research, response three was classified as external distraction and response four was classified as mind-wandering. Response five was classified as inattentive/nonalert. Response one was considered as on-task thoughts, while response two was considered as task-related interference.
Data Analysis
Data from each participant’s left eye was used (left and right eye pupil diameter were highly correlated, r = .89). Missing data points due to blinks, off-screen fixations, and/or eye-tracker malfunction were removed (roughly 15.6 % of the overall data with roughly equal amounts of data loss across blocks and conditions). The relevant period of missing data was not included in the averaging. Pretrial baseline responses were computed as the average pupil diameter during the fixation screen (2,000 ms). Pretrial baselines were z -scored normalized within each participant to correct for individual differences in pupil diameter. TEPRs were corrected by subtracting out the last 200 ms of the wait time and locked to when the numbers began counting up on a trial-by-trial basis for each participant. To examine the time course of the TEPRs, the pupil data were averaged into a series of 20 ms time windows following stimulus onset for each trial.
Behavioral Results
Reaction time (rt).
First we examined time on task effects. RTs were grouped into five blocks of 32 trials each. Consistent with prior research a classic vigilance decrement was observed such that as time on task increased so did reaction times (see Figure 2a ). There was a main effect of block, F (4, 144) = 26.83, MSE = 480.4, p < .01, partial η 2 = .43, and a significant linear trend, F (1, 36) = 54.30, MSE = 945.89, p < .01, partial η 2 = .60. Additionally, as might be expected the mean standard deviation of the RTs also increased across blocks (see Figure 2b ), suggesting that not only did participants get slower across blocks but they also became more variable in their responding. There was a main effect of block, F (4, 144) = 8.50, MSE = 747.3, p < .01, partial η 2 = .19, and a significant linear trend, F (1, 36) = 26.68, MSE = 934.92, p < .01, partial η 2 = .43. Note that we also computed coefficient of variation across blocks to see if variability increased after taking into account differences in the mean. Similar to the analysis on mean standard deviations, mean coefficient of variation increased across blocks, F (4, 144) = 5.73, MSE = .004, p < .01, partial η 2 = .14. Next, we examined whether lapses of attention would increase with time on task. Lapses of attention were defined as the slowest 20 % of reaction times. Specifically, we ranked ordered each participant’s RTs from fastest to slowest and placed into quintiles. The slowest quintile was taken as lapses. This measure has been used previously in many prior individual differences studies to examine how lapses of attention correlate with other cognitive abilities (e.g., Unsworth et al., 2010 ). As shown in Figure 2c , the number of lapses of attention steadily increased with time on task, with most lapses occurring in the final block. There was a main effect of block, F (4, 144) = 58.34, MSE = 6.03, p < .01, partial η 2 = .62, and a significant linear trend, F (1, 36) = 103.63, MSE = 10.55, p < .01, partial η 2 = .74. Thus, consistent with previous work in sustained attention and vigilance (Kribbs & Dinges, 1994 ; Parasuraman, 1986 ; Unsworth et al., 2010 ), the current results suggested that individuals became slower, more variable, and experienced more lapses of attention as time on the task increased.
a Mean reaction time (RT) as a function of block. b Mean standard deviation of reaction time as a function of block. c Mean number of lapses of attention as a function of block. Error bars reflect 1 standard error of the mean
Next, we examined the thought-probe responses. There was a significant effect of thought probe response, F (4, 144) = 4.59, MSE = .054, p < .01, partial η 2 = .12. Shown in Table 1 are the proportions of each thought-probe response. As can be seen, participants spent much of their time either focused on the task or thinking about their performance on the task (task-related interference). At the same time, there was considerable off-task thinking with participants reporting that they experienced external distraction, mind-wandering, and inattentiveness.
As shown in Figure 3a , examining the thought probes responses by block suggested a Block × Thought Probe response interaction, F (4, 144) = 4.99, MSE = 1.88, p < .01, partial η 2 = .12, such that on-task reports tended to decrease across blocks (linear trend, p = .012, partial η 2 = .16), but reports of mind-wandering (linear trend, p < .01, partial η 2 = .32) and inattentiveness (linear trend, p < .01, partial η 2 = .37) tended to increase across blocks. External distraction tended to increase across blocks as well (linear trend, p = .017, partial η 2 = .15). Task-related interference tended to remain fairly constant across blocks (linear trend, p = .23, partial η 2 = .04). Thus, as time on task increased, participants reported being less focused on the task and reported more off-task thoughts consistent with prior research (e.g., McVay & Kane, 2012a ; Thomson, Seli, Besner, & Smilek, 2014 ).
a Number of thought-probe responses as a function of attentional state and block. b Mean reaction time as a function of attentional state. Error bars reflect 1 standard error of the mean. Note. On-task = on-task thoughts; TRI = task-related interference; ED = external distraction; MW = mind-wandering; Inattn = inattentive/nonalert
Examining RTs for the different responses suggested that the different off-task reports were associated with much slower RTs than on-task reports, t = 9.24, p < .001 ( b = 17.75, SE = 1.92). Note, because not all participants reported all of the attentional states, we used linear mixed models to analyze the data. Linear mixed models are an extension of the general linear model in which both fixed and random effects are included. Thus, they are similar to mixed analysis of variance (ANOVA) but offer advantages over traditional mixed ANOVAs in terms of more power and the ability to handle unbalanced designs and missing data (e.g., Kliegl, Wei, Dambacher, Yan, & Zhou, 2011 ). In the model, attentional state was entered as a fixed factor, and subjects were entered as random factors. Note, there were 28 participants contributing to the on-task category, 34 contributing to task-related interference, 26 to external distraction, 31 to mind-wandering, and 23 to the inattentive category. Examining attentional state as fixed factor suggested differences in RTs across the different states, t = 9.10, p < .001 ( b = 8.77, SE =.93). Specifically, as shown in Figure 3b , comparing trials associated with each off-task thought report to on-task thought reports in separate linear mixed models suggested that off-task reports were 40 to 70 ms slower than trials where participants reported being fully on task (all p s < .01). Thus, when participants reported being distracted, mind-wandering, or being inattentive, performance was slower than when they reported being on task.
Pupil Diameter
Next, we turn to our primary analyses of interest, examining pupil size. As noted previously, pupil diameter was measured continuously throughout the task. Therefore, both pretrial baseline and TEPRs were examined.
Pretrial Pupil Diameter
First, we examined time-on-task effects for pretrial pupil diameter. As shown in Figure 4a , pretrial pupil diameter decreased throughout the task, with the largest decrease happening from Block 1 to Block 2. There was a main effect of block, F (4, 144) = 4.08, MSE = .12, p < .01, partial η 2 = .10, and a significant linear trend, F (1, 36) = 4.88, MSE = .20, p = .034, partial η 2 = .34. This is consistent with prior research, which has shown a vigilance decrement in baseline pupil diameter (Fried et al., 2014 ; but see Beatty 1982b ). Additionally, as seen in Figure 4b , pretrial baseline pupil diameter tended to become more variable with time on task. There was a main effect of block, F (4, 140) = 3.75, MSE = .04, p < .01, partial η 2 = .10, and a significant linear trend, F (1, 35) = 9.30, MSE = .01, p < .01, partial η 2 = .21. Overall, the decrease in pupil diameter and the increase in pupil variability are consistent with increases in pupillary unrest as time on task increases, suggesting that as time on task increased participants alertness and arousal decreased (Morad et al., 2000 ; Wilhelm et al., 2001 ).
a Normalized mean pretrial baseline pupil diameter as a function of block. b Mean standard deviation of normalized pretrial baseline pupil diameter. Error bars reflect one standard error of the mean
Next, we examined pretrial pupil diameter for lapse and nonlapse trials. Specifically, we examined the normalized pretrial baseline pupil diameter for trials classified as a lapse (20 % slowest trails) compared to nonlapse trials (average of the other 80 % of trials). As shown in Figure 5a , pretrial baseline pupil diameter was slightly larger for trials that experienced a subsequent lapse of attention compared to nonlapse trials, t (36) = 2.73, p = .01, d = .45. Similar results were obtained when comparing quintile 5 to each of the other quintiles separately (all p s < .046). Thus, when participants had a long RT, these trials were preceded by larger than normal pretrial pupil diameters, consistent with the notion that participants were experiencing task disengagement (Gilzenrat et al., 2010 ).
Normalized mean pre-trial baseline pupil diameter as a function of non-lapse or lapse trials. Error bars reflect one standard error of the mean
Turning to one of the most important analyses, examining pretrial baseline pupil diameter as a function of attentional state suggested clear differences. Again because not everyone reported every attentional state, we used mixed models to analyze the data. Attentional state was entered as a fixed factor, and subjects were entered as random factors. First, examining on-task reports versus off-task reports (i.e., combining external distraction, mind-wandering, and inattentive) suggested that on-task reports ( M = .06, SE = .06) were associated with larger pretrial baselines than were off-task reports ( M = -.08, SE = .04), t = -1.97, p = .049 ( b = -.14, SE = .07). This replicates prior work by Grandchamp et al. ( 2014 ) and Mittner et al. ( 2014 ) but is inconsistent with Franklin et al. ( 2013 ). Perhaps the differences are due to differences in the types of lapses that can be considered as off task. To examine this, we analyzed pretrial baseline pupil diameter as a function of the different attentional states. The analysis suggested a main effect of attentional state, t = -3.21, p < .001 ( b = -.06, SE = .02). Footnote 1 As shown in Fig. 6a and b , pretrial baseline pupil diameter clearly distinguished the different forms of off-task thought from one another and from on-task thoughts. Specifically, when participants reported being externally distracted, their baseline pupil diameters were much larger than normal (i.e., compared to the overall average baseline pupil diameter; p < .01), and tended to be larger than when participants reported being on task ( p = .059). When participants reported either mind-wandering or being inattentive, their baseline pupil diameters were much smaller than the overall average baseline pupil diameter and much smaller than when participants reported being on task (both p s < .01). Furthermore, both mind-wandering and inattentive reports were associated with smaller pretrial baseline pupil diameters than reports of external distraction (both p s < .01), but pretrial pupil diameter for mind-wandering and inattentive reports were not different ( p = .55). These results demonstrate that different types of lapses of attention are associated with different pretrial baseline pupil levels and further suggest that these lapses are indexing different parts of the LC-NE curve. Footnote 2
a Normalized mean pretrial pupil diameter as a function of attentional state. b Normalized mean pretrial pupil diameter as a function of attentional state and time. Error bars reflect 1 standard error of the mean. Note. On-task = on-task thoughts; TRI = task-related interference; ED = external distraction; MW = mind-wandering; Inattn = inattentive/nonalert
Task-Evoked Pupillary Response
Our final set of analyses focused on TEPRs to see if the TEPRs would decrease with time on task consistent with the decrease seen in pretrial baseline and consistent with prior research (e.g., Beatty, 1982b ). The TEPRs were corrected by subtracting out pupil diameter from the last 200 ms of the wait time and locked to when the numbers began counting up. Across all trials and participants there were a minimum of 700 data points per bin. Shown in Figure 7 are the TEPRs as a function of block. Note that the pupil waveforms are used mainly for visualization, whereas the dependent measure in the analysis is the peak task-evoked response. There was a clear reduction in the peak task-evoked response as a function of block. There was a main effect of block, F (4, 140) = 12.25, MSE = .001, p < .01, partial η 2 = .26, and a significant linear trend, F (1, 35) = 33.06, MSE = .001, p < .01, partial η 2 = .49. Thus, consistent with prior research, TEPRs decreased with time on task (Beatty, 1982b ).
Task-evoked pupillary responses as a function of block
a Grand averaged task-evoked pupillary responses as a function of attentional state. b Peak response as a function of attentional state. Error bars reflect 1 standard error of the mean. Note. On-task = on-task thoughts; TRI = task-related interference; ED = external distraction; MW = mind-wandering; Inattn = inattentive/nonalert
Next, we examined whether TEPRs would change as a function of attentional state. Specifically, on-task responses should be associated with larger phasic responses than the various off-task responses. As shown in Figure 8a , this was indeed the case, with the different off-task responses exhibiting smaller phasic responses than on-task reports. Similar to the block phasic response, the TEPRs were corrected by subtracting out pupil diameter from the last 200 ms of the wait time and locked to when the numbers began counting up. As shown in Figure 8b , the peak response differed as a function of attentional state, t = -2.67, p = .008 ( b = -.01, SE = .003). Specifically, when comparing TEPRs for on-task reports vs. off-task reports (i.e., combining external distraction, mind-wandering, and inattentive), it was suggested that on-task reports were associated with larger phasic responses than off-task reports, t = -2.66, p = .008 ( b = -.03, SE = .01). To examine these further, separate linear mixed models comparing each off-task response to on-task responses were done. The difference between on-task and off-task reports was mainly driven by differences between on-task responses and mind-wandering ( p = .012) and inattentiveness ( p = .044). The effect did not reach significance for external distraction ( p = .14), given larger variability associated with fewer responses. Additionally, there did not seem to be a difference between TEPRs for on-task and task-related interference ( p = .14). Overall, these results are consistent with the notion that unfocused attentional states are associated with reduced TEPRs.
In the current study, we examined whether pupillary responses (both pretrial and phasic) could be used to distinguish between different attentional states. During a sustained-attention task, we found that when participants reported being off task they had smaller than normal pretrial baseline pupil diameters and smaller than normal phasic responses to the stimuli. These results are consistent with some prior research that has similarly shown that when participants are off task both baseline and phasic responses are smaller (e.g., Grandchamp et al., 2014 ; Mittner et al., 2014 ). However, the results are inconsistent with prior research by Franklin et al. ( 2013 ), who showed that when participants are off task they have larger baseline pupil diameters than when they report being on task. One potential difference between these studies is the tasks used. Both in the current study and in Mittner et al. ( 2014 ), participants performed a challenging attention-control task (here, the psychomotor vigilance task and in Mittner et al. a stop-signal task), whereas in Franklin et al. ( 2013 ) participants read a text. Thus, differences may arise due to differences in how challenging the tasks are, with participants in the attention-control tasks being required to consistently maintain attention on task whereas during a self-paced reading task there may be more opportunities to intentionally disengage from the task. That is, with the self-paced reading task it is possible to take a break and intentionally disengage from the task, resulting in more exploratory behavior thought to be associated with higher LC-NE tonic activity. In the more attention-demanding tasks, it is necessary to consistently maintain attention on the task given the fast-paced nature of the trials. In these tasks any intentional disengagement can result in much poorer performance. At the same time, given the demand to sustain attention on these tasks, it is possible that as time on task increases, overall arousal decreases, leading to fatigue and lowered alertness. Thus, the different types of tasks may encourage different attentional states associated with different pupillary responses.
Consistent with theories of LC-NE functioning, it is possible that some lapses of attention are a result of lowered arousal and alertness linked to the lower portion of the LC-NE curve. These lapses should be linked with poorer behavioral performance, small baseline pupil diameters, and small phasic responses. As suggested by Lenartowicz et al. ( 2013 ), depending on whether attention is focused internally or externally, lowered arousal levels can be associated with either mind-wander or external distraction. Additionally, it is possible that other lapses of attention are a result of heightened arousal linked with the upper portion of the LC-NE curve. These lapses should also be linked with poorer behavioral performance and small phasic responses, but, critically, these lapses should be linked with larger than normal baseline pupil diameters. Again, as suggested by Lenartowicz et al. ( 2013 ), depending on whether attention is focused internally or externally, heightened arousal levels can be associated with different types of mind-wandering and external distraction. The current results are very much in line with this hypothesis. Specifically, we found that when participants reported being inattentive or mind-wandering, their RTs were longer, their pretrial baseline pupil diameters were smaller, and their phasic responses were smaller than when they reported being on task. Additionally, when participants reported being distracted, their RTs were longer, their pretrial baseline pupil diameters were larger, and their phasic responses were (nonsignificantly) smaller than when they reported being on task. Consistent with recent theorizing, these results strongly suggest that there are different flavors of lapses of attention with each being linked with different modes of LC-NE functioning as indicated by differences in the pupillary responses. These results demonstrate that fluctuations in attentional state can be tracked with changes in pupil dilations and further suggest that changes in pupil dilation can distinguish different types of lapses of attention.
At the same time, the current results suggest that there is likely not a simple one-to-one correspondence between different attentional states and pupil dilation. Rather, depending on the current context, task, and individual, it is likely that different attentional states will be associated with either high or low pupil diameters. It is likely that there is a complex interaction of within- and between-subjects effects. Some participants may have a propensity to shift to high arousal (anxious/stressed), some may shift to low arousal (inattentive/bored), and still others may shift between different states more readily (more variable overall). Furthermore, these effects may also depend on where you are at on the arousal function to begin with. Thus, additional research is needed to better examine the relation between pupillary responses and attentional states, perhaps via experimental manipulations that force or encourage certain states (anxious, bored, exploratory, etc.). Additionally, future research is needed to better delineate different types of mind-wandering and external distraction as a function of arousal level. One problem with the current study is that we relied on very broad categories of attentional state via the thought probes. This makes it difficult to distinguish between different types of attentional states (i.e., mind-wandering when bored vs. mind-wandering when anxious). Future research should rely on more fine-grained probes to better capture the different varieties of lapse of attention and subjective attentional states.
Examining time-on-task effects, we found traditional behavioral effects with average RTs increasing and RT variability increasing with time on task. Furthermore, lapses of attention increased substantially with time on task. We also found that, as time on task increased, on-task reports decreased, while reports of inattention and mind-wandering increased. Thus, as time on task increased, alertness decreased and fluctuations in attention increased, resulting in participants being less able to consistently sustain attention on the task. In terms of pupillary responses, we found that as time on task increased, pretrial baseline pupil diameter decreased and variability in pretrial baseline pupil diameter increased. These results are consistent with prior work on pupillary unrest in which, as alertness and arousal decrease and participants become fatigued, the pupil tends to decrease in size with increasing variability (Morad et al., 2000 ; Wilhelm et al., 2001 ). Consistent with prior work, we also found that lapses of attention were associated with larger than normal pretrial baseline pupils on average, suggesting that as time on task increased, participants periodically disengaged from the task (Gilzenrat et al., 2010 ). Thus, time on task led to overall lowered alertness levels and more fluctuations between the different LC-NE modes. Finally, examining phasic pupillary responses, we found that as time on task increased, phasic pupillary responses decreased (Beatty, 1982b ).
Collectively, the current results are consistent with prior LC-NE research, which has shown that during periods of lowered vigilance, tonic LC activity is reduced and phasic LC responses are diminished, leading to poorer behavioral performance consistent with lapses of attention (Aston-Jones et al., 1994 ; Aston-Jones, Iba, Clayton, Rajkowski, & Cohen, 2007 ). That is, as time on task increases, alertness and arousal decrease, making it increasingly difficult to sustain attention on task. With these decreases in arousal and alertness, attention fluctuates widely between different states. Subjectively, this results in participants reporting more inattention, mind-wandering, and distraction, as it is increasingly difficult to maintain focus on the current task and prevents thoughts from drifting to unrelated matters. These increases in lapses of attention lead to very slow and to more inconsistency in reaction times. Importantly the current results suggest that pupillary changes accurately track these changes in fluctuations in attentional state in a manner consistent with prior LC-NE theorizing (e.g., Aston-Jones & Cohen, 2005 ; Gilzenrat et al., 2010 ; Jepma & Nieuwenhuis, 2011 ; Murphy, Robertson, Balsters, & O’Connell, 2011 ). As such, the current results provide important information—not only on changes in sustained attention as time on task increases but also on the notion that there are different flavors of lapses of attention associated with different pupillary responses, theoretically due to different modes of LC-NE system. The current results not only inform theories of sustained attention and lapses of attention but also provide an important tool for tracking and distinguishing various types of lapses of attention. Thus, pupillometry provides the potential for an online unobtrusive and objective marker of fluctuations in attentional state that can be used to predict when lapses of attention are about to occur and potentially intervene and interrupt or correct those lapses. Future work is needed to further examine the extent to which pupillary responses accurately track different types of lapses of attention in a variety of situations and contexts and to examine the feasibility of using pupillometry as an online marker of fluctuations in attentional state.
It is possible that differences in pupil size across the different attentional states are due to eye movements. Eye movements were defined as any movements that deviated from center fixation by more than 20 %. There were no differences across the attentional states in terms of number of eye movements ( p = .79), average eye position ( p = .68), or absolute distance from fixation ( p = .80) given that most of the time participants were staring in the center of the screen where the stimuli were presented.
Given that there were also effects of block on pretrial baseline pupil and given that off-task thought reports increased as a function of block, we examined whether the differences in attentional state would remain after including block into the model as a covariate. The main effect of attentional state remained ( t = -2.76, p = .004), and the interaction between attentional state and block was not quite significant ( t = -1.66, p = .097) suggesting that although pretrial pupil diameter changed as a function of attentional state and time on task (see discussion), the effect of attentional state was not exclusively mediated by time-on-task effects.
Alnaes, D., Sneve, M. H., Espeseth, T., Endestad, T., van de Pavert, S. H. P., & Laeng, B. (2014). Pupil size signals mental effort deployed during multiple object tracking and predicts brain activity in the dorsal attention network and the locus coeruleus. Journal of Vision, 14, 1–20.
Article PubMed Google Scholar
Aston-Jones, G., & Cohen, J. D. (2005). An integrative theory of locus coeruleus–norepinephrine function: Adaptive gain and optimal performance. Annual Review of Neuroscience, 28, 403–450.
Aston-Jones. G., Rajkowski, J., Kubiak, P., Alexinsky, T. (1994). Locus coeruleus neurons in the monkey are selectively activated by attended stimuli in a vigilance task. The Journal of Neuroscience, 14 , 4467–4480.
Aston-Jones, G. S., Iba, M., Clayton, E., Rajkowski, J., & Cohen, J. (2007). The locus coeruleus and regulation of behavioral flexibility and attention: Clinical implications. In G. A. Ordway, M. A. Schwartz, & A. Frazer (Eds.), Brain norepinephrine: Neurobiology and therapeutics (pp. 196–235). Cambridge: Cambridge University Press.
Chapter Google Scholar
Beatty, J. (1982a). Task-evoked pupillary responses, processing load, and the structure of processing resources. Psychological Bulletin, 91, 276–292.
Beatty, J. (1982b). Phasic not tonic pupillary responses vary with auditory vigilance performance. Psychophysiology, 19, 167–172.
Beatty, J., & Lucero-Wagoner, B. (2000). The pupillary system. In J. T. Cacioppo, L. G. Tassinary, & G. G. Berntson (Eds.), Handbook of psychophysiology (pp. 142–162). New York: Cambridge University Press.
Google Scholar
Berridge, C. W., & Waterhouse, B. D. (2003). The locus coeruleus-noradrenergic system: Modulation of behavioral state and state-dependent cognitive processes. Brain Research Reviews, 42, 33–84.
Cohen, J. D., Aston-Jones, G., & Gilzenrat, M. S. (2004). A systems-level perspective on attention and cognitive control: Guided activation, adaptive gating, conflict monitoring, and exploitation vs. exploration. In M. I. Posner (Ed.), Cognitive neuroscience of attention (pp. 71–90). New York: Guilford Press.
Dinges, D. F., & Powell, J. W. (1985). Microcomputer analyses of performance on a portable, simple visual RT task during sustained operations. Behavior Research Methods, Instruments, & Computers, 17, 652–655.
Article Google Scholar
Dorrian, J., Rogers, N. L., & Dinges, D. F. (2005). Psychomotor vigilance performance: A neurocognitive assay sensitive to sleep loss. In C. Kushida (Ed.), Sleep deprivation: Clinical issues, pharmacology and sleep loss effects . New York: Marcel Dekker.
Drummond, S. P. A., Bischoff-Grethe, A., Dinges, D. F., Ayalon, L., Mednick, S. C., & Meloy, M. J. (2005). The neural basis of the psychomotor vigilance task. Sleep, 28, 1059–1068.
PubMed Google Scholar
Duncan, J. (1995). Attention, intelligence, and the frontal lobes. In M. Gazzaniga (Ed.), The cognitive neurosciences (pp. 721–733). Cambridge: MIT Press.
Franklin, M. S., Broadway, J. M., Mrazek, M. D., Smallwood, J., & Schooler, J. W. (2013). Window to the wandering mind: Pupillometry of spontaneous thought while reading. The Quarterly Journal of Experimental Psychology, 1–15.
Fried, M., Tsitsiashvili, E., Bonney, Y. S., Sterkin, A., Wygnanski-Jaffe, T., Epstein, T., & Polat, U. (2014). ADHD subjects fail to suppress eye blinks and mircosaccades while anticipating visual stimuli but recover with medication. Vision Research, 101, 62–72.
Gilzenrat, M. S., Nieuwenhuis, S., Jepma, M., & Cohen, J. D. (2010). Pupil diameter tracks changes in control state predicted by the adaptive gain theory of locus coeruleus function. Cognitive, Affective, & Behavioral Neuroscience, 10, 252–269.
Grandchamp, R., Braboszcz, C., & Delorme, A. (2014). Occulometric variations during mind wandering. Frontiers in Psychology, 5, 31.
Article PubMed PubMed Central Google Scholar
Hess, E. H., & Polt, J. M. (1964). Pupil size in relation to mental activity during simple problem-solving. Science, 143, 1190–1192.
Hou, R. H., Freeman, C., Langley, R. W., Szabadi, E., & Bradshaw, C. M. (2005). Does modafinil activate the locus coeruleus in man? Comparison of modafinil and clonidine on arousal and autonomic functions in human volunteers. Psychopharmacology, 181, 537–549.
Jepma, M., & Nieuwenhuis, S. (2011). Pupil diameter predicts changes in the exploration-exploitation trade-off: Evidence for the adaptive gain theory. Journal of Cognitive Neuroscience, 23, 1587–1596.
Joshi, S., Li, Y., Kalwani, R. M., & Gold, J. I. (2016). Relationship between pupil diameter and neuronal activity in the locus coeruleus, colliculi, and cingulate cortex. Neuron. doi: 10.1016/j.neuron.2015.11.028 .
Kahneman, D. (1973). Attention and effort . Upper Saddle River: Prentice Hall.
Kahneman, D., & Beatty, J. (1966). Pupil diameter and load on memory. Science, 154, 1583–1585.
Kang, O. E., Huffer, K. E., & Wheatley, T. P. (2014). Pupil dilation dynamics track attention to high-level information. PLOS ONE, 9 , e102463.
Kliegl, R., Wei, P., Dambacher, M., Yan, M., & Zhou, X. (2011). Experimental effects and individual differences in Linear Mixed Models: Estimating the relationship between spatial, object, and attraction effects in visual attention. Frontiers in Psychology, 1, 238.
PubMed PubMed Central Google Scholar
Kribbs, N. B., & Dinges, D. (1994). Vigilance decrements and sleepiness. In R. D. Ogilvie & J. R. Harsh (Eds.), Sleep onset: Normal and abnormal processes . Washington, DC: American Psychological Association Press.
Kristjansson, S. D., Stern, J. A., Brown, T. B., & Rohrbaugh, J. W. (2009). Detecting phasic lapses of alterness using pupillometric measures. Applied Ergonomics, 40, 978–986.
Lenartowicz, A., Simpson, G. V., & Cohen, M. S. (2013). Perspective: Causes and functional significance of temporal variations in attention control. Frontiers in Human Neuroscience, 7, 381.
McVay, J. C., & Kane, M. J. (2012a). Drifting from slow to “D’oh!” Working memory capacity and mind wandering predict extreme reaction times and executive-control errors. Journal of Experimental Psychology: Learning, Memory, and Cognition, 38, 525–549.
McVay, J. C., & Kane, M. J. (2012b). Why does working memory capacity predict variation in reading comprehension? On the influence of mind wandering and executive attention. Journal of Experimental Psychology: General, 141, 302–320.
Miller, E. K., & Cohen, J. D. (2001). An integrative theory of prefrontal cortex functioning. Annual Review of Neuroscience, 24, 167–202.
Mittner, M., Boekel, W., Tucker, A., Turner, B. M., Heathcote, A., & Forstmann, B. U. (2014). When the brain takes a break: A model-based analysis of mind wandering. The Journal of Neuroscience, 34, 16286–16295.
Morad, Y., Lemberg, H., Yofe, N., & Dagan, Y. (2000). Pupillography as an objective indicator of fatigue. Current Eye Research, 21, 535–542.
Murphy, P. R., O’Connell, R. G., O’Sullivan, M., Robertson, I. H., & Balsters, J. H. (2014). Pupil diameter covaries with BOLD activity in human locus coeruleus. Human Brain Mapping, 35, 4140–4154.
Murphy, P. R., Robertson, I. H., Balsters, J. H., & O’Connell, R. G. (2011). Pupillometry and P3 index the locus coeruleus-noradrenergic arousal function in humans. Psychophysiology, 48, 1532–1543.
Nieuwenhuis, S., de Geus, E. J., & Aston-Jones, G. (2011). The anatomical and functional relationship between the P3 and autonomic components of the orienting response. Psychophysiology, 48, 162–175.
Parasuraman, R. (1986). Vigilance, monitoring, and search. Handbook of perception and human performance. Vol. 2: Cognitive processes and performance . New York: Wiley.
Rajkowski, J., Kubiak, P., & Aston-Jones, G. (1993). Correlations between locus coeruleus (LC) neural activity, pupil diameter and behavior in monkey support a role of LC in attention. Society for Neuroscience Abstracts, 19, 974.
Reason, J. T. (1984). Lapses of attention in everyday life. In R. Parasuraman & D. R. Davies (Eds.), Varieties of attention . Orlando: Academic Press.
Reason, J. T. (1990). Human error . Cambridge: Cambridge University Press.
Book Google Scholar
Roberts, R. J., & Pennington, B. F. (1996). An integrative framework for examining prefrontal cognitive processes. Developmental Neuropsychology, 12, 105–126.
Schooler, J. W., Reichle, E. D., & Halpern, D. V. (2005). Zoning out while reading: Evidence for dissociations between experience and metaconsciousness. In D. Levin (Ed.), Thinking and seeing: Visual metacognition in adults and children (pp. 203–226). Cambridge: MIT Press.
Smallwood, J., Brown, K. S., Baird, B., Mrazek, M. D., Franklin, M. S., & Schooler, J. W. (2012). Insulation for daydreams: A role for tonic norepinephrine in the facilitation of internally guided thought. PLOS ONE, 7 , e33706.
Smallwood, J., Brown, K. S., Tipper, C., Giesbrecht, B., Franklin, M. S., Mrazek, M. D., … Schooler, J. W. (2011). Pupillometric evidence for the decoupling of attention from perceptual input during offline thought. PLOS ONE, 6, e18298.
Smallwood, J., & Schooler, J. W. (2006). The restless mind. Psychological Bulletin, 132, 946–958.
Stawarczyk, D., Majerus, S., Maj, M., Van der Linden, M., & D’Argembeau, A. (2011). Mind-wandering: Phenomenology and function as assessed with a novel experience sampling method. Acta Psychologica, 136, 370–381.
Thomson, D. R., Seli, P., Besner, D., & Smilek, D. (2014). On the link between mind-wandering and task performance over time. Consciousness & Cognition, 27, 14–26.
Unsworth, N., & McMillan, B. D. (2014a). Similarities and differences between mind-wandering and external distraction: A latent variable analysis of lapses of attention and their relation to cognitive abilities. Acta Psychologica, 150, 14–25.
Unsworth, N., & McMillan, B. D. (2014b). Trial-to-trial fluctuations in attentional state and their relation to intelligence. Journal of Experimental Psychology: Learning, Memory, & Cognition, 40, 882–891.
Unsworth, N., McMillan, B. D., Brewer, G. A., & Spillers, G. J. (2012). Everyday attention failures: An individual differences investigation. Journal of Experimental Psychology: Learning, Memory, & Cognition, 38, 1765–1772.
Unsworth, N., Redick, T. S., Lakey, C. E., & Young, D. L. (2010). Lapses in sustained attention and their relation to executive and fluid abilities: An individual differences investigation. Intelligence, 38, 111–122.
Unsworth, N., & Robison, M. K. (2015). Individual differences in the allocation of attention to items in working memory: Evidence from pupillometry. Psychonomic Bulletin & Review, 3, 757–765.
Unsworth, N., Schrock, J. C., & Engle, R. W. (2004). Working memory capacity and the antisaccade task: Individual differences in voluntary saccade control. Journal of Experimental Psychology: Learning, Memory, and Cognition, 30, 1302–1321.
Usher, M., Cohen, J. D., Servan-Schreiber, D., Rajkowski, J., & Aston-Jones, G. (1999). The role of locus coeruleus in the regulation of cognitive performance. Science, 283, 549–554.
Ward, A. F., & Wegner, D. M. (2013). Mind-blanking: When the mind goes away. Frontiers in Psychology, 4, 650.
Wilhelm, B., Giedke, H., Ludtke, H., Bittner, E., Hofmann, A., & Wilhelm, H. (2001). Daytime variations in central nervous system activation measured by a pupillographic sleepiness test. Journal of Sleep Research, 10, 1–7.
Yerkes, R. M., & Dodson, J. D. (1908). The relation of strength of stimulus to rapidity of habit-formation. Journal of Comparative Neurology and Psychology, 18, 459–482.
Download references
Author information
Authors and affiliations.
Department of Psychology, University of Oregon, Eugene, OR, 97403, USA
Nash Unsworth & Matthew K. Robison
You can also search for this author in PubMed Google Scholar
Corresponding author
Correspondence to Nash Unsworth .
Rights and permissions
Reprints and permissions
About this article
Unsworth, N., Robison, M.K. Pupillary correlates of lapses of sustained attention. Cogn Affect Behav Neurosci 16 , 601–615 (2016). https://doi.org/10.3758/s13415-016-0417-4
Download citation
Published : 01 April 2016
Issue Date : August 2016
DOI : https://doi.org/10.3758/s13415-016-0417-4
Share this article
Anyone you share the following link with will be able to read this content:
Sorry, a shareable link is not currently available for this article.
Provided by the Springer Nature SharedIt content-sharing initiative
- Cognitive control
- Norephinephrine
- Find a journal
- Publish with us
- Track your research
- Print this article
Research Article
Tracking the dynamics of mind wandering: insights from pupillometry.
- Claudia Pelagatti
- Paola Binda
Both Dr. Paola Binda, PhD, and Dr. Manila Vannucci, PhD, are corresponding authors for this article. They can both be contacted here .
Mind wandering (MW) refers to the shift of attention away from a primary task towards thoughts unrelated to the task. Here we show that significant new insight into the dynamics of this process can be gained by using pupillometry. Participants performed a monotonous vigilance task which was intermixed with task-irrelevant verbal cues. At fixed times, we interrupted them and asked what they were thinking about immediately prior to the probe and what had triggered their thought. We observed pupil dilation after the presentation of verbal cues reported to have triggered MW, compared with other verbal cues with similar emotional content. Thus, MW is associated with pupil dilation. We also analysed the pupil-constriction response to the task-stimuli (vertical and horizontal bars, to be categorized as targets and non-targets for the vigilance task), and found that this was unchanged during MW. We conclude that pupil size provides an index of MW, objective and covert and that this may be exploited in further studies to understand whether and how MW affects the processing of sensory stimuli.
- Page/Article: 38
- DOI: 10.5334/joc.41
- Accepted on 12 Jul 2018
- Published on 19 Jul 2018
- Peer Reviewed
Thank you for visiting nature.com. You are using a browser version with limited support for CSS. To obtain the best experience, we recommend you use a more up to date browser (or turn off compatibility mode in Internet Explorer). In the meantime, to ensure continued support, we are displaying the site without styles and JavaScript.
- View all journals
- My Account Login
- Explore content
- About the journal
- Publish with us
- Sign up for alerts
- Open access
- Published: 15 August 2017
Pupil Size Tracks Attentional Performance In Attention-Deficit/Hyperactivity Disorder
- G. Wainstein 1 na1 ,
- D. Rojas-Líbano ORCID: orcid.org/0000-0002-9517-5545 1 , 2 na1 ,
- N. A. Crossley 1 , 3 ,
- X. Carrasco 4 ,
- F. Aboitiz 1 &
- T. Ossandón 1
Scientific Reports volume 7 , Article number: 8228 ( 2017 ) Cite this article
30k Accesses
65 Citations
6 Altmetric
Metrics details
- Diagnostic markers
Attention-deficit/hyperactivity disorder (ADHD) diagnosis is based on reported symptoms, which carries the potential risk of over- or under-diagnosis. A biological marker that helps to objectively define the disorder, providing information about its pathophysiology, is needed. A promising marker of cognitive states in humans is pupil size, which reflects the activity of an ‘arousal’ network, related to the norepinephrine system. We monitored pupil size from ADHD and control subjects, during a visuo-spatial working memory task. A sub group of ADHD children performed the task twice, with and without methylphenidate, a norepinephrine–dopamine reuptake inhibitor. Off-medication patients showed a decreased pupil diameter during the task. This difference was no longer present when patients were on-medication. Pupil size correlated with the subjects’ performance and reaction time variability, two vastly studied indicators of attention. Furthermore, this effect was modulated by medication. Through pupil size, we provide evidence of an involvement of the noradrenergic system during an attentional task. Our results suggest that pupil size could serve as a biomarker in ADHD.
Similar content being viewed by others
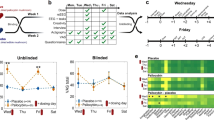
Microdosing with psilocybin mushrooms: a double-blind placebo-controlled study
Federico Cavanna, Stephanie Muller, … Enzo Tagliazucchi
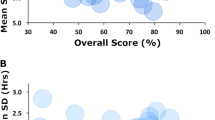
Sleep quality, duration, and consistency are associated with better academic performance in college students
Kana Okano, Jakub R. Kaczmarzyk, … Jeffrey C. Grossman
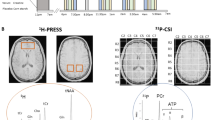
Single dose creatine improves cognitive performance and induces changes in cerebral high energy phosphates during sleep deprivation
Ali Gordji-Nejad, Andreas Matusch, … Andreas Bauer
Introduction
Attention-deficit/hyperactivity disorder (ADHD) is the most prevalent childhood neuropsychiatric disorder 1 , 2 . This condition is characterized by inattention, impulsiveness and hyperactivity, and is becoming increasingly recognized that many patients continue having these types of difficulties in adulthood 3 . Most of ADHD symptoms are related to problems in behavioral and cognitive control, and have been attributed to a deficient dopaminergic signaling 2 , 4 , 5 . First-line treatment for ADHD is given by stimulants, mainly methylphenidate, and the usual second-line treatment is atomoxetine, a noradrenaline reuptake inhibitor 2 , 6 . Both kinds of drugs increase the catecholamine availability at synapses. This, together with evidence of a weak but consistent link between genetic polymorphisms associated to the catecholaminergic system and ADHD, prompted the widely accepted hypothesis that in this condition there is an underlying deficit in catecholaminergic neurotransmission 1 , 7 .
Currently, diagnosis is being performed solely on the basis of observed behavior and reported symptoms, which carries the potential risk of over-diagnosis or under-diagnosis 6 , 8 . Like any complex disorder, ADHD patients display clear heterogeneities at the clinical and biological levels. These differences probably explain why some patients do not respond to medication 6 , 7 , 8 , 9 . A biological marker that helps to objectively define the disorder, providing information about its pathophysiology and potential responses to medication, is needed 9 , 10 .
A promising potential marker of cognitive states in humans is pupil size 11 , 12 , 13 , 14 , 15 . After controlling for stimulus luminance, pupil size correlates with task difficulty, emotional valence, physical effort, motor output and arousal states 11 , 16 , 17 , 18 , 19 , 20 , 21 . Independent findings suggest that these fluctuations in pupil size reflect the state of the brain norepinephrine (NE) system 11 , 22 , 23 , 24 , 25 , 26 . This system originates in the locus-coeruleus (LC) and projects throughout the cerebral cortex, hippocampus, thalamus and midbrain, among others 22 , 23 , 24 , 27 , 28 , 29 . Brain areas associated with attentional processing (e.g., parietal cortex, pulvinar nucleus, superior colliculus) receive particularly dense LC-NE innervations 12 , 25 . Data from animal models, both rodents and non-human primates, have shown a central role for the LC in selective attention 11 , 23 , 24 , 25 .
Nowadays, pupil size can be monitored in a completely noninvasive way in humans, using a remote camera and infrared light. We hypothesized that if pupil size reflects the activity of the LC-NE system, which is one of the important attentional systems in humans, then pupil size is a potential and unexplored marker for attentional states in ADHD. If this is the case, changes in pupil size during an attentional task should reflect the behavioral differences observed between ADHD patients and control subjects.
We monitored pupil size from ADHD and control subjects during a visuo-spatial working memory task (see Fig. 1A ). Deficits in these tasks are one of the most consistent impairments of executive functions in patients with ADHD 30 , 31 . Indeed, unaffected siblings present deficits in this type of task, which are intermediate between ADHD and controls 31 , a finding that has led some authors to propose it as an endophenotype 2 , 32 . In our task, three images plus a distractor were sequentially presented and then a ‘probe’ image was shown. Subjects had to evaluate if the probe image had been presented before. ADHD patients performed the task in two separate sessions, on- and off-medication, which in all cases was methylphenidate. We found that pupil size changed during the probe presentation, the attentionally most relevant cue of each trial, and covaried with the subject’s variability in response time and performance. Furthermore, this effect was modulated by medication.
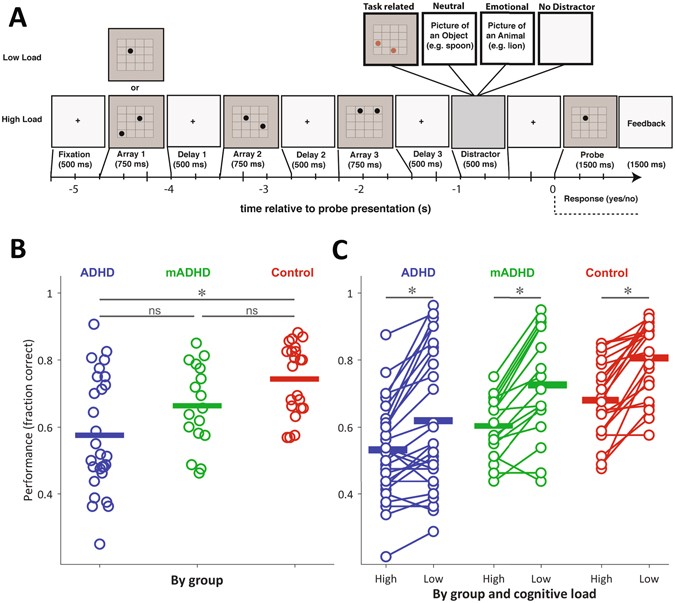
( A ) Schematic representation of a single trial. Following the presentation of the probe, subjects indicated with a button press whether the position of the dot had been presented in one of the previous arrays. (B) Task performance by group. Each dot corresponds to the mean session performance of a subject. Horizontal bars correspond to the group mean. The on-medication ADHD group (mADHD) is a subset of the ADHD group, tested on a different day. (C) Performance by group and cognitive load. Each dot corresponds to the mean session performance of a subject, in high and low cognitive load conditions. In both ( B ) and ( C ), performance is expressed as the fraction of correct trials of the session. Differences at p < 0.05 are highlighted.
As a first step, we performed the analysis using all the data (28 ADHD subjects, 22 controls, and a subgroup (17) of the 28 ADHD children who performed the task twice, on-medication and off-medication). Here we described the data and compared between groups, both the behavioral and the physiological measures. As a second step, we specifically focused on the subset of subjects which performed the task on- and off-medication. In this way, we were able to assess for medication-associated changes on the same subject.
Task performance results
To assess for performance differences between groups, we first conducted a Kruskal-Wallis analysis of variance, which gave significant differences (χ 2 (d.f.=2) = 13.4, p = 0.0012). Post-hoc test performed through the Bonferroni method, showed differences only between ADHD and Control groups (ADHD vs. mADHD: p = 0.4018; mADHD vs. Control: p = 0.2146; ADHD vs. Control: p = 0.0008). We obtained the same differences via Tukey’s Honestly Significant Difference Procedure. When trials were parsed by cognitive load (low: 1-dot memoranda, high: 2-dot memoranda), the data confirmed that 2-dot arrays were harder for the subjects, across all groups. There were significant differences in performance levels for low and high cognitive loads, as assessed by a Wilcoxon signed-rank test (Control: high 0.68 ± 0.11, low 0.81 ± 0.11, z = 4.02; p < 0.0001; ADHD: high 0.53 ± 0.14, low 0.62 ± 0.21, z = 3.5; p < 0.0001; mADHD: high 0.60 ± 0.09, low 0.73 ± 0.16, z = 3.31; p < 0.0001). The plot in Fig. 1C shows the change in performance for each subject, related to low and high cognitive loads.
Pupil diameter changes during the trial
Average pupil curves across trials and subjects (Fig. 2A ) reliably co-varied with visual stimuli presentation in the three groups. Each curve in Fig. 2A , which corresponds to an average across subjects, shows diameter increases associated with the presentation of the dot arrays, the distractor, and the probe dot. On average, the largest increase in pupil diameter in the three groups occurred after probe onset (to see individual pupil curves, see Supplementary Figure 1 ). To visualize and compare the actual maxima across trials, we performed a parallel analysis. Instead of looking at the average curve per subject, we used the same pupil timeseries to average the maximum pupil size for each subject and trial, after probe onset (see Methods). These values are shown in Fig. 2B . To assess for differences between groups in maximum pupil size after probe onset, we conducted a Kruskal-Wallis analysis of variance, which gave significant differences (χ 2 (d.f.=2) = 15.57, p = 0.0004). Post-hoc tests conducted using the Bonferroni method showed significant differences between ADHD and mADHD, and also between ADHD and Control, but not between mADHD and Control groups (ADHD vs. mADHD: p = 0.0361; mADHD vs. Control: p = 1.00; ADHD vs. Control: p = 0.0004). We obtained the same differences via Tukey’s Honestly Significant Difference Procedure. As such, medication tended to “normalize” the pupil response of patients during the attentional task. Pupil diameter average magnitudes for the first three arrays presentations are shown in Supplementary Fig. 2 .
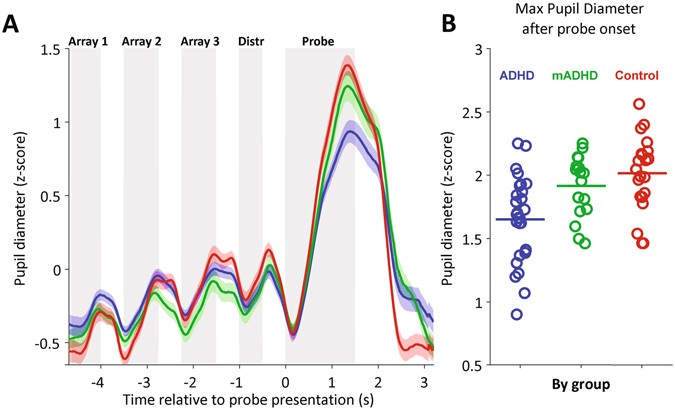
Mean pupil change during a trial and maximum pupil diameter after probe onset. (A) Mean pupil change during a trial, parsed by group. Each trace corresponds to the mean of each group (ADHD, n = 28; mADHD, n = 17; Control, n = 22). Color shaded areas correspond to the standard error of the mean. Grey shaded areas mark the periods of stimuli presentation during the trial. Dot Arrays 1–3: dot array presentations (See Fig. 1A ); Distr: distractor image presentation; Probe: probe dot presentation. (B) Maximum pupil diameter measured after probe onset. Each dot represents the mean session value for a subject (i.e., average across trials). Horizontal bars correspond to session averages across subjects.
Associations between pupil diameter and behavioral variables
We then evaluated associations between pupil diameter and two of the most relevant behavioral variables: performance and variability in reaction time. In all the combinations, these variables showed consistent associations, as assessed statistically by the Spearman correlation coefficient (see Fig. 3 ). We used the subjects from all three conditions to analyze these associations. The maximum pupil diameter measured after probe presentation was inversely associated to the variability in the subject’s reaction time (Spearman correlation, rho = −0.68, p < 0.0001, n = 67). On the other hand, maximum pupil diameter was directly associated with the subjects’ performance in the task (rho = 0.63, p < 0.0001, n = 67), indicating that pupil size during the crucial part of the task was associated with the subjects’ outcome. Finally, consistent with previous reports and with the two previous associations, we found that performance was inversely associated with the variability in reaction time (rho = −0.725, p < 0.001, n = 67). In terms of correlations within groups, we consistently found that the largest correlation values corresponded to the ADHD group, intermediate values to the mADHD group, and lowest to the Control group (see rho values in plots of Fig. 3 ). Performance did not co-vary with raw reaction time (see Supplementary Figure 4 ). Furthermore, pupil diameter average magnitudes for the first three arrays presentations positively covariates with arrays presentation (see Supplementary Figure 2 ) in control (Spearman correlation, rho = 0.5864, p = 2.29858e-07, n = 66) and ADHD group (Spearman correlation, rho = 0.27962, p < 0.01, n = 84). Nevertheless, this increase in pupil diameter does not correlates with performance and pupil diameter measured after probe presentation within subjects (see Supplementary Figure 3 ).
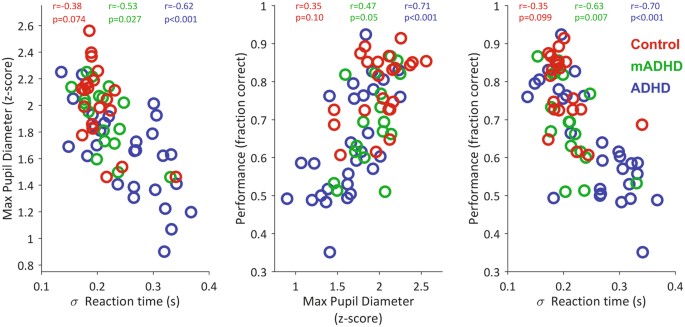
Relationships between task performance, reaction time variability and maximum pupil diameter after probe presentation. Each dot corresponds to the mean session value per subject. The values of the Spearman correlation coefficient (r: rho), separately by group, are shown at the top of each plot. Reaction time variability is presented as the standard deviation of the reaction time, which in turn corresponds to the time elapsed between probe presentation and subject’s response.
Paired comparisons between conditions on- and off-medication
For a total of 17 subjects, we were able to collect one off-medication session (children suspended their medication 24 hours before performing the task) and a matching on-medication session for the same subject. We used these data to evaluate differences between the on-medication and off-medication conditions for the experimental variables, both physiological (pupil diameter) and behavioral (performance, reaction time variability).
During on-medication sessions, children displayed a larger pupil diameter during probe presentation compared to off-medication sessions, as assessed by a Wilcoxon signed-rank test (z = −2.95, p = 0.003, n = 17 subjects) (see Fig. 4 ). Performance, on the other hand, did not vary significantly between on- and off-medication conditions (z = −0.69, p = 0.4925, n = 17 subjects), neither did the standard deviation of the subjects’ reaction time (z = 1.54, p = 0.1239, n = 17 subjects).
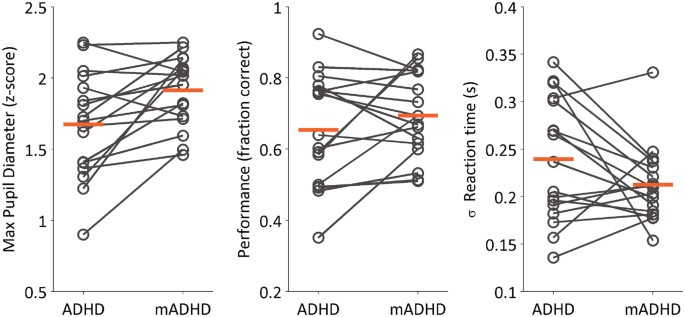
Paired changes between off-medicated (ADHD) and on-medicated (mADHD) conditions, for the subset of subjects which performed the task in both conditions (n = 17). Left: Change in maximum pupil diameter measured after probe dot presentation. Center: Change in performance, expressed as the fraction of correct trials of the session. Right: Standard deviation of the subject’s reaction time (time elapsed between probe presentation and subject’s response). In all plots, each dot represents the session mean for a subject. Orange horizontal bars correspond to the group mean.
Medication-associated changes in pupil diameter and behavioral variables
Finally, we analyzed the relations between pupil size and performance, and between pupil size and reaction time, specifically in the subset of subjects which performed the task in on- and off-medication conditions. To do this, we subtracted the mean variable values of the off-medication sessions from the mean values of on-medication sessions, separately for each subject. We added the prefix ‘delta’ (Δ) to the new variables representing the on-medicated minus off-medicated values. We then plotted these differences against each other (See Fig. 5A ). For the case of Δ pupil diameter and Δ performance, we found a positive association, as measured by Spearman correlation (rho = 0.75, p = 0.0007). In the case of Δ pupil diameter and Δ reaction time variability, we also found an association (rho = −0.63, p = 0.0071). These results indicate that if the medication was accompanied by a large increase in performance (or a large decrease in reaction time variability) for a given subject, then it was also accompanied by a large change in pupil diameter. Interestingly, we noted that the subjects who displayed a larger change in performance were subjects which performed first the task off-medication (filled circles in Fig. 5A ). We therefore next looked at mean values parsed by session, irrespective of medication condition (Fig. 5B ). We found that there was a small but significant effect of session on performance (Wilcoxon signed-rank test, z = −2.76, p = 0.0056, Hedges’ g = −0.63, first minus second) and not on pupil size (Wilcoxon signed-rank test, z = −1.27, p = 0.227, Hedges’ g = −0.33, first minus second).
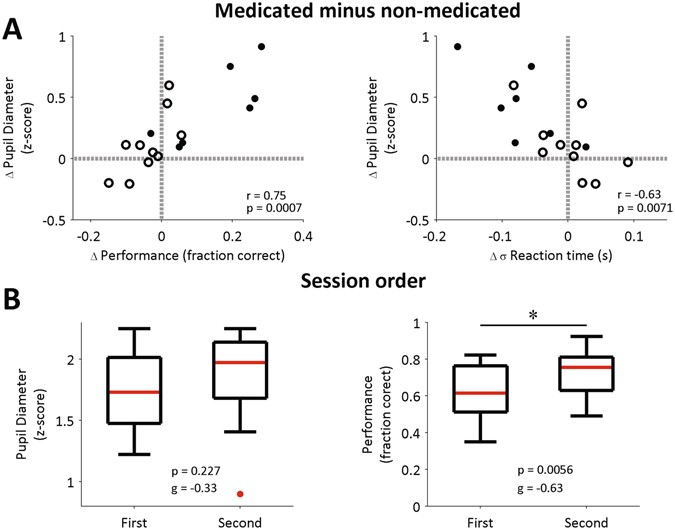
Medication-associated changes and session-order comparisons for ADHD subjects. ( A ) Association between change (delta, Δ) in pupil diameter after probe presentation, and changes in performance (left) and standard deviation of reaction time (right). The change is calculated as the mean difference between on-medicated and off-medicated conditions. Each dot represents the difference in session means for a subject. Grey reference lines are drawn at zero changes. Empty circles: subjects whose first session was on-medication; Filled circles: subjects whose first session was off-medication. r: Spearman’s rank correlation coefficient. p: p-value for testing the hypothesis of no correlation. ( B ) Pupil diameter (left) and performance (right) separately by session. Data were parsed by session, regardless of the medication condition (on- or off-). Each boxplot was built using the mean values from each of the 17 subjects. p: p-values from Wilcoxon signed-rank test. g: Hedges’ g (measure of effect size).
These results show that there could be an effect of medication on the observed pupil and reaction time changes, but in our data there is a potential confound with training, measured as session order.
We present evidence supporting pupil changes during an attentional task as a promising biomarker for ADHD. It has been recently shown in animal experiments that pupil diameter tracks arousal and attentional states 12 , 23 , 27 . Specifically, pupil diameter covaries with neuronal activity in the LC-NE system, the so-called ‘arousal’ or ‘alerting’ network. The LC-driven norepinephrine modulation, which reaches almost the entire cortical surface, has a direct readout in pupil size 23 . This confluence of evidence from NE involvement in attentional circuits and control of pupil size prompted us to explore pupil size as a biological marker in ADHD.
We tested ADHD and control children in a visuo-spatial working memory task (Fig. 1A ) previously known to be challenging for ADHD patients. A subset of ADHD children performed the task both in on-medication and off-medication conditions. Off-medication ADHD patients showed decreased pupil diameter response during the task as compared to controls (see Fig. 2 ). On average, this difference was no longer present when patients were on-medication. Specifically, we saw in our task an event-related pupil response which tracks the array presentations and displays the largest increase during the probe dot presentation. When the probe dot was presented, subjects had to pay attention to it, compare it with what they recall about the previous three arrays and make a decision. In this crucial moment of the task, the size of the induced pupil response helped us to distinguish between patients and control subjects. Given that pupil diameter reflects the activity of the LC-NE system 11 , 12 , 22 , 23 , these results support the involvement of the cerebral NE system in ADHD.
We found a correlation between pupil size and subjects’ performance, measured as response accuracy and reaction time variablility, in the ADHD group. These performance metrics, are two vastly studied behavioral ADHD markers in attentional tasks 9 , 33 , 34 . In this study, the correlations between these markers and pupil size were weaker in the on-medication group and display their lowest values in the control group. This association between pupil size and behavior seems to be restricted to the period after probe onset. For example, the sustained baseline increase across array presentations (see Fig. 2A ) did not correlate with response accuracy or reaction time variability (see Supplementary Figures 2 and 3 ). It has been proposed that these manifestations of ADHD (increased reaction time variability and poor accuracy in attentional tasks) could be caused by transient disruptions of attentional network activity 33 , 34 . These disruptions are in turn caused by an increase in the activity of other internal networks, such as the default mode network. In addition, recent models have proposed the role of LC-NE circuits in so-called ‘mind wandering’ periods, which might potentiate the default mode network and decrease the activity of attentional networks 35 . This interference is characterized by a decrease in the phasic gain of the LC-NE system which, in turn, causes decreased pupil diameters 35 . Thus, there are compelling reasons for using pupil-size dynamics to characterize and diagnose ADHD.
We also found that the subjects whose performance and reaction time variability changed more with the medication, also displayed larger pupil-size change between on-medicated and off-medicated conditions (Fig. 5 ). Hence, the co-variation between pupil size change and behavioral change seems subject-specific. We note that the session order (on-medication or off-medication) was randomized in our subjects, which means that some of them learned the task off-medication and then were tested a second time on-medication. Besides, performance, pupil diameter and reaction time variability did not consistently change across trial blocks (see Supplementary Figure 5 ). This suggests that motivational or learning effects were not modulating these responses.
Overall, the association between pupil diameter changes and ADHD behavioral performance markers, and how these associations differ between ADHD and normal children, suggest that pupil diameter changes during a visual-spatial working memory task may be a useful biological marker of ADHD. These associations are also consistent with the hypothesis that ADHD is a neurodevelopmental disorder related with the broadly distributed NE system, and not only a problem confined to a prefrontal-striatal dopamine circuit, as implied by the classical version of the dopaminergic hypothesis of ADHD 5 , 36 .
A total of 50 subjects participated in the study. 28 subjects were patients diagnosed with ADHD (4 girls, age: 10.71 ± 0.54 years old). All ADHD children were being treated with methylphenidate. 22 subjects were non-ADHD assigned to our control group (4 girls, age: 11.58 ± 0.50 years-old). A subgroup of 17 ADHD patients (3 girls, age: 11.19 ± 0.86 years-old) performed the task twice, on- and off-medication (time between sessions: 180.23 ± 125.17 days). The order of the sessions (on- or off-medication) was randomized to avoid carry-over effects. In the on-medication session, ADHD subjects took their regularly prescribed dose 2–2.5 hr before the task, which is the time corresponding to the maximum drug concentration after oral administration 37 . For off-medication sessions, children discontinued their medication 24 h prior to the day of testing, which is enough time to remove 80% of the circulating drug levels 38 , 39 . No significant differences were found between the groups in age, IQ, or educational level. The ADHD group had a mean IQ of 100 (SD = ± 11.6), while the non-ADHD group had a mean IQ of 105 (SD = ± 7.3). All ADHD subjects were diagnosed as ADHD-Combined Subtype by a trained child neurologist according to the DSM-IV criteria 40 . All participants were also screened for neurologic or psychiatric comorbid conditions using a protocol that included parents’ interview, M.I.N.I. Kid test 41 , and general psychological and physical assessment of the children. The Conner’s Abbreviated Parent–Teacher Questionnaire 41 is usually utilized to screen for symptoms of ADHD in the clinical setting. In the present study, it was used as an additional symptoms-counting tool. Participants with ADHD and those from the non-ADHD group were evaluated using this instrument as a controlling variable. Parents granted informed consent for their children’s participation, and children signed an informed assent form. The procedures in the study were approved by the Ethics Committee of the School of Medicine of the Pontificia Universidad Católica de Chile (Protocol number 11082), in accordance with the Declaration of Helsinski.
Subjects performed a Sternberg-type delayed visuo-spatial working memory task, adapted from Dolcos & McCarthy 42 . The memoranda were 1- or 2-dot arrays, with the dots located variably in any of the sixteen spaces of a 4 × 4 grid (See Fig. 1A ).
On each trial, subjects were instructed to start by fixating on a black cross located at the center of the screen. After 500 ms, dot array presentation commenced. Three different dot arrays were presented on each trial. Each array was presented for 750 ms, with a 500 ms delay between arrays, during which a fixation cross was presented (see Fig. 1A ). After the last delay period, a distractor image was presented for 500 ms. After the distractor, a ‘probe’ dot was presented for 1.5 seconds. This was a dot within the grid, and subjects had to answer ‘yes’ if the probe dot had been presented in one of the trial’s previous arrays, or ‘no’ if it had not. Immediately after probe offset we provided a feedback image for 1500 ms, indicating if the subject response was correct or incorrect. The participants were instructed to respond as fast as possible.
We used three distractors: (1) A task-related dot array; (2) a neutral natural image; and (3) an emotional image. Distractors were constructed and modified from public domain images, adapted from Vidal, Ossandon et al . 43 . In 25% of the trials, no distractor was presented. Trial types were presented randomly.
There were also two trial types, according to cognitive load: in low-load trials, only one dot was presented on each image, whereas in high-load trials, two dots were presented on each image. Therefore, in low-load trials subjects had to retain the location of three dots (one per image) and in high-load trials they had to retain the location of six dots (two per image).
A total of 160 trials were presented on each session, separated in 8 blocks of 20 trials. Sessions usually lasted 30 minutes.
Data Acquisition
Pupil diameter data was acquired with Eyelink 1000 (SR Research Ltd., Mississauga, Ontario, Canada), with a 1 kHz sampling frequency. Subjects sat in front of a table containing the computer screen for image presentation and the eye tracker device. During the task, the subjects kept their head in a forehead/chin rest (SR Research Ltd.). Subjects were placed at a viewing distance of 30 cm from the display monitor. We measured illuminance data with a lux-meter positioned in the same chin-rest used by the subjects, at the same distance from the screen. The only modestly large differences in illuminance occurred between delay and array displays. The distractors presented more variability in illuminance and had lower illuminance values, on average (see Supplementary Figure 6 ). Illuminance changes were always on the order of 1 lux (as a reference, a change from white to black screens, in our setup, is associated with a drop of ~100 lux).
Behavioral Data Analysis
Stimuli were presented using Presentation® software (Neurobehavioral Systems, Inc.), and subjects delivered their responses using a keypad. Alongside checking for correct responses, we also recorded the reaction time as the time delay between the probe presentation and the subject’s response. This allowed us to calculate the variability in reaction time, a parameter known to correlate with cognitive performance in ADHD children 44 , 45 .
Pupil Data Analysis
Pupil diameter analysis was performed using Matlab® software with in-house functions. First of all, periods of blinks, in which no pupil diameter information was available, were detected by the Eyelink software. Pupil data surrounding blinks were removed from the time series used in the analyses. Pupil diameter during these periods was estimated using a cubic spline interpolation 46 , implemented through Matlab function spline .
To obtain the pupil diameter average profile (Fig. 2A ), data of each participant were baseline-adjusted and smoothed by a bandpass Butterworth filter between 0.025 Hz and 4 Hz. A low-pass frequency filter extracted the high frequency noise 29 , and a high-pass filter was applied to detrend the basal slow change of the pupil diameter across trials. Furthermore, outliers, defined as periods of pupil change (derivative function) higher than 3 standard errors from the mean were discarded. Finally, all trials with more than 50% of missing data (due to blinks or outliers) were not considered in the analysis. Unless otherwise stated, data analysis was restricted to the 8-s trial period (Fig. 1A ). After filtering and outlier removal, the pupil timeseries was normalized by means of a z-score, separately for each trial.
To calculate the pupil diameter maxima during probe presentation (Fig. 2B ), we used the same z-scored pupil time series used in Fig. 2A . We defined a window between 500 ms after probe onset and 500 ms after probe offset. Within that window, we calculated the maximum value of pupil diameter for each trial. We then computed the mean value of those maxima, separately per subject, to obtain each data point in Fig. 2B .
All our statistical tests were implemented in Matlab® (release 2016a). Due to the non-normal nature of the data, we used non-parametric tests to assess statistically for differences. For paired comparisons, we used the Wilcoxon signed-rank test, with Matlab function signrank . For non-paired comparisons, we implemented permutations tests using a t-statistic (difference between group means normalized by a pooled standard deviation). In this case, we first computed the t-statistic for the actual (non-permuted) data. We then implemented the permutations and calculated the t-statistic for each permutation. To assess significance, we compared the non-permuted t-value with the distribution of permuted t-values and calculated if it lied within 95% of the distribution (i.e., we used a significance level alpha = 0.05). All tests were performed using the subject as the unit of analysis (i.e., the ‘n’ of the test is always the number of subjects).
Data availability
The authors declare that the data gathered for this study are available within the paper. We also state that the data are available from the authors upon reasonable request.
Aboitiz, F. & Castellanos, F. X. In Attention Deficit Hyperactivity Disorder (ed S. Evans and B. Hoza) (Kingston, NJ:Civic Research Institute, 2011).
Kiely, B. & Adesman, A. What we do not know about ADHD… yet. Current opinion in pediatrics 27 , 395–404, doi: 10.1097/mop.0000000000000229 (2015).
Article PubMed Google Scholar
Bonvicini, C., Faraone, S. V. & Scassellati, C. Attention-deficit hyperactivity disorder in adults: A systematic review and meta-analysis of genetic, pharmacogenetic and biochemical studies. Molecular psychiatry 21 , 872–884, doi: 10.1038/mp.2016.74 (2016).
Article CAS PubMed PubMed Central Google Scholar
Clark, C. R., Geffen, G. M. & Geffen, L. B. Catecholamines and attention. I: Animal and clinical studies. Neurosci Biobehav Rev 11 , 341–352 (1987).
Article CAS Google Scholar
Swanson, J. M. et al . Etiologic subtypes of attention-deficit/hyperactivity disorder: brain imaging, molecular genetic and environmental factors and the dopamine hypothesis. Neuropsychology review 17 , 39–59, doi: 10.1007/s11065-007-9019-9 (2007).
Hamed, A. M., Kauer, A. J. & Stevens, H. E. Why the Diagnosis of Attention Deficit Hyperactivity Disorder Matters. Frontiers in psychiatry 6 , 168, doi: 10.3389/fpsyt.2015.00168 (2015).
Article PubMed PubMed Central Google Scholar
Rauh, V. A. & Margolis, A. E. Research Review: Environmental exposures, neurodevelopment, and child mental health - new paradigms for the study of brain and behavioral effects. Journal of child psychology and psychiatry, and allied disciplines 57 , 775–793, doi: 10.1111/jcpp.12537 (2016).
Thapar, A. & Cooper, M. Attention deficit hyperactivity disorder. The Lancet 387 , 1240–1250, doi: 10.1016/S0140-6736(15)00238-X (2016).
Article Google Scholar
Aboitiz, F., Ossandon, T., Zamorano, F., Palma, B. & Carrasco, X. Irrelevant stimulus processing in ADHD: catecholamine dynamics and attentional networks. Frontiers in psychology 5 , 183, doi: 10.3389/fpsyg.2014.00183 (2014).
Kapur, S., Phillips, A. G. & Insel, T. R. Why has it taken so long for biological psychiatry to develop clinical tests and what to do about it? Molecular psychiatry 17 , 1174–1179, doi: 10.1038/mp.2012.105 (2012).
Article CAS PubMed Google Scholar
Reimer, J. et al . Pupil fluctuations track fast switching of cortical states during quiet wakefulness. Neuron 84 , 355–362, doi: 10.1016/j.neuron.2014.09.033 (2014).
Reimer, J. et al . Pupil fluctuations track rapid changes in adrenergic and cholinergic activity in cortex. Nature communications 7 , 13289, doi: 10.1038/ncomms13289 (2016).
Article ADS CAS PubMed PubMed Central Google Scholar
Siegle, G. J., Steinhauer, S. R., Stenger, V. A., Konecky, R. & Carter, C. S. Use of concurrent pupil dilation assessment to inform interpretation and analysis of fMRI data. NeuroImage 20 , 114–124 (2003).
Smallwood, J. & Schooler, J. W. The science of mind wandering: empirically navigating the stream of consciousness. Annual review of psychology 66 , 487–518, doi: 10.1146/annurev-psych-010814-015331 (2015).
Unsworth, N. & Engle, R. W. The nature of individual differences in working memory capacity: active maintenance in primary memory and controlled search from secondary memory. Psychol Rev 114 , 104–132, doi: 10.1037/0033-295X.114.1.104 (2007).
Ahern, S. & Beatty, J. Pupillary responses during information processing vary with Scholastic Aptitude Test scores. Science (New York, N.Y.) 205 , 1289–1292 (1979).
Article ADS CAS Google Scholar
Henderson, R. R., Bradley, M. M. & Lang, P. J. Modulation of the initial light reflex during affective picture viewing. Psychophysiology 51 , 815–818, doi: 10.1111/psyp.12236 (2014).
Mandrick, K., Peysakhovich, V., Remy, F., Lepron, E. & Causse, M. Neural and psychophysiological correlates of human performance under stress and high mental workload. Biological psychology 121 , 62–73, doi: 10.1016/j.biopsycho.2016.10.002 (2016).
Peysakhovich, V., Causse, M., Scannella, S. & Dehais, F. Frequency analysis of a task-evoked pupillary response: Luminance-independent measure of mental effort. International journal of psychophysiology: official journal of the International Organization of Psychophysiology 97 , 30–37, doi: 10.1016/j.ijpsycho.2015.04.019 (2015).
Varazzani, C., San-Galli, A., Gilardeau, S. & Bouret, S. Noradrenaline and dopamine neurons in the reward/effort trade-off: a direct electrophysiological comparison in behaving monkeys. J Neurosci 35 , 7866–7877, doi: 10.1523/jneurosci.0454-15.2015 (2015).
Zenon, A., Sidibe, M. & Olivier, E. Pupil size variations correlate with physical effort perception. Frontiers in behavioral neuroscience 8 , 286, doi: 10.3389/fnbeh.2014.00286 (2014).
Aston-Jones, G. & Cohen, J. D. An integrative theory of locus coeruleus-norepinephrine function: adaptive gain and optimal performance. Annual review of neuroscience 28 , 403–450, doi: 10.1146/annurev.neuro.28.061604.135709 (2005).
Joshi, S., Li, Y., Kalwani, R. M. & Gold, J. I. Relationships between Pupil Diameter and Neuronal Activity in the Locus Coeruleus, Colliculi, and Cingulate Cortex. Neuron 89 , 221–234, doi: 10.1016/j.neuron.2015.11.028 (2016).
McGinley, M. J. et al . Waking State: Rapid Variations Modulate Neural and Behavioral Responses. Neuron 87 , 1143–1161, doi: 10.1016/j.neuron.2015.09.012 (2015).
Sara, S. J. & Bouret, S. Orienting and reorienting: the locus coeruleus mediates cognition through arousal. Neuron 76 , 130–141, doi: 10.1016/j.neuron.2012.09.011 (2012).
Gabay, S., Pertzov, Y. & Henik, A. Orienting of attention, pupil size, and the norepinephrine system. Attention, perception & psychophysics 73 , 123–129, doi: 10.3758/s13414-010-0015-4 (2011).
Chandler, D. J. Evidence for a specialized role of the locus coeruleus noradrenergic system in cortical circuitries and behavioral operations. Brain research 1641 , 197–206, doi: 10.1016/j.brainres.2015.11.022 (2016).
Corbetta, M., Patel, G. & Shulman, G. L. The reorienting system of the human brain: From environment to theory of mind. Neuron 58 , 306–324, doi: 10.1016/j.neuron.2008.04.017 (2008).
Privitera, C. M., Renninger, L. W., Carney, T., Klein, S. & Aguilar, M. Pupil dilation during visual target detection. J Vis 10 , 3, doi: 10.1167/10.10.3 (2010).
Martinussen, R., Hayden, J., Hogg-Johnson, S. & Tannock, R. A meta-analysis of working memory impairments in children with attention-deficit/hyperactivity disorder. Journal of the American Academy of Child and Adolescent Psychiatry 44 , 377–384, doi: 10.1097/01.chi.0000153228.72591.73 (2005).
van Ewijk, H. et al . Visuospatial working memory in ADHD patients, unaffected siblings, and healthy controls. Journal of attention disorders 18 , 369–378, doi: 10.1177/1087054713482582 (2014).
Castellanos, F. X. & Tannock, R. Neuroscience of attention-deficit/hyperactivity disorder: the search for endophenotypes. Nat Rev Neurosci 3 , 617–628, doi: 10.1038/nrn896 (2002).
Castellanos, F. X. & Proal, E. Large-scale brain systems in ADHD: beyond the prefrontal-striatal model. Trends Cogn Sci 16 , 17–26, doi: 10.1016/j.tics.2011.11.007 (2012).
Castellanos, F. X., Sonuga-Barke, E. J., Milham, M. P. & Tannock, R. Characterizing cognition in ADHD: beyond executive dysfunction. Trends Cogn Sci 10 , 117–123, doi: 10.1016/j.tics.2006.01.011 (2006).
Mittner, M., Hawkins, G. E., Boekel, W. & Forstmann, B. U. A Neural Model of Mind Wandering. Trends Cogn Sci 20 , 570–578, doi: 10.1016/j.tics.2016.06.004 (2016).
Gonon, F. The dopaminergic hypothesis of attention-deficit/hyperactivity disorder needs re-examining. Trends in neurosciences 32 , 2–8, doi: 10.1016/j.tins.2008.09.010 (2009).
Kimko, H. C., Cross, J. T. & Abernethy, D. R. Pharmacokinetics and Clinical Effectiveness of Methylphenidate. Clinical Pharmacokinetics 37 , 457–470, doi: 10.2165/00003088-199937060-00002 (1999).
Ortega, R., Lopez, V., Carrasco, X., Anllo-Vento, L. & Aboitiz, F. Exogenous orienting of visual-spatial attention in ADHD children. Brain research 1493 , 68–79, doi: 10.1016/j.brainres.2012.11.036 (2013).
Vaidya, C. J. et al . Selective effects of methylphenidate in attention deficit hyperactivity disorder: A functional magnetic resonance study. Proceedings of the National Academy of Sciences 95 , 14494–14499, doi: 10.1073/pnas.95.24.14494 (1998).
American Psychiatric Association, A. P. A. DSM-IV-TR . (American Psychiatric Publishing, 2000).
Sheehan, D. V. et al . The Mini-International Neuropsychiatric Interview (M.I.N.I.): the development and validation of a structured diagnostic psychiatric interview for DSM-IV and ICD-10. The Journal of clinical psychiatry 59 (Suppl 20), 34-57, 22–33;quiz (1998).
Dolcos, F. & McCarthy, G. Brain systems mediating cognitive interference by emotional distraction. The Journal of neuroscience: the official journal of the Society for Neuroscience 26 , 2072–2079, doi: 10.1523/JNEUROSCI.5042-05.2006 (2006).
Vidal, J. R. et al . Category-Specific Visual Responses: An Intracranial Study Comparing Gamma, Beta, Alpha, and ERP Response Selectivity. Frontiers in human neuroscience 4 , 195, doi: 10.3389/fnhum.2010.00195 (2010).
Johnson, K. A. et al . Response variability in attention deficit hyperactivity disorder: evidence for neuropsychological heterogeneity. Neuropsychologia 45 , 630–638, doi: 10.1016/j.neuropsychologia.2006.03.034 (2007).
Vaurio, R. G., Simmonds, D. J. & Mostofsky, S. H. Increased intra-individual reaction time variability in attention-deficit/hyperactivity disorder across response inhibition tasks with different cognitive demands. Neuropsychologia 47 , 2389–2396, doi: 10.1016/j.neuropsychologia.2009.01.022 (2009).
Blom, T., Mathot, S., Olivers, C. N. & Van der Stigchel, S. The pupillary light response reflects encoding, but not maintenance, in visual working memory. Journal of experimental psychology. Human perception and performance 42 , 1716–1723, doi: 10.1037/xhp0000252 (2016).
Download references
Acknowledgements
Our research was supported by CONICYT, FONDECYT 1140996 to TO, FONDECYT 1160736 to NC, FONDECYT 1160258 to FA and CONICYT PIA ACT1414.
Author information
G. Wainstein and D. Rojas-Líbano contributed equally to this work.
Authors and Affiliations
Departamento de Psiquiatría, Escuela de Medicina and Centro Interdisciplinario de Neurociencia, Pontificia Universidad Católica de Chile, Santiago, Chile
G. Wainstein, D. Rojas-Líbano, N. A. Crossley, F. Aboitiz & T. Ossandón
Laboratorio de Neurociencia Cognitiva y Social, Facultad de Psicología, Universidad Diego Portales, Santiago, Chile
D. Rojas-Líbano
Department of Psychosis Studies, Institute of Psychiatry, Psychology and Neuroscience. King’s College London, London, UK
N. A. Crossley
Servicio de Neurología y Psiquiatría, Hospital de Niños Dr. Luis Calvo Mackenna, Facultad de Medicina, Universidad de Chile, Santiago, Chile
X. Carrasco
You can also search for this author in PubMed Google Scholar
Contributions
G.W.: Analyzed data, prepared figures, wrote manuscript. D.R.-L.: Analyzed data, prepared figures, wrote manuscript. N.C.: Discussed data, revised and commented manuscript. X.C.: Diagnosis and evaluation of ADHD patients, discussed data, revised and commented manuscript. F.A.: Provided recording equipment, discussed data, revised and commented manuscript. T.O.: Conceived the project, carried out experiments, analyzed data, prepared figures, wrote manuscript.
Corresponding author
Correspondence to T. Ossandón .
Ethics declarations
Competing interests.
The authors declare that they have no competing interests.
Additional information
Publisher's note: Springer Nature remains neutral with regard to jurisdictional claims in published maps and institutional affiliations.
Electronic supplementary material
Supplementary information, rights and permissions.
Open Access This article is licensed under a Creative Commons Attribution 4.0 International License, which permits use, sharing, adaptation, distribution and reproduction in any medium or format, as long as you give appropriate credit to the original author(s) and the source, provide a link to the Creative Commons license, and indicate if changes were made. The images or other third party material in this article are included in the article’s Creative Commons license, unless indicated otherwise in a credit line to the material. If material is not included in the article’s Creative Commons license and your intended use is not permitted by statutory regulation or exceeds the permitted use, you will need to obtain permission directly from the copyright holder. To view a copy of this license, visit http://creativecommons.org/licenses/by/4.0/ .
Reprints and permissions
About this article
Cite this article.
Wainstein, G., Rojas-Líbano, D., Crossley, N.A. et al. Pupil Size Tracks Attentional Performance In Attention-Deficit/Hyperactivity Disorder. Sci Rep 7 , 8228 (2017). https://doi.org/10.1038/s41598-017-08246-w
Download citation
Received : 20 April 2017
Accepted : 10 July 2017
Published : 15 August 2017
DOI : https://doi.org/10.1038/s41598-017-08246-w
Share this article
Anyone you share the following link with will be able to read this content:
Sorry, a shareable link is not currently available for this article.
Provided by the Springer Nature SharedIt content-sharing initiative
This article is cited by
Eye-movement indices of arousal predict adhd and comorbid externalizing symptoms over a 2-year period.
- Johan Lundin Kleberg
- Matilda A. Frick
- Karin C. Brocki
Scientific Reports (2023)
Attention and impulsivity assessment using virtual reality games
- David Mendez-Encinas
- Aaron Sujar
- David Delgado-Gomez
Wavelet-based approach for diagnosing attention deficit hyperactivity disorder (ADHD)
- Dixon Vimalajeewa
- Ethan McDonald
- Brani Vidakovic
Scientific Reports (2022)
A Robust Machine Learning Based Framework for the Automated Detection of ADHD Using Pupillometric Biomarkers and Time Series Analysis
- William Das
- Shubh Khanna
Scientific Reports (2021)

The path from trigeminal asymmetry to cognitive impairment: a behavioral and molecular study
- Maria Paola Tramonti Fantozzi
- Giulia Lazzarini
- Diego Manzoni
By submitting a comment you agree to abide by our Terms and Community Guidelines . If you find something abusive or that does not comply with our terms or guidelines please flag it as inappropriate.
Quick links
- Explore articles by subject
- Guide to authors
- Editorial policies
Sign up for the Nature Briefing newsletter — what matters in science, free to your inbox daily.

ORIGINAL RESEARCH article
Oculometric variations during mind wandering.
- 1 Centre de Recherche Cerveau et Cognition, Paul Sabatier University, Toulouse, France
- 2 Centre de Recherche Cerveau et Cognition, CNRS, UMR5549, Toulouse, France
- 3 Swartz Center for Computational Neuroscience, Institute for Neural Computation, University of California San Diego, La Jolla CA, USA
A significant body of literature supports the contention that pupil size varies depending on cognitive load, affective state, and level of drowsiness. Here we assessed whether oculometric measures such as gaze position, blink frequency and pupil size were correlated with the occurrence and time course of self-reported mind-wandering episodes. We recorded the pupil size of two subjects engaged in a monotonous breath counting task while keeping their eyes on a fixation cross. This task is conducive to producing mind-wandering episodes. Each subject performed ten 20-min sessions, for total duration of about 4 h. Subjects were instructed to report spontaneous mind-wandering episodes by pressing a button when they lost count of their breath. After each button press, subjects filled in a short questionnaire describing the characteristics of their mind-wandering episode. We observed larger pupil size during the breath-focusing period compared to the mind-wandering period ( p < 0.01 for both subjects). Our findings contradict previous research showing a higher baseline pupil size during mind wandering episodes in visual tasks. We discuss possible explanations for this discrepancy. We also analyzed nine other oculometric measures including blink rate, blink duration and gaze position. We built a support vector machine (SVM) classifier and showed that mean pupil size was the most reliable predictor of mind wandering in both subjects. The classification accuracy of mind wandering data segments vs. breath-focusing data segments was 81% for the first subject and 77% for the second subject. Additionally, we analyzed oculometric measures in light of the phenomenological data collected in the questionnaires. We showed that how well subjects remembered their thoughts while mind wandering was positively correlated with pupil size (subject 1, p < 0.001; subject 2, p < 0.05). Feelings of well being were also positively correlated with pupil size (subject 1, p < 0.001; subject 2, p < 0.001). Our results suggest that oculometric data could be used as a neurocognitive marker of mind-wandering episodes.
Introduction
As humans, our existence in this world is both the most natural yet complex thing we experience. Technological developments have brought new ways to study, quantify and characterize the mind through brain imaging. Among the many different aspects of cognition, attention has been at the center of a long line of research and a central process in the building of our stream of consciousness ( Baars, 1993 , 1997 ; Posner, 1994 ; Posner and Rothbart, 1998 ; Simons and Chabris, 1999 ; Koch and Tsuchiya, 2007 ). Most studies of attention have focused on an externally oriented type of attention, using exteroceptive tasks such as detection tasks, categorization tasks, and working memory tasks. Internal mental events, such as phenomenological experiences of feelings, thoughts, and body sensations that are not directly triggered by our environment, are dimensions of attention that have not yet been extensively studied. In particular, spontaneous thoughts often attract our attention, supplanting perception of the external world ( Smallwood et al., 2008 ; Schooler et al., 2011 ). When engaged in a task we are familiar with (especially when it does not require high attentional engagement), we are more likely to switch to a mental state which is now commonly referred to as mind wandering (MW) ( Smallwood and Schooler, 2006 ).
Finding the neural or physiological markers of MW has become important in order to better characterize and understand this mental state. However, studying this phenomenon is not a trivial task, as it is by its very nature a spontaneous, task-unrelated, internal mental process of which the subject is often unaware. It is thus difficult to study, document and replicate mind wandering using classical psychophysical paradigms ( Gruberger and Zangen, 2011 ). Despite these difficulties, MW has been characterized in several ways: behavioral measures ( Smallwood and Schooler, 2006 ; Smallwood et al., 2008 ), EEG ( Smallwood et al., 2008 ; Braboszcz and Delorme, 2011 ), fMRI ( Smith et al., 2006 ; Christoff et al., 2009 ), and pupil size ( Smallwood et al., 2011 ). Smallwood et al. (2011) showed a reduced entrainment of pupil dilation to external stimuli during mind wandering occurring during a choice reaction time task and a working memory task. They also observed increased pupil diameter during periods where mind wandering was more likely to occur. We were interested in determining whether the same effect (in addition to other potential oculometric changes) occurred during self-reported MW episodes.
There are two main reasons for our study. First, previous oculometric research on mind wandering relied on probed mind wandering protocols where subjects are asked at regular intervals if they are mind wandering. We were interested in verifying that self caught mind wandering episodes showed the same kind of oculometric effects. Also, Smallwood et al. (2011) focused on pupil size only, while we believe there are additional eye metrics that may potentially correlate with mind wandering. Second, the two tasks involved in Smallwood and colleagues' study were a choice reaction time task and working memory task. We wanted to investigate a more interoceptive concentration task, i.e., focusing on counting breath cycles, and self-monitoring of mind wandering. The choice of this task is related to the study of mind wandering in the context of meditation, similar to work done by Braboszcz and Delorme (2011) , and Hasenkamp et al. (2012) .
Our self-caught mind wandering protocol as described in Braboszcz and Delorme (2011) is original for two main reasons: first, it includes spontaneous meta-conscious events, (i.e., when the participant notices he or she is mind wandering) and the neuro-cognitive processes underlying meta-conscious events are poorly understood. Second, this type of protocol requires the self-monitoring of attention (and more generally of mental content) which is involved in various situations and tasks in our daily lives, and more specifically in attention training practices such as meditation. Two recent studies have shown that self-reported mind wandering paradigms can be used to characterize EEG ( Braboszcz and Delorme, 2011 ) and fMRI ( Hasenkamp et al., 2012 ) correlates of mind wandering in the context of a breath counting task. Here, we study for the first time oculometric correlates in the context of this task. In our task, subjects are not probed at a regular interval about their state of mental focus. Instead, they are asked to perform a breath counting task while keeping their eyes on a fixation cross, and are instructed to press a button whenever they realize they are not performing the breath counting task (i.e., they were mind wandering) ( Braboszcz and Delorme, 2011 ). This breath counting task is similar to meditation practices that focus on the breath. This task is more interoceptive, and may be more ecological (similar to reading tasks used in previous research) than other mind-wandering psychophysics tasks.
While oculometric variations during mind wandering have not been extensively studied, we believe it may be an important physiological marker of this mental state. More specifically, pupil size variation has been associated with loecus coerelus (LC) activity ( Usher et al., 1999 ; Carter et al., 2010 ), mainly through the noerepinephrine circuit. Neural activity in the noradrenergic locus coeruleus correlates with periods of wakefulness and arousal ( Carter et al., 2010 ). It has also been shown that electrotonic coupling in noradrenergic LC neurons may play an important role in attentional modulation, and the regulation of goal-directed vs. exploratory behaviors ( Usher et al., 1999 ).
In order to study oculometric traits and differences between mind wandering and states of concentration, we conducted statistical analysis on pupil size variation, eye blinks and gaze position. This standard statistical analysis was complemented by a classification analysis aimed at assessing the potential of using oculometric measures to detect mind wandering at the single trial level. Finally, psychometric data pertaining to self-evaluation of mind wandering episodes are reported and analyzed in light of the oculometric data.
Materials and Methods
Two participants S1 female (age 25) and S2 male (age 31) volunteered for this experiment after providing written informed consent. Both participants had normal or corrected to normal vision. They were both right handed and reported no mental or neurological disorder. The two subjects did not receive any monetary compensation for their participation. The experimental protocol was approved by the local ethical committee (CPP 2010-A00744-35). Both subjects had performed the task before and had been practicing meditation.
Stimuli and Procedure
The experimental task was adapted from Braboszcz and Delorme (2011) . Subjects sat in a dimly lit room with their heads on a chin rest. A computer screen was placed 60 cm in front of them. Stimuli presentation was performed using the MATLAB platform (The MathWorks) and the Psychophysics Toolbox 3 ( Brainard, 1997 ; Pelli, 1997 ). Instructions were displayed at the beginning of each session on the screen. We asked subjects to gaze continuously at a fixation cross displayed in the center of the screen. The task of the subjects was to count backward each of their breath cycles (inhale/exhale) from 10 to 1. At 1, they were instructed to restart counting backward from 10. This backward counting task was adopted as some subjects reported being able to count forward and mind wander simultaneously ( Braboszcz and Delorme, 2011 ). We will refer to this mental state as the F mental state for Focusing. Subjects also had to indicate whenever they realized they had lost track of their breath count (i.e., that their attention had drifted) by pressing the left mouse button. Immediately following the button press, a short 1-page phenomenological questionnaire was presented on the computer screen. The questionnaire allowed the subject to characterize their mind wandering episodes—it usually took subjects less than 30 s to complete the questionnaire (average 28 ± 12 s). Subjects were required to answer each question. The graphic user interface, instructions to subjects and questionnaire content are described in supplementary materials (Supplementary Figures 1 and 2 ). Each question was explained to the subject during a preliminary test, allowing the subject to familiarize himself both with the questions and the user interface. After the questionnaire was completed, subjects had to press the right mouse button to indicate they were ready to restart the breath counting task. The experimental task is illustrated in Figure 1 .

Figure 1. Experimental task. Subjects performed a breath counting/focusing task until they spontaneously started to mind wander. Upon realizing they were mind wandering (i.e., that they had lost the count of their breath), they pressed a button. They then filled a short questionnaire indicating the content of their mind wandering episode and pressed a button to resume the task.
EEG data were recorded using a 64-channel Biosemi Active Two system. These data will not be analyzed in this report. While performing the breath counting task, subjects were also presented with a passive auditory oddball protocol that they were instructed to ignore ( Braboszcz and Delorme, 2011 ). The subjects had to ignore all sounds and stay focused on the breath counting task. The auditory oddball protocol was composed of 70-ms pure sounds at 500 Hz for the standard stimuli (70% of the stimuli) and 70-ms pure sounds at 1000 Hz for the oddball stimuli (30% of the stimuli). Auditory stimuli were presented every second at a volume of 72 dB. The oddball stimuli are related to EEG data recordings and analysis and are not analyzed in this report.
Each session lasted 20 min (excluding the time spent filling out questionnaires). Ten sessions of the 20-min breath-counting task were recorded for each subject. Sessions were scheduled every 2–3 days, excluding week-ends, over a period of 5 weeks.
Ocular Data Recording
Eye movements and pupil size were monitored with an IView Hi-Speed monocular eye tracker (SensoMotoric Instruments, Berlin, Germany) setup on the subject's dominant eye (right eye for both subjects). This infrared tracking system samples eye position at 240 Hz. Before each session, a 13-point calibration was performed using a 1280 by 1024 pixels calibration picture matching the resolution of the screen. During this calibration step, subjects had to follow a white dot moving randomly across the 13-point calibration sequence. Pupil size was estimated in pixel-square using the area-based algorithm provided in IView X software version 1.7. This algorithm estimates pupil area using the diameter of the disk that best matches the pupil digital image.
Preprocessing—Blink and Artifact Detection
When considering eyetracking data, blinks result in zero values in the continuous pupil size signal—since the size of the pupil is equal to zero when eyes are closed. Blinks also usually induce non-zero values artifacts at boundaries, as the eyetracker erratically loses track of the pupil when the eyelid partially covers it. This often results in single spikes, spike bursts and sustained spikes periods, which make the exact blink onset and blink offset detection more difficult. Moreover, these types of artifacts are not only present around blinks but may also occur at random places in eyetracking data. The preprocessing had two goals: on the one hand, detect blinks onset and blinks offset as accurately as possible in order to compute blinks duration; on the other hand detect blinks and artifacts in order to interpolate these data portions.
We designed a two-step artifact removal and blink identification procedure inspired by Pedrotti et al. (2011) : the first step aims to define statistics of the blink-free signal which is used to detect and remove non-blink related artifact and the second step uses a fine grain blink detection procedure to compute blink duration and interpolate signals during blinks. The automated procedure we developed to achieve this is summarized in Figure 2 and detailed below.
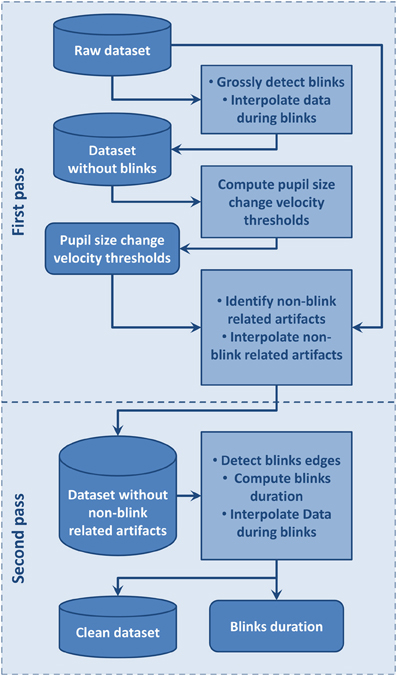
Figure 2. Flow chart of the automated artifacts and blinks detection procedure used to clean data and compute blinks duration .
We first distinguished non-blink-related artifacts from blink-related ones (Figure 2 ). Before applying this procedure, we visually inspected the signal to be sure that no dataset showed periods including artifacts extending over the whole dataset. The blink-free datasets were temporary datasets obtained by removing blink using a conservative procedure where blinks were defined as the period from 100 ms before to 300 ms after a section of pupil-size data equal to 0. This large interval around a blink was used to insure it contained the whole blink period, including the perturbed sections due to eyelid closing or opening, so that pupil size change velocity statistics would not be contaminated by blinks. Blinks separated by a time interval lower than 100 ms were considered as belonging to the same blink and were merged. We then used a pupil size change velocity thresholds specific to each dataset to detect artifacts at blink boundaries such as: lowThreshold = μ − a .σ and highThreshold = μ + a .σ where μ is the mean of pupil size change velocity over the considered dataset, σ its standard deviation, and a a coefficient fixed to 1.5. These thresholds were used on corresponding original datasets where all data points with a pupil size change velocity higher than highThreshold or lower than lowThreshold were flagged as artifact. Artifacts separated by less than 16 ms were merged. Among these artifacts, we then distinguished blink-related artifacts as the artifactual samples situated around zero values. Blink-related artifacts were merged with the blink periods. Non-blink related artifactual values were interpolated in order to avoid interference with the second step where blink onsets and offsets are accurately identified.
For the second step, we used the fine grain blink detection algorithm designed by Engbert and Mergenthaler (2006) . This algorithm is tailored for saccade detection but may also be applied to estimate precisely blink onsets and offsets. After detecting blink onsets and offsets, we then interpolated linearly the data during blinks. Blinks separated by less than 100 ms were then merged as a single blink in line with Hupé et al. (2009) . This fine grain blink detection procedure allows computation of accurate blink duration and other blink statistics. Once these blink and artifactual periods were defined and interpolated, statistics of pupil size change and gaze position were computed to be analyzed along with the behavioral data.
Data Analysis
Epoch selection.
After the data were cleaned, we conducted analyses on pupil size, blinks and gaze position. We extracted mind-wandering epochs as the 10-s interval prior to MW button presses. Focusing (F) epochs were defined as the 10-s interval after re-focusing button presses. In order to avoid overlap between MW epochs and F epochs, we discarded all epochs where time interval between successive F and MW button press events was lower than 30 s. We also used subject's answers to the questionnaire regarding the subjective mind-wandering duration to remove epochs where the mind-wandering occurrence was reported to be less than 2 s. Epochs where the questionnaire indicated that the button was pressed by error were not considered in the analysis. In addition, epochs where the subject failed to press the button to indicate that he or she was restarting the breath-counting task were discarded. Data epochs containing more than 40% of interpolated data points ( Smallwood et al., 2011 ) or blinks with duration greater than 4 s within the analysis intervals were also discarded. This resulted in 121 MW and 113 F clean data epochs for S1 and 88 MW and F clean data epochs for S2.
In order to avoid contamination of the pupil data by peripheral motor effects which are known to increase pupil size ( Hupé et al., 2009 ), we excluded data 1 s before and after button press from the analysis. Thus, for the dependent variables linked to blinks, pupil size, and gaze position, the analysis window was defined as a 9 s period ranging from −10 s to −1 s before mind wandering button press for MW epochs and as a 9 s period ranging from 1 s to 10 s after the re-focusing button press for F epochs.
For each subject we conducted two types of analysis on pupil size data. For the first analysis, we computed the arithmetic mean and standard deviation of the pupil size for each epoch in each mental state (MW and F). A Wilcoxon rank sum test was then used to assess significant differences between the MW and F states. The second analysis compared the median time courses of pupil size during MW and F epochs. For this analysis, median pupil size was computed at each time point across epochs. A linear regression was then computed on the studied time period in order to extract the estimated slope (a) of the pupil size time course and related Pearson coefficient of correlation (r).
First, in order to study if blink duration and blink frequency changed with the time spent performing the task, two measures were derived from blinks. Blink duration was computed based on the fine grain blink detection procedure described in section Preprocessing—Blink and Artifact Detection. Blinks with durations longer than 1.5 s were discarded. For each session, mean blink rate and duration were calculated on the continuous data using 1 min sliding windows with 30 s overlap. Mean blink rate and duration were then averaged for all windows across sessions for each subject. Pearson correlation coefficients between mean blink duration and time spent performing the experiment on one hand, and mean blink rate and time spent performing the experiment on the other hand were calculated for each subject.
Second, a contrast analysis between MW and focusing was conducted on the 9-s epochs preceding MW button press and following re-focusing. Mean blink rate and mean blink duration were computed for each type of epoch. As epochs were only 9 s long, blink rate expressed in blinks per minute was approximated by multiplying the number of blinks within each 9 s epoch by 6.67 (i.e., 60 s divided by 9 s). A Wilcoxon rank sum test was performed on each dependent variable to assess differences between conditions.
Gaze position
We used the gaze position measure returned by IView X software to assess gaze position. The eye tracker used the orbital pupil position—“Mapped Screen Coordinates” under the IView X software—and returned values in terms of pixel location on the stimulus presentation screen. X and Y coordinates are given in pixels relative to the calibration area used during the calibration procedure. We interpolated the data on these two channels during periods where we interpolated the data on the pupil size channel. Mean gaze position and standard deviation for the X and Y gaze position were calculated for the MW and the F using the same procedure described previously for blink rate and blink duration. The same Wilcoxon rank sum test was used to assess differences between the obtained distributions of values computed on MW and F epochs.
Correlation between oculometric data and psychometric measures
We conducted a correlation analysis between oculometric data obtained during the MW episodes and the answers to questionnaires. All non-artifactual data epochs were considered in this analysis.
Oculometric data automatic classification
We conducted additional offline analysis to assess if it was possible to classify data based on blink, gaze position and pupil size at the single trial level.
To further control that we were classifying mind wandering data, we considered a third type of data epochs for the classification algorithm: control epochs (CT) defined as a 9 s interval centered in-between MW button press and previous F button press, with no overlap with MW or F epochs. Following the same epoch selection procedure and applying the same constraints than for MW and F epochs, 121 CT clean control epochs could be extracted for S1 and 88 CT clean epochs could be extracted for S2.
We attempted to classify each pair of epoch type (MW vs. CT; MW vs. F; F vs. CT) for each subject using a linear 2-norm soft-margin Support Vector Machine (SVM) using a Gaussian Radial Basis Function kernel with a scaling factor, sigma, of 1. A box constraint of 0.2 was used for the soft margin. The Quadratic Programming method was used to find the separating hyperplan. Data were shifted to zero mean and scaled to unit variance prior to SVM training. MATLAB (The MathWorks) function svmtrain was used to train the SVM.
Evaluation of the classifier accuracy was done using 10-fold cross-validation, where 90% of the data is used for training and 10% of the data is used for testing the classifier. Moreover, for each dataset, we performed the 10-fold cross-validation 100 times—with different partition of the data—to obtain 100 accuracy values, which were used to estimate mean accuracy.
We performed classification on a multivariate dataset composed of 9 variables computed on MW, F, and CT 9 s epochs: “Pupil Size Slope,” “Pupil Size Mean,” “Pupil Size Std,” “Blink Rate,” “Blink Duration Mean,” “Gaze X Position Mean,” “Gaze X Position Std,” “Gaze Y Position Mean,” “Gaze Y Position Std.” We first computed the classification accuracy using the combined variables. We also performed classification on each of these variables independently.
Mind Wandering Behavioral Data
Descriptive statistics of mind-wandering episodes for each subjects are shown in Supplementary Table S1 . The detail of each session is reported along with the average and standard deviation across sessions. Subject 1 noticed and signaled a total of 264 mind-wandering episodes, whereas subject 2 reported only 160 such episodes. The number of questionnaires indicating unwanted button presses was low for both subjects, with 4 for subject S1, and only 1 for S2. The average MW rate was of one MW occurrence every 45 ± 8 s (mean ± SD ) for subject 1 and 73 ± 14 s for subject 2.
When comparing the 9-s period following the task refocusing button press with the 9-s period before the mind-wandering button press, Wilcoxon rank tests indicated a significant difference in both pupil size slope and mean pupil size. For both subjects, pupil sizes were significantly smaller during the MW period than during the F period. For both subjects, pupil slope was more negative during the F period than during the MW period. Results are summarized in Table 1 . Figure 3 shows the average time course of median pupil size across trials for each subject for the F and the MW periods.
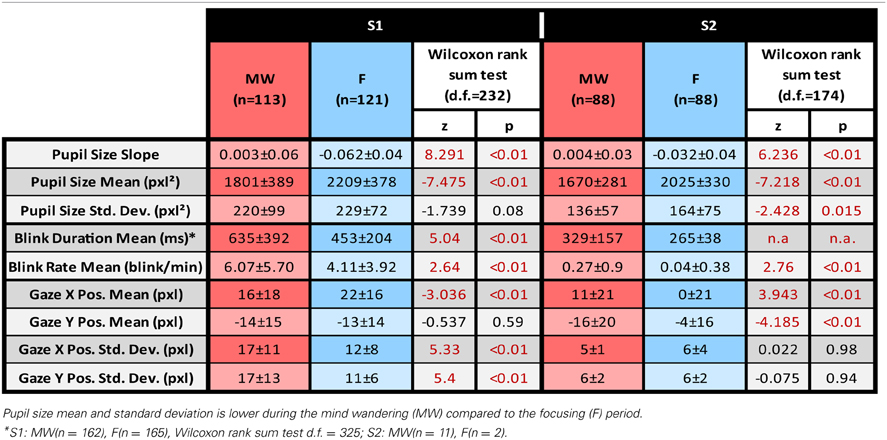
Table 1. Mean ± Standard Deviation for each dependent variable .
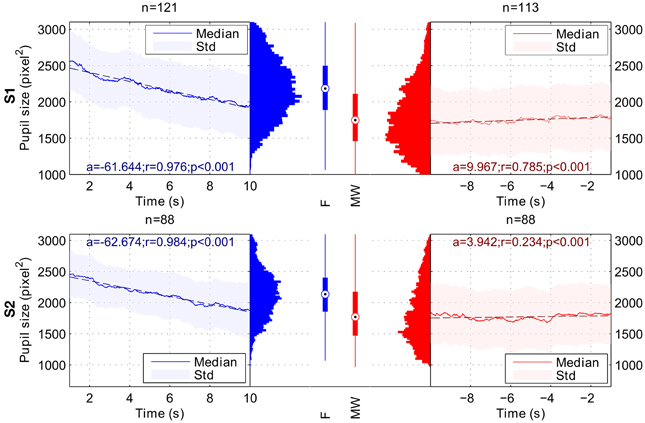
Figure 3. Variation of pupil size during Focusing (F; in blue) and Mind Wandering periods (MW; in red). Results for subject 1 are shown on the top row (S1) , and results for subject 2 on the bottom row (S2) . a, r and p -values stand respectively for slope, Pearson's correlation coefficient and p -value of the linear regression for the considered time intervals. Linear regressions are represented in dashed lines. In the Focusing period, we observed a significant decrease in terms of pupil size. In the Mind Wandering period, we observed a significant increase in terms of pupil size for both subjects. Global pupil size averaged over time for each interval shows a lower median for MW period.
Blink Frequency
Using Wilcoxon rank sum test, we observed a significant increase in blink rate for the mind wandering period compared to the focusing periods for both S1 ( z = 2.64, df = 232, p = 0.008) and S2 ( z = 2.76, df = 174, p = 0.006). Blinks were also longer during mind wandering periods compared to focusing periods for both subjects but statistics could only be computed for subject 1 considering the low number of blinks for subject 2 (S1 z = 5.04, df = 325, p < 10 −6 ). Statistical results are detailed in Table 1 .
Unlike previous reports ( Van Orden et al., 2000 ; Schleicher et al., 2008 ), we did not observe a clear blink rate increase as subjects spent more time performing the task. A slight increase was found for S1 but it was only marginally significant with a low correlation coefficient (S1, slope = 0.032 blinks per minute, r (2) = 0.1, p = 0.049). The increase was not significant for S2 (S2, slope = 0.0002 blinks per minute, r (2) < 0.001, p = 0.98). Blink duration was constant over time for S1 but a weak, albeit significant, correlation between blink duration and time spent on task was found for S2 (slope = 0.00013, r (2) = 0.036, p = 0.007).
Gaze Position
We tested if gaze position changed as a function of mind wandering or focusing. Figure 4 represents gaze position density maps computed on mind wandering and focusing epochs. Results are summarized in Table 1 .
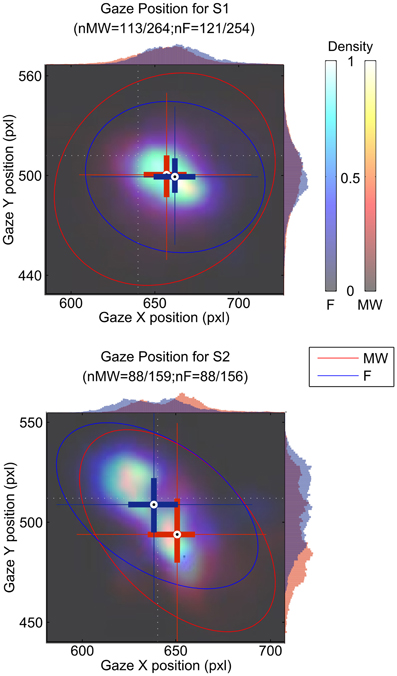
Figure 4. Gaze position during the focusing period (blue cross and histogram) and the mind wandering period (red cross and histogram). Gaze position is indicated in pixel (pxl) on both the x and the y axis. The position of the cross indicates the center of gravity for all blink positions for the mind wandering and for the focusing conditions. Ellipses indicate 95% confidence intervals. Histograms of position on the x- and y-axes are also shown on the top and right side of each plot. The light dotted white lines indicate the position of the fixation cross.
Differences were found both in terms of horizontal and vertical position of the gaze. X gaze position was significantly different between the MW and F periods for both S1 ( z = −3.04, df = 232, p = 0.002) and S2 ( z = 3.94, df = 174, p < 10 −4 ). Difference in Y gaze position between MW and F epochs was only significant for S2 ( z = −4.19, df = 174, p < 10 −4 ).
Classification Results
Classification results for the two subjects are summarized in Table 2 . Oculometric information used for classification has been ordered according to the averaged accuracy reached when classifying MW vs. F epochs. Classification using the 10 combined oculometric measures considered in Table 2 gave the best results with accuracy over 75% for both subjects when classifying MW vs. F and F vs. CT. Pupil size mean, and Pupil size slope led to the best results for univariate classification of MW vs. F, and CT vs. F. Interestingly, the combined measures provided higher classification accuracy than the best univariate classification for S1, but not for subject S2 for which, the Pupil Size Mean measure alone was better than the combined measures. This could indicate over fitting when using the combined measures. When considering MW vs. CT epochs, performances were close to chance level.
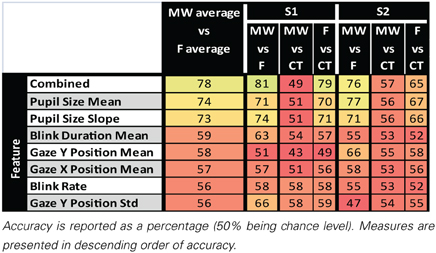
Table 2. Results of pairwise classification of MW, CT, and F epochs for single oculometric measure and combined oculometric measures .
Questionnaire Results
Supplementary Figure 3 summarizes the answers of each subject to the questionnaire. We observed that for both subject the majority of mind wandering reports concerned the occurrence of thoughts simultaneous to the execution of the breath-counting task. Most often, there was no time lag between the occurrence of the mind wandering event and its report by the subject. The majority of mind wandering-episodes were reported to last for approximately 10 s.
Regarding thought content of MW episodes, for both subjects the majority of thoughts involved both mental images and inner dialog, and where most often related to events taking place in the surrounding environment. The majority of thought content concerned namely thoughts relative to the task, or the passive observation of a given situation. Thoughts were only rarely related to events taking place far in the past or far in the future.
The emotional content of MW episodes was mostly either slightly negative or neutral. The subject's level of vigilance was mostly either normal (calm) or lower than normal (drowsiness). Subjects differed in the quality of the feelings they reported, with subject 1 experiencing more discomfort than subject 2, while subject 2 seemed more tired than subject 1.
Overall, these preliminary observations provide insights into the characteristics of mind wandering recorded in two subjects during a breath counting task. Collecting data on more subjects would be necessary to draw more general conclusions about the content of mind wandering.
The purpose of collecting this psychometric data was to conduct a correlation analysis between subjects' answers and oculometric measures. Results are summarized in Figure 5 .
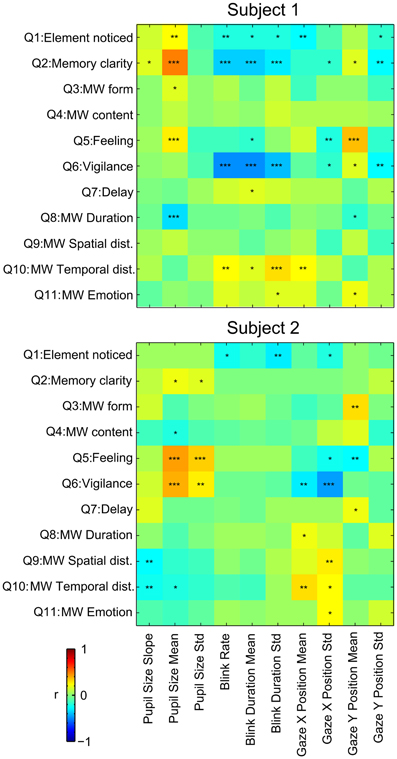
Figure 5. Correlation between answers to the questionnaires and oculometric measures during the MW epochs. Pearson's r is reported by color of each cell. * p ≤ 0.05, ** p ≤ 0.01, *** p ≤ 0.001.
Memory clarity (Q1) of the mind wandering content and feeling of well being (Q5) were positively correlated with mean pupil size during MW epochs for both subjects. Memory clarity (Q5) and Vigilance (Q6) were inversely correlated with blink rate and duration for subject 1. Absence of results for subject 2 on these measures could be due to the low number of blinks for this subject. X gaze position was positively correlated with the temporal distance (Q10) for both subjects. Most other significant correlations observed were idiosyncratic, and different in the two subjects.
We studied spontaneous mind-wandering episodes in two subjects. To accumulate enough mind wandering episodes, each subject performed 10 sessions of 20 min each over the course of about 5 weeks. Albeit challenging in terms of data collection, this study is a proof of concept that studying self-reported mind wandering episodes along with psychometric data is a valid scientific protocol and may yield interesting results.
In this pilot study, we have shown that mind wandering correlates with pupil size, gaze position and blink rate. We showed a significant decrease in pupil size during mind wandering. Consistent with our result, Van Orden showed that, in line with reports by Lowenstein and Loewenfeld (1962) , Yoss et al. (1970) and McLaren et al. (1992) , pupil diameter was typically smaller during periods of increased error.
Previous work has shown that pupil baseline diameter increased during periods where mind wandering was more likely to occur ( Smallwood et al., 2011 ), which is the opposite of what was observed in our data. However, Smallwood's task ( 2011 ) involved paying attention to external visual cues and was based on a time-locked paradigm. It is possible that the increase in pupil size in Smallwood's task corresponds to a regulatory mechanism to favor processing of external stimuli during mind wandering. Moreover, their analysis is based on much shorter time windows (a 3–4 s) locked on stimuli. The task in our study does not involve monitoring of visual stimuli. This difference in task could explain the difference in terms of pupil dilation we observed compared to Schooler et al. (2011) .
It has also been shown in previous studies that sleepiness results in pupil size decreasing with time ( Lowenstein and Loewenfeld, 1962 ; Yoss et al., 1970 ). The gradual decrease of the average pupil size can also be related to several factors: time on task and decrease of the cognitive load. Additional data and analyses would be needed to show that the small changes in pupil size induced by mind wandering in our task are independent of these factors.
We observed an increase in the number of blinks per minute during mind wandering periods in both subjects. A previous study on mind wandering and blink rate during a reading task by Smilek et al. (2010) showed that mind wandering periods contain more blinks than on-task periods. These results and ours are consistent with the hypothesis that blinks might be involved in trade-off modulation between attention to thoughts produced during mind wandering, and attention to task-related stimuli.
We observed a different average location for the gaze of both S1 and S2 during the mind wandering compared to the focusing period. While the position of the gaze was on the fixation cross for S2 (Figure 4 ), S1 appeared to be fixating on average on a different point while focusing. It is unclear if this arose because of problems in the software calibration or if S1 actually shifted his gaze during the task. Note that the shift in position only correspond to about 10 pixels, which only represent about 2 mm on the computer screen.
We also observed increased eye movement amplitude as indexed by the standard deviation of gaze position during mind wandering. Consistent with this result, using a reading task, Reichle et al. (2010) observed erratic eye movements immediately preceding self-caught mind wandering episodes. However, they also report longer fixation periods, which could influence the standard deviation of the gaze position during focusing periods. Uzzaman and Joordens (2011) also showed that overall eye movements were slower and less frequent during reports of probe-caught mind wandering. In another study, Smilek et al. (2010) showed that during extended periods of reading, mind wandering episodes contain fewer fixations on the reading material. These results argue in favor of the theoretical claim that the cognitive processes that normally influence eye movements to enhance semantic processing during reading exert less control during mindless reading. Our results are consistent with this hypothesis that control of gaze stability seems to be less efficient during MW.
The type of task to perform may also influence eye movements during mind wandering. When performing a driving task that required visual exploration, He et al. (2011) observed that, while mind wandering, participants tended to focus visual attention more narrowly on the road, which is opposite of what we observed. However, in all studies, the primary task and its oculomotor characteristics, being complex eye movements for reading, wide ocular movements for visual exploration, or on the contrary gaze fixation while counting breath, seem to be executed in a less efficient way, which is consistent with the decreased subjective involvement with the external world during mind wandering.
Note that this last hypothesis is consistent with the decoupling hypothesis where two attentional states are distinguished: the offline and online mental mode resulting from a competition between internal and external information streams for access to a global workspace ( Dehaene and Naccache, 2001 ). During the “online mode,” attention is turned toward external task relevant information, whereas during the “offline mode” attention is turned inward, favoring the inner stream of information and diminishing external information access to the global mental workspace.
Classification
For both subjects, we observed high classification accuracy of MW, CT, and F epochs using a variety of oculometric measures, pupil size leading to the highest classification performance. However, it is interesting to note that in all cases, classification accuracy of MW vs. F was greater than classification accuracy of F vs. CT, which was itself greater than classification accuracy of MW vs. CT. It thus seems that MW epochs are more similar to CT epochs than to F epochs as classification performances of MW vs. CT are closer to chance level. This might be due to the fact that despite the selection of control epochs in between F and MW events, mind-wandering could have already started in CT epochs. Moreover, definition of MW epochs relies on subjects' capacity to self-detect and self-report their own mind wandering. Therefore, undetected or unreported mind wandering can occur during the considered CT periods. It is worth mentioning that this classification scheme is far from real-time detection of mind wandering occurrence as classification is not done taking random signal epochs or using a sliding time window. However, these results still show that it is possible to use oculometric data to distinguish between focusing or mind wandering data epochs.
Limits to the Self-Paced Mind Wandering Protocol
We want to emphasize that, in our protocol, we currently have no way to know if a subject reports less mind wandering episodes because he experiences less mind wandering episodes or because he is less sensitive in detecting mind wandering episodes. The mean mind wandering detection rate across subjects is of about one mind wandering occurrence per minute but shows high variability (mean: 54 ± 38 s). Consistent with our results, Hasenkamp et al. (2012) reported an average of one mind wandering episode signaled every 80 s for the same task duration of 20 min.
In our paradigm, it is also difficult to assess the exact onset of mind wandering periods, which may be variable from one trial to the other. As a consequence, trial averaging is also likely to result in the masking of some temporal structure of mind wandering. Thus, we are mainly studying the offset of mind wandering episodes, as indicated by subjects' button presses.
While this study is limited in scope since it corresponds to data from only two subjects, it demonstrates that oculometric data can potentially be used as an marker of mind wandering. It also shows that collecting multiple sessions in few subjects may yield reliable and meaningful results for mind wandering research.
Conflict of Interest Statement
The authors declare that the research was conducted in the absence of any commercial or financial relationships that could be construed as a potential conflict of interest.
Acknowledgments
This work was supported by a thesis fellowship from the French ministry of research, a grant from the FRM Foundation and a grant from ANR. The authors wish to thank Tracy Brandmeyer for her helpful comments on the manuscript.
Supplementary Material
The Supplementary Material for this article can be found online at: http://www.frontiersin.org/journal/10.3389/fpsyg.2014.00031/abstract
Supplementary Figure 1. Instruction screen displayed at the beginning of each session.
Supplementary Figure 2. Questionnaire used to collect phenomenological data. A sample questionnaire, identical to the questionnaire actually used during the session, was displayed prior to the session start, just after the instruction screen presented in Supplementary Figure 1 . Subjects could familiarize with the Graphical User Interface (GUI) and the content of the questionnaire as long as they wanted before starting the experiment session. The questionnaire was filled by pressing buttons on the GUI. The “Cancel” button was used in case the subject clicked on left mouse button by mistake. Button are turned green when clicked. Validate button is displayed only when the subject answered all questions.
Supplementary Figure 3. Questionnaire answers' statistics summed over the 10 sessions (nS1 = 264, nS2 = 160).
Supplementary Table 1. Descriptive statistics computed on mind-wandering occurrences for each subject, and averaged across subjects. The number of mind-wandering events in this table do not include questionnaires where the subject pressed the cancel button.
Baars, B. J. (1993). A Cognitive Theory of Consciousness . Cambridge: Cambridge University Press.
Baars, B. J. (1997). Some essential differences between consciousness and attention, perception, and working memory. Conscious. Cogn . 6, 363–371. doi: 10.1006/ccog.1997.0307
CrossRef Full Text
Braboszcz, C., and Delorme, A. (2011). Lost in thoughts: neural markers of low alertness during mind wandering. Neuroimage 54, 3040–3047. doi: 10.1016/j.neuroimage.2010.10.008
Pubmed Abstract | Pubmed Full Text | CrossRef Full Text
Brainard, D. H. (1997). The psychophysics toolbox. Spat. Vis . 10, 433–436. doi: 10.1163/156856897X00357
Carter, M. E., Yizhar, O., Chikahisa, S., Nguyen, H., Adamantidis, A., Nishino, S., et al. (2010). Tuning arousal with optogenetic modulation of locus coeruleus neurons. Nat. Neurosci . 13, 1526–1533. doi: 10.1038/nn.2682
Christoff, K., Gordon, A. M., Smallwood, J., Smith, R., and Schooler, J. W. (2009). Experience sampling during fMRI reveals default network and executive system contributions to mind wandering. Proc. Natl. Acad. Sci. U.S.A . 106, 8719. doi: 10.1073/pnas.0900234106
Dehaene, S., and Naccache, L. (2001). Towards a cognitive neuroscience of consciousness: basic evidence and a workspace framework. Cognition 79, 1–37. doi: 10.1016/S0010-0277(00)00123-2
Engbert, R., and Mergenthaler, K. (2006). Microsaccades are triggered by low retinal image slip. Proc. Natl. Acad. Sci. U.S.A .103, 7192–7197. doi: 10.1073/pnas.0509557103
Gruberger, M., and Zangen, A. (2011). Towards a neuroscience of mind-wandering. Front. Hum. Neurosci . 5:56. doi: 10.3389/fnhum.2011.00056
Hasenkamp, W., Wilson-Mendenhall, C. D., Duncan, E., and Barsalou, L. W. (2012). Mind wandering and attention during focused meditation: a fine-grained temporal analysis of fluctuating cognitive states. Neuroimage 59, 750–760. doi: 10.1016/j.neuroimage.2011.07.008
He, J., Becic, E., Lee, Y.-C., and McCarley, J. S. (2011). Mind wandering behind the wheel performance and oculomotor correlates. Hum. Factors 53, 13–21. doi: 10.1177/0018720810391530
Hupé, J.-M., Lamirel, C., and Lorenceau, J. (2009). Pupil dynamics during bistable motion perception. J. Vis . 9:10. doi: 10.1167/9.7.10
Koch, C., and Tsuchiya, N. (2007). Attention and consciousness: two distinct brain processes. Trends Cogn. Sci . 11, 16–22. doi: 10.1016/j.tics.2006.10.012
Lowenstein, O., and Loewenfeld, I. E. (1962). “The pupil,” in The Eye , ed H. Davson (New York, NY: Academic Press), 231–267.
McLaren, J. W., Erie, J. C., and Brubaker, R. F. (1992). Computerized analysis of pupillograms in studies of alertness. Invest. Ophthalmol. Vis. Sci . 33, 671–676.
Pubmed Abstract | Pubmed Full Text
Pedrotti, M., Lei, S., Dzaack, J., and Rötting, M. (2011). A data-driven algorithm for offline pupil signal preprocessing and eyeblink detection in low-speed eye-tracking protocols. Behav. Res . 43, 372–383. doi: 10.3758/s13428-010-0055-7
Pelli, D. G. (1997). The VideoToolbox software for visual psychophysics: transforming numbers into movies. Spat. Vis . 10, 437–442. doi: 10.1163/156856897X00366
Posner, M. I. (1994). Attention: the mechanisms of consciousness. Proc. Natl. Acad. Sci. U.S.A . 91, 7398–403. doi: 10.1073/pnas.91.16.7398
Posner, M. I., and Rothbart, M. K. (1998). Attention, self-regulation and consciousness. Philos. Trans. R. Soc. Lond. B Biol. Sci . 353, 1915–27. doi: 10.1098/rstb.1998.0344
Reichle, E. D., Reineberg, A. E., and Schooler, J. W. (2010). Eye movements during mindless reading. Psychol. Sci . 21, 1300–1310. doi: 10.1177/0956797610378686
Schleicher, R., Galley, N., Briest, S., and Galley, L. (2008). Blinks and saccades as indicators of fatigue in sleepiness warnings: looking tired? Ergonomics 51, 982–1010. doi: 10.1080/00140130701817062
Schooler, J. W., Smallwood, J., Christoff, K., Handy, T. C., Reichle, E. D., and Sayette, M. A. (2011). Meta-awareness, perceptual decoupling and the wandering mind. Trends Cogn. Sci . 15, 319–326. doi: 10.1016/j.tics.2011.05.006
Simons, D. J., and Chabris, C. F. (1999). Gorillas in our midst: sustained inattentional blindness for dynamic events. Perception 28, 1059–1074. doi: 10.1068/p2952
Smallwood, J., Beach, E., Schooler, J. W., and Handy, T. C. (2008). Going AWOL in the brain: mind wandering reduces cortical analysis of external events. J. Cogn. Neurosci . 20, 458–469. doi: 10.1162/jocn.2008.20037
Smallwood, J., Brown, K. S., Tipper, C., Giesbrecht, B., Franklin, M. S., Mrazek, M. D., et al. (2011). Pupillometric evidence for the decoupling of attention from perceptual input during offline thought. PLoS ONE 6:e18298. doi: 10.1371/journal.pone.0018298
Smallwood, J., and Schooler, J. W. (2006). The restless mind. Psychol. Bull . 132, 946–958. doi: 10.1037/0033-2909.132.6.946
Smilek, D., Carriere, J. S. A., and Cheyne, J. A. (2010). Out of mind, out of sight: eye blinking as indicator and embodiment of mind wandering. Psychol. Sci . 21, 786–789. doi: 10.1177/0956797610368063
Smith, R., Keramatian, K., Smallwood, J., Schooler, J., Luus, B., and Christoff, K. (2006). “Mind-wandering with and without awareness: an fMRI study of spontaneous thought processes,” in Proceedings of the 28th Annual Conference of the Cognitive Science Society , ed R. Sun, (Vancouver: Erlbaum), 804–809.
Usher, M., Cohen, J. D., Servan-Schreiber, D., Rajkowski, J., and Aston-Jones, G. (1999). The role of locus coeruleus in the regulation of cognitive performance. Science 283, 549–554. doi: 10.1126/science.283.5401.549
Uzzaman, S., and Joordens, S. (2011). The eyes know what you are thinking: eye movements as an objective measure of mind wandering. Conscious. Cogn . 20, 1882–1886. doi: 10.1016/j.concog.2011.09.010
Van Orden, K. F., Jung, T.-P., and Makeig, S. (2000). Combined eye activity measures accurately estimate changes in sustained visual task performance. Biol. Psychol . 52, 221–240. doi: 10.1016/S0301-0511(99)00043-5
Yoss, R. E., Moyer, N. J., and Hollenhorst, R. W. (1970). Pupil size and spontaneous pupillary waves associated with alertness, drowsiness, and sleep. Neurology 20, 545–545. doi: 10.1212/WNL.20.6.545
Keywords: mind wandering, pupil size, blinks, gaze position, classification
Citation: Grandchamp R, Braboszcz C and Delorme A (2014) Oculometric variations during mind wandering. Front. Psychol . 5 :31. doi: 10.3389/fpsyg.2014.00031
Received: 13 June 2013; Accepted: 10 January 2014; Published online: 11 February 2014.
Reviewed by:
Copyright © 2014 Grandchamp, Braboszcz and Delorme. This is an open-access article distributed under the terms of the Creative Commons Attribution License (CC BY) . The use, distribution or reproduction in other forums is permitted, provided the original author(s) or licensor are credited and that the original publication in this journal is cited, in accordance with accepted academic practice. No use, distribution or reproduction is permitted which does not comply with these terms.
*Correspondence: Romain Grandchamp, Centre de Recherche Cerveau et Cognition, CNRS, UMR 5549, Pavillon Baudot CHU Purpan, BP 25202, 31052 Toulouse, France e-mail: [email protected]
Disclaimer: All claims expressed in this article are solely those of the authors and do not necessarily represent those of their affiliated organizations, or those of the publisher, the editors and the reviewers. Any product that may be evaluated in this article or claim that may be made by its manufacturer is not guaranteed or endorsed by the publisher.
Click through the PLOS taxonomy to find articles in your field.
For more information about PLOS Subject Areas, click here .
Loading metrics
Open Access
Peer-reviewed
Research Article
Pupil Diameter Tracks Lapses of Attention
* E-mail: [email protected]
Affiliations Institute of Psychology, Leiden University, Leiden, the Netherlands, Leiden Institute for Brain and Cognition (LIBC), Leiden, the Netherlands

Affiliations Institute of Psychology, Leiden University, Leiden, the Netherlands, Leiden Institute for Brain and Cognition (LIBC), Leiden, the Netherlands, Department of Neurophysiology and Pathophysiology, University Medical Center Hamburg-Eppendorf, Hamburg, Germany
- Ruud L. van den Brink,
- Peter R. Murphy,
- Sander Nieuwenhuis
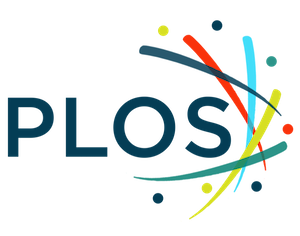
- Published: October 21, 2016
- https://doi.org/10.1371/journal.pone.0165274
- Reader Comments
Our ability to sustain attention for prolonged periods of time is limited. Studies on the relationship between lapses of attention and psychophysiological markers of attentional state, such as pupil diameter, have yielded contradicting results. Here, we investigated the relationship between tonic fluctuations in pupil diameter and performance on a demanding sustained attention task. We found robust linear relationships between baseline pupil diameter and several measures of task performance, suggesting that attentional lapses tended to occur when pupil diameter was small. However, these observations were primarily driven by the joint effects of time-on-task on baseline pupil diameter and task performance. The linear relationships disappeared when we statistically controlled for time-on-task effects and were replaced by consistent inverted U-shaped relationships between baseline pupil diameter and each of the task performance measures, such that most false alarms and the longest and most variable response times occurred when pupil diameter was both relatively small and large. Finally, we observed strong linear relationships between the temporal derivative of pupil diameter and task performance measures, which were largely independent of time-on-task. Our results help to reconcile contradicting findings in the literature on pupil-linked changes in attentional state, and are consistent with the adaptive gain theory of locus coeruleus-norepinephrine function. Moreover, they suggest that the derivative of baseline pupil diameter is a potentially useful psychophysiological marker that could be used in the on-line prediction and prevention of attentional lapses.
Citation: van den Brink RL, Murphy PR, Nieuwenhuis S (2016) Pupil Diameter Tracks Lapses of Attention. PLoS ONE 11(10): e0165274. https://doi.org/10.1371/journal.pone.0165274
Editor: Ramesh Kumar Mishra, University of Hyderabad, INDIA
Received: June 6, 2016; Accepted: October 10, 2016; Published: October 21, 2016
Copyright: © 2016 van den Brink et al. This is an open access article distributed under the terms of the Creative Commons Attribution License , which permits unrestricted use, distribution, and reproduction in any medium, provided the original author and source are credited.
Data Availability: The authors confirm that all data underlying the findings are fully available without restriction. All raw data are deposited in the Dryad Digital Repository (doi: 10.5061/dryad.mp332 ).
Funding: This research was supported by a Consolidator Grant of the European Research Council (grant number GA 283314-NOREPI) ( https://erc.europa.eu/ ).
Competing interests: The authors have declared that no competing interests exist.
Introduction
The ability to sustain attention for prolonged periods of time is essential for normal functioning in everyday life. Lapses of attention can have dramatic consequences, such as when a car driver is absent-minded and brakes too late in response to an unexpected traffic backup, or when an air traffic controller fails to spot that two aircraft are about to cross paths. Physiological markers that indicate when such lapses of attention are more likely to occur could yield insight into the cognitive mechanisms that underlie attentional lapses, as well as provide preventative measures. A potentially useful physiological marker for detecting lapses of attention might be pupil diameter. The diameter of the pupil has long been known as a marker of cognitive load and attentional performance [ 1 , 2 ]. More recently, some researchers have considered endogenous (‘baseline’) variability in pupil diameter as an indicator of fluctuations in attentional control state (e.g., [ 3 – 5 ]).
Despite the potential utility of pupil diameter as a marker of attentional engagement, the available studies in which the relationship between baseline pupil diameter and sustained attentional performance has been investigated display a remarkable lack of consistency. Some researchers have reported that moments of poor task performance or off-task thought are associated with larger baseline diameter [ 6 – 9 ]. Others have reported that poor task performance is associated with smaller baseline diameter [ 10 – 14 ], or is preceded by a progressive decline in pupil diameter [ 10 , 15 ]. Finally, some studies have found that poor task performance can be accompanied by both relatively small and relatively large baseline diameter [ 7 , 9 , 15 , 16 ]. Several theoretical and methodological factors may be responsible for this discrepancy. For instance, the studies reviewed here differed considerably with regard to the measures they used to assess attentional performance: response time (RT; [ 7 , 8 ]); a proportion of slowest responses [ 9 , 12 ]; variability in RTs [ 15 ]; perceptual sensitivity [ 11 , 17 , 18 ]; and self-reported off-task thought [ 6 , 10 , 13 ].
Another factor that may contribute to the lack of consistency in this literature concerns time-on-task effects. Prolonged task performance often results in decrements in attentional performance due to reduced vigilance [ 19 , 20 ], and concurrent changes in pupil diameter [ 9 , 11 , 14 ]. For instance, Hopstaken and colleagues found a progressive decrease in both baseline diameter and perceptual sensitivity with prolonged performance of an N-back task [ 11 ]. A similar decline in both pupil diameter and performance was reported by Van Orden and colleagues, using a sustained attention task [ 14 ]. However, in other studies time-on-task has been reported to lead to contrasting effects on pupil diameter and task performance. For instance, Murphy and colleagues found a progressive increase over time in baseline diameter during an oddball task and a trend towards poorer performance over time [ 15 ]. Beatty reported a decrement over time in perceptual sensitivity during an oddball task, but no change in baseline diameter [ 17 ]. These time-on-task effects are often not taken into account when assessing the relationship between pupil diameter and performance (but see Kristjansson et al. [ 12 ] and Mathôt et al. [ 21 ] for notable exceptions). Thus, depending on the behavioral task and context, shared effects of time-on-task could in principle impose a relationship between diameter and task performance, or obscure a more nuanced relationship.
An example of such a nuanced relationship is the Yerkes-Dodson law, the phenomenon that performance often varies as an inverted-U function of arousal, such that both under- and over-arousal are associated with poor performance [ 22 ]. Aston-Jones and Cohen [ 3 ], in their adaptive gain theory, proposed that this relationship reflects the effects of neuromodulation originating from the locus coeruleus-norepinephrine (LC-NE) system. The LC is a small nucleus in the pontine tegmentum that collateralizes broadly and supplies NE to almost the entire brain [ 3 , 23 ]. Over longer time periods, the level of baseline activity of LC neurons fluctuates with task performance. Intermediate levels of baseline LC activity are associated with (near-)optimal performance, whereas shifts toward either end of the baseline activity continuum are associated with declining performance [ 3 , 24 – 26 ]. Notably, activity in the LC has been reported to correlate with the size of the pupil [ 3 , 27 – 29 ]. Thus, taken together, this framework predicts that both periods of small baseline diameter and periods of large baseline diameter should be associated with impaired attentional performance. Unfortunately, most studies so far have been confined to categorical comparisons of pupil diameter between on-task and off-task thought, or fast and slow response times, without taking nonlinear relationships into consideration.
Thus, in the present study we carried out a detailed investigation of the interrelationships between performance on a sustained attention task, slow baseline fluctuations in the diameter of the pupil, and the effects of time-on-task on both these variables. In contrast to some previous studies, we assessed these relationships at a within-participant, moment-by-moment level, using multiple measures of attentional state. This approach requires large numbers of trials, which in many studies is made difficult by the fact that short intertrial intervals can lead to contamination of pre-trial baseline pupil measurements by task-related pupil dilations on the previous trial. Here, we overcame this challenge by using a fast-paced, isoluminant, gradual-onset continuous performance task [ 30 ] to minimize stimulus-evoked pupil dilations, and by regressing out the remaining task-related transient pupil dilations.
Our results show that attentional performance and baseline diameter progressively declined over time, resulting in strong linear relationships between these variables. However, when we controlled for time-on-task, the relationships between task performance and pupil diameter became U-shaped, consistent with the Yerkes-Dodson law and the adaptive gain theory of LC function [ 3 ]. Moreover, we explored the relationship between performance and changes in pupil size quantified as the temporal derivative of baseline diameter. This measure was inspired by prior work in rodents, showing that the derivative of pupil diameter tracks changes in cortical state and signal detection performance [ 16 , 31 ]. As opposed to baseline diameter, its derivative showed a linear relationship with behavioral performance that was robust to the effect of time-on-task.
Materials and Methods
Participants.
A total of 30 right-handed individuals took part in the study. Two participants were excluded due to technical difficulties with the eye tracker, resulting in a final N of 28 (mean age: 20.9; SD 2.5; min/max 18–26; 6 male). Exclusion criteria included a history of psychiatric disorders or wearing glasses. All participants gave written informed consent prior to the experiment and were compensated with €7,50 or course credit. The study was approved by the Leiden University Institutional Review Board (IRB).
We used a modified version of the gradual continuous performance task (gradCPT) described by Esterman et al . [ 30 ]. Participants were asked to respond to images of cities by pressing the space bar and withhold a response when presented with an image of a mountain ( Fig 1a ). City trials were more frequent (90% of trials) than mountain trials (10%). The images subtended approximately 6 degrees of visual angle, were isoluminant, grayscale, and were presented on a black background. The images linearly and continuously morphed from one into the next, with an 800-ms interval between 100% coherence levels (stimulus onset asynchrony, SOA). This was done to provide a task context in which the participant had to continuously monitor the stimulus stream, and thus could not take ‘mini breaks’ in between trials. To allow the pupil to normalize, the first and last seven images in each block were scrambled. On these trials the participant did not respond and these trials were not included in any of the analyses.
- PPT PowerPoint slide
- PNG larger image
- TIFF original image
a) Gradual continuous performance task. Each block started and ended with 7 scrambled images. The participant was asked to respond to city scenes but not to mountain scenes. Each image continuously morphed into the next, with an 800-ms interval between 100% coherence levels. b) Behavioral results. Data are smoothed for display only. All measures showed a significant linear increase with time-on-task, p- values are listed in the lower right corner of each panel. Error bars represent the SEM.
https://doi.org/10.1371/journal.pone.0165274.g001
Participants were first familiarized with the environment and task by passively viewing all images, without continuous transitions. Then, they practiced the task for 34 trials at ~45% of the normal speed, and then for another 75 trials at the regular speed. Each participant performed a total of 3 blocks of 600 trials (~8 minutes each) per block. Participants took a forced break of at least 5 minutes between blocks and were offered a small snack (chocolate chip cookie) during this interval. The total duration of the experiment was approximately 40 minutes.
Measures of task performance
Hits (responses to cities) and correct rejections (withheld responses to mountains) were considered as correct trials. Misses constituted withheld responses to cities. Within the context of continuous performance tasks, lapses of attention are usually defined as false alarms [ 30 , 32 ]. In our study false alarms corresponded to responses to mountains. However, as noted in the introduction, a variety of other measures have been used to infer attentional state. Therefore, besides false alarm rate, we included three additional performance metrics: 1) the proportion of trials that fell within the slowest quintile of RTs within the block; 2) average RT; and 3) the RT coefficient of variation (RTCV)—that is, the standard deviation of RT divided by the block mean RT.
RTs were measured relative to the onset of each stimulus. For example, an RT of 640 ms (i.e., 80% of the SOA) indicated that the participant responded when the displayed image consisted of 80% trial n , and 20% trial n -1. An iterative algorithm assigned responses to trials in the case of multiple responses, or unusually fast responses (before 70% coherence of trial n ) and unusually slow responses (after 40% coherence of trial n +1). First, the number of correct responses was optimized. Then, ambiguous responses were assigned to a neighboring trial if either of them had no response. If both had a response, it was assigned to the closest city (go) trial. Lastly, if a trial was assigned multiple responses, the fastest response was selected. This procedure was identical to the one described by Esterman et al . [ 30 ], and is unlikely to have substantially influenced the results, given that ambiguous responses were relatively rare (<4% of the trials).
Pupillometry
Participants were seated in a dimly lit room with their head stabilized by a chin rest. During the task participants were asked to keep their eyes focused on a small white fixation dot in the center of the image. We measured the diameter of the right pupil at a sampling rate of 1 kHz with an EyeLink 1000 eye tracker. Prior to the start of each block the eye tracker was calibrated and validated with a 9-point fixation routine.
Moments when the eye tracker received no pupil signal (e.g., during blinks) were marked automatically during data acquisition by the manufacturer’s blink detection algorithm. Afterwards, an iterative algorithm detected additional moments of poor signal quality (e.g., due to partial occlusion of the pupil by the eyelashes). For 200 iterations over the entire signal time series for a given participant and block, any sample for which the difference in pupil diameter compared to the previous sample exceeded a threshold was marked as 0. The default threshold was set to 25 pixels, but the threshold was individually-tailored for participants for whom the algorithm failed to identify sharp spikes in the data or inappropriately marked clean sections of data. All marked data sections were then interpolated across using shape-preserving piecewise cubic interpolation. On average 6.8% (SD 4.6, min/max 0.2/18.7) of the data points were interpolated. After interpolation each pupil time series was low-pass filtered at 6 Hz to remove any residual high-frequency noise.
Finally, we used multiple linear regression to remove the stimulus- and response-related phasic dilations ( Fig 2 ) from the unsegmented pupil time series. This procedure minimized the extent to which phasic pupil dilations convoluted the estimates of tonic variations in the diameter of the pupil. Note that this approach is highly similar to analysis of the first-stage general linear model of functional magnetic resonance imaging data, to correct for signal variance associated with trial-type-specific evoked responses (as implemented by e.g. [ 30 ]).
Trial-averaged modeled (red) and empirical (black) stimulus-related pupil dilations. The vertical dashed line represents event (stimulus or response) onset. Error bars represent the SEM.
https://doi.org/10.1371/journal.pone.0165274.g002
Performance decrements with time-on-task
We first verified whether behavioral performance degraded over the course of a block, as is expected in demanding tasks like the gradCPT that require continually sustained attention [ 30 , 32 ]. To do so, we calculated temporally resolved metrics of trial-averaged behavior by applying a sliding window to the behavioral data of each block of each participant. The window had a width of 50 trials (40 seconds duration) and was slid across the data in steps of 15 trials. For each of these windows we calculated several measures of task performance: 1) the proportion of false alarms; 2) the proportion of trials that fell within the slowest quintile of RTs within the block; 3) average RT; and 4) the RTCV (see Materials & Methods ). For each of these measures this approach resulted in a continuous time series for each block. We then Z-scored the time series and fitted a straight line to them. The slopes of the fitted lines indicated whether the time series were on average increasing or decreasing (or not changing) over time. We averaged the slopes across blocks for each participant and tested if the distribution of slopes was larger than 0 using a one-tailed t -test. As expected, we found significant performance decrements for all behavioral measures. Over the course of a block, progressively more false alarms occurred ( t (27) = 1.74, p = 0.047), and RTs became longer (RT: t (27) = 2.47, p = 0.010; quintile: t (27) = 3.31, p = 0.001) and more variable ( t (27) = 3.06, p = 0.003; Fig 1b ). The proportion of misses also increased with time ( t (27) = 3.31, p = 0.001), but misses were rare (0.2% of all trials) and will thus not be considered in any further analyses. In sum, over the course of a block performance deteriorated. For the sake of simplicity, we hereafter refer to the effect of time within blocks as ‘time-on-task’ effects.
The effects of time-on-task on tonic pupil fluctuations
Having established that behavioral performance on the task degraded over time, we next turned to the pupil data. We applied a sliding window to the unsegmented pupil data that was identical to the one applied to the behavioral data (a width of 50 trials and a step size of 15 trials). We extracted two measures: 1) the average pupil diameter in each window, hereafter referred to as ‘baseline diameter’; and 2) the average temporal derivative of baseline diameter, which quantifies the extent to which the pupil tended to dilate or constrict within each window. The derivative measure was calculated as the average difference between each two consecutive samples within the window (using MATLAB’s ‘diff’ function). This is equivalent to the difference in baseline diameter between the first and last sample of the window. For each of the pupillary measures this resulted in a time series that was identical in length to the time series of the behavioral measures.
As a direct follow-up on the behavioral analyses, we first examined whether the pupillary measures also showed time-on-task effects. To do so, we fitted a straight line to each pupil time series. The slope of the fitted line was informative of linear trends over time. We averaged the slopes across blocks for each participant and compared the distribution of slopes to 0 using a two-tailed t -test. We had no clear hypothesis regarding the direction of the time-on-task effect for the derivative of pupil diameter, so for this test we also used a two-tailed t -test. Both pupil measures showed significant linear time-on-task effects. Over the course of a block baseline diameter became smaller ( t (27) = 8.10, p < 0.001; Fig 3a ). On average, its derivative was initially negative and became less negative over time ( t (27) = 4.40, p < 0.001; Fig 3b ), reflecting the fact that the pupil progressively decreased in diameter during the early-to-mid portions of a block and reached a relatively stable diameter thereafter.
a) Time-on-task effect for baseline diameter, and b) for diameter derivative. p- values are listed in the top left corner of each panel. The derivative is shown as variance-normalized but without the mean removed. Values below the horizontal dotted line indicate that on average the pupil is constricting, whereas values above the line indicate that the pupil is dilating. c) The relationship between pupil diameter and its derivative. Baseline pupil diameter plotted as a function of the derivative. Diameter is smallest when the pupil is dilating the fastest. USD: Units standard deviation.
https://doi.org/10.1371/journal.pone.0165274.g003
Before examining the relationship between baseline diameter and its derivative vis-à-vis the behavioral performance measures, we wanted to make sure that the two pupil measures were not highly correlated with each other, so that they might be expected to explain unique variance in the behavioral measures. In order to clarify the relationship between baseline diameter and its derivative, we correlated their respective time series derived from the sliding-window approach, for each participant and each block, and compared the distribution of Fisher-transformed correlation coefficients averaged across blocks to zero using a t -test. Although the correlation was consistently negative across participants ( t (27) = -2.48, p = 0.020; Fig 3c ), the average correlation coefficient was rather small: -0.12. Thus, the two pupil measures only weakly co-varied ( R 2 < 1.5%) and their capacities to explain unique portions of the variance in behavior were high.
The relationship between tonic pupil fluctuations and behavior
We next used multiple regression to examine linear relationships between the time series of each of the Z-scored pupillary measures and each of the Z-scored behavioral measures, within participants and within blocks. A separate model was constructed for each of the pupillary/behavioral measure pairings. We also included quadratic regressors in these models, but only report the quadratic relationships between baseline diameter and the behavioral measures. The inclusion of quadratic regressors in the regression models for the derivative did not affect the direction and significance of the linear regression coefficients. We averaged the resulting regression coefficients across blocks for each participant and compared the distribution of regression coefficients to 0 using t -tests. We expected the typical Yerkes-Dodson relationship between baseline diameter and behavior (but see below), and therefore used one-tailed t -tests to compare the quadratic regression coefficients to zero. Furthermore, because all analyses concerning the derivative of baseline diameter were exploratory, we used two-tailed t- tests in these analyses. The linear and quadratic relationships between the pupil measures and the behavioral measures are summarized in Fig 4 .
Regression coefficients are shown per pupil measure and behavioral measure. USD: Units standard deviation. Error bars represent the SEM. *: p < 0.05; **: p < 0.01; ***: p < 0.001.
https://doi.org/10.1371/journal.pone.0165274.g004
We found a significant positive quadratic relationship between baseline diameter and false alarm rate ( t (27) = 1.99, p = 0.029), indicating that false alarm rate tended to increase at both the upper and lower extremes of baseline pupil diameter. This finding is consistent with the long-recognized inverted U-shaped relationship between arousal and task performance [ 22 ]. However, we found no such quadratic relationship for the other behavioral measures (all p s > 0.05).
Given the linear time-on-task effects on baseline diameter and each of the behavioral measures, it may be expected that baseline diameter be linearly related to false alarm rate, RT, RTCV, and the proportion of trials that fell within the slowest RT quintile. We thus used one-tailed t -tests to test this hypothesis. In line with this notion, all behavioral measures were negatively related to baseline diameter (false alarm rate: t (27) = 2.28, p = 0.02; quintile: t (27) = -2.60, p = 0.008; RT: t (27) = -2.38, p = 0.012; RTCV t (27) = -2.27, p = 0.016). Thus, more false alarms and longer and more variable RTs tended to occur when baseline diameter was smallest which, as shown earlier, also tended to coincide with the end of task blocks. The linear relationships between baseline diameter and each of the behavioral measures are shown in Fig 5a .
Pupil data were z-scored within participants and blocks, aggregated across participants, and then divided up into 30 bins, and the behavioral data were sorted according to pupil diameter. Large positive values on the Y-axis indicate relatively poor behavioral performance. The initially linear relationship between baseline diameter and behavior becomes U-shaped after controlling for time-on-task, whereas the relationship between the derivative of baseline diameter and behavior remains linear after controlling for time-on-task. Straight lines are least squares regression lines, curved lines are fitted 2 nd -order polynomials.
https://doi.org/10.1371/journal.pone.0165274.g005
Interestingly, the derivative of pupil diameter showed a significant positive linear relationship with all behavioral measures (false alarm rate: t (27) = 3.71, p = 0.001; quintile: t (27) = 3.10, p = 0.005; RT: t (27) = 2.10, p = 0.046; RTCV: t (27) = 3.11, p = 0.005). The positive relationship indicated that periods during which the pupil was relatively stable or dilating (i.e., the value of the derivative was positive/least negative) were characterized by the most false alarms and the slowest and most variable RTs ( Fig 5b ). In other words, periods in which the pupil showed little change in size over time or tended to dilate slowly, were marked by the poorest behavioral performance.
In order to rule out the possibility that these results were dependent on the choice of window size, we repeated the regression analysis for a range of sliding window sizes (40 s to 4 min, and an 8-s difference in width between each consecutive window size). For each window size, we then computed the regression coefficients indicating linear and quadratic relationships between the time series of each of the Z-scored pupillary measures and each of the Z-scored behavioral measures. We then averaged the resulting regression coefficients across blocks, and across the behavioral measures, and computed their area under the curve (AUC) across window sizes. This AUC summary statistic indicated whether on average the behavioral measures showed a relationship (linear or quadratic) with the two pupil measures. Finally, we tested if the group-level distribution of AUCs differed from 0 using one-tailed t -tests. If the linear pupil-behavior relationships were not dependent on the choice of a single (arbitrary) window size, we expected the AUC of the linear regression coefficients to go in the same direction as the initial regression coefficients. That is, we would expect the AUC to be negative for diameter, and positive for the derivative. As expected, the linear AUCs were significantly different from 0 and in the predicted direction for both pupil measures (diameter: t (27) = -2.62, p = 0.007; derivative: t (27) = 4.05, p < 0.001). Also in line with our expectations, the quadratic AUC for baseline diameter did not differ from zero ( t (27) = 0.09, p = 0.47). Altogether, these results show that periods during which the pupil was smallest and remained relatively stable or dilated again were marked by the poorest behavioral performance on the task. These effects were consistent across a range of time scales.
The relationship between tonic pupil fluctuations and behavior, controlled for time-on-task
It is possible that the relationships between baseline diameter and behavior reported above simply reflect the strong effects of time-on-task on these two types of variables, rather than a more intrinsic, time-invariant relationship. We therefore wondered whether shared effects of time-on-task on baseline diameter and behavior might be obscuring more subtle relationships between the associated measures. To address this possibility, we explored whether the relationship between the pupillary measures and behavior remained after statistically controlling for time-on-task. To do so, we performed similar regression analyses as before, except that we included a linearly increasing predictor that tracked time-on-task (i.e., the time elapsed within each block). As a result, the regression coefficients represented the relationship between the pupillary measures and behavior, independent of a linear time-on-task effect.
As can be seen in Fig 6 , the initial linear relationships between baseline diameter and the RT measures became quadratic when time-on-task was taken into account. Both relatively small and large diameters were associated with an increased false alarm rate, and slower and more variable RTs (false alarm rate: t (27) = 1.99, p = 0.028; quintile: t (27) = 1.45, p = 0.08; RT: t (27) = 2.06, p = 0.025; RTCV: t (27) = 2.79, p = 0.005), whereas linear relationships between pupil size and these behavioral measures were no longer present (all p > 0.2). This suggests that a U-shaped relationship between baseline diameter and RT measures was indeed initially obscured by strong time-on-task effects ( Fig 5c ). In contrast, the linear relationships between the derivative and the behavioral measures that were evident in the original regression models were largely preserved in the model that statistically controlled for time-on-task (false alarm rate: t (27) = 3.09, p = 0.005; quintile: t (27) = 2.13, p = 0.041; RT: t (27) = 0.93, p = 0.360; RTCV: t (27) = 3.07, p = 0.005; Fig 5d ). These effects indicate that periods in which linearly detrended pupil diameter was generally increasing were associated with relatively impaired performance.
Regression coefficients per pupil measure and behavioral measure with time-on-task included as a variable of non-interest. Error bars represent the SEM. *: p < 0.05; **: p < 0.01.
https://doi.org/10.1371/journal.pone.0165274.g006
Again, these results were not dependent on the choice of window size, because the AUC summary statistics across window sizes and behavioral measures showed a similar shift from linear to quadratic for baseline diameter after statistically controlling for time-on-task (linear AUC: t (27) = 0.29, p = 0.39; quadratic AUC: t (27) = 3.08, p = 0.002). The linear relationship between the derivative and behavior was also preserved in the AUC across window sizes (linear AUC: t (27) = 3.08, p = 0.002).
Together, these results suggest that time-on-task was driving the initially observed linear relationships between mean baseline diameter and task performance, and to some extent obscured latent quadratic relationships between these variables. In contrast, the linear relationship between task performance and the derivative of pupil diameter was mostly robust to controlling for time-on-task. As we discuss below, this relationship likely reflects the quadratic relationship between diameter and behavior that occurred independent of time-on-task.
Using a fast-paced sustained attention task, we found robust linear relationships between baseline pupil diameter and several behavioral manifestations of attentional lapses. However, these linear relationships primarily reflected the joint effect of time-on-task on baseline pupil and behavior: as performance deteriorated over the course of a block (as indexed by increased false alarm rate and slower and more variable RTs), the pupil became progressively smaller. Importantly, when this effect of time-on-task was statistically partialled out, the relationship between baseline diameter and behavior became U-shaped: more false alarms, and longer and more variable RTs occurred during periods of both relatively small and relatively large baseline diameter, a pattern that is consistent with the Yerkes-Dodson law of mental task performance [ 22 ] and the adaptive gain theory of LC-NE function [ 3 ].
Previous studies on the relationship between pupil diameter and attentional state have yielded contradicting results. Some studies have reported that moments of poor task performance or off-task thought are associated with larger baseline diameter [ 6 – 9 ]. Conversely, others have reported that poor task performance is associated with smaller baseline diameter [ 10 – 14 ]. Our research suggests three methodological reasons for these mixed results. First, a multitude of measures have been used to assess attentional state. Although the performance measures used in the current study generally showed similar relationships with pupil diameter, there were some differences between the measures. For example, as opposed to the RT measures, false alarm rate already displayed a U-shaped relationship with diameter before the effect of time-on-task was partialled out. This discrepancy may be explained by the possibility that slow and variable RTs primarily reflect a decrease in attentional focus [ 34 ]—equivalent to a lower (e.g. [ 35 ]) and/or more variable [ 36 ] rate of decision formation—whereas false alarms may reflect either a decrease in attentional focus or an inadvertent lowering of the response threshold [ 37 ]. Thus these signatures of attentional lapses may have partially dissociable mechanistic bases. To make sure that key conclusions do not depend on the specific choice of measure, future studies should ideally use a range of performance measures, as we have done here. Second, the majority of previous studies have reported only categorical comparisons (e.g., on-task versus off-task thought [ 6 , 10 , 38 ]; or normal versus slow RTs [ 9 , 12 ]) to assess the relationship between pupil diameter and attentional state. However, such comparisons cannot reveal potential non-linear relationships between pupil and behavior. Thus, the manner in which the relationship between baseline diameter and attentional state is assessed restricts the conclusions that can be drawn from the data.
Finally, our results suggest that contradictory findings in the literature may also be due to differences between studies in the presence and nature of parallel effects of time-on-task on pupil diameter and behavior. In tasks that are demanding, such as our task, the dominant finding is that attentional lapses and mind wandering are associated with a smaller baseline pupil diameter than non-lapses or on-task thought (e.g. [ 11 – 13 ]). This pattern may simply be due to a progressive decrement in behavioral performance along with a monotonic decline in pupil diameter over time, perhaps reflecting a shift from center to left on the Yerkes-Dodson curve and a corresponding abandonment of exploitative behavior [ 3 ], or reduced top-down control of behavior [ 21 ]. Such time-dependent shifts on the Yerkes-Dodson curve could be the consequence of depleted cognitive resources. As noted by Hopstaken et al. [ 10 ], there is substantial overlap between the behavioral consequences of mental fatigue and the characteristics of low-arousal states. Nevertheless, the mechanistic origin of simultaneous effects of time-on-task on pupil diameter and performance remains an interesting open question for future research. In less demanding tasks, by contrast, time-related performance decrements are often less severe, and pupil diameter has even been reported to increase over time in such settings [ 15 ]. Such an absence of shared time-on-task effects might in turn afford greater scope for revealing more nuanced relationships between pupil diameter and task performance in the observed data. We suggest that future studies should carefully distinguish between pupil-behavior relationships due to time-on-task and potentially more subtle relationships that operate on a faster time scale. As we have shown, this dissociation can be easily achieved via the implementation of appropriate statistical control.
Aside from yielding insight into the mechanisms underlying attentional lapses, an important long-term goal of studies such as ours is to establish psychophysiological markers that can be used in on-line biofeedback systems, aimed at predicting and preventing lapses of attention. Recently, deBettencourt et al. [ 39 ] made an important step towards the realization of such a system. By providing participants with well-timed performance feedback based on the on-line analysis of brain imaging data, they could improve participants’ performance on a sustained attention task. However, the involvement of brain imaging equipment imposes obvious restrictions on the real-world applicability of this technique. Our results, however, indicate that the pupil could potentially be used to predict when lapses of attention are likely to occur. Given the relatively non-invasive and cost-effective nature of eye-tracking, such a system would offer substantial advantages over neuroimaging-based systems. However, it should be noted that the average regression coefficients that captured the relationship between the dynamics of the pupil and the dynamics of behavior, although consistent across participants, were modest in size (between 0.1 and 0.2). Thus, future work is needed to establish the practical feasibility of using pupil diameter and its derivative as on-line markers of attentional lapses.
Our findings that the average derivative of the pupil diameter time series was linearly related to behavioral performance, and that this relationship was independent of time-on-task, indicate that the derivative of pupil diameter offers a potential marker of attentional performance. The robustness of the derivative to time-on-task compared to baseline diameter may be explained by the way we computed this measure. Specifically, the derivative reflected the difference in baseline diameter between the first and last time point in the sliding window. Thus, this measure was less affected by block-wide trends in pupil diameter but instead captured changes at the temporal scale of the applied sliding window. Moreover, the derivative of a U-shaped signal is monotonically increasing ( f ( x ) = a x 2 + c → f ‘( x ) = 2a x ). The relationship between pupil diameter and its derivative are fixed in this way, because the latter is computed as a function of the former. Any quadratic relationship between a variable (e.g., baseline diameter) and another variable (e.g., behavior) will therefore be measurable as a linear relationship between the derivative of the first variable (baseline diameter derivative) and the second variable (behavior). This holds true even in the presence of a superimposed linear relationship between diameter and behavior (e.g., due to time-on-task effects), because the linear part of the function will simply reduce to a constant in the derivative ( f ( x ) = a x 2 + b x + c → f ‘( x ) = 2a x + b). Thus, the linear relationship between the baseline diameter derivative and behavior likely reflected the quadratic relationship between baseline diameter and behavior that occurred independent of time-on-task.
A bio-feedback system could thus incorporate the derivative of pupil diameter and a receiver operating characteristic analysis could be performed to examine how reliably the signal preceding a behavioral response discriminates between lapse and non-lapse trials. Future studies could also incorporate purely momentary fluctuations in the derivative of the pupil (cf. [ 16 , 31 ]) as opposed to changes during a longer window. These instantaneous fluctuations are, however, beyond the scope of the current study, as we were primarily interested in tonic fluctuations that evolve over longer time periods and how they relate to global fluctuations in attentional performance. Such global fluctuations are more akin to real-world fluctuations in behavior in settings that require prolonged sustained attention, as when an air-traffic controller must monitor a display for long periods of time.
In conclusion, our results demonstrate that time-on-task, a factor that is often ignored in studies on the relationship between pupil diameter and attentional state, can obscure non-linear pupil-behavior relationships. The non-linear (inverted U-shaped) relationship between baseline pupil diameter and attentional performance that we observed after partialling out time-on-task effects is consistent with the adaptive gain theory of LC-NE function [ 3 ]. Finally, our results indicate that the derivative of pupil diameter is a potential marker of attentional performance that could be used for the on-line prediction and prevention of attentional lapses.
Acknowledgments
We thank Michael Esterman for providing the behavioral task. This research was supported by a Consolidator Grant of the European Research Council.
Author Contributions
- Conceptualization: RLvdB PRM SN.
- Data curation: RLvdB.
- Formal analysis: RLvdB PRM.
- Funding acquisition: SN.
- Investigation: RLvdB.
- Methodology: RLvdB PRM SN.
- Project administration: SN.
- Software: RLvdB.
- Supervision: PM SN.
- Visualization: RLvdB.
- Writing – original draft: RLvdB.
- Writing – review & editing: RLvdB PRM SN.
- View Article
- PubMed/NCBI
- Google Scholar
Pupillometry tracks fluctuations in working memory performance
Affiliations.
- 1 Department of Psychology, Arizona State University, 950 S. McAllister Ave., Tempe, AZ, 85287, USA. [email protected].
- 2 Department of Psychology, University of Oregon, Eugene, OR, USA.
- PMID: 30411207
- DOI: 10.3758/s13414-018-1618-4
In 3 experiments, we examined fluctuations in working memory (WM) performance and associated changes in pretrial and task-evoked pupil diameter. Additionally, we examined whether particularly poor trials were accompanied by self-reports of off-task attentional states. The results demonstrated that task-evoked pupillary responses can be used to measure moment-to-moment fluctuations in the success of WM maintenance during delay intervals. Further, when individuals reported being in an off-task attentional state, their WM performance suffered. Additionally, when probed directly after a particularly poor trial, participants reported being in an off-task attentional state more often than at random intervals throughout the task. So behavioral, subjective, and physiological data converged when people experienced WM failures. Although pretrial pupil diameter did not consistently differentiate between successful and unsuccessful trials, variability in pretrial pupil diameter accounted for a significant portion of variance in WM task performance. This effect persisted after controlling for mean task-evoked pupillary response and variability in task-evoked pupillary responses. Thus, one of the major reasons people varied in the consistency with which they utilized their WM system was variability in arousal. Such variability in arousal is potentially due to variation in the functioning of the locus coeruleus-norepinephrine (LC-NE) neuromodulatory system, and thus may underlie individual differences in WM capacity and attention control.
Keywords: Attention; Mind-wandering; Pupillometry; Working memory.
- Analysis of Variance
- Arousal / physiology
- Attention / physiology*
- Individuality
- Memory, Short-Term / physiology*
- Pupil / physiology*
- Task Performance and Analysis

An official website of the United States government
The .gov means it’s official. Federal government websites often end in .gov or .mil. Before sharing sensitive information, make sure you’re on a federal government site.
The site is secure. The https:// ensures that you are connecting to the official website and that any information you provide is encrypted and transmitted securely.
- Publications
- Account settings
Preview improvements coming to the PMC website in October 2024. Learn More or Try it out now .
- Advanced Search
- Journal List
- Cereb Cortex

Catching wandering minds with tapping fingers: neural and behavioral insights into task-unrelated cognition
Josephine m groot.
Department of Psychology, UiT – The Arctic University of Norway, Tromsø 9037 , Norway
Integrative Model-Based Cognitive Neuroscience Research Unit, University of Amsterdam, Amsterdam 1018 WB , The Netherlands
Gábor Csifcsák
Sven wientjes.
Department of Experimental Psychology, University of Ghent, Ghent 9000 , Belgium
Birte U Forstmann
Matthias mittner, associated data.
When the human mind wanders, it engages in episodes during which attention is focused on self-generated thoughts rather than on external task demands. Although the sustained attention to response task is commonly used to examine relationships between mind wandering and executive functions, limited executive resources are required for optimal task performance. In the current study, we aimed to investigate the relationship between mind wandering and executive functions more closely by employing a recently developed finger-tapping task to monitor fluctuations in attention and executive control through task performance and periodical experience sampling during concurrent functional magnetic resonance imaging (fMRI) and pupillometry. Our results show that mind wandering was preceded by increases in finger-tapping variability, which was correlated with activity in dorsal and ventral attention networks. The entropy of random finger-tapping sequences was related to activity in frontoparietal regions associated with executive control, demonstrating the suitability of this paradigm for studying executive functioning. The neural correlates of behavioral performance, pupillary dynamics, and self-reported attentional state diverged, thus indicating a dissociation between direct and indirect markers of mind wandering. Together, the investigation of these relationships at both the behavioral and neural level provided novel insights into the identification of underlying mechanisms of mind wandering.
Introduction
The phenomenon of mind wandering in humans can be described as the spontaneous stream of consciousness that comprises thoughts, emotions, and memories ( Smallwood and Schooler 2015 ) that are often related to personal goals and concerns ( Shepard 2019 ) and pervasively occur during daily life and experimental tasks ( Killingsworth and Gilbert 2010 ; Seli, Beaty, et al. 2018 ). Unsurprisingly given this broad definition, mind wandering has been studied in a wide range of settings and under different labels, for example, stimulus-independent, spontaneous, and task-unrelated thought ( Callard et al. 2013 ). Researchers have identified important dimensions of mind wandering, including intentionality ( Seli et al. 2016 ), emotional valence ( Banks et al. 2016 ), temporality ( Maillet et al. 2017 ), and meta-awareness ( Schooler et al. 2011 ). Here, we define mind wandering as self-generated thoughts that arise independently from external sensory input and pertain to any content that is unrelated to the task athand.
Notwithstanding the diversity of contexts in which mind wandering has been previously investigated, researchers continue in their pursuit to further unravel its underlying neural mechanisms and its effect on other cognitive processes and behavior. In particular, although there is little debate regarding the involvement of executive functions in mind wandering in general, there is no consensus on exactly how mind wandering interacts with executive control systems and whether it is better characterized as executive function use ( Teasdale et al. 1995 ; Smallwood and Schooler 2006 ) or as the result of executive failure ( McVay and Kane 2010 ; Kane and McVay 2012 ). Whereas some behavioral research has associated mind wandering with failures of executive control processes ( Smallwood et al. 2004 ; McVay and Kane 2009 ), converging evidence from neuroimaging studies suggest that mind wandering recruits widespread cortical networks involved in goal-directed behavior, including the frontoparietal control network (FPCN) and dorsal and ventral attention networks ( Christoff et al. 2009 ; Fox et al. 2015 ; Dixon et al. 2018 ; Turnbull et al. 2019 ). Interestingly, there are even reports of associations between task-related attention, as opposed to mind wandering, and greater activation of the default mode network (DMN; Esterman et al. 2014 ; Kucyi et al. 2017 ; Groot, Boayue, et al. 2021 ), a network previously considered to mainly engage during resting-state and self-referential processing ( Raichle 2015 ). Furthermore, a recent resting-state fMRI study with experience sampling demonstrated that activity in the DMN was associated with self-generated thoughts that were not independent from the external environment whereas activity in the dorsal attention network (DAN) related specifically to increases in perceived control over spontaneous thought ( Van Calster et al. 2017 ). Together, these findings undermine the assumption that task-related and task-unrelated states of mind can be independently partitioned into specific functional networks and warrant the development of sensitive behavioral paradigms that disentangle the complex interplay between executive functions and forms of spontaneous thought.
The majority of mind wandering research reports data from self-reports through periodical thought probing and performance errors, usually during a sustained attention to response Task (SART; Christoff et al. 2009 ; Christoff 2012 ; Hawkins et al. 2019 ; Boayue et al. 2019 ). However, the SART is often slow paced and target stimuli are presented infrequently, preventing more fine-grained tracking of ongoing fluctuations in both attention and executive control. Arguably, a more suitable paradigm to investigate executive functioning is the random number generation task (RNGT; Baddeley et al. 1998 ) as it is assumed that the generation of random sequences of numbers or letters requires highly controlled executive processes that strategically monitor and inhibit habitual tendencies in order to avoid repetition of response patterns ( Jahanshahi et al. 2000 ; Joppich et al. 2004 ; Jahanshahi et al. 2006 ; Peters et al. 2007 ). Indeed, competing processes such as mind wandering or dual task performance result in the reduced ability to produce such random behavior ( Teasdale et al. 1995 ; Boayue et al. 2020 ). Thus, investigation of the relationships between the degree of sequence randomness and the occurrence of mind wandering episodes has the potential to provide insights into how the mental processes supporting departures from a task-focused state compete with the cognitive resources needed for executive task performance.
Besides monitoring executive function use, findings from several studies suggest trial-to-trial response time variability as a promising and sensitive marker for fluctuations in attentional focus ( Bastian and Sackur 2013 ; Jubera-Garcia et al. 2019 ; Zanesco et al. 2020 ), especially when combined with a monotone and simplistic finger-tapping task that facilitates mind wandering ( Seli et al. 2013 ; Kucyi et al. 2017 ). Building on these findings, Boayue et al. (2020 ) recently developed a fast-paced paradigm that combines both these aspects of behavior into a finger-tapping random-sequence generation task (FT-RSGT), allowing ongoing assessment of the degree of self-generated randomness as well as behavioral variability at high temporal resolution. In a series of experiments, it was demonstrated that both measures were consistently related to mind wandering in opposite ways: variability in finger-tapping increased whereas sequence randomness decreased prior to self-reports of mind wandering throughout thetask.
Similarly, several studies have attempted to identify psychophysiological markers reliably related to an individual’s attentional state. In particular, a growing body of evidence suggests that spontaneous changes in pupil size are linked to dynamic fluctuations between internal versus external attention and awareness ( Laeng et al. 2012 ; Schneider et al. 2016 ; DiNuzzo et al. 2019 ). Changes in slowly fluctuating baseline pupil size as well as fast evoked pupillary responses are thought to be modulated by the locus coeruleus–norepinephrinergic system (LC/NE; Aston-Jones and Cohen 2005 ; Joshi et al. 2016 ) and have introduced new opportunities to objectively monitor mind wandering and arousal state ( Mittner et al. 2014 ; Unsworth and Robison 2016 ). However, research on the relationship between mind wandering and tonic pupil size has yielded more inconsistent results as both larger and smaller tonic pupils have been associated with mind wandering ( Smallwood et al. 2012 ; Grandchamp et al. 2014 ; Konishi et al. 2017 ; Jubera-Garcia et al. 2019 ). This is possibly due to differences in task demands and thereby the required levels of vigilance ( Unsworth and Robison 2018 ) or, as proposed in a recent theoretical model, variations in tonic pupil size may reflect qualitatively distinct task-unrelated states ( Mittner et al. 2016 ).
In summary, the DMN, attention and executive control networks, and the LC/NE-system are all implicated in mind wandering but empirical evidence into how these neural systems interact to give rise to mind wandering is at present incomplete. Building on previous findings, we aimed to address this by employing an fMRI version of the FT-RSGT that combines experience sampling with objectively defined measures interpreted as indirect makers for changes in ongoing attentional state, including sensitive behavioral indices and pupillometric measures. Following Boayue et al. (2020) , we expected increases in the variability of finger-tapping and decreases in the degree of randomness of the tapping-sequence preceding self-reported mind wandering episodes. Furthermore, we aimed to validate that performance of the FT-RSGT indeed relies on executive control processes that are known to be recruited during the original RNGT. To this end, we contrasted brain activation during the generation of random tapping-sequences with a simple alternating finger-tapping task and expected to observe greater activation in frontoparietal regions involved in executive control during random finger-tapping.
Additionally, we explored the pattern of neural activation in relation to both direct and indirect markers of mind wandering by directly assessing the patterns of network-wide activity in the periods preceding experience sampling probes as well as the brain regions that correlated with behavioral performance. In line with a previous finger-tapping study, we expected to observe recruitment of ventral and dorsal attention networks and cerebellum when variability in finger-tapping was high and DMN activation when finger-tapping was more stabilized ( Kucyi et al. 2017 ). The degree of sequence randomness was expected to correlate with activity in frontoparietal and sensorimotor areas associated with executive control and self-determined action ( Schubert et al. 1998 ; Jahanshahi et al. 2000 ). Direct predictions regarding network activation preceding self-reports of mind wandering are less evident considering the contradictory findings in the literature ( Christoff et al. 2009 ; Mittner et al. 2014 ; Groot, Boayue, et al. 2021 ), but similar patterns of neural recruitment are expected for direct (self-report) and indirect (objective task performance and pupil dynamics) measures of mind wandering given that these relationships are replicated on the behavioral level. Finally, dynamic changes in tonic and phasic pupil size were assessed and related to self-reported mind wandering throughout the task. Whereas phasic pupil responses to task-related events are generally expected to be smaller during mind wandering due to perceptual decoupling ( Smallwood et al. 2011 ; Unsworth and Robison 2018 ), the exact relationship with tonic pupil size is less clear. We therefore also investigated the neural substrates of both pupillary components to explore whether the brain regions correlating with changes in tonic and phasic pupil size would demonstrate similarity to the pattern of neural activity associated with mind wandering.
Materials and Methods
Participants.
The study was approved by the Ethics Review Board of the Faculty of Social and Behavioral Sciences at the University of Amsterdam. Participants were 27 healthy adult volunteers aged 20–45 years (15 male, mean age = 27.5, SD = 7.2 years) who were recruited from the Amsterdam ultra-high field adult lifespan database (AHEAD; Alkemade et al. 2020 ). Participants had normal or corrected-to-normal vision, no self-reported (history of) psychiatric or neurological illness, and no contraindications for MRI as assessed with a standard safety questionnaire. To avoid biases in task performance related to individual differences in rhythmic abilities and finger tapping, experienced and (semi-)professional musicians were excluded from the study. Written informed consent was obtained prior to the experiment and participation was compensated with a standard monetary reward of €15 for a total duration of 90 min. All materials, anonymized data, and code are publicly available in an Open Science Framework (OSF) repository ( Groot, Csifcsák, et al. 2021 ).
Finger-Tapping Random-Sequence Generation Task
Participants completed 18 experimental and 9 control blocks of the finger-tapping random-sequence generation task (FT-RSGT, Boayue et al. 2020 ; Fig. 1 ) in a pseudorandomized order. Stimuli were presented on a 32 inch BOLD screen using PsychoPy ( Peirce 2007 ). At the start of each block, instructions appeared for 4000 ms at the center of the screen to indicate whether it was an experimental (“RANDOM”) or control (“ALTERNATING”) block. In the alternating task, participants were instructed to simply press the response buttons with their left and right index fingers in an alternating sequence (L-R-L-R-L-R-etc.). In the random task, they were asked to generate a sequence of left and right button presses with maximum unpredictability, or randomness (e.g., L-R-L-L-L-R-etc.). Hence, the two tasks were identical with respect to stimulus presentation and execution of motor responses but differed in the randomness criterion and thus, in the extent to which executive control processes were necessary to maintain performance. The concept of randomness was explained with a coin flip analogy: Similar to flipping a coin, a left versus right button press should occur at equal probability and be independent from past or future button presses. To ensure that participants understood these instructions, they performed a short practice run of the task and answered quiz-questions about the concept of randomness (e.g., “If there have been three right presses, must there always be a left press?”). If mistakes were made, further instructions and practice were provided until the task was mastered.

Schematic of the finger-tapping random-sequence generation task (FT-RSGT). Participants produced either alternating sequences of left versus right finger-tapping (alternating blocks) or generated sequences trying to maximize their randomness (random blocks). The rhythm of finger-tapping was continuously indicated by an auditory stimulus paced at 750 ms (metronome). At the end of each block, participants were probed to report to what degree their thoughts were unrelated to the task on a six-point Likert scale (reproduced from Groot, Csifcsák, et al. 2021 ).
Throughout the experiment, participants had to synchronize their finger-tapping with an ongoing metronome that was presented as an auditory stimulus (440 Hz pitch for 75 ms) every 750 ms. Previous experiments determined that this pace is optimal for engaging in generating random sequences compared to slower and faster metronomes ( Boayue et al. 2020 ). During finger-tapping, participants fixated on a centered fixation cross that was presented on a gray background while attending to the stimuli through MR-compatible headphones. Every block consisted of 80 stimuli on average (range = 74–87) and ended with a thought probe so that the onsets of thought probes were pseudorandomized to occur between 55.5 and 65.3 s (60 s on average). Thought probes were presented for 6000 ms plus a random jitter between 0 and 1000 ms, formulated as: “Where was your attention (i.e., your thoughts) focused just before this question?”. Responses to the thought probes were ordered on a six-point Likert scale with the following annotations: “clearly on-task,” “partly on-task,” “slightly on-task,” “slightly off-task,” “partly off-task,” and “clearly off-task”. To indicate their answer, participants pressed left and right response buttons to navigate an arrow pointing at the categories. The starting point of the arrow on either extreme end of the Likert scale was randomized across thought probes. Participants were explicitly instructed that “off-task” included all thoughts unrelated to the task, for example, daydreaming, personal memories, or future plans whereas “on-task” referred to task-related thoughts, such as thinking about which button to press next or focusing the rhythm of the metronome. The total duration of the task was ~30 min.
Acquisition and Preprocessing
Two aspects of FT-RSGT performance were assessed. First, behavioral variability (BV) was calculated as the standard deviation of the intertap intervals (ITIs) of the 25 finger-taps preceding each thought probe. No filtering or preprocessing was performed so that missing or double taps per trial were included in the calculation. The raw standard deviations were log-transformed to approximate a normal distribution. Second, the degree of randomness in the self-generated sequence of left and right finger-taps was measured with the approximate entropy (AE) metric ( Pincus and Kalman 1997 ). As the generated sequences during alternating blocks were completely predictable (i.e., AE = 0), AE was calculated only for the random task condition. Specifically, AE quantifies the regularity in a sequence by evaluating the conditional probability that subsequences of length m that are similar remain similar for subsequences augmented by one position (more details on the calculation of AE are in Supplementary Material A ). A previous study showed that AE ( m = 2) measured in the FT-RSGT correlated with the entropy measure in a keyboard version of the original RNGT, validating its use as an index for executive control ( Boayue et al. 2020 ). AE was calculated for every thought probe across the same preceding 25-tap window and transformed as −ln(ln2 − AE). Both BV and AE were then standardized ( Z -scored) across subjects (i.e., the grand mean and standard deviations were used for standardization). The choice of the 25-tap window was decided a priori based on the assumption that mind wandering occurs in slowly fluctuating episodes spanning multiple seconds as well as for ensuring that sufficient data was gathered for reliable calculations of BV and AE. Additionally, previous experiments revealed that BV and AE based on this window size had the strongest relationship with self-reported mind wandering compared to shorter windows ( Boayue et al. 2020 ).
Pupillometry
The pupil area of the left eye was concurrently recorded during the fMRI session at a sampling rate of 1000 Hz with the Eyelink 1000 Plus tracking system (SR Research). The pypillometry package ( Mittner 2020 ) was used to determine subject-specific velocity profiles for blink detection based on the algorithms described by Mathôt (2013 ). Additional parameters were fine-tuned based on visual inspection of individual datasets, including the margin around blink onset and offset for linear interpolation and maximum distance in time between consecutive blinks for merging. Data were then filtered with a zero-phase shift Butterworth low-pass filter at 5 Hz that was set at 3 Hz for 12 datasets and at 2 Hz for three datasets as visual inspection revealed the presence of abundant high-frequency noise in the pupil signal. These steps ensure rigorous and optimized preprocessing of the pupil signal, circumventing artifacts associated with the high inter-individual variability in blinking transients and frequency. However, due to excessive blinking or technical issues with pupil tracking, the quality of six datasets remained inadequate and these subjects were therefore excluded from all further pupillometric analyses.
Selecting specific windows for extracting the mean pupil signal or peak amplitude is complicated in fast-paced task designs due to the build-up of evoked pupil responses that resemble increases in baseline pupil size and therefore contaminate the baseline estimates of subsequent trials. Therefore, to produce more valid estimates of single-trial tonic (baseline) and phasic (evoked) pupillary dynamics, a recently developed deconvolution-based approach was applied ( Mittner 2020 ). First, the data were downsampled to 250 Hz. Tonic fluctuations were estimated using B-spline basis functions constrained to pass through high prominence troughs in the pupil signal. A second iteration of this estimation, following subtraction of the first tonic estimate as well as modeled pupil–response functions (PRF; Hoeks and Levelt 1993 ) located at known task events, ensured that the final tonic estimate constituted a smooth curve that remained below the signal on which the phasic pupil responses are superimposed. Single-trial tonic pupil size was then calculated at every stimulus onset. To model phasic pupil responses to task-related events, regressors for every stimulus and tap onset were convolved with the pupil-response function (PRF; h = t n e -n / t max , where n = 10 and t max = 900; Hoeks and Levelt 1993 ) and fitted with a nonnegative least-squares solver ( Lawson and Hanson 1987 ) to recover the amplitude of phasic responses as estimated b coefficients. However, predictor multicollinearity was observed as stimulus and tap onsets occurred close in time. Therefore, the final single-trial phasic pupil responses were calculated as the sum of b coefficients from all events located within the 200-ms window before and after each stimulus onset. Finally, single-trial tonic and phasic pupil responses were standardized ( Z -scored) within subjects to remove incidental differences in absolute pupil size across subjects. More details on the deconvolution-based pupil analysis are described by Mittner (2021 ), and an implementation is provided in the pupillometry package ( Mittner 2020 ).
Functional Neuroimaging
Participants were scanned with a 3Tesla Philips Achieva MRI system with a 32-channel head coil. T 1 -weighted ( T 1 w) images were acquired with a turbo field-echo (TFE) sequence in 257 sagittal slices (FOV = 256 × 240 × 180 mm [F-H × A-P × R-L], TR = 11 ms, TE = 5.1 ms, acquired voxel size = 0.7 × 0.76 × 0.7 mm, reconstructed voxel size = 0.67 × 0.67 × 0.7 mm). Whole-brain functional images were acquired in a single fMRI run with single-shot fast field-echo (FFE) echo-planar imaging (EPI), collecting 56 transverse slices per volume with 0.2 mm slice gap (FOV = 224 × 224 × 123 mm, TR = 1800 ms, TE = 30 ms, flip angle = 70°, voxel size = 2 mm isotropic). An additional EPI field map with opposite phase-encoding direction was acquired to measure and correct for field distortions.
Imaging data were preprocessed with fMRIPrep v1.1.7 ( Esteban et al. 2018 ) using Nipype v1.1.3 ( Gorgolewski et al. 2011 ). Structural ( T 1 w) images were corrected for intensity non-uniformity with N4BiasFieldCorrection (ANTs v2.2.0; Tustison et al. 2010 ), skull-stripped with antsBrainExtraction using the OASIS target template, and spatially normalized to the ICBM 152 Nonlinear Asymmetrical template version 2009c (MNI152Nlin2009cAsym; Fonov et al. 2009 ) using the nonlinear registration tool in antsRegistration ( Avants et al. 2008 ). Brain tissue was segmented in cerebrospinal fluid (CSF), white matter (WM), and gray matter (GM) using FAST (FSL v5.0.9; Zhang et al. 2001 ). The functional images were corrected for susceptibility distortion with 3dQwarp (AFNI; Cox and Hyde 1997 ), using a deformation field estimated from the two EPI references with opposing phase-encoding directions. The unwarped BOLD reference based on the estimated susceptibility distortion was then co-registered to the T 1 w reference with FLIRT (FSL v5.0.9; Jenkinson and Smith 2001 ) using the boundary-based registration cost-function ( Greve and Fischl 2009 ) and 9 degrees of freedom to account for remaining BOLD distortions. Head-motion parameters (rotation and translation) were estimated with MCFLIRT (FSL v5.0.9; Jenkinson et al. 2002 ). The preprocessed data were resampled back to native space as well as to standard space (MNI152Nlin2009cAsym template) and smoothed with a 6 mm full-width half-maximum Gaussian kernel using SUSAN ( Smith and Brady 1997 ). All subsequent fMRI analyses were performed on the smoothed preprocessed timeseries in standard space.
Data Analysis
Bayesian hierarchical ordered probit regression models.
The relationships between self-reported mind wandering, behavioral performance, and pupillary dynamics were assessed with regression models using the thought probe responses from the experimental blocks (random task) of the FT-RSGT as dependent variable. Treating the ordinal probe responses as continuous introduces a range of statistical problems, including poor model fitting, low power, increasing the risk for type I and II errors, and spurious interaction effects ( Liddell and Kruschke 2018 ). To circumvent these issues, we applied Bayesian hierarchical ordered probit regression ( Boayue et al. 2019 , 2020 ; Bürkner and Vuorre 2019 ; Alexandersen et al. 2021 ) using the brms package (Bayesian Regression Models using Stan; Bürkner 2017 ). This method models the probability of each discrete point rather than relying on the assumption that the probe responses are normally distributed. In addition, within-subject variability in mind wandering can be taken into account with subject-level random intercepts. As a consequence, probit models are more suitable and sensitive to detect effects in Likert-scale data. For each regression coefficient, we report the posterior mean, its 95% highest-density interval (HDI), and the evidence ratio in favor of a positive (ER + ) or negative (ER − ) effect. The ER is calculated as the ratio between the probability of the effect being positive divided by the inverse probability of the effect being zero or negative (ER + ) or the inverse of that ratio (ER − ) and can be interpreted as an odds-ratio. We consider an effect as reliable when the area under the marginal posterior distribution that is larger than zero (for ER + ) or smaller than zero (for ER − ) is >0.95 (corresponding to an ER of 19 and the 95% HDI excluding zero). The models were fitted with four Hamiltonian Monte Carlo (HMC) chains, each with 1000 warm-up and 4000 post warm-up samples.
In the first probit model, the effects of time (probe number), BV, AE, and the BV × AE interaction on 486 thought probe responses (27 subjects × 18 blocks) were modeled. In accordance with our hypotheses, for time, BV, and BV × AE, we evaluated the evidence for the effect to be larger than zero (ER + ) and for AE to be smaller than zero (ER − ). Similarly, the effects of tonic and phasic pupillary dynamics as well as the tonic × phasic interaction were assessed in addition to time in a second regression model using the 18 random blocks from the 21 subjects with complete pupil datasets. For every thought probe, the extracted single-trial tonic and phasic features were averaged across the preceding 25 trials (18.75 s), ignoring trials with more than 40% missing pupil data. This criterion resulted in exclusion of six thought probes, therefore the model was fitted on 372 thought probe responses in total. The evidence for effects larger than zero (ER + ) for tonic pupil size and the tonic phasic interaction and smaller than zero (ER − ) for phasic pupil responses was evaluated.
fMRI Analysis: General Linear Models
Whole-brain general linear models (GLM) were fitted to the fMRIPrep-preprocessed time series using FSL FEAT ( Woolrich et al. 2001 ) to explore differences in brain activity between the two task conditions and to investigate the role of brain regions involved in mind wandering using 1) experience sampling probes, 2) task performance, and 3) pupillary dynamics. All first-level GLMs included four task-related regressors (left and right finger-taps, metronome stimuli, and thought probe onsets) that were convolved with a double-gamma hemodynamic response function (HRF). In addition, fMRIPrep-derived nuisance regressors calculated for every volume were added, including mean time courses in CSF and WM masks, framewise displacement (FD), six rotation and translation parameters, and discrete-cosine transform (DCT) basis functions to model low-frequency scanner drifts. The modeled data were obtained via ordinary least-squares linear regression. Second-level analyses were performed with FLAME ( Beckmann et al. 2003 ) to obtain group-level parametric contrast maps. Statistical significance of brain areas was evaluated with cluster z-thresholding ( Friston et al. 1994 ). First, a primary voxel-level threshold of z > 2.3 defined clusters of above-threshold voxel activations. Second, a cluster-level threshold of P < 0.05 was applied to eliminate non-significant clusters.
First, we explored the hypothesis that random-sequence generation recruits more widespread executive and attentional networks compared to alternating finger-tapping. In addition to the task-related and nuisance regressors, the occurrence of random and alternating blocks was modeled by two boxcar functions convolved with a double-gamma HRF. In a second model, patterns of brain activity associated with episodes of mind wandering compared to on-task thoughts were investigated by convolving boxcar functions that modeled the 10s intervals preceding off-task versus on-task thought probes ( Christoff et al. 2009 ). To account for individual differences in response tendencies, the six probe response categories were dichotomized using an algorithm that determined subject-specific boundaries, where the split-point for each subject was chosen to set the proportion of off-task versus on-task probes as close to 50% as possible. This approach allowed us to identify potential episodes of mind wandering in subjects that selected only a very narrow range of response categories to reflect their current attentional state, possibly due to some degree of satisficing, primacy, and social desirability biases ( Weinstein 2018 ; Weinstein et al. 2018 ). For example, if a subject exclusively answered with “clearly on-task” and “partly on-task,” responses in the latter category were labeled as off-task in the analysis. The total proportion of off-task reports was 49% using the split-point algorithm whereas this proportion was 36% when collapsing the first three categories into on-task and the other three into off-task. With the latter approach no significant brain activations preceding off-task reports could be observed. Since the two task conditions were presumed to differ in terms of executive resources necessary for performance and may therefore interact differently with mind wandering, probe regressor functions were created separately for random and alternating blocks.
Third, regressors for task performance were modeled to evaluate brain activity corresponding to increases and decreases in BV and AE. Starting at the 25th trial (metronome onset) per block, BV and AE (random blocks only) were calculated for every single trial based on the preceding 25 finger-taps. To create parametric regressors, single-trial BV and AE were transformed and standardized across subjects before nearest-neighbor interpolation and resampling to the resolution of the fMRI timeseries ( Fig. 2 ). The use of 25-tap sliding windows resulted in smooth and time-lagged regressor functions that did not require additional convolution with a canonical HRF. Our window size of 25 taps was determined a priori based on previous studies on the latency of mind wandering and on-task episodes ( Bastian and Sackur 2013 ; Pelagatti et al. 2020 ), in order to ensure comparable measures for BV and AE to the behavioral analysis, and considering the slow nature of the physiological BOLD response. In the final GLM, we explored changes in brain activity associated with increases and decreases in tonic and phasic pupillary dynamics. The single-trial tonic and phasic pupil features were interpolated with nearest-neighbor interpolation and resampled to the resolution of the fMRI timeseries (TR). Whereas tonic pupil was already modeled as a smooth and slowly fluctuating signal, phasic responses were convolved with a double-gammaHRF.

Behavioral performance during a random task block from a single subject (80 stimuli), showing inter-tap intervals (ITI) and left versus right button presses ( top ), used to calculate behavioral variability (BV) and approximate entropy (AE) starting at the 25th stimulus per block ( middle ), which were then linearly interpolated and resampled to the resolution of the fMRI timeseries for the GLM analysis ( bottom ) (reproduced from Groot, Csifcsák, et al. 2021 ).
For every resulting group-level statistical map we calculated the overlap with the 7- network parcellation ( Yeo et al. 2011 ), the Harvard-Oxford subcortical structural atlas (Harvard Center for Morphometric Analysis), and the probabilistic cerebellar atlas ( Diedrichsen et al. 2009 ) in standard MNI152 space. The cortical network parcellation included visual (VIS), somatomotor (SOM), dorsal attention (DAN), salience/ventral attention (VAN), limbic (LIM), control (CON), and default mode (DMN) networks. The subcortical parcellation consisted of thalamic nuclei, striatum, pallidum, hippocampus, amygdala, nucleus accumbens, and brainstem and were combined into a general subcortical mask for calculating the total percentage overlap and illustration purposes. All three atlases were resampled to the resolution of the functional timeseries using nearest-neighbor interpolation and binarized. The percentage of voxels in the statistical contrast maps that overlapped with each of the binarized atlases was then calculated, ignoring above-threshold voxel clusters that were located in cerebral white matter. Therefore, regardless of the size of significant clusters, every contrast map was always fully accounted for by the parcellation.
Effects of Task Condition on Mind Wandering Reports and Performance
In total, 36% of probe responses were in one of the three off-task categories. The mean probe response given by participants was 2.89 ( SD = 1.46, median = 3) on the six-point Likert-scale, demonstrating that participants reported that their thoughts were more often focused on performing the task rather than being engaged in mind wandering. Indeed, five subjects never reported that their thoughts were in any of the three off-task categories. There was no significant difference in mind wandering propensity between the random ( M = 2.86, SD = 0.95) and alternating task conditions ( M = 2.93, SD = 1.14, t (26) = −0.53, P = 0.602). Mean behavioral variability was, however, significantly higher during random ( M = 0.11, SD = 0.57) compared to alternating finger-tapping ( M = −0.07, SD = 0.53, t (26) = 3.30, P < 0.01) suggesting that the additional task of generating random sequences interfered with maintaining synchronized motor responses to the externally cued rhythm.
Task Performance and Pupil Dynamics Relate to Mind Wandering Reports
Random Sequence Generation Recruits Executive and Attentional Networks
When contrasted with random-sequence generation, we observed that alternating finger-tapping was associated with localized activity in the posterior cingulate cortex/precuneus and left angular gyrus, both regions that are core nodes of the DMN. In line with our hypothesis, the generation of random tapping sequences instead revealed widespread recruitment of cortical areas generally attributed to attention and executive control networks, including the superior parietal lobe, intraparietal sulcus, primary motor cortex, and dorsolateral prefrontal cortex of the right hemisphere as well as bilateral anterior insula, bilateral medial supplementary motor areas, and bilateral anterior and posterior parts of the cerebellum ( Fig. 3 , Supplementary Material B ). Together, these results provide evidence that the two tasks are qualitatively different regarding the cognitive resources necessary for performance and that random-sequence generation during the FT-RSGT requires the recruitment of brain regions associated with executive functioning.

Activations during random-sequence generation contrasted with alternating finger-tapping ( left ) and vice versa ( middle ) and percentage of contrast maps that overlap with a 7-network cortical, subcortical, and cerebellar parcellation ( right ). M1 = primary motor cortex; SPL = superior parietal lobe; IPS = intraparietal sulcus; DLPFC = dorsolateral prefrontal cortex; aINS = anterior insula; SMA = supplementary motor area; AG = angular gyrus; PCC/PCUN = posterior cingulate cortex/precuneus; SUB = subcortex; CBM = cerebellum (reproduced from Groot, Csifcsák, et al. 2021 ).
Mind Wandering Signatures Diverge for Direct versus Indirect Markers
Contrary to previous findings, brain activations directly preceding self-reports of mind wandering when contrasted with on-task reports could not be localized to the known cortical nodes of either the DMN or FPCN/DAN. Instead, we observed clusters of brain activation associated with mind wandering in visual, cerebellar, and subcortical areas that could be distinguished between the two task conditions. Specifically, mind wandering in the alternating task was preceded by activity in the left inferior occipital gyrus, temporal subgyral white matter (not plotted on the surface mesh), and parts of the bilateral anterior and posterior cerebellum, whereas mind wandering during the random task was associated with greater activity in the right striatum ( Fig. 4 A , Supplementary Material B ). To test whether these results were influenced by the selection of the selected data window, the same analysis was performed using 18 s preprobe intervals which resulted in similar activation patterns. In addition, the analysis was repeated combining both task conditions to assess whether separation of the tasks influenced the observed neural correlates of mind wandering. Across tasks, we observed significant activation mainly in the right striatum preceding mind wandering reports when contrasted to on-task reports ( Supplementary Material C ), showing high overlap (Dice similarity coefficient = 0.68) with the activations preceding mind wandering in the random task condition.

( A ) Active brain regions preceding mind wandering reports during the alternating ( left ) and random ( middle ) task conditions. ( B ) Regions correlating with increases ( left ) and decreases ( middle ) in behavioral variability. ( C ) Regions correlating with increases ( left ) and decreases ( middle ) in approximate entropy. ( right ) Percentage of contrast maps that overlap with a 7-network cortical, subcortical, and cerebellar parcellation. IOG = inferior occipital gyrus; STR = striatum; SPL = superior parietal lobe; SMG = supramarginal gyrus; MTG = middle temporal gyrus; aINS = anterior insula; SFG = superior frontal gyrus; ACC = anterior cingulate cortex; vmPFC = ventromedial prefrontal cortex; MFG = middle frontal gyrus; IPS = intraparietal sulcus; SOG = superior occipital gyrus; ITG = inferior temporal gyrus; SMA = supplementary motor area; SUB = subcortex; CBM = cerebellum (reproduced from Groot, Csifcsák, et al. 2021 ).
Next, we assessed patterns of neural activity corresponding to variability in finger-tapping ( Fig. 4 B , Supplementary Material B ) and obtained strikingly similar results as reported in a previous rhythmic finger-tapping study ( Kucyi et al. 2017 ). Increases in finger-tapping variability were correlated with activity in dorsal and ventral attention networks, including the superior parietal lobes, supramarginal gyri, posterior middle temporal gyri, anterior insula, midcingulate cortices, precuneus, lingual gyri of both hemispheres, and bilateral anterior cerebellum. Furthermore, less variable finger-tapping and thus more optimized task performance was associated with greater activity in left superior frontal gyrus, bilateral anterior cingulate cortex, and bilateral ventromedial prefrontal cortex, thereby mostly mapping to theDMN.
Similarly, we observed correlated neural activity in the expected frontoparietal regions for increases in the degree of randomness in the tapping-sequence as quantified with AE, namely within the right intraparietal sulcus, right posterior middle frontal gyrus, and left medial supplementary motor area, thus showing a similar pattern of neural network recruitment as observed for the random task condition in general. Decreases in AE, signaling decrements in task performance, were instead associated with the left inferior temporal sulcus (poorly visible on the plotted surface mesh) and the superior and inferior occipital gyri of the left hemisphere ( Fig. 4 C ). Similar results were obtained when the analyses were performed using 20-tap and 10-tap sliding windows for BV and AE regressor calculation, indicating that the observed patterns of neural activation are robust against changes in this analysis parameter.
Pupillary Dynamics Map to Subcortical and Visual Cortical Areas
Positive correlations with tonic pupil size were observed almost exclusively in subcortical and cerebellar areas, including the thalamus, internal capsule, and intracalcarine cortex of both hemispheres as well as the right hippocampus. In addition, a large brainstem cluster covered the locus coeruleus, substantia nigra, and subthalamic and ventral tegmental nuclei. Furthermore, widespread cerebellar activation was observed in the anterior and posterior lobes and dentate nuclei. Interestingly, brain regions that negatively correlated with tonic pupil size largely overlapped with visual and somatomotor cortical network parcellations, including the primary somatosensory cortices, superior frontal gyri, medial primary motor cortices, lateral occipital cortices, superior temporal gyri, hippocampal areas, and cuneal cortices of both hemispheres in addition to the left middle temporal gyrus and right fusiform gyrus ( Fig. 5 A , Supplementary Material B ). In contrast, activity in brain regions that corresponded with changes in phasic pupil responses to task-related events was less and extensive and more posteriorly localized. Specifically, larger phasic pupil responses were associated with greater activity in the left lingual gyrus, whereas smaller phasic pupil responses were associated with activation of the left superior temporal gyrus (poorly visible on the surface mesh), right inferior occipital gyrus, and bilateral superior occipital gyri ( Fig. 5 B ).

( A ) Active brain regions with increasing ( left ) and decreasing ( middle ) tonic pupil size. ( B ) Regions correlating with increasing ( left ) and decreasing ( middle ) phasic pupil responses to task-related events. ( Right ) Percentage of contrast maps that overlap with a 7-network cortical, subcortical, and cerebellar parcellation. HPC = hippocampus; LC = locus coeruleus; SN = substantia nigra; STN = subthalamic nucleus; VTA = ventral tegmental area; Tha = thalamus; IC = internal capsule; intCALC = intracalcarine cortex; SFG = superior frontal gyrus; S1 = primary somatosensory cortex; LOC = lateral occipital cortex; STG = superior temporal gyrus; MTG = middle temporal gyrus; M1 = primary motor cortex; CUN = cuneal cortex; FFG = fusiform gyrus; HPC = hippocampus; LG = lingual gyrus; IOG = inferior occipital gyrus; SOG = superior occipital gyrus. SUB = subcortex; CBM = cerebellum (reproduced from Groot, Csifcsák, et al. 2021 ).
To disentangle the complex interplay between mind wandering, executive functions, and behavior, we employed an fMRI version of a recently developed finger-tapping random-sequence generation task (FT-RSGT). This novel paradigm allows assessment of ongoing fluctuations in task-related and task-unrelated attentional states through self-reports and sensitive indices of task performance at high temporal resolution. Concurrent fMRI and pupillometric measures were used to investigate the neural substrates of direct and indirect markers of mind wandering.
Participants completed interleaved blocks of two different finger-tapping tasks, one where they performed alternating sequences and one that required randomized responding, that were otherwise identical in terms of stimulus presentation, task pacing, and thought probe frequency. We therefore hypothesized that the difference in neural recruitment between the two tasks should be mainly reflected in the activation of brain regions associated with executive functioning. Indeed, our results demonstrate greater involvement of attention and executive control networks during the generation of random sequences compared to alternating finger-tapping. Specifically, activation was localized in brain regions previously implicated in random number generation, including the right superior parietal lobe and intraparietal sulcus, primary and supplementary motor areas, right dorsolateral prefrontal cortex (DLPFC), anterior insula, and cerebellum ( Mattay et al. 1998 ; Jahanshahi et al. 2000 ; Gountouna et al. 2010 ). The DLPFC has been proposed to play an especially important role in the suppression of repetition in response patterns ( Jahanshahi et al. 1998 ; Jahanshahi et al. 2000 ; Joppich et al. 2004 ; Capone et al. 2014 ) and is a major node in the frontoparietal control network (FPCN) that is typically associated with strategic planning and goal-directed cognition. Thus, the coordinated activity of the DLPFC together with somatomotor areas, insula, and cerebellum likely orchestrates the complex behavior required for this task, including the evaluation and selection of spatiotemporal motor actions, suppression of sequence reiterations, and synchronization of responses to an externally-cued rhythm.
In addition, we observed a similar pattern of frontoparietal cortical activation associated with increases in sequence randomness, including the right intraparietal sulcus, right posterior middle frontal gyrus, and left medial supplementary motor area. This is consistent with an early study employing a finger-tapping task combined with random sequence generation ( Schubert et al. 1998 ) and suggests that these regions are especially important for self-determined action planning and execution. In particular, the intraparietal sulcus has been argued to serve important integrative functions of sensorimotor information required to monitor the ongoing movement sequence and adapt new movements according to the randomness criterion ( Schubert et al. 1998 ; Tanabe et al. 2005 ). However, the absence of correlated activity in the DLPFC with sequence randomness was surprising given previous findings ( Jahanshahi et al. 2000 ; Joppich et al. 2004 ). Interestingly, a recent study reported a similar dissociation as anodal tDCS of the left DLPFC failed to modulate sequence randomness as measured with approximate entropy ( Boayue et al. 2020 ). Since we did not find evidence for a difference in mind wandering propensity between the two tasks, it is possible that the DLPFC is not necessary for the optimization of sequence entropy but is rather involved in the task-specific coordination and distribution of executive control processes that may also periodically engage in task-unrelated processes such as mind wandering. This interpretation is in line with the proposed role of the DLPFC in context regulation ( Turnbull et al. 2019 ) and conforms to the observation that anodal tDCS of the DLPFC reduced involuntary shifts in task-unrelated attention ( Boayue et al. 2020 ).
Decreases in randomness, as would be expected to occur when thoughts drift away from the task as subjects engage in mind wandering, were instead associated with activity in the left inferior temporal sulcus and left superior and inferior occipital gyri. Although these regions are not typically associated with decrements in task performance, a large-scale meta-analysis ( Spreng et al. 2002 ) reported that the left inferior temporal sulcus is often associated with autobiographical memory retrieval and default mode functions. However, the ordinal regression analysis did not reveal a clear relationship between approximate entropy of the tapping-sequence and mind wandering, suggesting that mind wandering was less detrimental to this aspect of task performance compared to the maintenance of synchronized finger-tapping to the metronome as reflected in the strong relationship with behavioral variability. As we also observed generally higher tapping variability during the random task, participants were possibly more strongly engaged in maintaining task performance by optimizing sequence randomness, leading to the deterioration of tapping rhythmicity.
When executive task demands were low and participants were required to simply produce an alternating finger-tapping sequence, greater activity was observed in the core nodes of the DMN, namely the posterior cingulate cortex/precuneus and left angular gyrus. Given the context-regulation hypothesis, which states that the propensity to mind wander is adaptively adjusted to environmental demands ( Smallwood and Andrews-Hanna 2013 ), it is conceivable that the less effortful alternating finger-tapping task, as opposed to random sequence-generation, was more facilitative of mind wandering as reflected in greater DMN activation ( Mason et al. 2007 ). However, as we failed to observe activation of the DMN directly preceding self-reports of mind wandering, or any difference in mind wandering propensity between the two tasks, an alternative explanation may be that DMN recruitment rather indicated a greater reliance on automated behavior ( Shamloo and Helie 2016 ; Scheibner et al. 2017 ; Vatansever et al. 2017 ). This explanation is in line with our observation of correlated activity in the DMN (ventromedial prefrontal cortex and left superior frontal gyrus) when the rhythm of finger-tapping was more synchronized with the metronome, which is in agreement with previous findings characterizing stable or “in-the-zone” behavior ( Esterman et al. 2013 , 2014 ; Kucyi et al. 2016 ; Kucyi et al. 2017 ; Yamashita et al. 2020 ). Together, these results suggest that automatic and repetitive behavior that is considered less effortful might be governed by the DMN and fluctuations in behavioral variability may provide a sensitive marker for changes in attention as reflected in neural correlates of attention rather than sensorimotor networks. Furthermore, increases in finger-tapping variability were predictive of self-reported mind wandering episodes throughout the task, highlighting the robustness of this relationship across different tasks and studies ( Bastian and Sackur 2013 ; Hawkins et al. 2019 ; Boayue et al. 2020 ) and additionally substantiating behavioral variability as a sensitive marker for departures from task-focused attention.
We did not observe a difference in mind wandering propensity between the two task conditions, which is surprising given previous studies demonstrating an effect of task difficulty on mind wandering ( Seli, Konishi, et al. 2018 ; Brosowsky et al. 2021 ). One possible explanation as to why levels of mind wandering during the less demanding alternating finger-tapping task were similar to those during the random task is that exposure to the former, easier task was limited. Specifically, alternating blocks composed only one-third of the experiment and were pseudo-randomized, making it unlikely for two alternating blocks to occur sequentially. Therefore, occasional periods of 1-min lasting alternating finger-tapping were possibly not long enough to induce significantly more mind wandering, resulting in comparable levels of mind wandering between the two tasks.
Contrary to expectations, the patterns of neural recruitment directly preceding mind wandering self-reports and during periods of increased tapping variability did not converge. However, the observed divergence in our study is less surprising given the results of a previous study employing a continuous performance task ( Kucyi et al. 2016 ), in which the authors demonstrated greater activation of the DMN in relation to self-reported mind wandering as well as stable performance even though mind wandering was preceded by increases in response variability. Combined with our results, these findings suggest a certain level of independence in the relationships between the DMN and mind wandering on the one hand, and between the DMN and behavioral variability on the other. Although the authors of that study report DMN activation prior to mind wandering, our results failed to show such a relationship. Instead, we observed that mind wandering self-reports were preceded by local activation of the left inferior occipital gyrus and cerebellum during the alternating task and the right striatum during the random task. Regardless of the discrepancy with the neural recruitment during task performance, these results are puzzling on their own as they strongly deviate from the neural regions typically associated with mind wandering and spontaneous thought ( Christoff et al. 2009 ; Fox et al. 2015 ). However, several studies indicate that cerebellar regions are functionally connected to cortical intrinsic connectivity networks, including the DMN ( Habas et al. 2009 ; Buckner et al. 2011 ; Vatansever et al. 2015 ; Habas 2021 ), revealing a role for the cerebellum in cognition. In addition, earlier findings are suggestive of a role for the striatum in brain state maintenance through connections with the insula in order to sustain mind wandering episodes ( Tang et al. 2012 ; Chou et al. 2017 ) and a recent study reported the thalamus and basal forebrain as subcortical nodes of the DMN ( Alves et al. 2019 ). Furthermore, a recent study analyzing the dynamics within and between large-scale networks observed that mind wandering interacted with changes in the segregation and integration of visual and subcortical networks ( Zuberer et al. 2021 ). Specifically, mind wandering was associated with higher levels of integration of the visual network compared to optimal sustained attention, whereas the subcortical network showed stronger segregation, suggesting that visual and subcortical system dynamics are sensitive to perturbations from mind wandering. Hence, although the role of cerebellar and subcortical regions and cortico-subcortical network interactions in mind wandering is currently understudied, these findings warrant consideration for future research.
It should be noted that there exists a large heterogeneity in the design and the direct or indirect measurement of mind wandering across previous studies and different forms of mind wandering or spontaneous thought can be discerned based on their neural correlates ( Fox et al. 2015 ). In addition, some researchers have proposed a distinction between stimulus-independent versus stimulus-oriented mind wandering ( Gilbert et al. 2007 ; Maillet et al. 2017 ), a dimension that cannot be directly investigated in most continuous performance and sustained attention tasks that implement ongoing stimulus delivery. Instead, the FT-RSGT can be considered mostly a stimulus-independent paradigm and thus task performance in general relies more prominently on internal representations. An intriguing speculation arising from these considerations is that network configurations supporting such representations might be similar for task-related and task-unrelated processes, which would explain the absence in neural contrast. Alternatively, the divergence in brain activation identified through direct (self-report) versus indirect (objective) measures may arise from the difference in how they relate to its heterogeneous phenomenological aspects. For example, experience sampling may capture a wide variety of types of spontaneous thought, including episodes that are brief versus prolonged, aware versus unaware, and deliberate versus involuntary. Indeed, there is evidence that the spontaneous generation of mind wandering and its subjective experience are separable components ( Smallwood et al. 2007 ) that can also be distinguished on the neural level ( Christoff et al. 2009 ). In contrast, indices of objective performance may consistently “catch” a distinct and uniform aspect of mind wandering, such as its depth or intensity. As there is evidence that mind wandering without meta-awareness is more disruptive of task performance ( Smallwood et al. 2007 , 2008 ), increases in behavioral variability may especially reflect deep and unaware episodes of task disengagement. Future studies are necessary to further investigate these hypotheses.
Finally, we investigated the neural correlates of changes in slowly-fluctuating pupil dilations and constrictions as well as changes in the amplitude of evoked transient responses to task-related events as derivatives of tonic and phasic LC/NE dynamics, respectively ( Aston-Jones and Cohen 2005 ). In agreement with previous reports, spontaneous tonic pupil dilations were correlated with activity in occipito-temporal regions, thalamus, brainstem, and cerebellum, whereas negative correlations were observed within widespread visual and somatomotor cortical areas ( Murphy et al. 2014 ; Yellin et al. 2015 ; Schneider et al. 2016 ; DiNuzzo et al. 2019 ). Especially, the involvement of the LC and thalamus is unsurprising given their known role as drivers of cortical arousal and neural gain that is necessary for optimized task performance ( Aston-Jones et al. 1991 ; Aston-Jones and Cohen 2005 ; Saper et al. 2005 ) as well as the proposed role of the thalamus in orchestrating attentional switches between internally versus externally-directed awareness ( Wang et al. 2014 ; Cunningham et al. 2016 ; Sweeney-Reed et al. 2017 ) and directing attention to episodic memories ( Leszczynski and Staudigl 2016 ). In addition, the somatosensory cortices have been previously associated with spontaneous thought ( Fox et al. 2015 ) as well as visual imagery and thoughts relating to body-centered information during mind wandering ( Delamillieure et al. 2010 ; Fox et al. 2013 ). As tonic pupil size was negatively related to self-reported mind wandering, the observed activation of somatosensory cortices in association with tonic pupil constriction could therefore reflect involvement in mind wandering episodes.
Interestingly, occipital activation was frequently either directly or indirectly associated with mind wandering, including when sequence randomness decreased (left superior and inferior occipital gyri), during tonic (lateral occipital cortices) and phasic (right inferior and bilateral superior occipital gyri) pupil constriction, and preceding mind wandering reports in the alternating task (left inferior occipital gyrus), possibly suggesting similar underlying cognitive states. It has been argued that through cortical feedback mechanisms, the occipital cortex may play a role in cognition independent from perceptual input, such as internal visual representations that transpire during mental imagery and mind wandering ( Kosslyn et al. 2001 ; Petro et al. 2016 ). Together with previous work, these findings provide important insights into how tonic and phasic pupil dynamics may operate as indicators of task-unrelated mental states such as mind wandering ( Mittner et al. 2016 ; Konishi et al. 2017 ; Unsworth and Robison 2018 ; Groot, Boayue, et al. 2021 ). The ordinal regression analysis furthermore revealed a significant interaction between the two pupil components, suggesting that phasic responses only demonstrate a negative relationship with mind wandering when tonic pupil size is increased. This is in line with a recently proposed model of mind wandering based on the adaptive gain theory ( Mittner et al. 2016 ), which poses that two distinct task-unrelated states (“active mind wandering” and “off-focus”) are distinguishable based the level of tonic NE. Specifically, active mind wandering is characterized by similar tonic levels as the on-task state reflecting optimal neural gain and arousal, whereas the off-focus state represents an exploratory mode during which brain networks reconfigure to select relevant behavioral goals. If we assume that participants had generally low levels of arousal and vigilance during the FT-RSGT, the observed high levels of tonic pupil size could possibly reflect optimal levels of tonic NE, during which the amplitude of phasic responses maximally discriminate between active mind wandering (low phasic responses indicate task disengagement and perceptual decoupling) and on-task (high phasic responses reflect task-focused attention).
In summary, we demonstrated that the FT-RSGT relies on the recruitment of attentional and executive control networks, providing evidence for our hypothesis that the generation of random as opposed to alternating finger-tapping sequences requires the use of executive resources. Secondly, we observed positive significant relationships between self-reported episodes of mind wandering and time-on-task as well as behavioral variability, replicating earlier findings and validating the use of this task as an fMRI paradigm ( Boayue et al. 2020 ). Finally, we replicated at least partially the neural correlates of indirect markers of mind wandering and arousal state using sensitive indices of behavioral performance and pupillometric measures as derivatives for LC/NE functioning. In contrast, neither the previously reported cortical networks underlying mind wandering, nor the activation patterns associated with task performance could be observed in the neural contrasts preceding thought probes, suggesting a dissociation between indirect and direct (subjective) measures that may underline the vulnerability of thought probing for disentangling the neural underpinning of this heterogeneous mental state. Together, our results add to the growing body of work to better understand the mechanisms of ongoing fluctuations in attention and how various markers of mind wandering relate to each other at both the behavioral and neural level.
Supplementary Material
Grootjm_etal_2021_supplementary_material_bhab494, supplementb_bhab494, supplementc_bhab494, contributor information.
Josephine M Groot, Department of Psychology, UiT – The Arctic University of Norway, Tromsø 9037 , Norway. Integrative Model-Based Cognitive Neuroscience Research Unit, University of Amsterdam, Amsterdam 1018 WB , The Netherlands.
Gábor Csifcsák, Department of Psychology, UiT – The Arctic University of Norway, Tromsø 9037 , Norway.
Sven Wientjes, Department of Experimental Psychology, University of Ghent, Ghent 9000 , Belgium.
Birte U Forstmann, Integrative Model-Based Cognitive Neuroscience Research Unit, University of Amsterdam, Amsterdam 1018 WB , The Netherlands.
Matthias Mittner, Department of Psychology, UiT – The Arctic University of Norway, Tromsø 9037 , Norway.
Netherlands Organization of Scientific Research (NWO; grant number 016.Vici.185.052 to B.U.F.).
We would like to thank Steven Miletic and Dr Pierre-Louis Bazin from the Integrative Model-based Cognitive Neuroscience research unit at the University of Amsterdam for their help in the preparations and pilot experiments and for the insightful advice regarding the fMRI analyses conducted in this paper, respectively. Conflict of Interest : None declared.
- Alexandersen A, Csifcsák G, Mittner M. 2021. The effect of transcranial direct current stimulation on the interplay between executive control, behavioral variability, and mind wandering: a registered report . PsyArXiv Preprints. 10.31234/osf.io/qfcm9. [ PubMed ] [ CrossRef ] [ Google Scholar ]
- Alkemade A, Mulder MJ, Groot JM, Isaacs BR, Van Berendonk N, Lute N, Isherwood SJS, Bazin P-L, Forstmann BU. 2020. The Amsterdam ultra-high field adult lifespan database (AHEAD): a freely available multimodal 7 tesla submillimeter magnetic resonance imaging database . NeuroImage . 221 :117200. [ PubMed ] [ Google Scholar ]
- Alves PN, Foulon C, Karolis V, Bzdok D, Margulies DS, Volle E, De Schotten MT. 2019. An improved neuroanatomical model of the default-mode network reconciles previous neuroimaging and neuropathological findings . Commun Biol . 2 :370. [ PMC free article ] [ PubMed ] [ Google Scholar ]
- Aston-Jones G, Cohen JD. 2005. An integrative theory of locus coeruleus-norepinephrine function: adaptive gain and optimal performance . Ann Rev Neurosci . 28 :403–450. [ PubMed ] [ Google Scholar ]
- Aston-Jones G, Chiang C, Alexinsky T. 1991. Discharge of noradrenergic locus coeruleus neurons in behaving rats and monkeys suggests a role in vigilance . Prog Brain Res . 88 :501–520. [ PubMed ] [ Google Scholar ]
- Avants B, Epstein C, Grossman M, Gee J. 2008. Symmetric diffeomorphic image registration with cross-correlation: evaluating automated labeling of elderly and neurodegenerative brain . Med Image Anal . 12 :26–41. [ PMC free article ] [ PubMed ] [ Google Scholar ]
- Baddeley A, Emslie H, Kolodny J, Duncan J. 1998. Random generation and the executive control of working memory . Q J Exp Psychol A . 51 :819–852. [ PubMed ] [ Google Scholar ]
- Banks JB, Welhaf MS, Hood AVB, Boals A, Tartar JL. 2016. Examining the role of emotional valence of mind wandering: all mind wandering is not equal . Conscious Cogn . 43 :167–176. [ PubMed ] [ Google Scholar ]
- Bastian M, Sackur J. 2013. Mind wandering at the fingertips: automatic parsing of subjective states based on response time variability . Front Psychol . 4 :573. [ PMC free article ] [ PubMed ] [ Google Scholar ]
- Beckmann CF, Jenkinson M, Smith SM. 2003. General multilevel linear modeling for group analysis in FMRI . NeuroImage . 20 :1052–1063. [ PubMed ] [ Google Scholar ]
- Boayue NM, Csifcsák G, Aslaksen P, Turi Z, Antal A, Groot J, Hawkins GE, Forstmann B, Opitz A, Thielscher A, et al. 2019. Increasing propensity to mind-wander by transcranial direct current stimulation? A registered report . Eur J Neurosci . 1–19. [ PubMed ] [ Google Scholar ]
- Boayue NM, Csifcsák G, Kreis IV, Schmidt C, Finn I, Vollsund AEH, Mittner M. 2020. The interplay between executive control, behavioral variability and mind wandering: insights from a high-definition transcranial direct-current stimulation study . Eur J Neurosci . 53 :1498–1516. [ PubMed ] [ Google Scholar ]
- Brosowsky NP, Murray S, Schooler JW, Seli P. 2021. Thought dynamics under task demands: evaluating the influence of task difficulty on unconstrained thought . J Exp Psychol . 47 :1298–1312. [ PubMed ] [ Google Scholar ]
- Buckner RL, Krienen FM, Castellanos A, Diaz JC, Yeo BTT. 2011. The organization of the human cerebellum estimated by intrinsic functional connectivity . J Neurophysiol . 106 :2322–2345. [ PMC free article ] [ PubMed ] [ Google Scholar ]
- Bürkner P-C. 2017. Brms: an R package for Bayesian multilevel models using Stan . J Stat Softw . 80 :1–28. [ Google Scholar ]
- Bürkner P-C, Vuorre M. 2019. Ordinal regression models in psychology: a tutorial Adv Meth Pract Psychol Sci. 2 :77–101. [ Google Scholar ]
- Callard F, Smallwood J, Golchert J, Margulies DS. 2013. The era of the wandering mind? Twenty-first century research on self-generated mental activity . Front Psychol . 4 :891. [ PMC free article ] [ PubMed ] [ Google Scholar ]
- Capone F, Capone G, Ranieri F, Di Pino G, Oricchio G, Di Lazzaro V. 2014. The effect of practice on random number generation task: a transcranial direct current stimulation study . Neurobiol Learn Mem . 114 :51–57. [ PubMed ] [ Google Scholar ]
- Chou Y-H, Sundman M, Whitson HE, Gaur P, Chu M-L, Weingarten CP, Madden DJ, Wang L, Kirste I, Joliot M, et al. 2017. Maintenance and representation of mind wandering during resting-state fMRI . Sci Rep . 7 :40722. [ PMC free article ] [ PubMed ] [ Google Scholar ]
- Christoff K. 2012. Undirect thought: neural determinants and correlates . Brain Res . 1428 :51–59. [ PubMed ] [ Google Scholar ]
- Christoff K, Gordon AM, Smallwood J, Smith R, Schooler JW. 2009. Experience sampling during fMRI reveals default network and executive system contributions to mind wandering . Proc Natl Acad Sci U S A . 106 :8719–8724. [ PMC free article ] [ PubMed ] [ Google Scholar ]
- Cox RW, Hyde JS. 1997. Software tools for analysis and visualization of fMRI data . NMR Biomed . 10 :171–178. [ PubMed ] [ Google Scholar ]
- Cunningham SI, Tomasi D, Volkow ND. 2016. Structural and functional connectivity of the precuneus and thalamus to the default mode network . Hum Brain Mapp . 38 :938–956. [ PMC free article ] [ PubMed ] [ Google Scholar ]
- Delamillieure P, Doucet G, Mazoyer B, Turbelin MR, Delcroix N, Mellet E, Zago L, Crivello F, Petit L, Tzourio-Mazoyer N, et al. 2010. The resting state questionnaire: an introspective questionnaire for evaluation of inner experience during the conscious resting state . Brain Res Bull . 81 :565–573. [ PubMed ] [ Google Scholar ]
- Diedrichsen J, Balsters JH, Flavell J, Cussans E, Ramnani N. 2009. A probabilistic MR atlas of the human cerebellum . NeuroImage . 46 :39–46. [ PubMed ] [ Google Scholar ]
- DiNuzzo M, Mascali D, Moraschi M, Bussu G, Maugeri L, Mangini F, Fratini M, Giove F. 2019. Brain networks underlying eye’s pupil dynamics . Front Neurosci . 13 :965. [ PMC free article ] [ PubMed ] [ Google Scholar ]
- Dixon ML, De La Vega A, Mills C, Andrews-Hanna J, Spreng RN, Cole MW, Christoff K. 2018. Heterogeneity within the frontoparietal control network and its relationship to the default and dorsal attention networks . Proc Natl Acad Sci U S A . 115 :1598–1607. [ PMC free article ] [ PubMed ] [ Google Scholar ]
- Esteban O, Markiewicz CJ, Blair RW, Moodie CA, Isik AI, Erramuzpe A, Kent JD, Goncalves M, DuPre E, Snyder M, et al. 2018. fMRIPrep: a robust preprocessing pipeline for functional MRI . Nat Methods . 16 :111–116. [ PMC free article ] [ PubMed ] [ Google Scholar ]
- Esterman M, Noonan SK, Rosenberg M, Degutis J. 2013. In the zone or zoning out? Tracking behavioral and neural fluctuations during sustained attention . Cereb Cortex . 23 :2712–2723. [ PubMed ] [ Google Scholar ]
- Esterman M, Rosenburg MD, Noonan SK. 2014. Intrinsic fluctuations in sustained attention and distractor processing . J Neurosci . 34 :1724–1730. [ PMC free article ] [ PubMed ] [ Google Scholar ]
- Fonov VS, Evans AC, McKinstry RC, Almli CR, Collins DL. 2009. Unbiased nonlinear average age-appropriate brain templates from birth to adulthood . NeuroImage . 47 :102. [ Google Scholar ]
- Fox KCR, Nijeboer S, Solomonova E, Domhoff GW, Christoff K. 2013. Dreaming as mind wandering: evidence from functional neuroimaging and first-person content reports . Front Hum Neurosci . 7 :412. [ PMC free article ] [ PubMed ] [ Google Scholar ]
- Fox KCR, Spreng RN, Ellamil M, Andrews-Hanna JR, Christoff K. 2015. The wandering brain: meta-analysis of functional neuroimaging studies of mind-wandering and related spontaneous thought processes . NeuroImage . 111 :611–621. [ PubMed ] [ Google Scholar ]
- Friston KJ, Worsley KJ, Frackowiak RS, Mazziotta JC, Evans AC. 1994. Assessing the significance of focal activations using their spatial extent . Hum Brain Mapp . 1 :210–220. [ PubMed ] [ Google Scholar ]
- Gilbert SJ, Dumontheil I, Simons JS, Frith CD, Burgess PW. 2007. Comment on “wandering minds: the default mode network and stimulus-independent thought” . Science . 317 :43. [ PubMed ] [ Google Scholar ]
- Gorgolewski K, Burns CD, Madison C, Clark D, Halchenko YO, Waskom ML, Ghosh SS. 2011. Nipype: a flexible, lightweight and extensible neuroimaging data processing framework in python . Front Neuroinform . 5 :13. [ PMC free article ] [ PubMed ] [ Google Scholar ]
- Gountouna V-E, Job DE, McIntosh AM, Moorhead TWJ, Lymer GKL, Whalley HC, Hall J, Waiter GD, Brennan D, McGonigle DJ, et al. 2010. Functional magnetic resonance imaging (fMRI) reproducibility and variance components across visits and scanning sites with a finger tapping task . NeuroImage . 49 :552–560. [ PubMed ] [ Google Scholar ]
- Grandchamp R, Braboszcz C, Delorme A. 2014. Oculometric variations during mind wandering . Front Psychol . 5 :31. [ PMC free article ] [ PubMed ] [ Google Scholar ]
- Greve DN, Fischl B. 2009. Accurate and robust brain image alignment using boundary-based registration . NeuroImage . 48 :63–72. [ PMC free article ] [ PubMed ] [ Google Scholar ]
- Groot JM, Boayue NM, Csifcsák G, Boekel W, Huster R, Forstmann BU, Mittner M. 2021. Probing the neural signature of mind wandering with simultaneous fMRI-EEG and pupillometry . NeuroImage . 224 :117412. [ PubMed ] [ Google Scholar ]
- Groot JM, Csifcsák G, Wientjes S, Forstmann BU, Mittner M. 2021. Materials for “catching wandering minds with tapping fingers: neural and behavioral insights into task-unrelated cognition” . Open Science Framework . 10.17605/OSF.IO/56FCX [ PMC free article ] [ PubMed ] [ CrossRef ] [ Google Scholar ]
- Habas C. 2021. Functional connectivity of the cognitive cerebellum . Front Sys Neurosci . 15 :642225. [ PMC free article ] [ PubMed ] [ Google Scholar ]
- Habas C, Kamdar N, Nguyen D, Prater K, Beckmann CF, Menon V, Greicius MD. 2009. Distinct cerebellar contributions to intrinsic connectivity networks . J Neurosci . 29 :8586–8594. [ PMC free article ] [ PubMed ] [ Google Scholar ]
- Hawkins GE, Mittner M, Forstmann BU, Heathcote A. 2019. Modeling distracted performance . Cogn Psychol . 112 :48–80. [ PubMed ] [ Google Scholar ]
- Hoeks B, Levelt WJM. 1993. Pupillary dilation as a measure of attention: a quantitative system analysis . Behav Res Methods . 25 :16–26. [ Google Scholar ]
- Jahanshahi M, Profice P, Brown RG, Ridding MC, Dirnberger G, Rothwell JC. 1998. The effects of transcranial magnetic stimulation over the dorsolateral prefrontal cortex on suppression of habitual counting during random number generation . Brain . 121 :1533–1544. [ PubMed ] [ Google Scholar ]
- Jahanshahi M, Dirnberger G, Fuller R, Firth CD. 2000. The role of the dorsolateral prefrontal cortex in random number generation: a study with positron emission tomography . NeuroImage . 12 :713–725. [ PubMed ] [ Google Scholar ]
- Jahanshahi M, Saleem T, Ho AK, Dirnberger G, Fuller R. 2006. Random number generation as an index of controlled processing . Neuropsychology . 20 :391–399. [ PubMed ] [ Google Scholar ]
- Jenkinson M, Smith SM. 2001. A global optimisation method for robust affine registration of brain images . Med Image Anal . 5 :143–156. [ PubMed ] [ Google Scholar ]
- Jenkinson M, Bannister P, Brady JM, Smith SM. 2002. Improved optimisation for the robust and accurate linear registration and motion correction of brain images . NeuroImage . 17 :825–841. [ PubMed ] [ Google Scholar ]
- Joppich G, Däuper J, Dengler R, Johannes S, Rodriguez-Fornells A, Münte TF. 2004. Brain potentials index executive functions during random number generation . Neurosci Res . 49 :157–164. [ PubMed ] [ Google Scholar ]
- Joshi S, Li Y, Kalwani RM, Gold JI. 2016. Relationship between pupil diameter and neuronal activity in the locus coeruleus, colliculi, and cingulate cortex . Neuron . 89 :221–234. [ PMC free article ] [ PubMed ] [ Google Scholar ]
- Jubera-Garcia E, Gevers W, Van Opstal F. 2019. Influence of content and intensity of thought on behavioral and pupil changes during active mind wandering, off-focus, and on-task states . Atten Percept Psychophys . 82 :1125–1135. [ PMC free article ] [ PubMed ] [ Google Scholar ]
- Kane MJ, McVay JC. 2012. What mind wandering reveals about executive-control abilities and failures . Curr Dir Psychol Sci . 21 :348–354. [ Google Scholar ]
- Killingsworth MA, Gilbert DT. 2010. A wandering mind is an unhappy mind . Science . 330 :932. [ PubMed ] [ Google Scholar ]
- Konishi M, Brown K, Battaglini L, Smallwood J. 2017. When attention wanders: pupillometric signatures of fluctuations in external attention . Cognition . 168 :16–26. [ PubMed ] [ Google Scholar ]
- Kosslyn SM, Ganis G, Thompson WL. 2001. Neural foundations of imagery . Nat Rev Neurosci . 2 :635–642. [ PubMed ] [ Google Scholar ]
- Kucyi A, Esterman M, Riley CS, Valera EM. 2016. Spontaneous default network activity reflects behavioral variability independent of mind-wandering . Proc Natl Acad Sci . 113 :13899–13904. [ PMC free article ] [ PubMed ] [ Google Scholar ]
- Kucyi A, Hove MJ, Esterman M, Hutchison RM, Valera EM. 2017. Dynamic brain network correlates of spontaneous fluctuations in attention . Cereb Cortex . 27 :1831–1840. [ PMC free article ] [ PubMed ] [ Google Scholar ]
- Laeng B, Sirois S, Gredebäck G. 2012. Pupillometry: a window to the preconscious? Perspect Psychol Sci . 7 :18–27. [ PubMed ] [ Google Scholar ]
- Lawson C, Hanson RJ. 1987. Solving least squares problems . Philadelphia (PA): SIAM. [ Google Scholar ]
- Leszczynski M, Staudigl T. 2016. Memory-guided attention in the anterior thalamus . Neurosci Biobehav Rev . 66 :163–165. [ PubMed ] [ Google Scholar ]
- Liddell TM, Kruschke JK. 2018. Analyzing ordinal data with metric models: what could possibly go wrong? J Exp Soc Psychol . 79 :328–348. [ Google Scholar ]
- Maillet D, Seli P, Schacter DL. 2017. Mind-wandering and task stimuli: stimulus-dependent thoughts influence performance on memory tasks and are more often past- versus future-oriented . Conscious Cogn . 52 :55–67. [ PMC free article ] [ PubMed ] [ Google Scholar ]
- Mason MF, Norton MI, Van Horn JD, Wegner DM, Grafton ST, Macrae CN. 2007. Wandering minds: the default mode network and stimulus-independent thought . Science . 315 :393–395. [ PMC free article ] [ PubMed ] [ Google Scholar ]
- Mathôt S. 2013. A simple way to reconstruct pupil size during eye blinks . 10.6084/m9.figshare.688001. [ CrossRef ]
- Mattay VS, Callicott JH, Bertolino A, Santha AKS, Van Horn JD, Tallent KA, Frank JA, Weinberger DR. 1998. Hemispheric control of motor function: a whole brain echo planar fMRI study . Psychiatry Res Neuroimaging . 83 :7–22. [ PubMed ] [ Google Scholar ]
- McVay JC, Kane MJ. 2009. Conducting the train of thought: working memory capacity, goal neglect, and mind wandering in an executive-control task . J Exp Psychol Learn Mem Cogn . 35 :196–204. [ PMC free article ] [ PubMed ] [ Google Scholar ]
- McVay JC, Kane MJ. 2010. Does mind wandering reflect executive function or executive failure? Comment on Smallwood and Schooler (2006) and Watkins (2008) . Psychol Bull . 136 :188–207. [ PMC free article ] [ PubMed ] [ Google Scholar ]
- Mittner M. 2020. Pypillometry: a python package for pupillometric analyses . J Open Source Soft . 5 :2348. [ Google Scholar ]
- Mittner M. 2021. Estimation of tonic and phasic pupillometric signals . Figshare . 10.6084/m9.figshare.17022104.v2. [ CrossRef ] [ Google Scholar ]
- Mittner M, Boekel W, Tucker AM, Turner BM, Heathcote A, Forstmann BU. 2014. When the brain takes a break: a model-based analysis of mind wandering . J Neurosci . 34 :16286–16295. [ PMC free article ] [ PubMed ] [ Google Scholar ]
- Mittner M, Hawkins GE, Boekel W, Forstmann BU. 2016. A neural model of mind wandering . Trends Cogn Sci . 20 :570–578. [ PubMed ] [ Google Scholar ]
- Murphy PR, O’Connell RG, O’Sullivan M, Robertson IH, Balsters JH. 2014. Pupil diameter covaries with BOLD activity in human locus coeruleus . Hum Brain Mapp . 35 :4140–4154. [ PMC free article ] [ PubMed ] [ Google Scholar ]
- Peirce JW. 2007. PsychoPy - psychophysics software in python . J Neurosci Methods . 162 :8–13. [ PMC free article ] [ PubMed ] [ Google Scholar ]
- Pelagatti C, Binda P, Vannucci M. 2020. A closer look at the timecourse of mind wandering: pupillary response and behavior . PLoS One . 15 :0226792. [ PMC free article ] [ PubMed ] [ Google Scholar ]
- Peters M, Giesbrecht T, Jelicic M, Merckelback H. 2007. The random number generation task: psychometric properties and normative data on an executive function task in a mixed sample . J Int Neuropsychol Soc . 13 :626–634. [ PubMed ] [ Google Scholar ]
- Petro LS, Paton AT, Muckli L. 2016. Contextual modulation of primary visual cortex by auditory signals . Phil Trans R Soc B . 372 :20160104. [ PMC free article ] [ PubMed ] [ Google Scholar ]
- Pincus S, Kalman RE. 1997. Not all (possibly) “random” sequences are created equal . Proc Natl Acad Sci U S A . 94 :3513–3518. [ PMC free article ] [ PubMed ] [ Google Scholar ]
- Raichle ME. 2015. The brain’s default mode network . Annu Rev Neurosci . 38 :433–447. [ PubMed ] [ Google Scholar ]
- Saper CB, Scammel TE, Lu J. 2005. Hypothalamic regulation of sleep and circadian rhythms . Nature . 437 :1257–1263. [ PubMed ] [ Google Scholar ]
- Scheibner HJ, Bogler C, Gleich T, Haynes JD, Bermpohl F. 2017. Internal and external attention and the default mode network . NeuroImage . 148 :381–389. [ PubMed ] [ Google Scholar ]
- Schneider M, Hathway P, Leuchs L, Sämann PG, Czisch M, Spoormaker VI. 2016. Spontaneous pupil dilations during resting state are associated with activation of the salience network . NeuroImage . 139 :189–201. [ PubMed ] [ Google Scholar ]
- Schooler JW, Smallwood J, Christoff K, Handy TC, Reichle ED, Sayette MA. 2011. Meta-awareness, perceptual decoupling and the wandering mind . Trends Cogn Sci . 15 :319–326. [ PubMed ] [ Google Scholar ]
- Schubert T, von Cramon DY, Niendorf T, Pollman S, Bublak P. 1998. Cortical areas and the control of self-determined finger movements . Neuroreport . 9 :3171–3176. [ PubMed ] [ Google Scholar ]
- Seli P, Cheyne JA, Smilek D. 2013. Wandering minds and wavering rhyhtms: linking mind wandering and behavioral variability . J Exp Psychol . 39 :1–15. [ PubMed ] [ Google Scholar ]
- Seli P, Risko EF, Smilek D. 2016. On the necessity of distinguishing between unintentional and intentional mind wandering . Psychol Sci . 27 :685–691. [ PubMed ] [ Google Scholar ]
- Seli P, Beaty RE, Cheyne JA, Smilek D, Oakman J, Schacter DL. 2018. How pervasive is mind wandering, really? Conscious Cogn . 66 :74–78. [ PubMed ] [ Google Scholar ]
- Seli P, Konishi M, Risko EF, Smilek D. 2018. The role of task difficulty in theoretical accounts of mind wandering . Conscious Cogn . 65 :255–262. [ PubMed ] [ Google Scholar ]
- Shamloo F, Helie S. 2016. Changes in default mode network as automaticity develops in a categorization task . Behav Brain Res . 313 :324–333. [ PubMed ] [ Google Scholar ]
- Shepard J. 2019. Why does the mind wander? Neurosci Conscious . 5 :014. [ PMC free article ] [ PubMed ] [ Google Scholar ]
- Smallwood J, Andrews-Hanna J. 2013. Not all minds that wander are lost: the importance of a balanced perspective on the mind-wandering state . Front Psychol . 4 :441. [ PMC free article ] [ PubMed ] [ Google Scholar ]
- Smallwood J, Schooler JW. 2006. The restless mind . Psychol Bull . 132 :946–958. [ PubMed ] [ Google Scholar ]
- Smallwood J, Schooler JW. 2015. The science of mind wandering: empirically navigating the stream of consciousness . Ann Rev Psychol . 66 :487–518. [ PubMed ] [ Google Scholar ]
- Smallwood J, Davies JB, Heim D, Finnigan F, Sudberry M, O’Connor R, Obonsawin M. 2004. Subjective experience and the attentional lapse: task engagement and disengagement during sustained attention . Conscious Cogn . 13 :657–690. [ PubMed ] [ Google Scholar ]
- Smallwood J, McSpadden M, Schooler JW. 2007. The lights are on but no one’s home: meta-awareness and the decoupling of attention when the mind wanders . Psychon Bull Rev . 14 :527–533. [ PubMed ] [ Google Scholar ]
- Smallwood J, McSpadden M, Luus B, Schooler J. 2008. Segmenting the stream of consciousness: the psychological correlates of temporal structures in the time series data of a continuous performance task . Brain Cogn . 66 :50–56. [ PubMed ] [ Google Scholar ]
- Smallwood J, Brown KS, Tipper C, Giesbrecht B, Franklin MS, Mrazek MD, Carlson JM, Schooler JW. 2011. Pupillometric evidence for the decoupling of attention from perceptual input during offline thought . PLoS One . 6 :18298. [ PMC free article ] [ PubMed ] [ Google Scholar ]
- Smallwood J, Brown KS, Baird B, Mrazek MD, Franklin MS, Schooler JW. 2012. Insulation for daydreams: a role for tonic norepinephrine in the facilitation of internally guided thoughts . PLoS One . 7 :33706. [ PMC free article ] [ PubMed ] [ Google Scholar ]
- Smith SM, Brady JM. 1997. SUSAN – a new approach to low level image processing . Int J Comput Vis . 23 :45–78. [ Google Scholar ]
- Spreng NR, Mar RA, Kim ASN. 2002. The common neural basis of autobiographical memory, prospection, navigation, theory of mind, and the default mode: a quantitative meta-analysis . J Cogn Neurosci . 21 :489–510. [ PubMed ] [ Google Scholar ]
- Sweeney-Reed CM, Zaehle T, Voges J, Schmitt FC, Buentjen L, Borchardt V, Walter M, Hinrichs H, Heinze H-J, Rugg MD, et al. 2017. Anterior thalamic high frequency band activity is coupled with theta oscillations at rest . Front Hum Neurosci . 11 :358. [ PMC free article ] [ PubMed ] [ Google Scholar ]
- Tanabe C, Kato M, Miyauchi S, Hayashi S, Yanagida T. 2005. The sensorimotor transformation of cross-modal spatial information in the anterior intraparietal sulcus as revealed by functional MRI . Cogn Brain Res . 22 :385–396. [ PubMed ] [ Google Scholar ]
- Tang YY, Rothbart MK, Posner MI. 2012. Neural correlates of establishing, maintaining, and switching brain states . Trends Cogn Sci . 16 :330–337. [ PMC free article ] [ PubMed ] [ Google Scholar ]
- Teasdale JD, Dritschel BH, Taylor MJ, Proctor L, Lloyd CA, Nimmo-Smith I, Baddeley AD. 1995. Stimulus-independent thought depends on central executive resources . Mem Cogn . 23 :551–559. [ PubMed ] [ Google Scholar ]
- Turnbull A, Wang H-T, Murphy C, Ho NSP, Wang X, Sormaz M, Karapanagiotidis T, Leech RM, Bernhardt B, Margulies DS, et al. 2019. Left dorsolateral prefrontal cortex supports context-dependent prioritisation of off-task thought . Nat Commun . 10 :3816. [ PMC free article ] [ PubMed ] [ Google Scholar ]
- Tustison NJ, Avants BB, Cook PA, Zheng Y, Egan A, Yushkevich PA. 2010. N4ITK: improved N3 bias correction . IEEE Trans Med Imaging . 29 :1310–1320. [ PMC free article ] [ PubMed ] [ Google Scholar ]
- Unsworth N, Robison MK. 2016. Pupillary correlates of lapses of sustained attention . Cogn Affect Behav Neurosci . 16 :601–615. [ PubMed ] [ Google Scholar ]
- Unsworth N, Robison MK. 2018. Tracking arousal state and mind wandering with pupillometry . Cogn Affect Behav Neurosci . 18 :638–664. [ PubMed ] [ Google Scholar ]
- Van Calster L, D’Argembeau A, Salmon E, Peters F, Majerus S. 2017. Fluctuations of attentional networks and default mode network during the resting state reflect variations in cognitive states: evidence from a novel resting-state experience sampling method . J Cogn Neurosci . 29 :95–113. [ PubMed ] [ Google Scholar ]
- Vatansever D, Menon DK, Manktelow AE, Sahakian BJ, Stamatakis EA. 2015. Default mode network connectivity during task execution . NeuroImage . 122 :96–104. [ PubMed ] [ Google Scholar ]
- Vatansever D, Menon DK, Stamatakis EA. 2017. Default mode contributions to automated information processing . Proc Natl Acad Sci U S A . 114 :12821–12826. [ PMC free article ] [ PubMed ] [ Google Scholar ]
- Wang X, Xu M, Song Y, Li X, Zhen Z, Yang Z, Liu J. 2014. The network property of the thalamus in the default mode network is correlated with trait mindfulness . Neuroscience . 278 :91–301. [ PubMed ] [ Google Scholar ]
- Weinstein Y. 2018. Mind-wandering, how do I measure three with probes? Let me count the ways . Behav Res Methods . 50 :642–661. [ PubMed ] [ Google Scholar ]
- Weinstein Y, De Lima HJ, Van Der Zee T. 2018. Are you mind-wandering, or is your mind on task? The effect of probe framing on mind-wandering reports . Psychon Bull Rev . 25 :754–760. [ PubMed ] [ Google Scholar ]
- Woolrich MW, Ripley BD, Brady M, Smith SM. 2001. Temporal autocorrelation in univariate linear modeling of FMRI data . NeuroImage . 14 :1370–1386. [ PubMed ] [ Google Scholar ]
- Yamashita A, Rothlein D, Kucyi A, Valera EM, Esterman M. 2021. Brain state-based detection of attentional fluctuations and their modulation . NeuroImage . 236 :118072. [ PubMed ] [ Google Scholar ]
- Yellin D, Berkovich-Ohana A, Malach R. 2015. Coupling between pupil fluctuations and resting-state fMRI uncovers a slow build-up of antagonistic responses in the human cortex . NeuroImage . 106 :414–427. [ PubMed ] [ Google Scholar ]
- Yeo BTT, Krienen FM, Sepulcre J, Sabuncu MR, Lashkari D, Hollinshead M, Roffman JL, Smoller JW, Zöllei L, Polimeni JR, et al. 2011. The organization of the human cerebral cortex estimated by intrinsic functional connectivity . J Neurophysiol . 106 :1125–1165. [ PMC free article ] [ PubMed ] [ Google Scholar ]
- Zanesco AP, Denkova E, Jha AP. 2020. Self-reported mind wandering and response time variability differentiate prestimulus electroencephalography microstate dynamics during a sustained attention task . J Cogn Neurosci . 33 :28–45. [ PubMed ] [ Google Scholar ]
- Zhang Y, Brady M, Smith S. 2001. Segmentation of brain MR images through a hidden markov random field model and the expectation-maximization algorithm . IEEE Trans Med Imag . 20 :45–57. [ PubMed ] [ Google Scholar ]
- Zuberer A, Kucyi A, Yamashita A, Wu CM, Walter M, Valera EM, Esterman M. 2021. Integration and segregation across large-scale intrinsic brain networks as a marker of sustained attention and task-unrelated thought . NeuroImage . 229 :117610. [ PubMed ] [ Google Scholar ]

IMAGES
VIDEO
COMMENTS
Mind wandering (MW) refers to the shift of attention away from a primary task towards thoughts unrelated to the task. ... Pupil diameter measures were transformed from pixels to millimeters after calibrating the tracker with an artificial 4 mm pupil, positioned at the approximate location of the subjects' left eye. Gaze position data were ...
Similarly, our findings indicate increases in baseline pupil size during mind wandering compared to task-focused attention, which may reflect higher levels of tonic NE and has been proposed to underlie the reduced sensitivity to external interference favoring mental exploration (Murphy et al., 2011; Smallwood et al., 2012a).
Pupil diameter preceding these control trials can also be seen as the timecourse that pupil diameter should regularly show in this experiment, without the intrusion of a mind wandering episode. Also, in this case, there was a relative pupil dilation in the trials preceding the MW report.
Examining differences in tonic pupil diameter for mind-wandering and mind-blanking reports as a function of orientation of attention suggested an effect of attentional state, F(1, 91) = 5.88, MSE = .36, p = .017, η p 2 = .06, in which mind-wandering reports were associated with larger pretrial pupil diameters (M = .11, SD = .53) than were mind ...
Several previous studies have established a correlation between pupil diameter and mind wandering (Smallwood et al., 2011, 2012; Franklin et al., 2013). The theoretical interpretation that is usually used to explain this effect is based on previously reported correlations between pupil diameter and activity of LC neurons ( Rajkowski et al ...
Pupillometry: The Eyes Shed Fresh Light on the Mind. Recent studies provide promising methodological advances in the use of pupillometry as on-line measurement of cognitive processes and show that visual attention allocation, mind-wandering, mental imagery, and even rhyme expectations can influence the size of the human pupil.
Furthermore, both mind-wandering and inattentive reports were associated with smaller pretrial baseline pupil diameters than reports of external distraction (both ps < .01), but pretrial pupil diameter for mind-wandering and inattentive reports were not different (p = .55). These results demonstrate that different types of lapses of attention ...
We conclude that pupil size provides an index of MW, objective and covert and that this may be exploited in further studies to understand whether and how MW affects the processing of sensory stimuli. Mind wandering (MW) refers to the shift of attention away from a primary task towards thoughts unrelated to the task.
Pupil size correlated with the subjects' performance and reaction time variability, two vastly studied indicators of attention. ... J. W. The science of mind wandering: empirically navigating ...
In addition, several studies have investigated pupil diameter in a mind-wandering context: an increase in tonic pupil diameter precedes mind wandering-related errors [14. Smallwood J. et al. Pupillometric evidence for the decoupling of attention from perceptual input during offline thought. PLoS One. 2011; 6: e18298.
In tasks that are demanding, such as our task, the dominant finding is that attentional lapses and mind wandering are associated with a smaller baseline pupil diameter than non-lapses or on-task thought (e.g. [11-13]).
Recent studies provide promising methodological advances in the use of pupillometry as on-line measurement of cognitive processes and show that visual attention allocation, mind-wandering, mental imagery, and even rhyme expectations can influence the size of the human pupil.
Mind wandering (MW) is a phenomenon in which a person is distracted from the external environment and ongoing tasks. Among these, unintentional MWs are known to be associated with Attention ...
A significant body of literature supports the contention that pupil size varies depending on cognitive load, affective state, and level of drowsiness. Here we assessed whether oculometric measures such as gaze position, blink frequency and pupil size were correlated with the occurrence and time course of self-reported mind-wandering episodes.
Together, these results indicate that the differences in pupil diameter associated with mind-wandering are fully explained by changes in daytime sleepiness. 3.3.2. Blink parameters. If blinking is specifically involved in the perceptual decoupling aspect of mind-wandering ...
There may be possible relationships between the mind-wandering and perceptual rivalry, given that physiological responses such as fluctuations in pupil diameter, which is an index of attentional/arousal states, are related to the occurrence of both phenomena.
A similar pattern has been observed across studies of mind-wandering, with some finding increased pupil diameter co-occurring with self-reports of off task/mind-wandering episodes (Franklin, Broadway, Mrazek, Smallwood, & Schooler, 2013), while others have found the reverse (Grandchamp, Braboszcz, & Delorme, 2014).
In the latter case, self-reported mind wandering echoed the pupil diameter changes in demonstrating greater off-task behavior when preparing for a prosaccade trial. More important, trial type effects in pupil diameter emerged only when participants reported being "on-task," but disappeared during periods of mind wandering. These results ...
the latter case, self-reported mind wandering echoed the pupil diameter changes in demonstrating greater off-task behavior when preparing for a prosaccade trial. More important, trial type effects in pupil diameter emerged only when participants reported being "on-task," but disappeared during periods of mind wandering.
We found robust linear relationships between baseline pupil diameter and several measures of task performance, suggesting that attentional lapses tended to occur when pupil diameter was small. ... Schooler JW (2013) Window to the wandering mind: pupillometry of spontaneous thought while reading. Q J Exp Psychol (Hove) 66: 2289-2294. View ...
A significant body of literature supports the contention that pupil size varies depending on cognitive load, affective state, and level of drowsiness. Here we assessed whether oculometric measures such as gaze position, blink frequency and pupil size were correlated with the occurrence and time course of self-reported mind-wandering episodes.
In 3 experiments, we examined fluctuations in working memory (WM) performance and associated changes in pretrial and task-evoked pupil diameter. Additionally, we examined whether particularly poor trials were accompanied by self-reports of off-task attentional states. The results demonstrated that task-evoked pupillary responses can be used to ...
However, research on the relationship between mind wandering and tonic pupil size has yielded more inconsistent results as both larger and smaller tonic pupils have been associated with mind wandering (Smallwood et al. 2012; Grandchamp et al. 2014; Konishi et al. 2017; Jubera-Garcia et al. 2019).