- Search Search Please fill out this field.
- Definitions O - Z

Round Trip Transaction Costs: Meaning, Profitability, Example
Julia Kagan is a financial/consumer journalist and former senior editor, personal finance, of Investopedia.
:max_bytes(150000):strip_icc():format(webp)/Julia_Kagan_BW_web_ready-4-4e918378cc90496d84ee23642957234b.jpg)
What Are Round Trip Transaction Costs?
Round trip transaction costs refer to all the costs incurred in a securities or other financial transaction. Round trip transaction costs include commissions , exchange fees, bid/ask spreads, market impact costs, and occasionally taxes. Since such transaction costs can erode a substantial portion of trading profits, traders and investors strive to keep them as low as possible. Round trip transaction costs are also known as round turn transaction costs.
Key Takeaways
- Round trip transaction costs refer to all the costs incurred in a financial transaction, such as commissions and exchange fees.
- Over the past two decades, round trip transaction costs have declined significantly due to the termination of fixed brokerage commissions, but still remain a factor to consider in purchasing a security.
- The concept of 'round trip transaction costs' is similar to that of the 'all-in cost,' which is every cost involved in a financial transaction.
How Round Trip Transaction Costs Work
The impact of round trip transaction costs depends on the asset involved in the transaction. Transaction costs in real estate investment, for instance, can be significantly higher as a percentage of the asset compared to securities transactions. This is because real estate transaction costs include registration fees, legal expenses, and transfer taxes, in addition to listing fees and agent's commission.
Round trip transaction costs have declined significantly over the past two decades due to the abolition of fixed brokerage commissions and the proliferation of discount brokerages . As a result, transaction costs are no longer the deterrent to active investing that they were in the past.
The concept of 'round trip transaction costs' is similar to that of the ' all-in cost ,' which is every cost involved in a financial transaction. The term 'all-in costs' is used to explain the total fees and interest included in a financial transaction, such as a loan or CD purchase, or in a securities trade.
Round Trip Transaction Costs and Profitability
When an investor buys or sells a security, they may enlist a financial advisor or broker to help them do so. That advisor or broker most likely will charge a fee for their services. In some cases, an advisor will enlist a broker to execute the transaction, which means the advisor, as well as the broker, will be able to charge a fee for their services in the purchase. Investors will have to factor in the cumulative costs to determine whether an investment was profitable or caused a loss.
Round Trip Transaction Costs Example
Shares of Main Street Public House Corp. have a bid price of $20 and an ask price of $20.10. There is a $10 brokerage commission . If you bought 100 shares, then quickly sell all of them at the bid and ask prices above, what would the round-trip transaction costs be?
Purchase: ($20.10 per share x 100 shares) + $10 brokerage commission = $2,020
Sale: ($20 per share x 100 shares) - $10 brokerage commission = $1,990
The round-trip transaction cost is: $2,020 - $1,990 = $30
Morningstar. " Morningstar's Annual Fund Fee Study Finds Investors Saved Nearly $6 Billion in Fund Fees in 2019 ."
:max_bytes(150000):strip_icc():format(webp)/Regularlyscheduledinvestments-ac483b861b3f4f8f83dfc56d70e80d14.jpg)
- Terms of Service
- Editorial Policy
- Privacy Policy
- Your Privacy Choices

What are Round-Trip Transactions?
Complete Explanation of Round Tripping including Purpose, Example, & Risks
Home › Finance › Corporate Finance › What are Round-Trip Transactions?
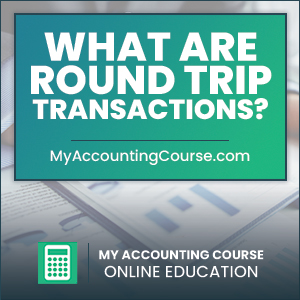
In the complex world of financial markets and corporate accounting, the term “round-trip transactions” often surfaces amidst discussions of financial ethics, regulatory compliance, and corporate governance.
These transactions, while not inherently illicit, tread a fine line between strategic financial management and the murky waters of manipulative practices.
This comprehensive guide aims to unravel the intricacies of round-trip transactions, shedding light on their purposes, risks, and the legal and ethical considerations they entail.
- Round-Trip Transactions Meaning
Key Takeaways
The purpose of round-trip transactions, how is round tripping used, round tripping example, the risks and implications of round-trip transactions, legal and regulatory framework, ethical considerations of round trip transactions, detecting and preventing round-trip transactions, what exactly defines a round-trip transaction in financial terms, why might a company engage in round-trip transactions, what are the potential risks of engaging in round-trip transactions, how can round-trip transactions be identified or prevented.
Round-trip transactions refer to a series of transactions in which a company sells an asset to another party with the agreement that the asset will be bought back at a later date, usually at a similar or predetermined price.
This cycle creates the appearance of genuine business activity without any substantive change in the company’s financial position or the asset’s ownership. While round-trip transactions span various industries, they are notably prevalent in the energy sector and financial markets, where companies might engage in these deals to inflate revenue figures or to create a facade of heightened market activity.
The distinction between legitimate and manipulative uses of round-trip transactions hinges on intent and disclosure. Legitimate uses are typically transparent and aim to achieve lawful financial or operational objectives, such as hedging against price fluctuations. Conversely, manipulative practices are designed to deceive stakeholders or regulatory bodies about a company’s true financial health or market activity.
Manipulative Impact on Financial Statements : Round-tripping is primarily used to artificially inflate a company’s revenue and trading volume, misleading stakeholders about the company’s true financial performance and market activity.
Legal and Ethical Risks : Engaging in round-trip transactions carries significant legal and ethical risks, including regulatory penalties and reputational damage, as these practices can be considered deceptive and manipulative.
Importance of Transparency and Regulation : The detection and prevention of round-trip transactions highlight the importance of transparent accounting practices and stringent regulatory oversight to ensure the integrity of financial markets and protect investor interests.
Round tripping is often used to artificially inflate a company’s revenue and trading volume, creating the appearance of a higher level of business activity than actually exists.
This practice can be employed to meet financial targets, influence stock prices, or enhance the attractiveness of the company to investors by manipulating financial statements. By artificially inflating revenue, a company can appear more financially robust and liquid than it truly is, potentially influencing stock prices and investor perception.
The allure of round-trip transactions lies in their ability to temporarily enhance a company’s financial standing without necessitating actual business growth or operational improvements. This can make a company more attractive to investors, lenders, and analysts in the short term, albeit at significant risk.
Companies might engage in round-trip transactions in several different ways. Here are the most common round-trip transactions:
Inflating Revenue : A company may engage in round-tripping by selling an asset to another entity and buying it back at a similar price. These transactions can be recorded as legitimate sales and purchases, artificially inflating the company’s revenue and sales volume without any real change in its economic situation, misleading stakeholders about the company’s financial performance.
Boosting Asset Turnover : By repeatedly selling and repurchasing assets in round-trip transactions, a company can give the impression of higher asset turnover than is actually the case. This can make the company appear more efficient in its use of assets, potentially misleading investors about its operational effectiveness.
Manipulating Market Activity: In the case of publicly traded companies, round-trip transactions can be used to create an illusion of heightened trading activity for the company’s shares. This can influence stock prices by suggesting a higher demand for the shares than actually exists, potentially attracting more investors based on misleading information.
An example of round-tripping involves a company, Company A, selling an asset to Company B for $1 million. Shortly thereafter, Company B sells the same asset back to Company A for approximately the same price, say $1.01 million.
This sequence of transactions makes it appear as though Company A has engaged in $1 million worth of sales, thereby inflating its revenue figures, even though there has been no real change in the economic position of either company.
This practice can be used to manipulate financial statements and give an inflated impression of the company’s financial health and trading volume, potentially misleading investors and regulators.
The primary risk associated with round-trip transactions is the potential for legal repercussions and loss of investor trust. Regulatory bodies in many jurisdictions scrutinize such practices closely, and companies found guilty of using round-trip transactions to manipulate financial outcomes can face hefty fines, legal sanctions, and reputational damage.
Notable incidents, such as the Enron scandal, highlight the catastrophic impact that deceptive financial practices can have on stock prices, market stability, and investor confidence.
Moreover, round-trip transactions can distort market perceptions, leading to inefficient capital allocation and undermining the integrity of financial markets. The artificial inflation of activity or liquidity can mislead stakeholders about market demand, price stability, and the true value of assets involved.
The legal status of round-trip transactions varies by jurisdiction, but there is a growing trend towards stricter regulation and oversight. Financial regulatory bodies worldwide have implemented guidelines and reporting requirements to curb the abuse of such transactions.
The role of auditors and financial regulators is pivotal in detecting manipulative practices, necessitating rigorous examination of financial records, transaction trails, and disclosure statements.
Beyond legal implications, round-trip transactions pose significant ethical dilemmas. The fine line between creative accounting and outright fraud is often blurred, challenging companies to maintain integrity and transparency in their financial reporting.
Ethical business practices and robust corporate governance structures are crucial in mitigating the temptation to engage in deceptive financial maneuvers.
Companies must foster a culture of honesty and accountability, ensuring that all stakeholders can rely on the veracity of financial statements and market activities.
For investors and regulators, identifying potential round-trip transactions involves scrutinizing sudden spikes in revenue or trading volume without corresponding changes in market conditions or company operations. Vigilance and due diligence are essential in assessing the authenticity of reported financial health and operational activity.
Companies, on their part, can prevent misuse by adopting transparent accounting practices, regularly auditing financial records, and ensuring that all transactions are conducted at arm’s length and properly disclosed. As the financial landscape evolves, so too must the strategies for maintaining fairness and integrity in corporate reporting and market transactions.
Round-trip transactions, while a legitimate tool in certain contexts, present a complex challenge in the realm of financial ethics and regulation. As companies navigate the pressures of financial performance and market competitiveness, the temptation to engage in such practices underscores the importance of robust regulatory frameworks, corporate governance, and ethical leadership.
The future of round-trip transactions will undoubtedly be shaped by ongoing efforts to balance financial innovation with transparency and integrity, ensuring the stability and trustworthiness of markets and corporate institutions. In this ever-changing environment, the collective responsibility of companies, regulators, and investors to foster transparency and integrity has never been more critical.
Frequently Asked Questions
A round-trip transaction refers to a set of transactions where an asset is sold and subsequently repurchased by the original seller, often at a similar price, to artificially inflate volume or revenue without any real change in asset ownership.
Companies may use round-trip transactions to meet financial targets or create the illusion of increased business activity, thereby enhancing their financial statements or market valuation temporarily.
Round-trip transactions can lead to legal penalties, reputational damage, and a loss of investor trust if used to manipulate financial statements or deceive stakeholders.
Identifying round-trip transactions involves scrutinizing financial records for transactions that inflate company activity without real economic substance, while prevention requires transparent accounting practices and rigorous financial oversight.
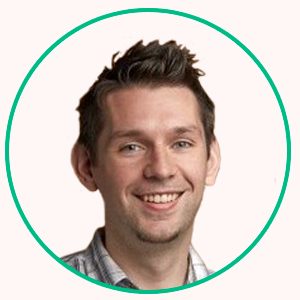
Accounting & CPA Exam Expert
Shaun Conrad is a Certified Public Accountant and CPA exam expert with a passion for teaching. After almost a decade of experience in public accounting, he created MyAccountingCourse.com to help people learn accounting & finance, pass the CPA exam, and start their career.
The Meaning of "Round Tripping" in Accounting
- Small Business
- Accounting & Bookkeeping
- ')" data-event="social share" data-info="Pinterest" aria-label="Share on Pinterest">
- ')" data-event="social share" data-info="Reddit" aria-label="Share on Reddit">
- ')" data-event="social share" data-info="Flipboard" aria-label="Share on Flipboard">
Does a Sales Discount Go on an Unadjusted Trial Balance?
Advantages and disadvantages of for-profit companies, accounting for an operating patent.
- What Does a Debit Balance in Manufacturing Overhead Do?
- How to Calculate Market Value of a Corporation
The accounting slang term "round tripping" refers to a series of transactions between companies that bolster the revenue of the companies involved but that, in the end, don't provide real economic benefit to either company. While not necessarily illegal, round tripping is at best disingenuous. It's important for a scrupulous businessperson to be able to recognize when a transaction may amount to round tripping – and, just as important, to know when a transaction that may look like round tripping is in fact legitimate.
Name Origins of "Round Tripping"
The name for the practice comes from "round trip" – a journey that takes you from one place to another and then back to your starting point. In essence, that's what's happening with financial round tripping: The companies involved start out in a certain financial position, and then they engage in a series of transactions. When all those transactions are settled, they're in the same financial position they were in when they started. They've made a "round trip." Such deals are also known as "lazy Susans."
Round Tripping: Meaning
A common round-tripping maneuver involves reciprocal sales of identical assets. Imagine you own an office supply store, and the owner of a stationery store comes to you with a proposal. He'll buy a pallet (40 cases) of copy paper from you at your retail price of $30 a case, for a total of $1,200. Meanwhile, you'll buy a pallet of the identical paper from him at the same price, $1,200. He's proposing a round-tripping deal. Other, more flagrant examples might include mutual payments for nonexistent "services," or one company "investing" in another, with the second company turning around and using the money to buy goods or services from the first.
Purpose of Round Tripping
According to Accounting Tools , the point of round tripping is to inflate revenue – to make a company look like it's doing more business than it really is. Although the bottom line for any company is profit, observers often judge a company's growth and size by its sales revenue. Round-trip deals generally don't inflate profit; after all, the $1,200 in revenue you would earn from selling paper to the stationery store would be offset by the $1,200 expense of buying an equal amount of paper. But each company gets that additional $1,200 in revenue, so it appears a little bigger and more active than it would without the round trip.
Legitimate Round Tripping Transactions
Say your office supply store has a delivery van whose tires are shot. You go to the tire shop next door and buy a new set of radials for $600. Three weeks later, the owner of the tire shop comes in and buys paper, printer ink, pens and other stuff worth $600 for his business. This would not be a round-trip situation. Each of you had a legitimate business purpose for these transactions; the fact that you both wound up with $600 in revenue and $600 in expenses, for a net profit of zero, is immaterial. Round tripping is about setting up reciprocal deals with no legitimate business purpose.
Legality of Round Tripping
The fact that legitimate deals can look like lazy Susans makes it hard to simply outlaw the practice. That said, WallStreet Mojo indicates that if a company is using self-canceling transactions to make its numbers look better to investors or lenders (which is the purpose of round tripping), it can be prosecuted for fraud. For instance, the Securities and Exchange Commission might charge several software companies relating to a round tripping fraud scheme for a series of transactions that an agency says provides no economic benefit and are intended only to boost the company's reported revenue.
- Accounting Tools: Round Tripping Definition
- WallStreet Mojo: Round Tripping
Related Articles
Why is the accrual basis of accounting accepted by gaap, difference between cash flow & sales revenue, is it ethical to record the transactions directly into the general ledger accounts, accounting definition of self balancing accounts, post-dump buyout strategy, difference in validate & verify in accounting, examples of aggressive accounting, examples of corporate malfeasance, what is a negative goodwill in accounting, most popular.
- 1 Why Is the Accrual Basis of Accounting Accepted by GAAP?
- 2 Difference Between Cash Flow & Sales Revenue
- 3 Is It Ethical to Record the Transactions Directly Into the General Ledger Accounts?
- 4 Accounting Definition of Self Balancing Accounts
- Round Trip Transaction
Get free proposals from vetted lawyers in our marketplace.
Technology Terms Glossary
- Personal License
- Public Domain
- Pull Strategy
- Pump and Dump
- Push Strategy
- Reverse Engineering
- Semiconductor
- Smart Contracts
- Software Bugs
- Source Code
What is a Round Trip Transaction?
Round trip transaction refers to the total incurred cost in a securities or similar financial transaction. Round trip transactions ultimately increase money flow in the market during the recession. These transactions also increase liquidity in an adverse market situation. You can use round-trip transactions in standard tax planning programs.
Round Trip Transaction Examples
Examples of the round trip transaction include:
- Example 1: Exchange fees
- Example 2: Commissions
- Example 3: Market impact costs
- Example 4: Bid or Ask spreads
- Example 5: Occasional taxes
Here is a comprehensive article about the round trip transaction.
Meet some of our Technology Lawyers
I was born and raised in Wayne, New Jersey and attended Seton Hall University, graduating cum laude. I followed my family down to Florida to attend Ave Maria School of Law where I graduated cum laude. I was admitted to the Florida Bar in 2018. During law school, I participated in the Certified Legal Internship program with the State Attorney's Office of the 20th Judicial Circuit and litigated 5 jury trials, 1 non jury trial and argued various motions before the court under the supervision of an Assistant State Attorney. I was an Assistant States Attorney for Collier County from 2018 to 2020 before moving into private practice in the areas of real estate and first party property from 2020 to 2021. As of November 2021, I started my own law practice that focuses on business planning, real estate and estate planning.
I work with private tech companies on entity formation, corporate governance, and commercial agreements. I was an in-house counsel for a unicorn fintech startup and am currently associated with a startup boutique while operating my solo practice. I received my JD from Berkeley Law, and served in the US Navy for 5 years as a combat linguist. I am fluent in Korean.
Whitney L. Smith's journey from entrepreneur to advocate is fueled by a profound understanding of the business world. With a decade of firsthand entrepreneurial experience, she entered law school driven by a mission to protect others' businesses. However, her passion for real estate law blossomed as she recognized the tremendous benefits rental property ownership offers to individuals seeking passive income and community development. Blending her deep understanding of transactional law with zealous courtroom advocacy, she empowers landlords to thrive. Born and raised in St. Petersburg, Florida, she is a proud graduate of Stetson College of Law and cherishes her role as a devoted parent to two children and a beloved pit bull companion.
Business, Real Estate, Tax, Estate Planning and Probate attorney with over 20 years experience in private practice in Colorado. Currently owner/operator of John M. Vaughan, Attorney at Law solo practitioner located in Boulder, CO. My practice focuses on transactional matters only.
I have 20-plus years of experience as a corporate general counsel, for public and private corporations, domestic and international. I have acted as corporate secretary for a publicly-held corporation and have substantial experience in corporate finance, M&A, corporate governance, incorporations, corporate maintenance, complex transactions, corporate termination and restructuring, as well as numerous aspects of regulatory and financial due diligence. In my various corporate roles, I have routinely drafted complex corporate contracts and deal-related documents such as stock purchase agreements, option and warrant agreements, MSAs, SOWs, term sheets, joint venture agreements, tender agreements purchase and sale agreements, technology licensing agreements, vendor agreements, service agreements, IP and technology security agreements, NDAs, etc. and have managed from both a legal and business perspective many projects in the financial, technology, energy and venture capital fields.
My practice focuses on business and commercial litigation. I have worked with companies of all sizes from sole member LLCs to those in the Fortune 500. I've advised clients on mergers, equity issuances, commercial transactions, joint ventures, employment issues, and non-competition. I've also drafted and negotiated the underlying agreements for these transactions and more.
Find the best lawyer for your project
Technology lawyers by city.
- Atlanta Technology Lawyers
- Austin Technology Lawyers
- Boston Technology Lawyers
- Chicago Technology Lawyers
- Dallas Technology Lawyers
- Denver Technology Lawyers
- Fort Lauderdale Technology Lawyers
- Houston Technology Lawyers
- Las Vegas Technology Lawyers
- Los Angeles Technology Lawyers
- Memphis Technology Lawyers
- Miami Technology Lawyers
- New York Technology Lawyers
- Oklahoma City Technology Lawyers
- Orlando Technology Lawyers
- Philadelphia Technology Lawyers
- Phoenix Technology Lawyers
- Richmond Technology Lawyers
- Salt Lake City Technology Lawyers
- San Antonio Technology Lawyers
- San Diego Technology Lawyers
- San Francisco Technology Lawyers
- Seattle Technology Lawyers
- Tampa Technology Lawyers

Quick, user friendly and one of the better ways I've come across to get ahold of lawyers willing to take new clients.
Contracts Counsel was incredibly helpful and easy to use. I submitted a project for a lawyer's help within a day I had received over 6 proposals from qualified lawyers. I submitted a bid that works best for my business and we went forward with the project.
I never knew how difficult it was to obtain representation or a lawyer, and ContractsCounsel was EXACTLY the type of service I was hoping for when I was in a pinch. Working with their service was efficient, effective and made me feel in control. Thank you so much and should I ever need attorney services down the road, I'll certainly be a repeat customer.
I got 5 bids within 24h of posting my project. I choose the person who provided the most detailed and relevant intro letter, highlighting their experience relevant to my project. I am very satisfied with the outcome and quality of the two agreements that were produced, they actually far exceed my expectations.
How It Works
Post Your Project
Get Free Bids to Compare
Hire Your Lawyer
Find lawyers and attorneys by city
- it Italiano
- fr Français
- ru Русский
- es Español
- zh 中文
- hi हिन्दी
- ar العربية
- pt Português
- ms Bahasa Melayu
- ko 한국어
- tr Türkçe
- ja 日本語
- nl Nederlands
- is Íslenska
Round Trip Transaction Costs
What Are Round Trip Transaction Costs?
Round trip transaction costs allude to all the costs incurred in a securities or other financial transaction. Round trip transaction costs include commissions , exchange fees, bid/ask spreads, market impact costs, and occasionally taxes. Since such transaction costs can dissolve a substantial portion of trading profits, traders and investors endeavor to keep them as low as could really be expected. Round trip transaction costs are otherwise called round turn transaction costs.
How Round Trip Transaction Costs Work
The impact of round trip transaction costs relies upon the asset involved in the transaction. Transaction costs in real estate investment, for instance, can be fundamentally higher as a percentage of the asset compared to securities transactions. This is on the grounds that real estate transaction costs include registration fees, legal expenses, and transfer taxes, as well as listing fees and specialist's commission.
Round trip transaction costs have declined fundamentally throughout the course of recent a long time due to the nullification of fixed brokerage commissions and the multiplication of discount brokerages . Subsequently, transaction costs are at this point not the obstacle to active investing that they were in the past.
The concept of 'round trip transaction costs' is like that of the ' all-in cost ,' which is each cost involved in a financial transaction. The term 'all-in costs' is utilized to explain the total fees and interest included in a financial transaction, like a loan or CD purchase, or in a securities trade.
Round Trip Transaction Costs and Profitability
At the point when an investor trades a security, they might enroll a financial advisor or broker to assist them with doing so. That advisor or broker undoubtedly will charge a fee for their services. At times, an advisor will enroll a broker to execute the transaction, and that means the advisor, as well as the broker, will actually want to charge a fee for their services in the purchase. Investors should factor in the cumulative costs to determine whether an investment was profitable or caused a loss.
Round Trip Transaction Costs Example
Shares of Main Street Public House Corp. have a bid price of $20 and a ask price of $20.10. There is a $10 brokerage commission . In the event that you bought 100 shares, immediately sell all of them at the bid and ask prices above, what might the round-trip transaction costs be?
Purchase: ($20.10 per share x 100 shares) + $10 brokerage commission = $2,020
Deal: ($20 per share x 100 shares) - $10 brokerage commission = $1,990
The round-trip transaction cost is: $2,020 - $1,990 = $30
- The concept of 'round trip transaction costs' is like that of the 'all-in cost,' which is each cost involved in a financial transaction.
- Throughout recent many years, round trip transaction costs have declined fundamentally due to the termination of fixed brokerage commissions, yet at the same time remain a factor to think about in purchasing a security.
- Round trip transaction costs allude to all the costs incurred in a financial transaction, for example, commissions and exchange fees.
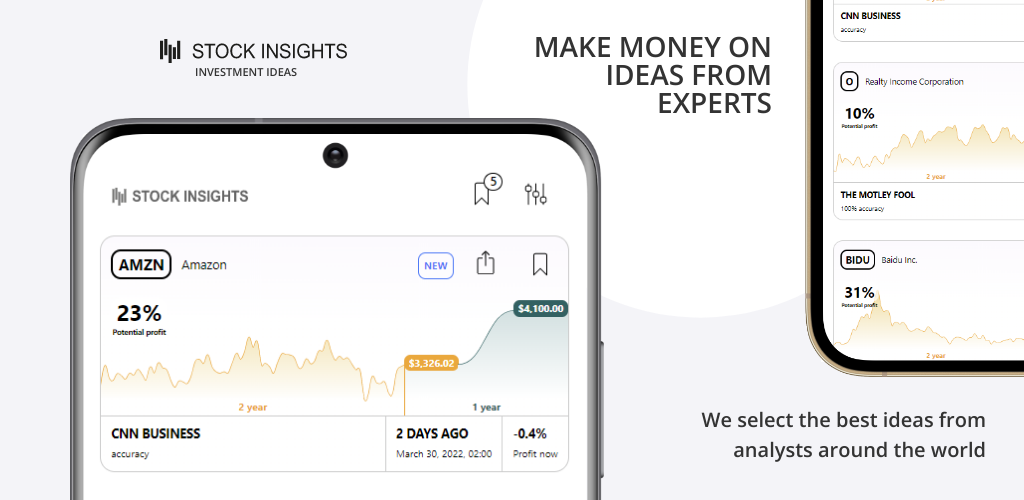
We couldn’t find any results matching your search.
Please try using other words for your search or explore other sections of the website for relevant information.
We’re sorry, we are currently experiencing some issues, please try again later.
Our team is working diligently to resolve the issue. Thank you for your patience and understanding.
Round-trip transactions costs
Costs of completing a transaction , including commissions , market impact costs , and taxes.
- Earnings per share (EPS)
- Market capitalization
- Outstanding
- Market value
- Over-the-counter (OTC)
- Sexvigintillion
- National Association of Securities Dealers (NASD)
Copyright © 2018, Campbell R. Harvey. All Worldwide Rights Reserved. Do not reproduce without explicit permission.
- Type a symbol or company name. When the symbol you want to add appears, add it to My Quotes by selecting it and pressing Enter/Return.
These symbols will be available throughout the site during your session.
Your symbols have been updated
Edit watchlist.
- Type a symbol or company name. When the symbol you want to add appears, add it to Watchlist by selecting it and pressing Enter/Return.
Opt in to Smart Portfolio
Smart Portfolio is supported by our partner TipRanks. By connecting my portfolio to TipRanks Smart Portfolio I agree to their Terms of Use .

Financial Tips, Guides & Know-Hows
Home > Finance > What Are Transaction Costs? Definition, How They Work, And Example
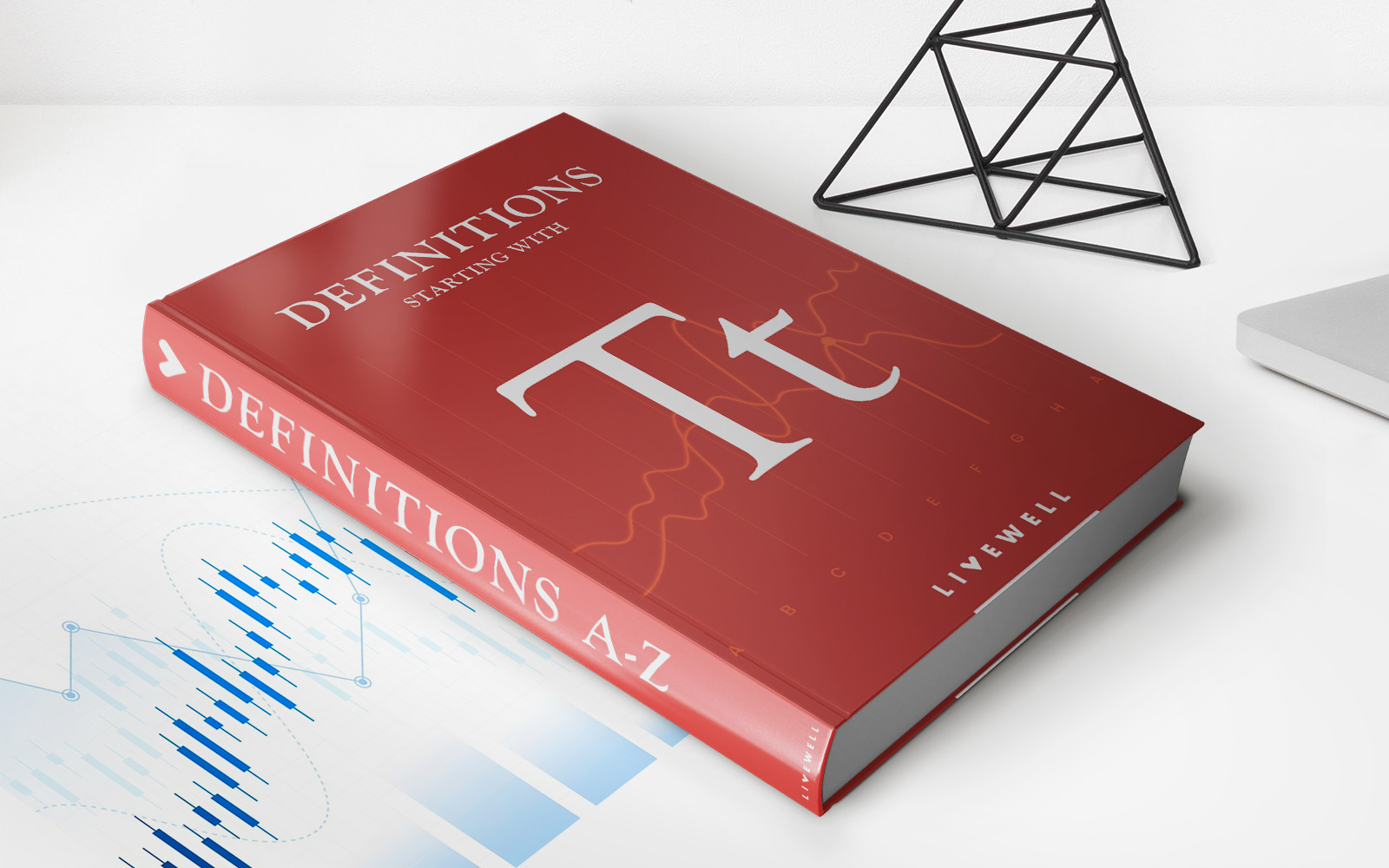
What Are Transaction Costs? Definition, How They Work, And Example
Published: February 10, 2024
Learn about transaction costs in finance, including their definition, how they work, and example scenarios. Enhance your financial knowledge with this comprehensive guide.
- Definition starting with T
(Many of the links in this article redirect to a specific reviewed product. Your purchase of these products through affiliate links helps to generate commission for LiveWell, at no extra cost. Learn more )
Understanding Transaction Costs in Finance
Whether you’re a seasoned investor or just starting to dip your toes into the world of finance, understanding transaction costs is crucial. As an expert in finance, I’m here to demystify this concept and shed light on what transaction costs are, how they work, and provide a real-life example. So, let’s dive in!
Key Takeaways:
- Transaction costs refer to the expenses incurred when buying or selling financial assets.
- They can include brokerage fees, commissions, bid-ask spreads, and taxes.
What Are Transaction Costs?
Transaction costs are the expenses associated with buying or selling financial assets such as stocks, bonds, or mutual funds. These costs can eat into your investment returns, so it’s essential to factor them into your decision-making process. While they may seem insignificant at first, transaction costs can add up over time, impacting your overall investment performance.
How Do Transaction Costs Work?
Transaction costs encompass various fees and charges that investors pay during the buying or selling process. Let’s break down some of the most common transaction costs:
- Brokerage Fees: When you trade through a broker, they charge a fee for their services. These fees can be fixed or based on a percentage of the trade value.
- Commissions: Commissions are fees paid to brokers or agents for facilitating trade. They can also be fixed or variable based on the size of the transaction.
- Bid-Ask Spreads: This refers to the difference between the highest price a buyer is willing to pay (bid) and the lowest price a seller is willing to accept (ask). The bid-ask spread represents a cost since you might have to buy at a slightly higher price or sell at a slightly lower price.
- Taxes: Depending on your jurisdiction, you may be subject to tax liabilities when buying or selling certain financial assets.
It’s important to note that transaction costs can vary depending on the size of the trade, the type of financial asset, and the specific brokerage firm or platform used. So, it’s crucial to research and understand the fee structure of your chosen broker before making any transactions.
An Example of Transaction Costs:
Let’s say you’re an investor looking to buy 100 shares of a company’s stock. Each share is valued at $50. Here’s a breakdown of the transaction costs involved:
- Brokerage fee: $10 flat fee per trade
- Commission: 1% of the trade value ($50 x 100 x 1% = $50)
- Bid-Ask Spread: $0.20 per share on both the buy and sell side ($0.20 x 100 = $20)
- Taxes: Assuming a tax rate of 10%, the tax liability would be $5 ($50 x 100 x 10% = $500 x 10% = $5)
Adding up these costs, we get:
- Total transaction costs = $10 (brokerage fee) + $50 (commission) + $20 (bid-ask spread) + $5 (taxes) = $85
So, in this example, the transaction costs amount to $85. It’s important to consider these costs when evaluating the profitability of your investment.
In Conclusion
Transaction costs are a reality of the financial markets. Understanding how they work and factoring them into your investment decisions is crucial for achieving your financial goals. By being aware of the various costs involved and considering them in your calculations, you can make more informed investment choices. Remember, every dollar saved on transaction costs adds to your overall returns in the long run.
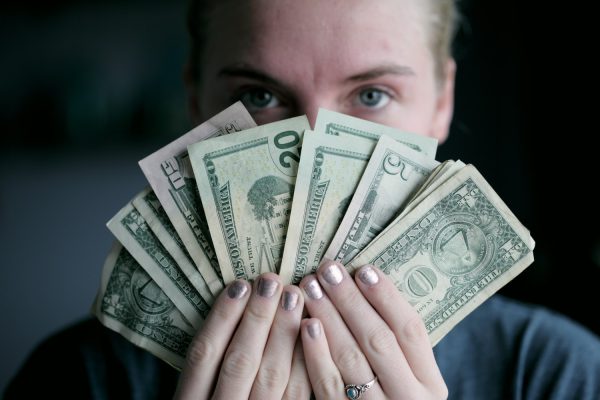
20 Quick Tips To Saving Your Way To A Million Dollars
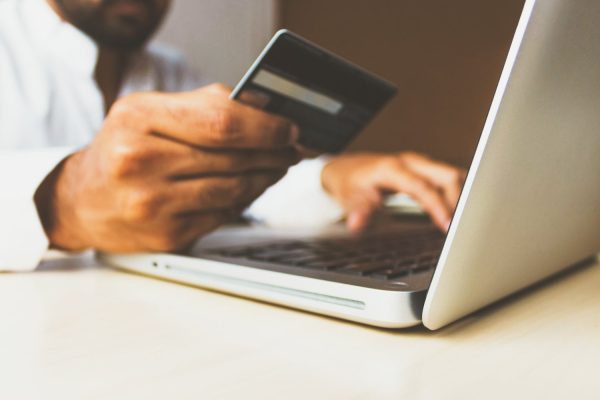
Our Review on The Credit One Credit Card
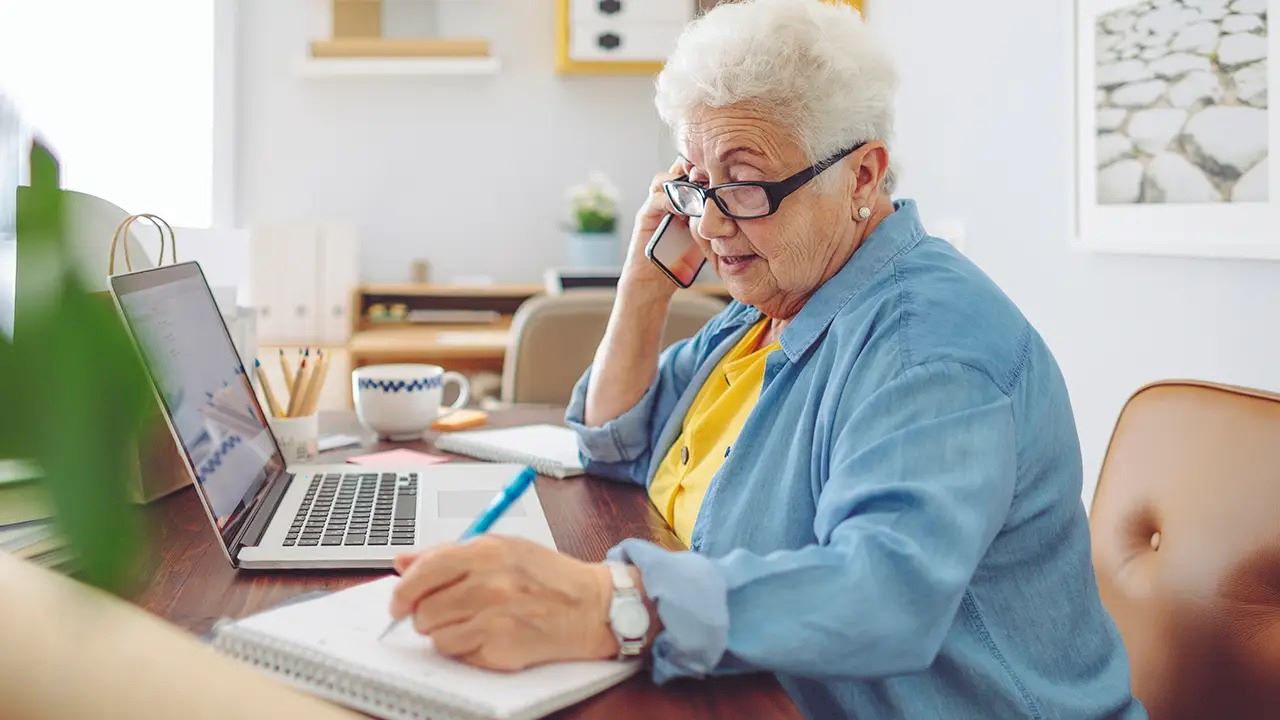
How Must A Replacing Producer Respond To An Applicant Wishing To Replace Existing Life Insurance?
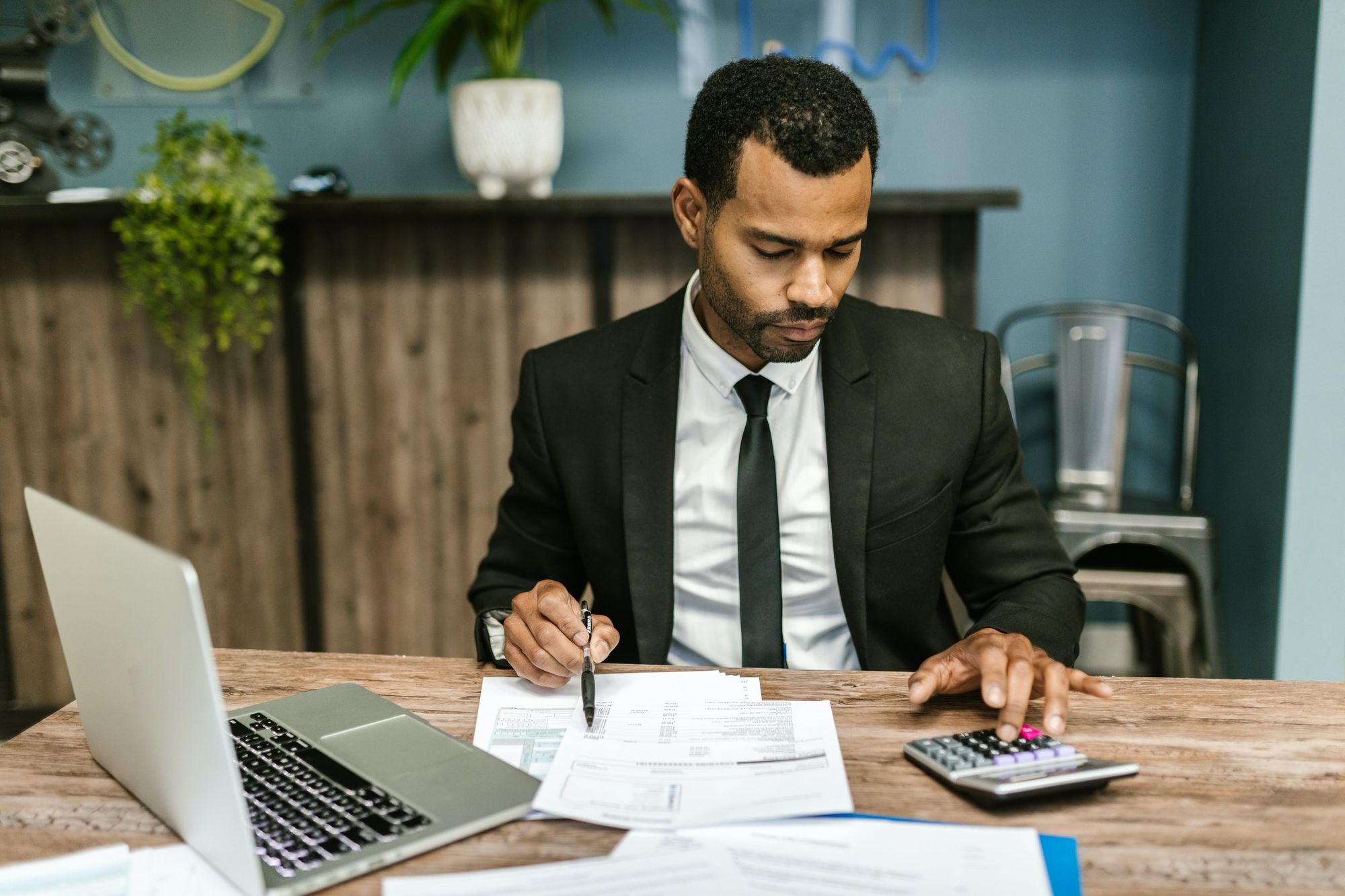
Why Is Using Debt In Capital Structure Good
Latest articles.
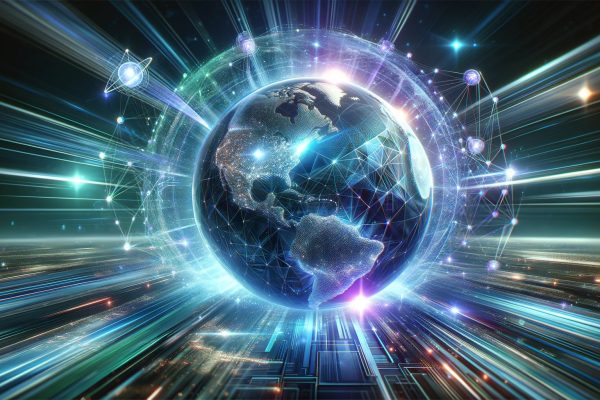
Understanding XRP’s Role in the Future of Money Transfers
Written By:
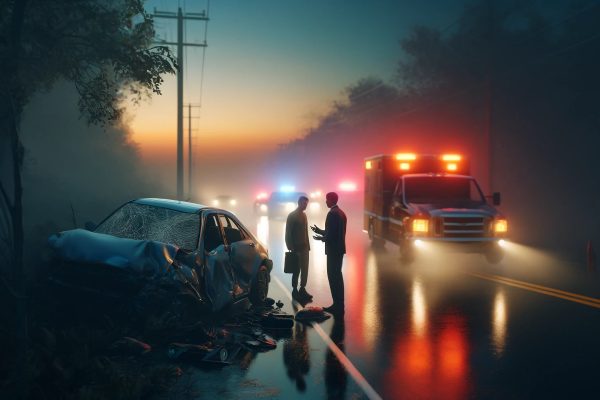
Navigating Post-Accident Challenges with Automobile Accident Lawyers
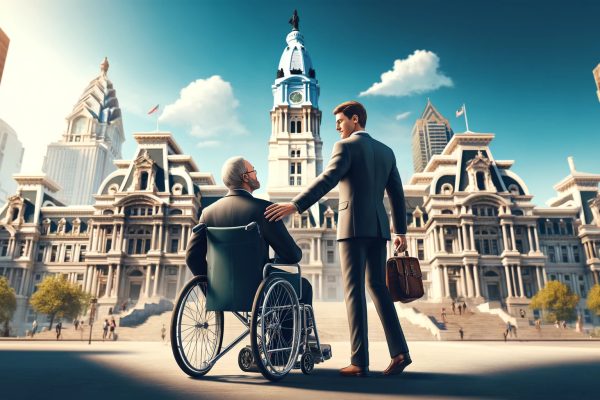
Navigating Disability Benefits Denial in Philadelphia: How a Disability Lawyer Can Help
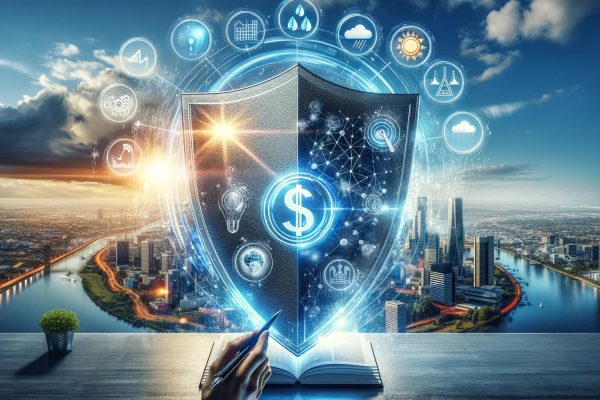
Preparing for the Unexpected: Building a Robust Insurance Strategy for Your Business
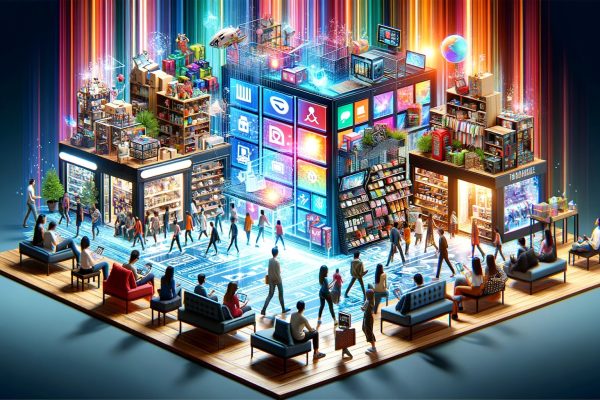
Custom Marketplace Development: Creating Unique Online Shopping Experiences
Related post.
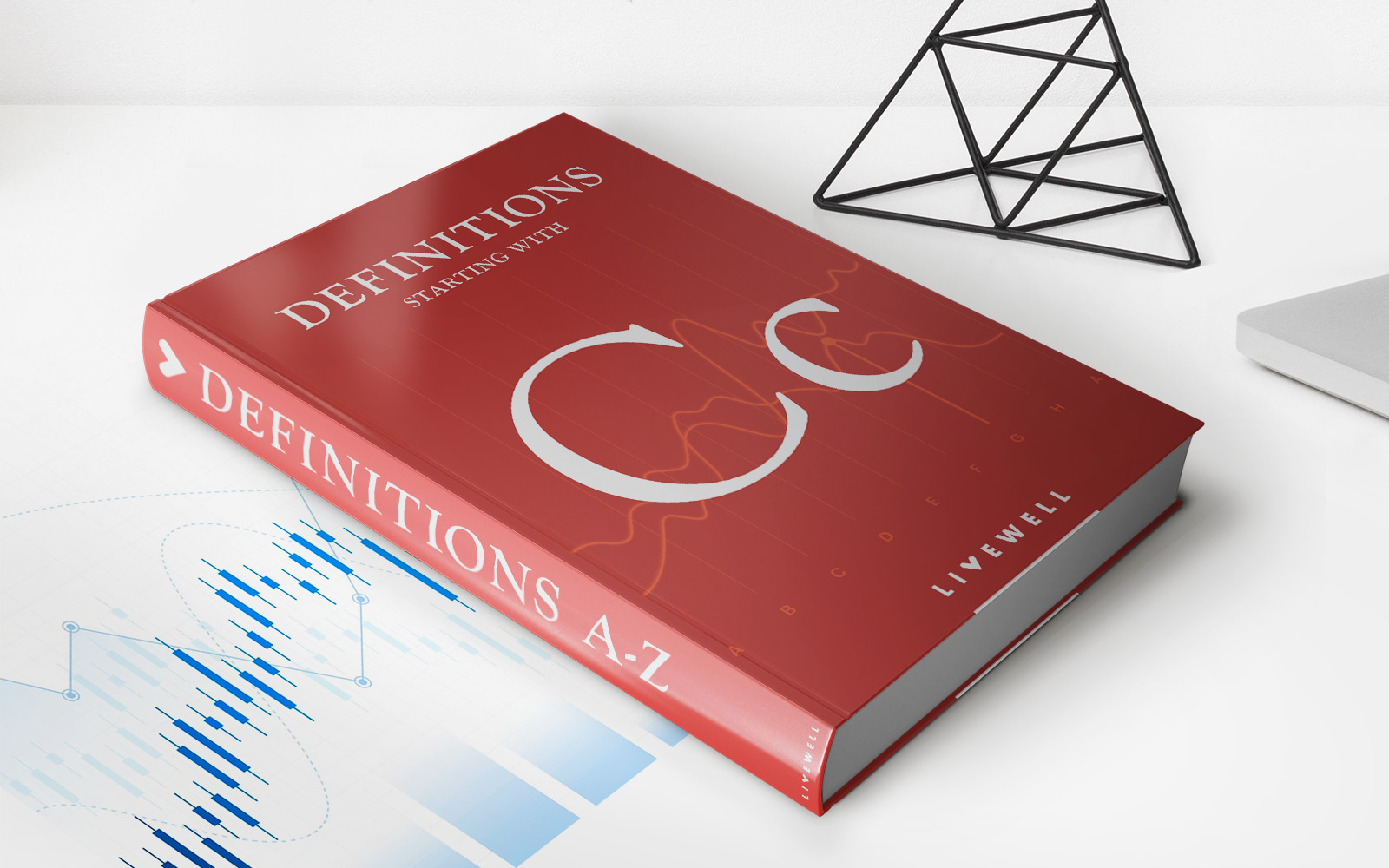
By: • Finance
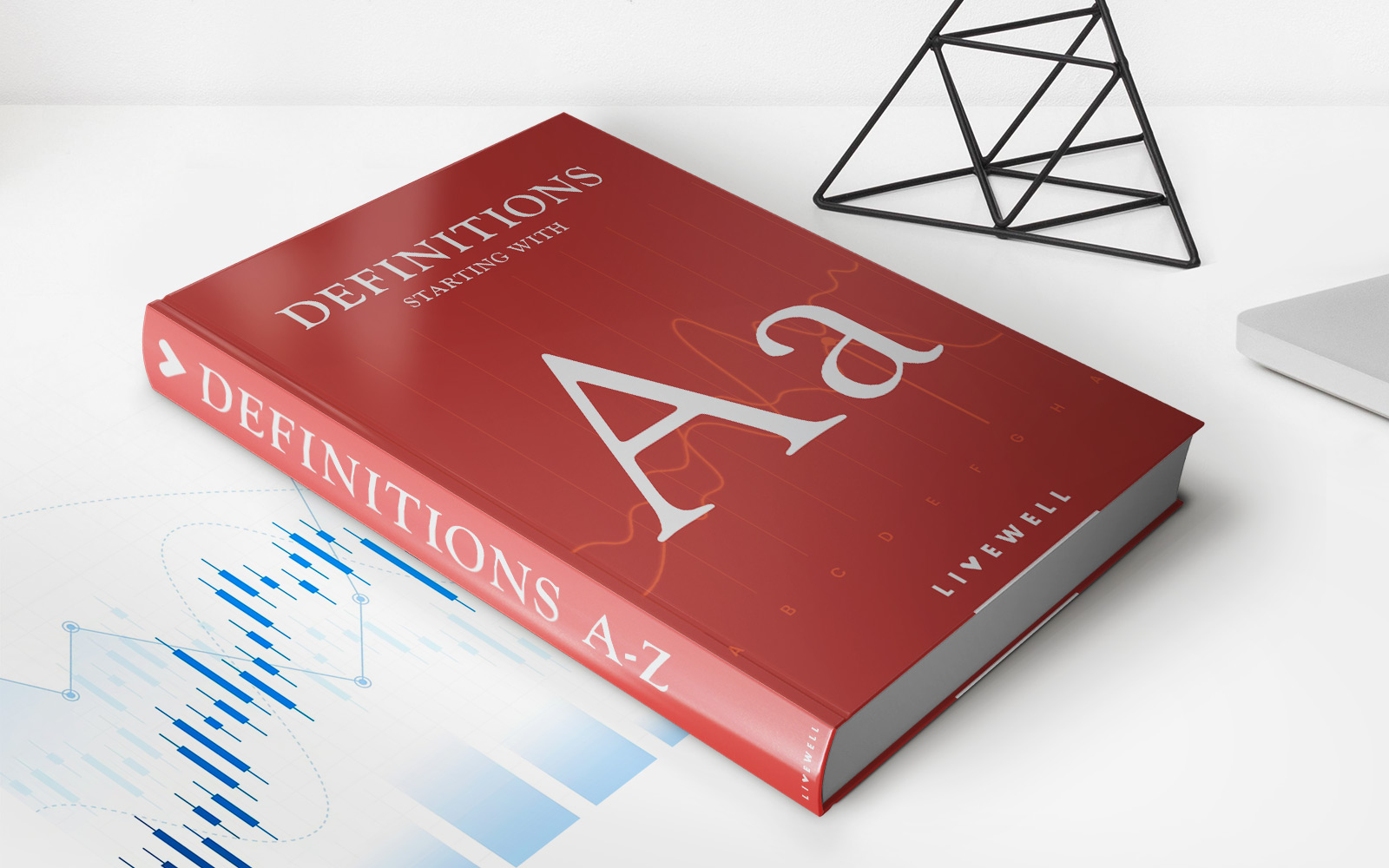
Please accept our Privacy Policy.
We uses cookies to improve your experience and to show you personalized ads. Please review our privacy policy by clicking here .
- https://livewell.com/finance/what-are-transaction-costs-definition-how-they-work-and-example/
Understanding ETFs
ETF premiums and discounts, explained
August 07, 2023
Various expenses make up the total cost of ownership of an exchange-traded fund (ETF), including its expense ratio and bid-ask spread . Here, we examine a third element of the total cost of ETF ownership—premiums and discounts, which occur when an ETF’s market price differs from the net asset value of its underlying securities.
We dispel some myths about ETF premiums and discounts, explain why they can occur, and suggest how investors can make informed decisions. We also look at how premiums and discounts differ by asset class.
How exchange-traded funds are valued
The value of an ETF is determined in a number of ways, each of which can affect an investor’s experience. First, there’s the net asset value (NAV). As with mutual funds, NAV is the assessed value of all of an ETF’s underlying securities. 1
In other words, a fund’s NAV is its fair value. But unlike with mutual funds, ETF investors don’t transact at NAV. 2 Instead, ETF prices are determined by the market.
An ETF’s market price is the most important price for investors—the one at which they buy and sell shares in the secondary market. Since market prices are ruled by supply and demand, an ETF’s market price can diverge from its NAV.
If there’s heavy demand from buyers, the price of an ETF can increase above its NAV (a premium). Conversely, if there’s heavy sell-side pressure, the price can dip below the NAV (a discount).
The ETF creation-redemption mechanism
The beauty of the ETF structure is the “creation-redemption” mechanism, which allows new ETF shares to be created to meet demand or redeemed to reduce supply as needed. Creations and redemptions help keep an ETF’s market price in line with its fair value and limits severe premiums and discounts. As long as the mechanism is running smoothly, ETF prices should stay near fair values.
Focus on what's most important
The biggest risk related to ETF premiums and discounts is realized when an investor purchases an ETF when it’s trading at a substantial premium and then sells it at a substantial discount. This is why it’s important to focus on the volatility of a fund’s premium or discount.
Many investors focus on an ETF’s average premium, but the stability of premiums and discounts over time will have a much more significant impact.
The following charts help illustrate this point:
Source: Morningstar, based on data from July 2018 through November 2019.
ETF A has an average premium of 18 basis points (0.18 percentage point), and ETF B has an average premium of 0 basis points. An investor looking at those averages might assume ETF B is a better product.
This would be incorrect.
Although ETF A does have a larger average premium, the premium is more stable, which reduces the investor’s risk and potential costs. If investors buy and sell at a stable premium, the cost is likely to be predictable and relatively modest. As the graph illustrates, an investor might buy ETF A at a premium of 22 basis points and sell at a premium of 18 basis points, incurring only 4 basis points of round-trip transactions costs. While it may seem a disadvantage to buy an ETF at a premium of 22 basis points, selling at a similar premium can offset this initial cost.
Conversely, ETF B has a smaller but more volatile premium, which exposes investors to the possibility of buying at a significant premium and selling at a significant discount. An investor who purchased at a premium of 64 basis points and sold at a discount of 61 basis points would incur 125 basis points (1.25 percentage points) of round-trip transaction costs. Selling at a steep discount only adds to the initial transaction cost.
Again, a larger but stable premium is often preferable to a lesser, volatile one. The standard deviation of historical premiums is arguably a clearer way to estimate transaction costs than the average premium.
It’s also worth noting that any ETF premium or discount published at the end of a trading day generally represents only the closing snapshot, comparing the ETF’s NAV to its closing price on its primary exchange. A premium or discount at the close may not accurately depict where the ETF traded relative to its NAV during the trading day.
Differences in ETF premiums and discounts across asset classes
Domestic etfs.
Domestic equity ETFs generally trade in line with their NAVs. Premiums and discounts are typically slight for a variety of reasons, including the lower cost of buying and selling the underlying domestic equities, the relative ease of pricing underlying stocks and ETFs simultaneously, and the lower fixed fees incurred.
In other words, pricing domestic ETFs is relatively straightforward and can frequently be achieved without too much deviation from NAV.
International ETFs
International ETFs may have more pronounced premiums and discounts. It’s more challenging to determine the fair value of the underlying constituents, partly because the markets where they’re listed may not be open during hours when U.S.-listed ETFs are trading.
Other costs may include larger fixed fees, higher commissions, stamp taxes and associated fees, foreign exchange hedging costs, and the imprecise nature of fair value factors. This makes the process to create or redeem international ETFs less precise, which translates to larger premiums and discounts.
Fixed income ETFs
Fixed Income ETFs usually trade at inherent premiums. Their NAVs are based on the bid prices of all their underlying securities, or the prices at which the funds could sell all of their holdings.
A fixed income ETF’s market price will typically be near the midpoint of all the underlying bonds in the ETF’s basket. This represents what it would cost market makers to buy the underlying bonds to create new ETF shares, though sometimes an ETF can trade up toward the cost to create new shares.
Key takeaways
Premiums and discounts are important components of the total cost of ETF ownership, and they vary by asset class. Their stability can be considerably more meaningful than their size . A larger, but stable, premium is often preferable to a lesser, volatile one.
While the existence of premiums and discounts should not lead investors to avoid ETFs, extremely volatile premiums and discounts can erode longer-term returns significantly. Investors can steer clear of relatively elevated ETF premiums and discounts by avoiding trading on days when markets are roiled. In any case, they should understand good ETF trading practices .
1 Certain funds may apply a fair value pricing methodology that will set NAV at different levels than the underlying securities’ official closing value.
2 ETF investors can make NAV trades; however, these trades typically need to be placed by a high-touch trade desk and are often executed with a markup or markdown to the stated NAV depending on the asset class and trade direction.
Related links:
- Case studies in the cost of ETF ownership (article, issued July 2023)
- On its 30th birthday, the ETF looms large (article, issued February 2023)
- Trading tips for ETF investors grappling with market volatility (article, issued November 2022)
All investing is subject to risk, including the possible loss of the money you invest.
Bond funds are subject to the risk that an issuer will fail to make payments on time, and that bond prices will decline because of rising interest rates or negative perceptions of an issuer's ability to make payments.
Investments in stocks or bonds issued by non-U.S. companies are subject to risks including country/regional risk and currency risk. These risks are especially high in emerging markets.
Contributors
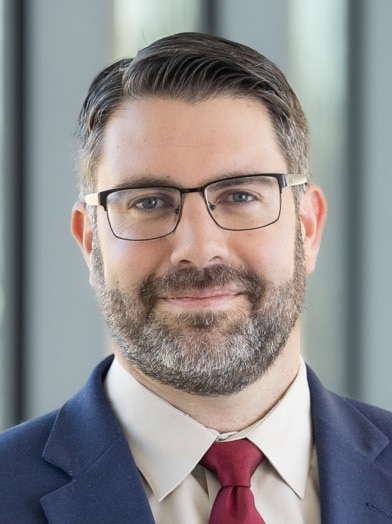
David Sharp
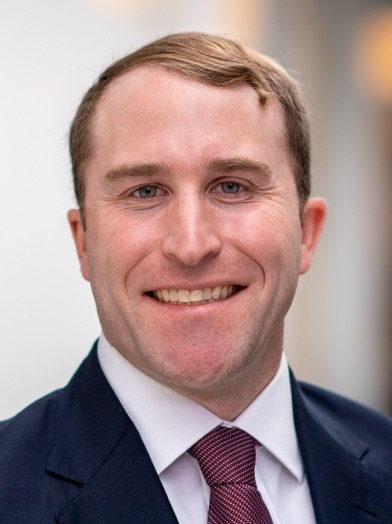
Patrick Hooper
Vanguard Information and Insights
Subscribe to portfolio considerations., get vanguard news, insights, and timely analysis on the market, delivered straight to your inbox..
Read our privacy policy to learn about how we keep personal information private. * Indicates a required field

Thank you for subscribing to Portfolio considerations.
You'll be notified when new content is published, but will only ever receive one email a day from vanguard insights., you’re already a subscriber, thank you what would you like to do next.
Vanguard is the trusted name in investing. Since our founding in 1975, we've put investors first.
Speculative trading, prospect theory and transaction costs
- Open access
- Published: 15 December 2022
- Volume 27 , pages 49–96, ( 2023 )
Cite this article
You have full access to this open access article
- Alex S. L. Tse 1 &
- Harry Zheng 2
2256 Accesses
1 Altmetric
Explore all metrics
A speculative agent with prospect theory preference chooses the optimal time to purchase and then to sell an indivisible risky asset to maximise the expected utility of the round-trip profit net of transaction costs. The optimisation problem is formulated as a sequential optimal stopping problem, and we provide a complete characterisation of the solution. Depending on the preference and market parameters, the optimal strategy can be “buy and hold”, “buy low, sell high”, “buy high, sell higher” or “no trading”. Behavioural preference and market friction interact in a subtle way which yields surprising implications on the agent’s trading patterns. For example, increasing the market entry fee does not necessarily curb speculative trading, but instead may induce a higher reference point under which the agent becomes more risk-seeking and in turn is more likely to trade.
Similar content being viewed by others
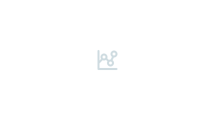
Fundamental theorem of asset pricing with acceptable risk in markets with frictions
Maria Arduca & Cosimo Munari
Semimartingale price systems in models with transaction costs beyond efficient friction
Christoph Kühn & Alexander Molitor
Creation and Control of Bubbles: Managers Compensation Schemes, Risk Aversion, and Wealth and Short Sale Constraints
Avoid common mistakes on your manuscript.
1 Introduction
When it comes to modelling trading behaviour, the standard economic paradigm is the maximisation of risk-averse agents’ expected utility in a frictionless market. This criterion, however, has been criticised on many levels. In terms of trading environment, financial friction is omnipresent in reality where transactions are subject to various costs. In terms of agents’ preferences, the behavioural economics literature suggests that many individuals do not make decisions in accordance to expected utility theory. First, utilities are not necessarily derived from final wealth, but typically what matters is the change in wealth relative to some reference point. Second, individuals are usually risk-averse over the domain of gains, but risk-seeking over the domain of losses—this can be captured by an S-shaped utility function. Finally, individuals may fail to take portfolio effects into account when making investment decisions, and this phenomenon is known as narrow framing. These psychological ideas are explored for example in the seminal work of Kahneman and Tversky [ 16 ], Tversky and Kahneman [ 26 , 27 ] and Kahneman and Lovallo [ 15 ].
In this paper, we develop a tractable dynamic trading model which captures a number of stylised behavioural biases of individuals as well as market frictions. In our setup, trading is costly due to proportional transaction costs as well as a fixed market entry fee. The goal of an agent is to find the optimal time to buy and then to sell an indivisible risky asset to maximise the expected utility of the round-trip profit under the prospect theory preference of Tversky and Kahneman [ 27 ]. While a realistic economy can consist of multiple assets, we can interpret the assumption of a single indivisible asset as a manifestation of narrow framing such that the trading decisions associated with one particular unit of indivisible asset can be completely isolated from the other investment opportunities. We believe the model is the best suitable to describe the trading behaviour of speculative agents. These “less-than-fully rational” agents purchase and sell an asset with a narrow objective of making a one-off round-trip profit rather than supporting consumption or stipulating a long-term portfolio growth.
A sequential optimal stopping problem featuring an S-shaped utility function is solved to identify the entry and exit time of the market by the agent. The solution approach is based on a backward induction idea. In the first stage, we focus on the exit strategy of the agent: Conditionally on the ownership of the asset purchased at a given price level (which determines the agent’s reference point), the optimal liquidation problem is solved. Then the value function of this exit problem reflects the utility value of purchasing the asset at a different price level. Upon comparison against the utility value of inaction, we obtain the payoff function of the real option to purchase the asset which is then used in the second stage problem concerning the entry decision of the agent: The agent picks the optimal time to enter the trade so as to maximise the expected payoff of this real option to purchase the asset.
The traditional route to analyse an optimal stopping problem is to first conjecture a candidate optimal stopping rule, and then the dynamic programming principle is invoked to derive a free boundary value problem that the value function should satisfy. Then one can attempt to solve for the free boundaries via value matching and smooth pasting. For this approach to work, we need to correctly identify the form of the optimal stopping rule, but this exercise may be not trivial. As it turns out, the optimal continuation region of our entry problem can either be connected or disconnected, depending on the model parameters. It is thus difficult to adopt such a guess-and-verify approach since we do not know the correct form of the optimal stopping rule upfront. In our analysis, a martingale method is employed to solve the underlying optimal stopping problems, which has the important advantage that no a priori conjecture on the optimal strategy is required. The optimal continuation/stopping set can be deduced directly by studying the smallest concave majorant of a suitably scaled payoff function.
Despite its relatively simple nature, our model is capable of generating a rich variety of trading behaviours such as “buy and hold”, “buy low, sell high”, “buy high, sell higher” and “no trading”. The risk-seeking preference of a behavioural agent over the loss domain will typically encourage him to enter the trade, but his precise trading behaviour depends crucially on the level of transaction costs relative to his preference parameters. Generally speaking, a high proportional (fixed) transaction cost discourages trading at a high (low) nominal price. When proportional costs are high and the asset is expensive, the agent prefers waiting until the price level declines, and hence he is more inclined to consider a “buy low, sell high” strategy. But if instead the fixed entry fee is high and the asset is cheap, the agent might prefer delaying the purchase decision until the asset reaches a higher price level, and this leads to a trading pattern of “buy high, sell higher”.
Both behavioural preferences and market frictions are studied extensively as separate topics in the mathematical finance literature. To the best of our knowledge, however, their interaction has not been explored to date. Under prospect theory, the risk attitude of the agent is heavily influenced by the reference point. In our model, the reference point is endogenised so that it depends on the cost of purchase including the transaction cost paid. The level of transaction cost therefore has a direct impact on the agent’s risk preference. This subtle interaction between risk preference and transaction cost leads to interesting policy implications on how speculative trading can be curbed effectively. For example, a surprising result is that imposing a fixed market entry fee might indeed accelerate rather than cool down trading participation.
Our paper is closely related to the literature on optimal stopping under an S-shaped utility function. Kyle et al. [ 18 ] and Henderson [ 9 ] consider a one-off optimal liquidation problem in which the agent solves for the optimal time to liquidate an endowed risky asset to maximise an expected prospect theory utility. They do not consider the purchase decision, and the reference point is taken as some exogenously given status quo. A main contribution of our paper is that we further endogenise the reference point which depends on the purchase price of the asset, and the optimal purchase price must be determined as a part of the optimisation problem. The recent work of Henderson and Muscat [ 11 ] extends the model of Henderson [ 9 ] by considering partial liquidation of multiple indivisible assets. Both the present paper and [ 11 ] consider a sequential optimal stopping problem as the underlying mathematical framework. However, the economic natures of the problems are completely different—we study the sequential decision of purchase and sale, while they exclusively focus on sales.
Another relevant class of works is the realisation utility model which further incorporates a reinvestment possibility within a behavioural optimal stopping model, such as Barberis and Xiong [ 2 ], Ingersoll and Jin [ 14 ], He and Yang [ 8 ], Kong et al. [ 17 ] and Dai et al. [ 3 ]. In such models, the agent repeatedly purchases and sells an asset to maximise the sum of utility bursts realised from the gain and loss associated with each round-trip transaction. In a certain sense, these models consider an endogenised reference point which is continuously updated based on the historical prices within each trading episode. However, the purchase decision is exogenously given in many of the models where the agent is simply assumed to buy the asset again immediately after a sale. These cited papers on realisation utility models all feature transaction costs which are required to make the problems well posed. As a result, the purchase pattern is not entirely realistic: If the agent is willing to sell an asset and then instantaneously repurchase an identical (or a different, but statistically identical) asset, then the agent is essentially throwing away money in the form of transaction costs without altering his own financial position. Only He and Yang [ 8 ] carefully analyse the purchase decision of the agent, but in any case they find that the purchase strategy is trivial—the agent either buys the asset immediately after a sale or never enters the trade again. Our model differs from the realisation utility model in that we do not consider perpetual reinvestment opportunities (which can be understood as narrow framing that the agent only focuses on a single episode of the trading experience when evaluating the entry and exit strategies). Nonetheless, the optimal purchase region of our model is non-trivial under typical parameters and encapsulates many realistic trading strategies.
Beyond the context of behavioural economics, there are a few works attempting to model the sequential purchase and sale decisions under an optimal switching framework. However, identification of a modelling setup which can generate reasonable trading patterns proves to be much more difficult than expected. On the one hand, Zervos et al. [ 29 ] report that “… the prime example of an asset price process, namely, the geometric Brownian motion, does not allow optimal buying and selling strategies that have a sequential nature”. Indeed, existing literature which gives “buy low, sell high” as an optimal trading strategy often relies on extra statistical features of the asset price process such as mean reversion. See for example Zhang and Zhang [ 30 ], Song et al. [ 25 ], Leung et al. [ 20 ] and Leung and Li [ 19 ]. On the other hand, momentum-based trading strategies are also rarely studied in the mathematical finance literature. The scarce examples include the work of Dai et al. [ 5 ] and Dai et al. [ 4 ] who find that a trend-following strategy is optimal under a regime-switching model for the asset price. We contribute to this strand of literature by showing that a trading model based on a simple geometric Brownian motion can also generate many realistic trading patterns including both a reversal strategy (buy low, sell high) and a momentum strategy (buy high, sell higher). This is achieved via incorporating standard elements of behavioural preferences and market frictions.
The optimal investment rule in the classical Merton [ 23 , 24 ] portfolio selection problem can also be viewed as a buy low, sell high strategy: Since the agent keeps a constant fraction of wealth invested in the risky asset, extra units of risky asset are sold (purchased) when the price increases (falls), ceteris paribus. In our paper, we focus on a single indivisible asset and do not consider portfolio effects.
The rest of the paper is organised as follows. Section 2 provides a description of the model and the underlying optimisation problem. In Sect. 3 , we outline the solution methods to a standard optimal stopping problem and discuss heuristically how the solution to our sequential optimal stopping problem can be characterised via the idea of backward induction. The main results are collected in Sect. 4 . Some comparative statics results and their policy implications are discussed in Sect. 5 . Several extensions of the baseline model are discussed in Sect. 6 . Section 7 concludes. A few technical proofs are deferred to the Appendix .
2 Problem description
Let \((\Omega , \mathcal{F}, (\mathcal{F}_{t}),\mathbb{P})\) be a filtered probability space satisfying the usual conditions and supporting a one-dimensional Brownian motion \(B=(B_{t})_{t\geq 0}\) . There is a single indivisible risky asset in the economy. Its price process \(P=(P_{t})_{t\geq 0}\) is modelled by a one-dimensional diffusion with state space \(\mathcal{J}\subseteq \mathbb{R}_{+}\) and dynamics of
where \(\mu :\mathcal{J}\to \mathbb{R}\) and \(\sigma :\mathcal{J}\to (0,\infty )\) are Borel functions. We assume that \(\mathcal{J}\) is an interval with endpoints \(0\leq a_{\mathcal{J}}< b_{\mathcal{J}}\leq \infty \) and that \(P\) is regular in \((a_{\mathcal{J}},b_{\mathcal{J}})\) , i.e., for any \(p,y\in (a_{\mathcal{J}},b_{\mathcal{J}})\) , we have \(\mathbb{P}[\tau _{y}<\infty |P_{0}=p]>0\) , where \(\tau _{y}:= \inf \{t\geq 0: P_{t}=y\}\) .
We assume that the interest rate is zero in our exposition. For the non-zero interest rate case, one can interpret the process \(P\) as the numéraire-adjusted price of the asset. Then the drift term \(\mu (\,\cdot \,)\) can be viewed as the instantaneous excess return of the risky asset.
Trading in the asset is costly. If the agent wants to purchase the asset at its current price \(p\) , he will need to pay \(\lambda p + \Psi \) to initiate the trade, where \(\lambda \in [1,\infty )\) so that \(\lambda - 1\) represents the proportional transaction cost on purchases and \(\Psi \geq 0\) represents a fixed market entry fee. When the agent sells the asset at price \(p\) , he will only receive \(\gamma p\) , where \(\gamma \in (0,1]\) so that \(1-\gamma \) represents the proportional transaction cost on sales.
The preference of the agent is described by the prospect theory of Tversky and Kahneman [ 27 ]. Under this framework, utility is derived from gains and losses relative to some reference point rather than from the total wealth. Individuals are typically risk-averse over the domain of gains and risk-seeking over the domain of losses. This can be captured by an S-shaped utility function \(U:\mathbb{R}\to \mathbb{R}\) with \(U(0)=0\) and such that \(U\) is concave (resp. convex) over \(\mathbb{R}_{+}\) (resp. \(\mathbb{R}_{-}\) ). Finally, individuals also exhibit loss-aversion so that the negative utility increment brought by a unit of loss is much larger in magnitude than the positive utility increment from a unit of gain.
In the behavioural optimal liquidation literature such as Kyle et al. [ 18 ] and Henderson [ 9 ], the liquidation payoff is always compared against some exogenously given constant reference point. In our setup, we assume the reference point depends on both an exogenous constant \(R\) as well as on the amount paid by the agent to purchase the asset. Suppose the agent has executed a speculative round-trip trade where he has bought and then sold the asset at stopping times \(\tau \) and \(\nu \) (with \(\tau \leq \nu \) ), respectively. The liquidation payoff \(\gamma P_{\nu}\) is evaluated against \(\lambda P_{\tau}+\Psi +R\) as the reference point, where \(\lambda P_{\tau}+\Psi \) is the capital spent on purchasing the asset and \(R\) is a constant outside the model specification. The parameter \(R\) can be interpreted as a preference parameter of the agent which reflects his “aspiration level” in the sense of Lopes and Oden [ 21 ], where a more motivated agent will set a higher economic benchmark as a profit target to beat. The realised utility of this round-trip trade is \(U(\gamma P_{\nu}-\lambda P_{\tau}-\Psi -R)\) .
A caveat, however, is that the agent is not obliged to enter or exit the trade at all if it is undesirable to do so. A realisation of \(\tau =\infty \) refers to the case that the purchase decision is deferred indefinitely, which is economically equivalent to not entering the trade at all. The liquidation value is zero because there is nothing to be sold, and the reference point becomes \(R\) since the required cash outflow \(\lambda P_{\tau}+\Psi \) for the purchase has never materialised. Thus the prospect theory value under this strategy is simply \(U(-R)\) . Similarly, the agent may enter the trade at some time point but never liquidate the asset. This corresponds to a realisation of \(\tau <\infty \) and \(\nu =\infty \) . In this case, the liquidation value is again zero and evaluated against the reference point \(\lambda P_{\tau}+\Psi +R\) . To summarise all the possibilities, the realised prospect theory utility associated with a trading strategy \((\tau ,\nu )\) is written as
The objective of the agent is to find the optimal purchase time \(\tau \) and sale time \(\nu \) to maximise the expected value of ( 2.1 ). Define the objective function as
Formally, the agent is solving the sequential optimal stopping problem
where \(\mathcal{T}\) is the set of \((\mathcal{F}_{t})\) -stopping times valued in \(\mathbb{R}_{+}\cup \{+\infty \}\) . Problem ( 2.3 ) has two features which make it non-standard relative to a typical optimal stopping problem. First, the decision space is two-dimensional. Second, the objective function has an explicit dependence on the stopping times \(\tau ,\nu \) via the indicator functions, which further complicates the analysis.
Similarly to Henderson [ 9 ], Xu and Zhou [ 28 ] and Henderson et al. [ 10 ], we do not explicitly consider subjective discounting in our baseline model. On the one hand, our model features cash flows at different time points and it is not entirely clear what is the most appropriate way to apply subjective discounting, because the standard prospect theory framework is not directly applicable to intertemporal choices. On the other hand, under discounting, an impatient agent is much more inclined to delay losses and to realise profits earlier, which will lead to an extreme disposition effect which is not consistent with the empirical trading pattern of retail investors; see the discussion in Henderson [ 9 ]. At a mathematical level, introducing subjective discounting also makes our problem harder to be analysed in full generality. We briefly discuss in Sect. 6.2 how subjective discounting might be incorporated and explore (numerically in some cases) how it affects the optimal trading behaviour.
3 The solution methods
In this section, we give an overview of how problem ( 2.3 ) can be solved. We begin by offering a short summary about the solution approach to solve a standard optimal stopping problem for a one-dimensional diffusion.
3.1 The martingale method for optimal stopping problems
We review the martingale method to solve an undiscounted optimal stopping problem; this is based on Dayanik and Karatzas [ 6 ].
Consider a general problem in the form of
for some payoff function \(G\) . In the standard theory of optimal stopping, the optimal stopping time can be characterised as the first exit time of the process from some open set \(\mathcal{C}\) , so that \(\tau =\inf \{t\geq 0: P_{t}\notin C\}\) . In a one-dimensional diffusion setting, it is sufficient to consider stopping times which have the form \(\tau _{a,b}:= \tau _{a} \wedge \tau _{b}\) , where \(\tau _{a}:= \inf \{t\geq 0: P_{t}=a\}\) and \(\tau _{b}:= \inf \{t\geq 0: P_{t}=b\}\) with \(a\leq p\leq b\) . Here \([a,b]\subseteq \mathcal{J}\) is an unknown interval to be identified (and it depends on \(p\) in general).
Let \(s(\,\cdot \,)\) be the scale function of the process \(P\) (it is unique up to an affine transformation), defined as a strictly increasing function such that \(\Theta :=s(P)\) is a local martingale. A simple application of Itô’s lemma shows that \(s(\,\cdot \,)\) should solve the second order differential equation
Let \(\theta :=s(p)\) . Then
where \(\phi :=G\circ s^{-1}\) . The above can be maximised with respect to \(a\) and \(b\) . Moreover, the dummy variables \(a\) and \(b\) can be replaced by \(a'=s(a)\) and \(b'=s(b)\) . Hence
and thus \(V(p)=v(s(p))\) . The scaled value function \(v(\theta )\) can be characterised as the smallest concave majorant of \(\phi (\theta )=G(s^{-1}(\theta ))\) , the scaled payoff function over \(s(\mathcal{J})\) , which is defined as an interval with endpoints \(s(a_{\mathcal{J}})\) and \(s(b_{\mathcal{J}})\) . The continuation and stopping set associated with the optimal stopping rule are given by \(\mathcal{C}=\{p\in \mathcal{J}:v(s(p))>\phi (s(p))\}\) and \(\mathcal{S}=\{p\in \mathcal{J}:v(s(p))=\phi (s(p))\}\) , respectively.
3.2 Decomposition of the sequential optimal stopping problem
In the following two subsections, we discuss heuristically how the value function of ( 2.3 ) can be constructed by considering two sub-problems based on the idea of backward induction. The wellposedness conditions as well as a formal verification of optimality are explored in Sect. 4 when we specialise the modelling setup.
3.2.1 Exit problem
Suppose for the moment that the agent has already purchased the asset at some known price level \(q\) via paying \(\lambda q+\Psi \) at some time in the past. Conditionally on this information, the reference point has been fixed at the known constant level \(H:=\lambda q + \Psi +R\) . Suppose the current time is labelled as \(t=0\) and the current price of the asset is \(P_{0}=p\) . The goal of the agent in the exit problem is to find the optimal time to sell this owned asset to maximise the expected prospect theory value of the sale proceeds relative to the reference point \(H\) . If the asset is (ever) sold at time \(\nu \) , the utility of gain and loss relative to the reference point is U ( γ P ν 1 { ν < ∞ } − H ) after taking the transaction cost on a sale into account. Since the realised utility is increasing in \(P_{\nu}\) and the process \(P\) is nonnegative, there is in general no incentive for the agent to forgo the sale opportunity. Hence heuristically, one can drop the indicator function 1 { ν < ∞ } and it is sufficient to consider the objective function \(G_{1}(P_{\nu};H):=U(\gamma P_{\nu}-H)\) . The agent then solves the optimal stopping problem
to determine the optimal time of the asset sale. The value function of the exit problem is then given by \(V_{1}(p; H)=\bar{g}_{1}(s(p); H)\) , where \(\bar{g}_{1}=\bar{g}_{1}(\theta ;H)\) is the smallest concave majorant of
We write the optimiser to problem ( 3.2 ) as \(\nu ^{*}(p;H)\) ; it depends on the initial price level \(p\) and the given reference point \(H\) .
3.2.2 Entry problem
Now we assume the agent does not own any asset to begin with. His objective is to determine the optimal time to purchase (and then to sell) the asset to maximise the expected utility of the liquidation proceeds relative to the endogenised reference point.
At a given current asset price level \(p\) , there are two possible actions for the agent. First, he can opt to initiate the speculative trade by buying the asset now, which fixes the reference point as \(\lambda p+\Psi +R\) , and then sell it later in the future. When the asset is liquidated at his choice of the sale time \(\nu \) , the realised utility is \(U(\gamma P_{\nu}-\lambda p-\Psi -R)\) . Conditionally on the decision to purchase the asset today at price \(p\) , the agent can find the best time of sale to maximise his expected utility by solving problem ( 3.2 ) for \(H=\lambda p + \Psi +R\) . Then the best possible expected utility he can attain is
provided that he decides to enter the trade at the given price of \(p\) .
Alternatively, the agent can forgo the opportunity to enter the trade and stay away from the market forever. In this case, the payoff is zero and the reference point is simply equal to \(R\) . The utility he will receive is just the constant \(U(-R)\) .
Therefore, the opportunity to enter the speculative trade can be viewed as a real option. At a given price level \(p\) , the agent is willing to enter the trade only if the maximal expected utility of trading is not less than that of inaction, i.e., if
This is similar to a financial option being in the money. The payoff of this real option to the agent in utility terms as a function of the price level \(p\) is given by
The entry problem for the agent is to find the optimal time to initiate the trade so as to maximise the expected value of ( 3.3 ). This is equivalent to solving
if the exit problem value function \(V_{1}\) is well defined. We identify \(\bar{g}_{2}=\bar{g}_{2}(\theta )\) as the smallest concave majorant of
Then the value function of the entry problem is \(V_{2}(p)=\bar{g}_{2}(s(p))\) .
Let the optimiser to ( 3.4 ) be \(\tau ^{*}(p)\) . With \(p\) being the initial price of the asset at \(t= 0\) , the agent will purchase the asset at the time \(t=\tau ^{*}(p)\) . Then conditionally on the realisation of the entry price level \(P_{\tau ^{*}(p)}\) , the agent solves the exit problem ( 3.2 ) with initial value \(P_{\tau ^{*}(p)}\) and reference point \(H=\lambda P_{\tau ^{*}(p)}+ \Psi +R\) . The corresponding optimiser is given by \(\nu ^{*}(P_{\tau ^{*}(p)};\lambda P_{\tau ^{*}(p)}+ \Psi +R)\) and reflects the time lapse between the initiation and closure of the trade. In particular, the agent will sell the asset at the time \(t=\tau ^{*}(p)+\nu ^{*}(P_{\tau ^{*}(p)};\lambda P_{\tau ^{*}(p)}+ \Psi +R)\) . This gives a complete characterisation of the optimal entry and exit strategy of the agent. Note that it is possible to have \(\mathbb{P}[\tau ^{*}(p)<\infty ]<1\) . In other words, there is a possibility that the entry strategy is not executed in finite time, and hence there is no decision to sell (see the discussion in Sect. 4 ).
Intuitively, we expect \(\mathcal{V}(p)=V_{2}(p)\) , where \(\mathcal{V}\) is the value function of the original sequential optimal stopping problem ( 2.3 ). This must be verified formally. Without any further specifications of the utility function \(U\) and the underlying price process \(P\) , however, it is hard to make further progress. For example, it is not even clear upfront whether ( 2.3 ) is a well-posed problem with a finite value function.
4 Main results
The procedures described in Sect. 3 are very generic and can guide us to write down a candidate for the value function of the sequential optimal stopping problem under a range of model specifications. To derive stronger analytical results, we specialise in the rest of this paper to the piecewise power utility function of Tversky and Kahneman [ 27 ] in the form
Here \(\alpha \in (0,1)\) so that \(1-\alpha \) is the level of risk-aversion and risk-seeking on the domains of gains and losses, and \(k>1\) controls the degree of loss-aversion. Experimental results in [ 27 ] give estimates of \(\alpha =0.88\) and \(k=2.25\) .
The price process of the risky asset \(P=(P_{t})_{t\geq 0}\) is assumed to be a geometric Brownian motion,
with \(\mu \geq 0\) and \(\sigma >0\) being the constant drift and volatility of the asset. Define \(\beta :=1-\frac{2\mu}{\sigma ^{2}}\leq 1\) . Then by substituting \(\mu (p)=\mu p\) and \(\sigma (p)=\sigma p\) in ( 3.1 ), the scale function of \(P\) can be found as
Finally, we assume \(R>0\) so that the aspiration level of the agent is always positive. This is not unreasonable since this parameter can be understood as some performance benchmark that an agent wants to outperform, and such a goal is typically positive.
We begin with a necessary condition under which ( 2.3 ) is well posed.
If \(\beta \leq 0\) or \(0<\beta <\alpha \) , then there exists a sequence of stopping times \((\tau _{n},\nu _{n})_{n=1,2,\dots }\) such that \(J(p;\tau _{n},\nu _{n})\to +\infty \) as \(n \to \infty \) , where \(J(p;\,\cdot \,,\,\cdot \,)\) is defined in ( 2.2 ).
Consider the sequence of stopping times defined by
If \(\beta \leq 0\) , then
so that the Brownian motion in the exponent has nonnegative drift. Hence \(P\) can reach any positive level in finite time, and so \(\nu _{n}<\infty \) and \(P_{\nu _{n}}=n\) , almost surely. Hence
as \(n\to \infty \) .
If \(\beta >0\) , then instead \(P\) cannot reach any arbitrary level above its starting value in finite time and we have \(\lim _{t\to \infty}P_{t}=0\) almost surely. Then \(\{v_{n}< \infty \}=\{P_{\nu _{n}}=n\}\) , and for sufficiently large \(n>p\) , one can compute that
as \(n\to \infty \) if \(\beta <\alpha \) . □
Mathematically speaking, the sequential optimal stopping problem ( 2.3 ) is ill posed under the parameter combination in Lemma 4.1 , and its value function diverges to infinity. This arises when the performance of the asset is too good relative to the agent’s risk-aversion level over gains. Equation ( 4.1 ) corresponds to a “buy and hold” trading rule as a possible optimal strategy: the agent purchases the asset immediately from the outset, and the profit-target level of sale can be set arbitrarily high.
Empirically, historical returns on equities are excessively high relative to their risk level. For example, the annualised mean and standard deviation of the equity premium (i.e., excess return above the risk-free rate) of the U.S. market over the time period 1889–1978 are 6.18% and 16.67%, respectively (see Mehra and Prescott [ 22 ]), so that \(\beta =1-2\mu /\sigma \approx -3.45\) , while the estimates based on the more recent time period 1950–2015 are 7.15% and 16.83% (see Bai and Lu [ 1 ]) so that \(\beta \approx -4.05\) . Although this may cast doubt on the practical relevance of the condition \(0<\alpha \leq \beta \) (where buy and hold is an optimal strategy if the condition does not hold), we should like to emphasise that \(\beta \) in general is a noisy statistical quantity which is hard to forecast. In our model, \(\beta \) should be interpreted as the agent’s subjective assessment of the asset performance which can be much more conservative than the historical estimates. The parameter \(\mu \) may also encapsulate subjective discounting which further lowers its value; see Sect. 6.2 .
From here onwards , we focus on the case \(0<\alpha \leq \beta \) which is a necessary condition for ( 2.3 ) to be well posed. The form of the solutions to the exit problem ( 3.2 ) and entry problem ( 3.4 ) are first provided, and then we discuss the economic intuition behind the associated trading strategies. Towards the end of this section, the optimality of the value function of the entry problem is formally verified to show that it indeed corresponds to the solution of the sequential optimal stopping problem ( 2.3 ).
We first state the solution to the exit problem ( 3.2 ); a similar result can be found in Henderson [ 9 ].
For the exit problem ( 3.2 ), if \(0<\alpha \leq \beta \) , the agent will sell the asset when its price level first reaches \(\frac{cH}{\gamma}\) or above , where \(c>1\) is a constant given by the solution to the equation
The value function is given by
Recall the notation introduced in Sect. 3.2.1 . For \(\beta >0\) , the scaled payoff function of the exit problem is given by
It is straightforward to work out the derivatives of \(g_{1}\) as
Given the standing assumption \(\beta \leq 1\) and the condition \(0<\alpha \leq \beta \) , \(g_{1}\) is increasing concave for \(\theta >(\frac{H}{\gamma})^{\beta}\) and increasing convex for \(0\leq \theta <(\frac{H}{\gamma})^{\beta}\) . The smallest concave majorant of \(g_{1}\) can be formed by drawing a straight line from \((0,g_{1}(0))\) which touches \(g_{1}\) at some \(\theta ^{*}>(\frac{H}{\gamma})^{\beta}\) . In particular, \(\theta ^{*}\) is a solution to \(\frac{g_{1}(\theta )-g_{1}(0)}{\theta}=g_{1}'(\theta )\) for \(\theta >(\frac{H}{\gamma})^{\beta}\) , i.e.,
We conjecture that the solution has the form \(\theta ^{*}=c^{\beta }(\frac{H}{\gamma})^{\beta}\) for some constant \(c>1\) . Then direct substitution shows that the constant \(c\) should solve ( 4.2 ). The smallest concave majorant of \(g_{1}\) is then
The value function is given by \(V_{1}(p;H)=\bar{g}_{1}(s(p))=\bar{g}_{1}(p^{\beta})\) , leading to ( 4.3 ). The corresponding optimal stopping time is
The optimal sale strategy is a gain-exit rule where the agent is looking to sell the asset when its price is sufficiently high without considering stop-loss. Note that the gain-exit target \(\frac{cH}{\gamma}\) is increasing in transaction costs (i.e., decreasing in \(\gamma \) ). This means that the agent tends to delay the sale decision in a more costly trading environment.
Inspired by the literature, we expect that more sophisticated exit strategies can be observed under alternative model setups. For example, the agent will consider stop-loss when the asset has negative drift (see Sect. 6.1 and Henderson [ 9 ]) or when there are multiple trading opportunities as in the realisation utility models (see Sect. 6.3.2 as well as Ingersoll and Jin [ 14 ] and He and Yang [ 8 ]). Introducing jump-diffusion price dynamics will further result in disconnected waiting regions where an agent who has recently suffered from a huge jump loss may refuse to stop-loss (Dai et al. [ 3 ] and Kong et al. [ 17 ]). We opt to work with a simpler baseline model to make the whole entry-and-exit problem as tractable as possible.
Since we require \(\beta >0\) , if the initial price of the asset is below the gain-exit target, there is a strictly positive probability that the asset is never sold. Moreover, an agent who fails to sell the asset at his target gain-exit level will suffer a total loss in the long run.
The expression of the target gain-exit threshold and in turn the value function of the exit problem are available in closed form, thanks to the specialisation that the degree of risk-aversion over gains is the same as that of risk-seeking over losses. This allows us to make a lot of analytical progress when studying the entry problem. We also lose the closed-form expressions in Lemma 4.3 if a fixed transaction cost on sales is introduced; in this case, the agent will only sell the asset when the utility proceed \(U(\gamma p - H-\Gamma )\) from the sale is larger than that of inaction \(U(-H)\) , where \(\Gamma \geq 0\) represents a fixed market exit fee. Then the payoff function of the exit problem becomes
We now proceed to describe the optimal entry strategy of the agent. The proofs of the two propositions below are given in the Appendix .
Proposition 4.7
Suppose \(\alpha \leq \beta < 1\) . For the entry problem ( 3.4 ), the value function is given by \(V_{2}(p)=\bar{g}_{2}(p^{\beta})\) , where \(\bar{g}_{2}=\bar{g}_{2}(\theta )\) is the smallest concave majorant of
There are three different cases :
(1) If \(\frac{\lambda}{\gamma}\leq (\frac{\alpha}{\beta k}c^{1-\beta}(c-1)^{ \alpha -1})^{\frac{1}{\beta}}\) , there exists \(p_{1}^{*}\in [0,\infty )\) such that the agent will enter the trade when the asset price is at or above \(p_{1}^{*}\) . The value function is
(2) If \(\frac{\lambda}{\gamma}> (\frac{\alpha}{\beta k}c^{1-\beta}(c-1)^{ \alpha -1})^{\frac{1}{\beta}}\) , there exists a constant \(C\in (0,\infty )\) independent of \(\Psi \) and \(R\) such that
(a) if \(\Psi < CR\) , there exist \(0\leq p_{1}^{*}< p_{2}^{*}<\infty \) such that the agent will enter the trade when the asset price is within the interval \([p_{1}^{*},p_{2}^{*}]\) . The value function is
(b) if \(\Psi \geq CR\) , the agent will never enter the trade . The value function is \(V_{2}(p)=-kR^{\alpha}\) . Moreover , in cases (1) and (2)(a), \(p_{1}^{*}=\frac{R+\Psi}{\gamma} (x_{1}^{*})^{1/\beta}\) , where \(x^{*}_{1}\) is the smaller solution to the equation
In case (2)(a), \(p_{2}^{*}=\frac{R+\Psi}{\gamma}(x_{2}^{*})^{1/\beta}\) , where \(x_{2}^{*}\) is the unique solution to the equation
In the special case \(\beta =1\) where the asset has zero drift, the results are slightly different from those in Proposition 4.7 .
Proposition 4.8
Suppose \(\alpha < \beta = 1\) . For the entry problem ( 3.4 ), we have :
(1) If \(\frac{\lambda}{\gamma}\leq \frac{\alpha}{k}(c-1)^{\alpha -1}\) , there exists \(p_{1}^{*}\in [0,\infty )\) such that the agent will enter the trade when the asset price is at or above \(p_{1}^{*}\) . The value function is given by ( 4.6 ) on setting \(\beta =1\) .
(2) If \(\frac{\alpha}{k}(c-1)^{\alpha -1}<\frac{\lambda}{\gamma}< \frac{(c-1)^{\alpha -1}}{k}\) , there exists a constant \(C\in (0,\infty )\) independent of \(\Psi \) and \(R\) such that
(a) if \(\Psi < CR\) , there exist \(0\leq p_{1}^{*}< p_{2}^{*}<\infty \) such that the agent will enter the trade when the asset price is within the interval \([p_{1}^{*},p_{2}^{*}]\) . The value function is given by ( 4.7 ) on setting \(\beta =1\) .
(b) if \(\Psi \geq CR\) , the agent will never enter the trade . The value function is \(V_{2}(p)=-kR^{\alpha}\) .
(3) If \(\frac{\lambda}{\gamma}\geq \frac{(c-1)^{\alpha -1}}{k}\) , the agent will never enter the trade . The value function is \(V_{2}(p)=-kR^{\alpha}\) .
Moreover , \(p_{1}^{*}\) has the same form as in Proposition 4.7 on setting \(\beta =1\) , and the expression of \(p_{2}^{*}\) is available in closed form as
For certain types of agents such as retail investors, fixed transaction costs are typically insignificant so that their trading behaviour might be better described by the specialisation \(\Psi =0\) . In this case, Propositions 4.7 and 4.8 can be simplified; see Corollary 4.11 and the discussion thereafter.
The value function of the entry problem is characterised as the smallest concave majorant of the payoff function in ( 4.4 ). Indeed, \(v_{1}\) defined in ( 4.4 ) is simply the scaled value function of the exit problem so that \(v_{1}(\theta )=V_{1}(\theta ^{1/\beta}; \lambda \theta ^{1/\beta}+ \Psi +R)\) , as discussed in Sect. 3.2.2 . At a mathematical level, the various cases arising in Propositions 4.7 and 4.8 are due to the different possible shapes of \(v_{1}\) under different combinations of parameters. Some illustrations are given in Fig. 1 .
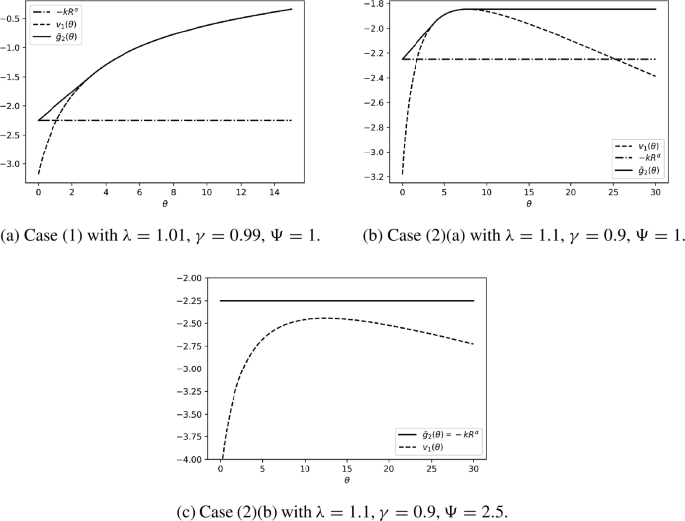
The scaled value functions of the entry problem for the different cases of Proposition 4.7 . Base parameters used are \(\alpha =0.5\) , \(k=2.25\) , \(R=1\) , \(\beta =0.85\)
Economically, the optimal entry strategy crucially depends on the level of transaction costs relative to the market and preference parameters. A fixed market entry fee in general discourages trading when the asset price is low. Paying a flat fee of $10 to purchase an asset at $20 is much less appealing compared to the case that the asset is trading at $1000, because in the former case the asset has to increase in value by 50% just to break even against the fixed transaction fee paid. Meanwhile, proportional transaction costs are the most significant for an asset trading at a high nominal price. A 10% transaction fee charged on a million worth of property is much more expensive in monetary terms relative to the same percentage fee charged on a penny stock.
In case (1) of both Propositions 4.7 and 4.8 , the proportional transaction costs are relatively low. Hence the agent does not mind purchasing the asset at a high nominal price. He will just avoid purchasing the asset when its price is very low due to the consideration of fixed transaction costs, and therefore the purchase region has the form \([p_{1}^{*},\infty )\) .
In case (2)(a), proportional transaction costs start becoming significant. On the one hand, the agent avoids initiating the trade when the asset price is too low since the fixed entry cost will be too large relative to the size of the trade. On the other hand, the agent does not want to trade an expensive asset when the proportional costs are large. Upon balancing these two factors, the agent will wait when the asset price is either too low or too high, and will only purchase the asset when the price first enters an interval \([p_{1}^{*}, p_{2}^{*}]\) . A very interesting feature of the optimal entry strategy is that the waiting region here is disconnected.
In case (2)(b) of Proposition 4.7 , or cases (2)(b) and (3) of Proposition 4.8 , the overall level of transaction costs is too high and hence the agent is discouraged from entering the trade in the first place. The key difference between Propositions 4.7 and 4.8 is that when the asset has a strictly positive drift ( \(\beta <1\) , i.e., \(\mu >0\) ), one must impose a strictly positive fixed entry cost in order to stop the agent from trading at all price levels (if \(\Psi =0\) , then either case (1) or (2)(a) in Proposition 4.7 applies, in which case the agent is willing to enter the trade at a certain price level). When the asset is a statistically fair gamble ( \(\beta =1\) , i.e., \(\mu =0\) ), a high proportional transaction cost alone is sufficient to discourage the agent from trading. It is interesting to note that the trading decision also depends on the agent’s aspiration level \(R\) . Comparing cases (2)(a) and (2)(b) in Propositions 4.7 and 4.8 , a low value of \(R\) will more often lead to the “no trading” case. The economic interpretation is that an agent with low aspiration level (i.e., a low target benchmark) is less likely to participate in trading, especially when the (proportional) costs of trading are high. In Sect. 6.4 , we briefly discuss how the aspiration level \(R\) may be endogenised.
When viewed in conjunction with the results of wellposedness (Lemma 4.1 ) and the optimal exit strategy (Lemma 4.3 ), our model can encapsulate many styles of trading behaviour. First, if \(\beta \leq 0\) or \(\beta <\alpha <1\) so that the problem is ill posed, we have already shown that a simple “buy and hold” strategy of the form ( 4.1 ) is optimal in the sense that the attained utility level can be arbitrarily high.
In cases (1) or (2)(a) of Propositions 4.7 and 4.8 , if the asset price starts below \(p_{1}^{*}\) at time zero, the agent will purchase the asset when its price level increases to \(p_{1}^{*}\) . Note that similarly to Remark 4.5 , the price process \(P\) may fail to reach a fixed level \(p_{1}^{*}>P_{0}\) in finite time. In this case, the entry strategy will not be executed and the payoff to the agent is zero. But otherwise, if a purchase is realised, then at the time of purchase, the reference point is set as \(H=\lambda p_{1}^{*}+\Psi +R\) . Then due to Lemma 4.3 , the agent is looking to sell this asset later when its price level further increases to \(\frac{cH}{\gamma}=\frac{c(\lambda p_{1}^{*}+\Psi +R)}{\gamma}\) . Since \(c>1\) , \(\gamma \leq 1\) and \(\lambda \geq 1\) , it is clear that the target sale level \(\frac{c(\lambda p_{1}^{*}+\Psi +R)}{\gamma}\) is strictly larger than \(p_{1}^{*}\) . This trading rule is thus a momentum strategy of the form “buy high, sell higher”.
If the asset price starts above \(p_{2}^{*}\) at time zero in case (2)(a), the agent will buy the asset when its price level drops to \(p_{2}^{*}\) and later sell when the price increases to \(\frac{c(\lambda p_{2}^{*}+\Psi +R)}{\gamma}>p_{2}^{*}\) . This is a counter-trend trading strategy of the form “buy low, sell high”.
Finally, in the high transaction cost cases (2)(b) of Proposition 4.7 and (2)(b) or (3) of Proposition 4.8 , the agent will never participate in trading at any asset price level.
The various cases above are generated by different levels of transaction costs relative to the other model parameters. The following two corollaries further highlight the role of transaction costs in relationship to the optimal trading strategies.
Corollary 4.10
If \(\lambda =\gamma =1\) , the agent will purchase the asset when its price level is at or above \(p_{1}^{*}\) for some \(p_{1}^{*}\in [0,\infty )\) .
The result follows if we can show that \((\frac{\alpha}{\beta k}c^{1-\beta}(c-1)^{\alpha -1})^{ \frac{1}{\beta}}>1\) so that case (1) of Propositions 4.7 and 4.8 always applies when \(\lambda =\gamma =1\) . Rewriting the required inequality in equivalent ways gives
where we have used ( 4.2 ). Using simple calculus, we can show that
for all \(x>1\) . This concludes the proof. □
Corollary 4.11
If the model parameters satisfy the conditions in cases (1) or (2)(a) in Propositions 4.7 and 4.8 and if \(\Psi =0\) , then we have \(p_{1}^{*}=0\) .
This follows immediately from Propositions 4.7 and 4.8 by observing that \(x= 0\) is the solution to ( 4.8 ) when \(\Psi =0\) . □
From Corollary 4.10 , if there is no proportional transaction cost, then the agent does not care about entering the trade at a high nominal price level because he no longer needs to worry about the large magnitude of the trading fee arising from the proportional nature of the transaction costs. Hence “buy low, sell high” will not be observed as an optimal strategy. Similarly, Corollary 4.11 suggests that in the absence of a fixed market entry fee, the agent is happy to purchase an asset at any arbitrarily low price (in the non-degenerate case) since now he does not need to take the size of the trade into account against any fixed cost for break-even considerations. Thus “buy high, sell higher” will not be an optimal strategy in this special case.
Remark 4.12
If we further assume \(R=\Psi =0\) (where the main results in Propositions 4.7 and 4.8 still hold except that ( 4.4 ) will have a different and simpler expression), then only case (1) or case (2)(b) of Proposition 4.7 can arise for \(\beta <1\) . Alternatively, only cases (1), (2)(b) or (3) of Proposition 4.8 can arise for \(\beta =1\) . But \(p_{1}^{*}=0\) when \(\Psi =0\) as in Corollary 4.11 . The entry behaviour is thus trivial—the agent either is willing to purchase the asset at any price level if \(\frac{\lambda}{\gamma}\leq (\frac{\alpha}{\beta k}c^{1-\beta}(c-1)^{ \alpha -1})^{\frac{1}{\beta}}\) , or never enters the trade if \(\frac{\lambda}{\gamma}>(\frac{\alpha}{\beta k}c^{1-\beta}(c-1)^{ \alpha -1})^{\frac{1}{\beta}}\) . From the modelling perspective, the constant \(R+\Psi \) is a crucial component which enables the model to produce non-trivial purchase behaviour; see Sect. 6.3.2 as well.
The decomposition of the original purchase-and-sale problem ( 2.3 ) into two sub-problems of optimal exit and optimal entry is based on some economic heuristics described in Sects. 3.2.1 and 3.2.2 . To close this section, we formally show that the value function of the entry problem in Propositions 4.7 and 4.8 indeed corresponds to the value function of the sequential optimal stopping problem ( 2.3 ).
Theorem 4.13
Recall the definition of \(V_{2}\) in Propositions 4.7 and 4.8 as the value function of the entry problem ( 3.4 ). We have \(\mathcal{V}(p)=V_{2}(p)\) , where \(\mathcal{V}\) is the value function of the sequential optimal stopping problem ( 2.3 ). The optimal purchase and sale rules are given by
Starting from ( 2.2 ), we have for any \(\tau ,\nu \in \mathcal{T}\) with \(\tau \leq \nu \) that
But using the Markovian structure of the problem,
Then we have
Taking the supremum on both sides leads to \(\mathcal{V}(p)\leq V_{2}(p)\) .
To complete the proof, it is sufficient to show \(J(p;\tau ^{*},\nu ^{*})=V_{2}(p)\) , where \(\tau ^{*},\nu ^{*}\) are defined in ( 4.10 ). This can be directly verified for the various cases covered in Propositions 4.7 and 4.8 with different initial price level \(p\) .
As an example, we cover case (2)(a) in Proposition 4.7 and a level \(p< p_{1}^{*}\) . We can deduce from the shape of \(V_{2}\) in this case that \(\tau ^{*}=\inf \{t\geq 0: P_{t}\notin (0,p_{1}^{*})\}\) . Since \(\beta >0\) , there are two possibilities: the asset price reaches the purchase target level \(p_{1}^{*}\) in finite time, which happens with probability \(\mathbb{P}[\tau ^{*}<\infty |P_{0}=p]= \frac{p^{\beta}}{(p_{1}^{*})^{\beta}}\) , or it never reaches \(p_{1}^{*}\) in finite time so that the agent never enters a trade and faces a realised utility of \(U(-R)\) , which happens with probability \(\mathbb{P}[\tau ^{*}=\infty |P_{0}=p]=1- \frac{p^{\beta}}{(p_{1}^{*})^{\beta}}\) . In the first scenario, after the agent purchases the asset at price \(p_{1}^{*}\) , he will sell the asset when its price further increases to \(x^{*}:=\frac{c}{\gamma}(\lambda p_{1}^{*}+\Psi +R)\) . The conditional probability of a successful sale is \(\mathbb{P}[\nu ^{*}<\infty |P_{\tau ^{*}}=p_{1}^{*}]= \frac{(p_{1}^{*})^{\beta}}{(x^{*})^{\beta}}\) , where the realised utility is \(U(\gamma x^{*}-\lambda p_{1}^{*}-\Psi -R)\) . Otherwise, the target sale level is never reached with conditional probability \(\mathbb{P}[\nu ^{*}=\infty |P_{\tau ^{*}}=p_{1}^{*}]=1- \frac{(p_{1}^{*})^{\beta}}{(x^{*})^{\beta}}\) , and the realised utility becomes \(U(-\lambda p_{1}^{*}-\Psi -R)\) . Then we can directly compute
where we have used the definition \(x^{*}:=\frac{c}{\gamma}(\lambda p_{1}^{*}+\Psi +R)\) and the fact that \(c\) is the solution to ( 4.2 ). The other cases can be handled similarly. □
5 Comparative statics of the optimal trading strategies
The critical trading boundaries in Propositions 4.7 and 4.8 , although not available in closed form in general, can be analysed to shed light on the comparative statics of the optimal trading strategies with respect to a few underlying model parameters. The proof of the following proposition is given in the Appendix .
Proposition 5.1
Away from case (2)(b) in Proposition 4.7 and cases (2)(b) and (3) in Proposition 4.8 , we have that
\(p_{1}^{*}\) is decreasing in \(\gamma \) and increasing in \(\Psi \) ;
\(p_{2}^{*}\) is decreasing in \(\lambda \) , increasing in \(\gamma \) and increasing in \(\Psi \) .
Figure 2 (b) shows the critical purchase boundaries \(p_{1}^{*}\) and \(p_{2}^{*}\) as \(\gamma \) varies. For very large values of \(\gamma \) such that the condition in case (1) of Proposition 4.7 holds, the optimal strategy is to buy the asset when its price exceeds \(p_{1}^{*}\) , and the agent is willing to enter the trade no matter how high the price is. Once \(\gamma \) is smaller than a certain critical value (labelled by the vertical dotted line in the figure), the parameter condition in case (2)(a) of Proposition 4.7 applies. The optimal strategy now becomes to purchase the asset only when its price is within a bounded range \([p_{1}^{*},p_{2}^{*}]\) . As \(\gamma \) further decreases, \(p_{1}^{*}\) increases while \(p_{2}^{*}\) decreases so that the purchase region \([p_{1}^{*},p_{2}^{*}]\) shrinks. Once \(\gamma \) reaches another critical value, \(p_{1}^{*}\) and \(p_{2}^{*}\) converge and the purchase region vanishes entirely. This corresponds to case (2)(b) of Proposition 4.7 that the agent will not enter the trade at any price level. As a reminder, the constant \(C\) in case (2) of Propositions 4.7 and 4.8 depends on \(\lambda \) and \(\gamma \) . Increasing \(\frac{\lambda}{\gamma}\) will result in a switch from case (2)(a) to case (2)(b).
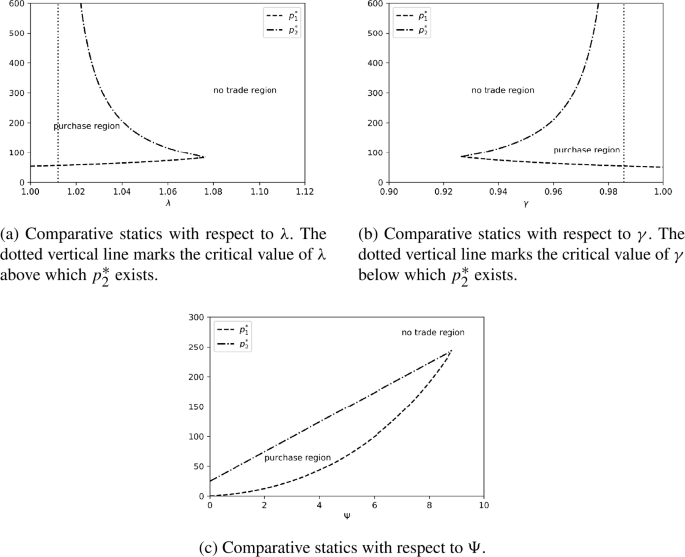
Comparative statics of the optimal purchase boundaries \(p_{1}^{*}\) and \(p_{2}^{*}\) . Base parameters used are \(\alpha =0.5\) , \(k=2.25\) , \(R=1\) , \(\beta =0.85\) , \(\lambda =1.05\) , \(\gamma =0.95\) , \(\Psi =5\)
We do not mention in Proposition 5.1 the effect of \(\lambda \) on \(p_{1}^{*}\) . Indeed, \(p_{1}^{*}\) is not monotonic in \(\lambda \) in general; see Fig. 3 . Hence when viewed in conjunction with \(p_{2}^{*}\) , the purchase region \([p_{1}^{*}, p_{2}^{*}]\) does not necessarily shrink uniformly when the proportional cost on purchases increases, i.e., the agent need not delay the purchase decision. Similar observations regarding potential non-monotonicity of trading decisions with respect to (proportional) transaction costs are made by Hobson et al. [ 12 , 13 ] in the context of portfolio optimisation.
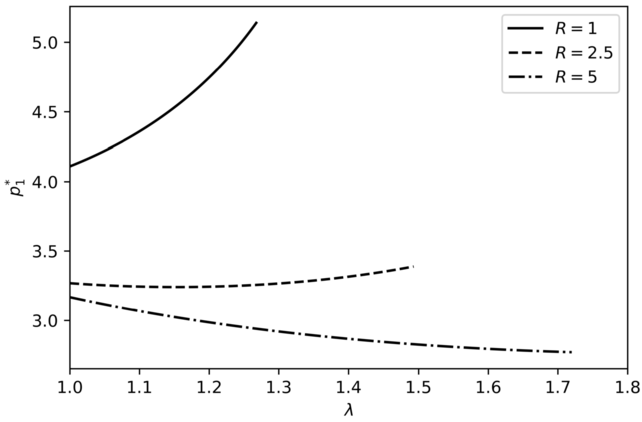
An illustration that \(p_{1}^{*}\) , the lower bound of the purchase region, need not be monotonic with respect to \(\lambda \) . Base parameters used are \(\alpha =0.5\) , \(k=2.25\) , \(\beta =0.85\) , \(\lambda =1.05\) , \(\gamma =0.95\) , \(\Psi =1\)
Similarly, we can also examine the impact of the fixed market entry cost on the purchase decision. As shown in Fig. 2 (c), \(p_{1}^{*}\) and \(p_{2}^{*}\) are both increasing in \(\Psi \) . The fact that \(p_{2}^{*}\) is increasing in \(\Psi \) is indeed somewhat counter-intuitive and has a few interesting policy implications. Suppose there is a market regulator who wants to discourage the agent from purchasing the asset (for example, as a means to cool down a highly speculative real estate market). A natural measure to curb trading participation is to increase transaction costs. However, Fig. 2 reveals that there is a subtle difference between the impact of proportional and fixed transaction costs on the agent’s trading behaviour.
From Fig. 2 (b), the effect of increasing proportional transaction costs on sales (i.e., decreasing \(\gamma \) ) is “monotonic” in terms of changing the trading decision of the agent. At any given current asset price level, decreasing \(\gamma \) (while all other parameters are held fixed) can only take the price from the purchase region to the no-trade region. Increasing proportional transaction costs on sales can therefore unambiguously suppress the trading activities in the market.
In contrast, the impact of the fixed market entry cost is somewhat unclear. Take Fig. 2 (c) as an example and suppose the current price of the asset is $100. If there is no fixed market entry fee initially (i.e., \(\Psi =0\) ), the agent will not participate in trading as the price is in the no-trade region. However, increasing \(\Psi \) from zero to $4 will now put the price in the purchase region so that the agent is willing to purchase the asset immediately (given that the current asset price stays the same at $100). This is exactly opposite to the intended outcome of the market regulator, because the increase in \(\Psi \) actually encourages trading participation.
The rationale behind this phenomenon is as follows. The speculative agent is evaluating the trading opportunity by comparing the potential sale proceeds against the reference point which is partly determined by the initial capital \(\lambda p + \Psi \) required to enter the trade if the purchase price is \(p\) . Increasing the fixed market entry fee \(\Psi \) increases the total costs required to initiate the trade and results in a higher effective reference point. However, under a prospect theory framework, the agent’s risk attitude is tied to the level of the reference point. When the purchase price is kept the same, a large \(\Psi \) will put the agent deeper in the loss territory over which he becomes highly risk-seeking. Thus he will give a higher valuation to the opportunity to enter the speculative trade and hence is more prone to immediate trade participation.
Of course, increasing \(\Psi \) will also decrease the potential profit of the trade, which is economically unfavourable. As the fixed cost further increases, say from \(\Psi =4\) to \(\Psi =8\) , the price will eventually enter the no-trade region again. Hence the precise effect of \(\Psi \) on the trading decision is ambiguous and governed by the two opposing forces of increasing the agent’s risk appetite versus decreasing profitability. When the economy is consisting of multiple agents with heterogeneous preferences, it is unclear whether increasing the fixed transaction costs can uniformly discourage trading participation for all agents.
The non-monotonicity of \(p_{1}^{*}\) with respect to \(\lambda \) , the proportional transaction cost on purchases, also implies that an increase in \(\lambda \) can potentially bring certain prices from the no-trade region to the purchase region. The rationale is the same as above that \(\lambda \) partly determines the cost of purchase and in turn the reference point. Hence increasing \(\lambda \) might actually make an agent find a speculative opportunity attractive.
6 Extensions
In this section, we briefly discuss several variations of our baseline model.
6.1 Risky asset with negative drift and voluntary stop-loss
Among all the non-trivial strategies derived in our baseline setup, the agent will never voluntarily realise a loss. This is not consistent with real world trading behaviour given the prevalent usage among market participants of stop-loss orders. One way to enable the model to generate stop-loss behaviour is to allow the excess return of the asset to be negative as inspired by Henderson [ 9 ]. A negative excess return is equivalent to \(\beta :=1-\frac{2\mu}{\sigma ^{2}}>1\) in our setup.
Suppose the model parameters are such that \(\beta >1\) . For the exit problem ( 3.2 ), the agent will sell the asset when its price level first exits the interval \(\big(\frac{c_{1} H}{\gamma},\frac{c_{2} H}{\gamma}\big)\) , where \(0< c_{1}<1<c_{2}\) are the solutions to the system of equations
The value function is
This follows from a slight extension of [ 9 , Proposition 3]. □
Given that a purchase of the asset has occurred at some time \(\tau \) which determines the reference level for the exit problem via \(H=\lambda P_{\tau}+\Psi +R\) , the agent is willing to impose a stop-loss level at \(\frac{c_{1} H}{\gamma}\) if the asset has a negative drift. Of course, it is not clear upfront whether the agent is willing to purchase an asset with negative drift in the first place. To understand the purchase behaviour, one needs to solve the entry problem
where \(V_{1}\) is now given by ( 6.1 ). Although the principle of the martingale method still applies, we have so far not managed to analyse the problem thoroughly to explicitly characterise different possible shapes of the scaled payoff function under different combinations of model parameters. We hence opt to obtain numerical solutions by solving the underlying variational inequality
by the standard projected successive over-relaxation (PSOR) method and infer the optimal purchase (stopping) and no-trade (continuation) regions by numerically identifying the sets \(\{p\geq 0: V_{2}(p)=G_{2}(p)\}\) and \(\{p\geq 0: V_{2}(p)>G_{2}(p)\}\) , respectively.
Figure 4 (a) shows the optimal entry decisions as \(\beta \) varies. When \(\beta \leq 1\) , the optimal purchase boundaries \(p_{1}^{*}\) and \(p_{2}^{*}\) are obtained semi-analytically from Propositions 4.7 and 4.8 , while the values for \(\beta >1\) are obtained numerically by the PSOR method. The agent is willing to purchase the asset if and only if the current price level is between \(p_{1}^{*}\) and \(p_{2}^{*}\) . As the drift of the asset becomes more and more negative (i.e., \(\beta \) increases), the purchase region \([p_{1}^{*},p^{*}_{2}]\) shrinks and eventually vanishes when \(\beta \) is around 1.4. Beyond this critical level, the agent will not purchase the asset at any price level because of its poor quality.
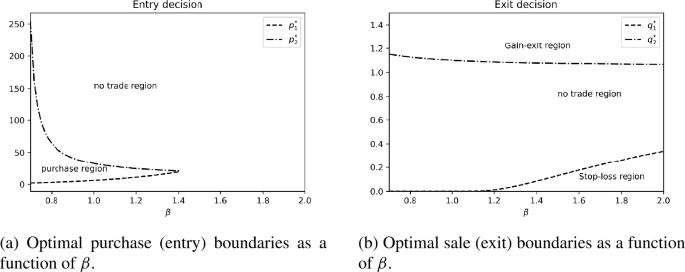
Optimal purchase and sale decisions as \(\beta \) varies; \(\beta < 1\) (resp. \(\beta >1\) ) indicates that the asset has a positive (resp. negative) drift. Base parameters used are \(\alpha =0.5\) , \(k=2.25\) , \(R=1\) , \(\lambda =1.05\) , \(\gamma =0.95\) , \(\Psi =1\)
In parallel, Fig. 4 (b) plots the optimal sale boundaries in the form \(q^{*}_{i}:=\frac{c_{i} }{\gamma}\) so that if the asset has been purchased at level \(p\) , it will be sold when its price level leaves the interval \((H q_{1}^{*},H q_{2}^{*})\) , where \(H=\lambda p+\Psi +R\) . If \(\beta \leq 1\) , it is never optimal to voluntarily realise a loss, which is equivalent to \(q_{1}^{*}=0\) . But for \(\beta >1\) , \(q^{*}_{1}\) becomes strictly positive which represents a stop-loss level. Figure 4 demonstrates that there exist some combinations of model parameters such that the agent is willing to purchase the asset at some price level and subsequently liquidate it at a loss. This happens when \(\beta \) is between 1 and 1.4 in our numerical example. Explicitly characterising conditions on the model parameters where this phenomenon occurs is an interesting research question.
6.2 Subjective discounting
To investigate the effect of impatience on the optimal strategy, it is constructive to consider a version of the problem with discounting. However, to the best of our knowledge, there is no consensus in the literature how discounting should be incorporated within a prospect theory framework with intertemporal cashflows. We briefly present two possible modelling choices.
6.2.1 Profit-discounting
The first idea is that the S-shaped utility function is applied to the net present value of the trading proceed, which we term as “ profit-discounting ”. We consider the problem
where \(\delta >0\) is the subjective discount factor. If we further assume \(\Psi =0\) (which is perhaps relevant in the context of retail trading where the fixed transaction cost is insignificant), then this problem is the same as the undiscounted one except that the drift of the asset is now replaced by \(\mu -\delta \) under the geometric Brownian motion assumption for the asset price. For small \(\delta \) such that \(\delta \leq \mu \) , our baseline results under the standing assumption \(\beta \leq 1\) apply. Otherwise, when \(\delta >\mu \) , the analysis becomes similar to the one in Sect. 6.1 . Increasing \(\delta \) has the same effect as increasing \(\beta \) , where an endowed asset tends to be liquidated sooner (lower gain-exit level and possibly higher stop-loss level) while the purchase region shrinks; see Fig. 5 . This result is quite interesting because impatience affects sale and purchase decisions somewhat differently. Increasing impatience will cause an agent who already owns the asset to sell sooner, which is in line with common intuition; but surprisingly, a higher \(\delta \) will also delay the purchase decision. The economic rationale is that an increasing discount rate makes the opportunity to sell the asset less valuable, and hence the agent is more reluctant to enter the trade in the first place.
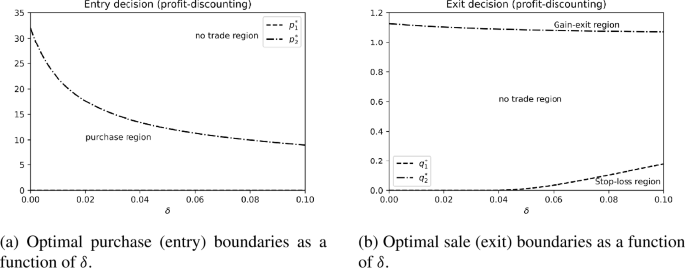
Optimal purchase and sale decisions as \(\delta \) varies under the profit-discounting criterion. Base parameters used are \(\alpha =0.5\) , \(k=2.25\) , \(R=1\) , \(\lambda =1.05\) , \(\gamma =0.95\) , \(\Psi =0\) , \(\mu =0.025\) , \(\sigma =0.5\)
If we insist on \(\Psi >0\) , then the objective function has an explicit dependence on \(\tau \) which will make the entry problem time-inhomogeneous. Such a problem is more difficult to be analysed analytically or numerically.
6.2.2 Utility-discounting
The second possibility to incorporate discounting is to assume the utility of the round-trip proceed is discounted by a single discount factor evaluated at the liquidation date. We call this approach “ utility-discounting ”. The problem is formulated as
The downside of this approach is that it does not properly take into the account the timing of the cash outflow (incurred at \(\tau \) ) and inflow (incurred at \(\nu \) ), but an advantage is that this formulation resembles a standard discounted optimal stopping problem.
It turns out that introducing discounting in this fashion will drastically change the agent’s entry behaviour, as summarised by the result below whose proof is given in Appendix A.2 .
Proposition 6.3
Suppose \(\delta >0\) and let \(\omega _{1}<0<\omega _{2}\) be the two distinct real roots of the quadratic equation \(\frac{\sigma ^{2}}{2}x^{2}+\big(\mu -\frac{\sigma ^{2}}{2}\big)x- \delta =0\) . Under the assumption \(\alpha < \beta \) , the pair of stopping times
is optimal for ( 6.2 ). In other words , the agent always enters the trade immediately at any price level and subsequently adopts a gain - exit strategy .
Proposition 6.3 applies to the case \(\beta >1\) as well. Unlike profit-discounting, the agent will never stop-loss under utility-discounting even when the asset drift is negative. More remarkably, the entry strategy now becomes trivial, provided that the standing assumption \(\alpha <\beta \) holds. It is also interesting to point out that the optimal strategy is not continuous at \(\delta =0\) , in the sense that letting \(\delta \downarrow 0\) in Proposition 6.3 does not recover the no-discounting baseline results in Propositions 4.7 or 4.8 . See Fig. 6 for an illustration where we show the optimal sale boundary as a function of \(\delta \) under utility-discounting. Once discounting is applied to the utility term (no matter how small \(\delta \) is), the impact of a poor trading performance in the form of negative utility can be mitigated by indefinitely deferring the realisation of a loss. The agent is then effectively protected from negative outcomes, and there is no downside to taking risk regardless of the asset quality or level of the transaction costs. The agent is impatient so that it is optimal to enter the trade as early as possible, and there is no reason to realise a loss thereafter because it can be discounted away.
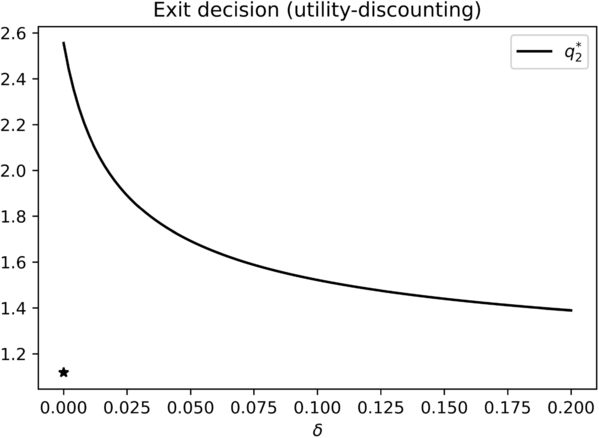
Optimal sale decisions as \(\delta \) varies under the utility-discounting criterion, where the agent will sell the endowed asset whenever the price level is at or above \(q^{*}_{2} H\) . There is a discontinuity at \(\delta =0\) , where the star ∗ marks the optimal threshold for the undiscounted problem. Base parameters used are \(\alpha =0.5\) , \(k=2.25\) , \(\beta =0.85\) , \(\lambda =1.05\) , \(\gamma =0.95\) , \(\Psi =1\)
In view of the above results, profit-discounting seems to yield more reasonable and flexible predictions of the agent’s optimal trading behaviour. Nonetheless, a proper understanding of the implications behind the two discounting approaches is of interest to the field of behavioural economics.
6.3 Multiple round-trip trades
We have exclusively focused on the case that the agent’s utility is derived from a single round-trip trade. But what if the agent can repeatedly purchase and sell the asset? Similarly to Sect. 6.2 , we discuss two possible modelling choices how utility over multiple trades can be computed.
6.3.1 Optimisation of utility over total trading profit
The first possibility is to consider utility derived from the total net present value of the trading proceeds, which is similar to the profit-discounting idea in Sect. 6.2 . Suppose the agent can perform \(N\) round-trip trades; then the objective function can be written as
Here \((\tau _{i})_{i=1,\dots ,N}\) (resp. \((\nu _{i})_{i=1,\dots ,N}\) ) is an increasing sequence of stopping times representing the entry (resp. exit) time of the \(i\) th trade with
In particular, the agent’s utility is now derived from the total trading profits from the \(N\) available round-trip trading opportunities.
The problem can still be approached by a similar backward induction principle which we outline below based on heuristics. Define
Here \(V^{(n)}_{b}(p;Q)\) represents the value function when there are \(n\) purchase and sale opportunities available under some reference point \(Q\) , and \(V^{(n)}_{s}(p;H)\) represents the value function when the agent already owns the asset and there are \(n-1\) purchase and \(n\) sale opportunities available under some reference point \(H\) . Then heuristically, we expect from the dynamic programming principle that
Hence we expect that
The first equality is due to the definition of \(V^{(n)}_{s}\) , and the second is expected to hold because for the optimal stopping problem in ( 6.4 ), it is clearly suboptimal to stop the process when \(V^{(n)}_{s}(P_{t};\lambda P_{t}+\Psi +Q)< U(-Q)\) and hence the payoff function can be replaced by \(\max \{V^{(n)}_{s}(P_{\tau _{1}};\lambda P_{\tau _{1}}+\Psi +Q),U(-Q) \}\) .
Based on a similar reasoning, we expect for \(n>1\) that
Then we expect that
The last equality holds because \(V^{(n)}_{b}(p;Q)\) is obviously increasing in \(p\) and decreasing in \(Q\) so that \(V^{(n)}_{b}(p;-\gamma p+H)\geq V^{(n)}_{b}(0;H)= U(-H)\) for all \(p\) and \(n\) . Finally, we obviously have
In summary, \(V^{(n)}_{s}\) and \(V^{(n)}_{b}\) should satisfy the recursion
The required value function for ( 6.3 ) with \(N\) round-trip trading opportunities is given by \(V^{(N)}_{b}(p;R)\) . A formal verification of the above assertion as well as a thorough analysis of this recursive problem is beyond the scope of the current paper.
6.3.2 Optimisation of total utilities from individual trading episodes
One may also assume that a burst of utility is derived upon completion of each round-trip trade, i.e., the objective function with \(N\) round-trip trading opportunities is
Unlike the criterion in Sect. 6.3.1 where a single utility is derived from the total profit of all trades, utility is now realised upon completion of each round-trip trade and the agent’s goal is to optimise the sum of those utilities. Define
Here \(V^{(n)}_{b}(p)\) is the value function with \(n\) purchase and sale opportunities remaining, and \(V^{(n)}_{s}(p;H)\) represents the value function when agent has an endowed asset (with some given reference point \(H\) for the first trading episode) and there are \(n-1\) purchase and \(n\) sale opportunities remaining.
Based on the dynamic programming principle, one can heuristically write down the recursive system satisfied by the value functions as
where ( 6.6 ) holds for \(n\geq 1\) and ( 6.7 ) holds for \(n\geq 2\) . For the “payoff function” in ( 6.7 ), the first term \(U(\gamma P_{\nu}-H)\) represents the utility burst when the endowed asset is sold, and the second term \(V_{b}^{(n-1)}(P_{\nu})\) reflects the maximal sum of expected utilities from the remaining \(n-1\) round-trip trading opportunities. Otherwise, if the agent does not own the asset to begin with and decides to purchase at time \(\tau \) , the maximal expected utility attainable is given by \(V^{(n)}_{s}(P_{\tau};\lambda P_{\tau}+\Psi +R)\) , where a new trading episode is initiated with reference point set to \(H=\lambda P_{\tau}+\Psi +R\) (the total cost of purchase at time \(\tau \) plus the exogenous aspiration level). But the agent can also choose not to purchase at all and forgo all the \(n\) remaining trading opportunities. This will result in a payoff of \(U(-R)\) for each trading opportunity given up. Hence in ( 6.6 ) the “payoff function” for the entry problem is given by \(\max \{V^{(n)}_{s}(P_{\tau};\lambda P_{\tau}+ \Psi + R),n U(-R) \}\) . When \(n=1\) , ( 6.5 ) and ( 6.6 ) agree with the sequential optimal stopping problem deduced in Sect. 3 .
This generalisation is conceptually close to the realisation utility model in the literature. The canonical formulation (in our notation) of such a model is
where \(Q=(Q_{t})_{t\geq 0}\) is the reference point process so that \(Q_{t}\) is the benchmark to be used for performance evaluation at time \(t\) , and \(G=(G_{t})_{t\geq 0}\) is the gain-and-loss process with \(G_{t}:= \gamma P_{t} - Q_{t}\) representing the size of realised gain-and-loss if the agent liquidates an owned asset at time \(t\) . Typically, the function \(U(G,Q)\) is assumed to be homogeneous in \(Q\) so that \(U(G,Q)=Q^{\eta}u(G/Q)\) for some \(\eta \in (0,1]\) and \(u(\,\cdot \,)\) is S-shaped.
There are many choices for the reference point process \(Q\) . Ingersoll and Jin [ 14 ] consider \(Q_{t}= P_{\tau _{i}}\) for \(t\in [\tau _{i},\tau _{i+1})\) (up to a constant multiplier), which is simply the most recent purchase price of the asset. Barberis and Xiong [ 2 ] and Dai et al. [ 3 ] take \(Q_{t}= P_{\tau _{i}} e^{r(t-\tau _{i})}\) for \(t\in [\tau _{i},\tau _{i+1})\) , which is the most recent purchase price growing at the risk-free rate. He and Yang [ 8 ] incorporate an additional term which asymmetrically adapts to the paper gain-and-loss. Kong et al. [ 17 ] study a path-dependent reference point which is a weighted average of the asset prices throughout the current trading episode. A common feature of the papers cited above is that \(Q_{t}\) is proportional to \(P_{\tau _{i}}\) over \(t\in [\tau _{i},\tau _{i+1})\) , and hence a dimension reduction is possible via introducing the new state variable \(X_{t}:=P_{t}/Q_{t}\) . This greatly simplifies the entry problem, but it also trivialises the optimal strategy—one either immediately enters the trade again after a sale or never enters the trade in the first place. This observation remains the same even if one introduces additional modelling elements such as a Poisson random termination time and an extra utility term over final wealth; see Proposition 3.4 of He and Yang [ 8 ].
Our formulation can be seen as a version of the realisation utility model with a finite number of trading opportunities, where the reference level process is (see Remark 6.4 below as well)
the gain-and-loss process is G t : = γ P t 1 { t < ∞ } − Q t , the utility function is given by \(U(G,Q)=Q^{\alpha}u(G/Q)\) with u ( x ) : = x α 1 { x ≥ 0 } − k | x | α 1 { x < 0 } , and the discount rate \(\delta \) is set to zero. The most important distinction of our framework from the existing realisation utility models is that our reference point consists of a constant component \(\Psi +R\) reflecting a fixed transaction cost and some baseline aspiration level. Without this component, the reference level over a particular trading episode is always proportional to the asset value at the beginning of the episode. Specifically, if \(\Psi =R=0\) , then using ( 6.5 )–( 6.7 ), the scaling property of \(U\) and the geometric Brownian motion assumption of \(P\) , we can inductively deduce for all \(n\) that \(V^{(n)}_{s}(p;H)=H^{\alpha}V(\frac{p}{H};1)\) and
If \(V^{(n)}_{s}(1/\lambda ;1)<0\) , then \(\tau \equiv +\infty \) is optimal and the associated value function for the entry problem is \(V^{(n)}_{b}(p)=0\) . If instead \(V^{(n)}_{s}(1/\lambda ;1)>0\) , then we have \(V^{(n)}_{b}(p)=\lambda ^{\alpha}V^{(n)}_{s}(1/\lambda ;1)\sup _{\tau} \mathbb{E}[P_{\tau}^{\alpha}|P_{0}=p]=\lambda ^{\alpha}V^{(n)}_{s}(1/ \lambda ;1)p^{\alpha}\) under the standing assumption \(\alpha \leq \beta \) . The corresponding optimal entry strategy is \(\tau \equiv 0\) .
The above observations in conjunction with our main theoretical results for \(n=1\) suggest that incorporating a constant component within the dynamic reference point (e.g. in the form of a fixed transaction cost or a default aspiration level) might enable a realisation utility model to generate more realistic and non-trivial purchase behaviours. For the sale decision, recall that in the baseline model with \(n=1\) , the optimal sale strategy is a simple gain-exit rule (Lemma 4.3 ). We expect this to change when \(n>1\) , since the effective payoff function of the exit problem ( 6.7 ) now contains an additional term \(V^{(n-1)}_{b}(p)\) which will drastically change the convexity/concavity of the scaled exit payoff function. We leave the full analysis of this problem for future work.
At first sight, ( 6.8 ) looks more complicated than the models proposed in the existing literature because our value of \(Q_{t}\) depends on whether the agent is inside a trading episode holding the asset ( \(t\in [\tau _{i},\nu _{i})\) for some \(i\) ) or outside a trading episode without any asset ( \(t\in [\nu _{i},\tau _{i+1})\) for some \(i\) ). From an economic point of view, the reference point should not depend on \(P_{\tau _{i}}\) any more once the \(i\) th trading episode is complete, and hence should be reset to the baseline aspiration level until the start of the \((i+1)\) th trading episode. In the special case \(\tau _{i+1}=\nu _{i}\) for all \(i\) so that there is no time delay between the exit of an existing trade and the entry of a new trade, ( 6.8 ) simplifies to \(Q_{t}=\lambda P_{\tau _{i}}+\Psi +R\) for \(t\in [\tau _{i},\tau _{i+1})\) , which resembles the usual definition in the literature. In the absence of a constant component within the reference point process, the entry decision is trivial as \(\tau _{i+1}=\nu _{i}\) for all \(i\) is indeed optimal because of the scaling property discussed previously; so there is no need to “correctly” specify \(Q_{t}\) over \(t\in [\nu _{i},\tau _{i+1})\) . But this result is no longer true when \(R\) or \(\Psi \) is non-zero, and hence it is necessary to model the reference point process more carefully.
6.4 Endogenous aspiration level
Among all the model parameters, the “aspiration level” \(R\) of the agent is the hardest one to be interpreted and estimated. It can be a purely psychological parameter representing the agent’s subjective threshold which distinguishes gains and losses. In some applications such as delegated portfolio management, \(R\) can also be the performance target imposed on the agent by a manager. Instead of calibrating \(R\) where the exercise can be very context-specific, one may seek to endogenise this parameter by introducing a further optimality criterion.
Consider a principal–agent setup as an example. The principal imposes an aspiration level on the agent in the form of a performance target. For a given \(R\) , the agent’s optimal trading rule \((\tau ^{*}(R),\nu ^{*}(R))\) can be obtained by solving ( 2.3 ). If the principal has a utility function \(\tilde{U}(\,\cdot \,)\) over the trading profit, then a particular choice of \(R\) will bring the principal an expected utility level of
where we have suppressed the dependence of \(\tilde{V}\) on \(p\) . The above can be maximised (numerically) with respect to \(R\) , where the solution in general depends on the initial asset price \(P_{0}\) . In Fig. 7 , we consider a risk-averse principal with utility function \(\tilde{U}(x)=-e^{-\eta x}\) , where \(\eta >0\) is the constant absolute risk-aversion level and \(\tilde{V}(R)\) can be maximised at some interior \(R\) in this particular example. The higher the Sharpe ratio of the asset (equivalent to a lower level of \(\beta \) ), the higher the level of endogenous aspiration. In other words, a more aggressive performance goal is set in a bullish market. However, there are also examples where the principal’s maximisation problem is degenerate (e.g. \(\tilde{V}(R)\) being monotonically increasing or decreasing in \(R\) ). We leave the complete analysis of such principal–agent problems for future research.
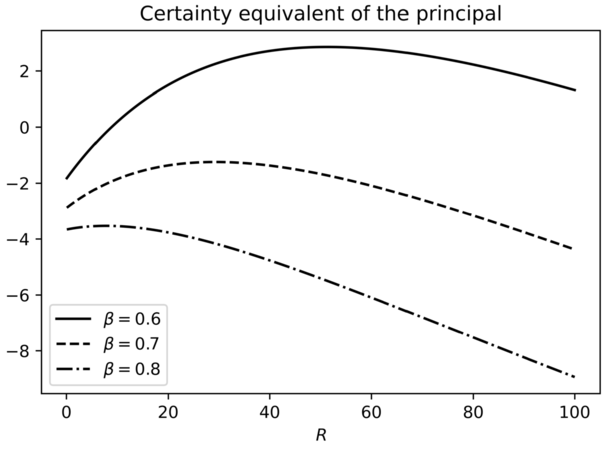
The principal’s certainty equivalent \(-\ln (- \tilde{V}(R))/\eta \) as a function of \(R\) when \(\tilde{U}(x)=-e^{-\eta x}\) . Base parameters used are \(\alpha =0.5\) , \(k=2.25\) , \(\lambda =1.05\) , \(\gamma =0.95\) , \(\Psi =1\) , \(\eta =0.01\) , \(P_{0}=30\)
An alternative consideration to endogenise \(R\) is to modify the agent’s utility function such that a round-trip profit of \(x\) leads to a utility value of \(U(x-R,R)\) , where \(U(\,\cdot \,,\,\cdot \,)\) is increasing in the first and decreasing in the second argument. The second argument of \(U\) can reflect the agent’s desire for “self-improvement” and “self-enhancement” which is achieved by choosing a high aspiration level \(R\) ; see for example Falk and Knell [ 7 ]. The optimal \(R\) can then be determined alongside with the agent’s optimal trading rule.
7 Concluding remarks
This paper considers a dynamic trading model under prospect theory preference with transaction costs. By solving a sequential optimal stopping problem, we find that the optimal trading strategy can have various forms depending on the model parameters and the price level of the asset. The impact of transaction costs is subtle. In contrast to conventional wisdom, increasing transaction costs does not necessarily deter economic agents from trading participation because the agents may face a higher reference point and in turn be more risk-aggressive in an expensive trading environment. These results could potentially be useful to policy makers to better understand how undesirable speculative trading behaviour in certain markets can be curbed effectively.
Our key mathematical results are derived under a somewhat stylised modelling specification. In particular, asymmetry of the degree of risk-aversion/seeking over gains/losses, a fixed transaction cost on sales and a negative aspiration level are currently omitted from the baseline analysis. While these omissions allow us to derive a sharp characterisation and comparative statics of the optimal trading rules, it will nonetheless be constructive to extend the model to further examine the impact of other economic factors. Section 6 also highlights a number of possible extensions for further rigorous mathematical analysis in the presence of a negative drift, subjective discounting, repeated trading opportunities and an endogenous aspiration level. In particular, a natural extension is to further explore the implications of our results for the literature of realisation utility, where we believe that a different specification of the reference point process (e.g. incorporation of a constant component) can lead to more realistic predictions of purchase behaviour.
A more ambitious goal is to further incorporate probability weighting within our continuous-time optimal stopping model (as in Xu and Zhou [ 28 ] and Henderson et al. [ 10 ]) to fully reflect the features of the cumulative prospect theory framework of Tversky and Kahneman [ 27 ]. However, technical difficulties are likely to arise due to the time-inconsistency brought by probability weighting. A precise formulation of the problem as well as the development of appropriate mathematical techniques should be an another interesting proposal for future research.
Finally, the surprising comparative statics documented in this paper also reveal that the economic interaction between market frictions and behavioural preferences can be subtle or even counter-intuitive. The model considered in this paper is just one of the many possible motivations that these two topics should not be studied in isolation, and this could potentially open up a new strand of literature to advance our understanding towards trading behaviour in a more realistic setup.
Bai, H., Zhang, L.: Searching for the equity premium. J. Financ. Econ. 143 , 897–926 (2022)
Article Google Scholar
Barberis, N., Xiong, W.: Realisation utility. J. Financ. Econ. 104 , 251–271 (2012)
Dai, M., Qin, C., Wang, N.: Portfolio rebalancing with realisation utility. Working Paper 29821, National Bureau of Economic Research, 2022. Available online at https://www.nber.org/papers/w29821
Dai, M., Yang, Z., Zhang, Q., Zhu, Q.J.: Optimal trend following trading rules. Math. Oper. Res. 41 , 626–642 (2016)
Article MathSciNet MATH Google Scholar
Dai, M., Zhang, Q., Zhu, Q.J.: Trend following trading under a regime switching model. SIAM J. Financ. Math. 1 , 780–810 (2010)
Dayanik, S., Karatzas, I.: On the optimal stopping problem for one-dimensional diffusions. Stoch. Process. Appl. 107 , 173–212 (2003)
Falk, A., Knell, M.: Choosing the Joneses: endogenous goals and reference standards. Scand. J. Econ. 106 , 417–435 (2004)
He, X., Yang, L.: Realisation utility with adaptive reference points. Math. Finance 29 , 409–447 (2019)
Henderson, V.: Prospect theory, liquidation, and the disposition effect. Manag. Sci. 58 , 445–460 (2012)
Henderson, V., Hobson, D., Tse, A.S.L.: Probability weighting, stop-loss and the disposition effect. J. Econ. Theory 178 , 360–397 (2018)
Henderson, V., Muscat, J.: Partial liquidation under reference-dependent preferences. Finance Stoch. 24 , 335–357 (2020)
Hobson, D., Tse, A.S.L., Zhu, Y.: Optimal consumption and investment under transaction costs. Math. Finance 29 , 483–506 (2019)
Hobson, D., Tse, A.S.L., Zhu, Y.: A multi-asset investment and consumption problem with transaction costs. Finance Stoch. 23 , 641–676 (2019)
Ingersoll, J.E., Jin, L.J.: Realisation utility with reference-dependent preferences. Rev. Financ. Stud. 26 , 723–767 (2013)
Kahneman, D., Lovallo, D.: Timid choices and bold forecasts: a cognitive perspective on risk taking. Manag. Sci. 39 , 17–31 (1993)
Kahneman, D., Tversky, A.: Prospect theory: an analysis of decision under risk. Econometrica 47 , 363–391 (1979)
Kong, L., Qin, C., Yue, X.: Realisation utility with path-dependent reference points. SIAM J. Financ. Math. 13 , 1063–1111 (2022)
Article MATH Google Scholar
Kyle, A.S., Ou-Yang, H., Xiong, W.: Prospect theory and liquidation decisions. J. Econ. Theory 129 , 273–288 (2006)
Leung, T., Li, X.: Optimal mean reversion trading with transaction costs and stop-loss exit. Int. J. Theor. Appl. Finance 18 , 1550020 (2015)
Leung, T., Li, X., Wang, Z.: Optimal multiple trading times under the exponential OU model with transaction costs. Stoch. Models 31 , 554–587 (2015)
Lopes, L.L., Oden, G.C.: The role of aspiration level in risky choice: a comparison of cumulative prospect theory and SP/A theory. J. Math. Psychol. 43 , 286–313 (1999)
Mehra, R., Prescott, E.C.: The equity premium: a puzzle. J. Monet. Econ. 15 , 145–161 (1985)
Merton, R.C.: Lifetime portfolio selection under uncertainty: the continuous-time case. Rev. Econ. Stat. 51 , 247–257 (1969)
Merton, R.C.: Optimum consumption and portfolio rules in a continuous-time model. J. Econ. Theory 3 , 373–413 (1971)
Song, Q., Yin, G., Zhang, Q.: Stochastic optimisation methods for buying-low-and-selling-high strategies. Stoch. Anal. Appl. 27 , 523–542 (2009)
Tversky, A., Kahneman, D.: The framing of decisions and the psychology of choice. Science 211 (4481), 453–458 (1981)
Tversky, A., Kahneman, D.: Advances in prospect theory: cumulative representation of uncertainty. J. Risk Uncertain. 5 , 297–323 (1992)
Xu, Z.Q., Zhou, X.Y.: Optimal stopping under probability distortion. Ann. Appl. Probab. 23 , 251–282 (2013)
Zervos, M., Johnson, T.C., Alazemi, F.: Buy-low and sell-high investment strategies. Math. Finance 23 , 560–578 (2013)
Zhang, H., Zhang, Q.: Trading a mean-reverting asset: buy low and sell high. Automatica 44 , 1511–1518 (2008)
Download references
Acknowledgement
The authors are grateful to two anonymous reviewers whose comments and suggestions helped improve the previous version of the paper. This research was supported in part by the EPSRC (UK) grant EP/V008331/1.
Author information
Authors and affiliations.
Department of Mathematics, University College London, London, WC1H 0AY, UK
Alex S. L. Tse
Department of Mathematics, Imperial College London, London, SW7 2AZ, UK
Harry Zheng
You can also search for this author in PubMed Google Scholar
Corresponding author
Correspondence to Alex S. L. Tse .
Ethics declarations
Competing interests.
The authors declare no competing interests.
Additional information
Publisher’s note.
Springer Nature remains neutral with regard to jurisdictional claims in published maps and institutional affiliations.
1.1 A.1 Proofs of Propositions 4.7 , 4.8 and 5.1
We start with two useful lemmas.
Write \(\xi :=\frac{\lambda}{\gamma}\) . For the function \(f\) defined in ( 4.5 ), we have
1) Suppose \(\alpha <\beta <1\) . Then :
(a) If \(\xi \leq (\frac{\alpha}{\beta k}c^{1-\beta}(c-1)^{\alpha -1})^{ \frac{1}{\beta}}\) , then \(f\) is an increasing concave function .
(b) If \(\xi >(\frac{\alpha}{\beta k}c^{1-\beta}(c-1)^{\alpha -1})^{ \frac{1}{\beta}}\) , then \(f\) is concave increasing on \([0,x_{2}^{*}]\) , concave decreasing on \([x_{2}^{*},\tilde{x}]\) , and convex decreasing on \([\tilde{x},\infty )\) . Here \(x_{2}^{*}\) and \(\tilde{x}\) are respectively the solutions to the equation
2) Suppose \(\alpha <\beta =1\) . Then :
(a) If \(\xi \leq \frac{\alpha}{k}(c-1)^{\alpha -1}\) , then \(f\) is an increasing concave function .
(b) If \(\frac{\alpha}{ k}(c-1)^{\alpha -1}<\xi \leq \frac{1}{ k}(c-1)^{ \alpha -1}\) , then \(f\) is concave increasing on \([0,x_{2}^{*}]\) , concave decreasing on \([x_{2}^{*},\tilde{x}]\) , and convex decreasing on \([\tilde{x},\infty )\) with
(c) If \(\xi >\frac{1}{ k}(c-1)^{\alpha -1}\) , then \(f\) is a decreasing function .
We can rewrite \(f\) as
so that \(\lim _{x\to \infty}f(x)=\pm \infty \) when \(\xi \gtrless (\frac{\alpha}{\beta k}c^{1-\beta}(c-1)^{\alpha -1})^{ \frac{1}{\beta}}\) . The corner case of \(\xi = (\frac{\alpha}{\beta k}c^{1-\beta}(c-1)^{\alpha -1})^{ \frac{1}{\beta}}\) can be analysed by a simple application of L’Hôpital’s rule.
We now derive the shapes of \(f\) by first focusing on the case \(\beta \neq 1\) . Direct differentiation gives
We first investigate the convexity/concavity of \(f\) by studying the sign of \(f''(x)\) , which is determined by that of \(h_{2}(x^{-\frac{1}{\beta}})\) . Check that
for all \(z>0\) since \(\alpha \leq \beta \leq 1\) , and thus \(h_{2}\) is concave. Then there are two possibilities.
If \(\xi \leq (\frac{\alpha}{\beta k}c^{1-\beta}(c-1)^{\alpha -1})^{ \frac{1}{\beta}}\) , then \(h_{2}(0)\leq 0\) and
where we have used the facts that \(\alpha <1\) and \(\frac{\alpha}{\beta}-\beta +\alpha -1<\alpha -\beta \leq 0\) . Since \(h_{2}\) is concave, we must have \(h_{2}(z)\leq 0\) for all \(z>0\) . Hence \(f_{2}''(x)\leq 0\) for all \(x\geq 0\) , i.e., \(f\) is a concave function.
If instead \(\xi >(\frac{\alpha}{\beta k}c^{1-\beta}(c-1)^{\alpha -1})^{ \frac{1}{\beta}}\) , then \(h_{2}(0)> 0\) and
so that \(h_{2}(z)\to -\infty \) as \(z\to \infty \) . As \(h_{2}\) is concave, we must have \(z \mapsto h_{2}(z)\) down-crossing zero exactly once on \((0,\infty )\) . Hence \(f_{2}''(x)\propto h_{2}(x^{-\frac{1}{\beta}})\) has exactly one sign change from negative to positive, i.e., \(f\) is concave for small \(x\) and convex for large \(x\) with a unique inflection point \(\tilde{x}\) which is given by the solution to \(h_{2}(x^{-\frac{1}{\beta}})=0\) . This corresponds to ( A.2 ).
Now we look at the monotonicity of \(f\) via the sign of \(f'(x)\) which in turn is determined by that of \(h_{1}(x^{-\frac{1}{\beta}})\) . Check that
Observe that \(h_{1}'\) is increasing and thus \(h_{1}\) is convex. There are two cases.
If \(\xi \leq (\frac{\alpha}{\beta k}c^{1-\beta}(c-1)^{\alpha -1})^{ \frac{1}{\beta}}\) , then \(h_{1}(0)\geq 0\) and
As \(h_{1}\) is convex, we must have \(h_{1}(z)\geq 0\) for all \(z>0\) . Hence \(f'(x)\geq 0\) for all \(x\geq 0\) , i.e., \(f\) is an increasing function. Together with the consideration of \(f''\) in this parameter regime, \(f\) is an increasing concave function.
If \(\xi >(\frac{\alpha}{\beta k}c^{1-\beta}(c-1)^{\alpha -1})^{ \frac{1}{\beta}}\) , then we have \(h_{1}(0)<0\) instead. We also have
and hence \(h_{1}(z)\to \infty \) as \(z\to \infty \) . Since \(h_{1}\) is convex, it must up-cross zero exactly once on \((0,\infty )\) . Therefore \(f'(x)\propto h_{1}(x^{-\frac{1}{\beta}})\) changes sign exactly once, from which we conclude that \(f\) is first increasing and then decreasing with a unique turning point \(x_{2}^{*}\) . Moreover, \(x_{2}^{*}\) is the solution to \(h_{1}(x^{-\frac{1}{\beta}})=0\) , which is equivalent to ( A.1 ). Taking the behaviour of \(f''\) into consideration, we conclude that \(f\) is increasing concave on \([0,x_{2}^{*}]\) , decreasing concave on \([x_{2}^{*},\tilde{x}]\) , and decreasing convex on \([\tilde{x},\infty )\) .
The case \(\beta =1\) can be handled similarly. The key difference is that ( A.4 ) no longer holds when \(\beta =1\) , but we have instead
which can be either positive or negative. If \(\xi >\frac{(c-1)^{\alpha -1}}{k}\) , we have \(h_{1}(z)\to -\infty \) as \(z\to \infty \) . We can then deduce that \(f'(x)\) is negative for all \(x\) and thus \(f\) is decreasing. □
If \(\xi :=\frac{\lambda}{\gamma}>(\frac{\alpha}{\beta k}c^{1-\beta}(c-1)^{ \alpha -1})^{\frac{1}{\beta}}\) , then \(f(x)<0\) for all \(x\) , where \(f\) is defined in ( 4.5 ).
The result follows directly from the definition of \(f\) that
With the help of Lemmas A.1 and A.2 , we now prove Propositions 4.7 , 4.8 and 5.1 .
Proof of Proposition 4.7
From the discussion in Sect. 3 , we have to identify the smallest concave majorant \(\bar{g}_{2}(\,\cdot \,)\) of the function
where \(V_{1}\) is the value function of the exit problem given in Lemma 4.3 . Since \(c>1\) and we assume that \(R> 0\) , \(\Psi \geq 0\) and \(\gamma \leq 1\leq \lambda \) , we have \(c(\frac{\lambda \theta ^{\frac{1}{\beta}}+\Psi +R}{\gamma}) \geq \theta ^{\frac{1}{\beta}}\) , and hence the first regime in ( 4.3 ) will always apply when evaluating \(V_{1}(\theta ^{\frac{1}{\beta}}; \lambda \theta ^{ \frac{1}{\beta}} + \Psi + R)\) , i.e.
where \(f\) is defined in ( 4.5 ). The shape of \(f\) under different parameter combinations is given by Lemma A.1 , and thus we have the following cases.
When \(\xi :=\frac{\lambda}{\gamma}\leq (\frac{\alpha}{\beta k}c^{1- \beta}(c-1)^{\alpha -1})^{\frac{1}{\beta}}\) , \(f\) is increasing concave and we have \(\lim _{x\to \infty}f(x)= +\infty \) . These properties are inherited by \(v_{1}\) . Furthermore,
Thus \(g_{2}\) is constructed by truncating an increasing concave function from below at \(-kR^{\alpha}\) . The smallest concave majorant of \(g_{2}\) is formed by drawing a tangent line passing through \((0,-kR^{\alpha})\) which touches \(v_{1}\) at some \(\theta ^{*}_{1}\) ; see Fig. 1 (a) above. The exact form of the smallest concave majorant is
which is equivalent to ( 4.6 ) upon observing that \(V_{2}(p)=\bar{g}_{2}(p^{\beta})\) .
The optimal strategy is to sell the asset when its transformed price \(\Theta _{t}\) first reaches \(\theta _{1}^{*}\) or above. The corresponding threshold in the original price scale is given by \(p_{1}^{*}:=s^{-1}( \theta _{1}^{*})=(\theta _{1}^{*})^{1/\beta}\) . Since \(\theta _{1}^{*}\) is the point of contact of the tangent line to \(v_{1}\) which passes through \((0,-kR^{\alpha})\) , \(\theta _{1}^{*}\) should solve
Furthermore, we can deduce from a graphical inspection that the solution to ( A.6 ) is a down-crossing. For the case of large proportional transaction costs where we have \(\xi =\frac{\lambda}{\gamma}> (\frac{\alpha}{\beta k}c^{1-\beta}(c-1)^{\alpha -1})^{ \frac{1}{\beta}}\) , the straight line passing \((0,-kR^{\alpha})\) can touch \(v_{1}\) at two distinct locations. A simple geometric inspection tells us that the required root is the smaller one. Using the representation of \(v_{1}(\theta )\) in ( A.5 ), ( A.6 ) can be rewritten as
A further substitution of \(x=(\frac{\gamma}{R+\Psi})^{\beta }\theta \) leads to
Then \(p_{1}^{*}=(\theta _{1}^{*})^{1/\beta}=\frac{R+\Psi}{\gamma}(x_{1}^{*})^{1/ \beta}\) , where \(x_{1}^{*}\) is defined as the solution to ( A.7 ) which is equivalent to ( 4.8 ).
When \(\xi =\frac{\lambda}{\gamma}>(\frac{\alpha}{\beta k}c^{1-\beta}(c-1)^{ \alpha -1})^{\frac{1}{\beta}}\) , Lemma A.1 implies that \(v_{1}\) is first concave increasing, reaching a global maximum at some \(\theta _{2}^{*}\) , then concave decreasing and finally convex decreasing with \(\lim _{\theta \to \infty}v_{1}(\theta )= -\infty \) . There are two further possibilities.
If \(v_{1}(\theta _{2}^{*})> -kR^{\alpha}\) , there must exist \(0\leq \hat{\theta}_{1}<\hat{\theta}_{2}\) such that \(g_{2}(\theta )=-kR^{\alpha}\) on \([0,\hat{\theta}_{1}]\cup [\hat{\theta}_{2},\infty )\) and \(g_{2}(\theta )=v_{1}(\theta )\) on \([\hat{\theta}_{1},\hat{\theta}_{2}]\) . The smallest concave majorant of \(g_{2}(\theta )\) is formed by a chord passing through \((0,-kR^{\alpha})\) which touches \(v_{1}\) at some \(\theta _{1}^{*}<\theta _{2}^{*}\) on \(\{\theta < \theta _{1}^{*}\}\) , a horizontal line at level \(g(\theta _{2}^{*})\) on \(\{\theta >\theta _{2}^{*}\}\) , and the function \(g_{2}\) itself on \(\{\theta _{1}^{*}\leq \theta \leq \theta _{2}^{*}\}\) ; see Fig. 1 (b) above. The smallest concave majorant is
This gives the form of the value function in ( 4.7 ).
The optimal strategy is to purchase the asset when its transformed price \(\Theta _{t}\) first enters the interval \([\theta _{1}^{*},\theta _{2}^{*}]\) . The boundary of the purchase regions in the original scale can be recovered via \(p_{i}^{*}=(\theta _{i}^{*})^{1/\beta}\) for \(i=1,2\) . Given that \(\theta _{2}^{*}\) is the maximiser of \(v_{2}(\theta )\) , using the representation of ( A.5 ), \(\theta _{2}^{*}\) should then solve \(f'((\frac{\gamma}{R+\Psi})^{\beta}\theta )=0\) . Using ( A.3 ), \(x_{2}^{*}:=(\frac{\gamma}{R+\Psi})^{\beta }\theta _{2}^{*}\) is a solution to
Then \(p_{2}^{*}=(\theta _{2}^{*})^{1/\beta}=\frac{R+\Psi}{\gamma}(x_{2}^{*})^{1/ \beta}\) , where \(x_{2}^{*}\) is given by the solution to ( 4.9 ).
If \(v_{1}(\theta _{2}^{*})\leq -kR^{\alpha}\) instead, then \(v_{1}(\theta )\leq -kR^{\alpha}\) for all \(\theta \) . Thus \(g_{2}( \theta )=-kR^{\alpha}\) which is a flat horizontal line, and it is also the smallest concave majorant of itself, i.e., \(\bar{g}_{2}(\theta )=-kR^{\alpha}\) . The optimal strategy is not to trade at all at any price level so that the utility received is always \(U(-R)=-kR^{\alpha}\) ; see Fig. 1 (c) above. The “never purchase” case arises if and only if \(v_{1}(\theta _{2}^{*})\leq -kR^{\alpha}\) or, equivalently,
where \(x_{2}^{*}\) is the maximiser of \(f\) introduced in Lemma A.1 and is independent of \(\Psi \) and \(R\) . Using the fact that \(f(0)=-k\) and Lemma A.2 , we deduce that \(-k< f(x^{*})< 0\) and hence there must exist \(C:=(-\frac{k}{f(x^{*})})^{1/\alpha}-1>0\) such that \((1+\frac{\Psi}{R} )^{\alpha}f(x^{*}) \leq -k\) if and only if \(\Psi /R\geq C\) . □
Proof of Proposition 4.8
Omitted since it is largely the same as the proof of Proposition 4.7 . □
Proof of Proposition 5.1
From the proof of Proposition 4.7 , the required solution to ( 4.8 ) is a down-crossing. Then given that the left-hand side of ( 4.8 ) is increasing in \(\Psi \) (when evaluated at \(x=x_{1}^{*}\) ), we can deduce that \(x_{1}^{*}\) and in turn \(p_{1}^{*}\) are both increasing in \(\Psi \) .
To show that \(p_{1}^{*}\) is decreasing in \(\gamma \) , consider the substitution \(q=\frac{x^{1/\beta}}{\gamma}\) . Then \(p_{1}^{*}= (R+\Psi )q_{1}^{*}\) , where \(q_{1}^{*}\) is the solution to
where the right-hand side of ( A.8 ) is decreasing in \(\gamma \) . Hence \(q_{1}^{*}\) and in turn \(p_{1}^{*}\) are both decreasing in \(\gamma \) .
The monotonicity of \(p_{2}^{*}\) with respect to \(\Psi \) is trivial because ( 4.9 ) which defines \(x_{2}^{*}\) does not depend on \(\Psi \) . To check the monotonicity with respect to \(\gamma \) , consider the substitution \(q=\frac{x^{1/\beta}}{\gamma}\) again so that \(p_{2}^{*}=(R+\Psi )q_{2}^{*}\) , where \(q_{2}^{*}\) is defined as the solution to
From the proof of Lemma A.1 , the solution to \(h_{1}(x^{-\frac{1}{\beta}})=0\) is a down-crossing. This property is inherited by ( A.9 ). Moreover, the left-hand side of ( A.9 ) is increasing in \(\gamma \) . Hence \(q_{2}^{*}\) and in turn \(p_{2}^{*}\) is increasing in \(\gamma \) .
Similarly, consider the substitution \(y=\lambda ^{\beta }x\) . Then \(p_{2}^{*}=\frac{R+\Psi}{\lambda \gamma }(y_{2}^{*})^{1/\beta}\) , where \(y_{2}^{*}\) is defined as the solution to
The left-hand side of ( A.10 ) is decreasing in \(\lambda \) , and hence \(y_{2}^{*}\) is decreasing in \(\lambda \) . Therefore \(p_{2}^{*}\) is decreasing in \(\lambda \) as well. □
1.2 A.2 Proof of Proposition 6.3
We first show that the solution method in Sect. 3.1 can be extended to the problem with utility-discounting. A general exposition can be found in Dayanik and Karatzas [ 6 ], but we outline the key ideas under our specific model to introduce some notation to be used.
Let \(\mathcal{A}:=\frac{\sigma ^{2}}{2}\frac{d^{2}}{dp^{2}}+\mu \frac{d}{dp}\) be the infinitesimal generator of \(P\) . The second order ordinary differential equation \(\mathcal{A}u(p)=\delta u(p)\) with \(\delta >0\) admits \(r_{1}(p):=p^{\omega _{1}}\) and \(r_{2}(p):=p^{\omega _{2}}\) as two linearly independent solutions, where \(\omega _{1}<0<\omega _{2}\) are the distinct real roots to the quadratic equation
Moreover, for \(p\in [a,b]\subseteq \mathcal{J}\) , the function φ ( p ; a , b ) : = E [ e − δ τ a 1 { τ a < τ b } | P 0 = p ] is the solution to \(\mathcal{A}\varphi =\delta \varphi \) with boundary conditions \(\varphi (a;a,b)=1\) and \(\varphi (b;a,b)=0\) , where \(\tau _{\ell}:=\inf \{t\geq 0: P_{t}=\ell \}\) (likewise, ϑ ( p ; a , b ) : = E [ e − δ τ b 1 { τ b < τ a } | P 0 = p ] has similar properties). From this, we obtain
Now consider a discounted optimal stopping problem of the form
As before, it is sufficient to look for an optimal stopping time which is of the form \(\tau _{a,b}:=\tau _{a}\wedge \tau _{b}\) . Then
where \(s(x):=r_{2}(x)/r_{1}(x)=x^{\omega _{2}-\omega _{1}}\) , \(\theta :=s(p)\) and
The optimal stopping rule can be deduced by maximising the above with respect to \(a\) and \(b\) . Upon replacing the dummy variables via \(a'=s(a)\) and \(b'=s(b)\) , we have
The supremum in the second to last term can be characterised by the smallest concave majorant \(\bar{\phi}\) of the scaled payoff function \(\phi =\frac{G}{r_{1}}\circ s^{-1}\) , and the value function in the original coordinates is given by \(V(p)=v(s(p))=r_{1}(p)\bar{\phi}(s(p))\) .
Proof of Proposition 6.3
Similarly to the baseline problem, ( 6.2 ) which features utility-discounting can be solved by decomposing the problem into the sub-problems of exit and entry. The discounted exit problem is
where \(H\geq 0\) is some given constant. The scaled payoff function is given by
Note that \(g_{2}(0)=g_{2}((\frac{H}{\gamma})^{\omega _{2}-\omega _{1}} )=0\) and \(g_{2}(\theta )< 0\) on \(\{0<\theta < (\frac{H}{\gamma})^{\omega _{2}-\omega _{1}} \}\) . Furthermore, on \(\{\theta > (\frac{H}{\gamma})^{\omega _{2}-\omega _{1}} \}\) , we have
We now show that on \([0,1]\) , the quadratic function \(z \mapsto h(z)\) is strictly negative, which in turn will imply that \(g_{1}\) is a strictly increasing and concave function for \(\theta \geq (\frac{H}{\gamma})^{\omega _{2}-\omega _{1}}\) . Note that \(\omega _{1}+\omega _{2}=1-\frac{2\mu}{\sigma ^{2}}=\beta \) by considering the sum of the roots of the quadratic equation ( A.11 ). Then we have \(h(0)=-\alpha (1-\alpha )<0\) and
since \(\omega _{1}<0\) and \(0<\alpha <\beta =\omega _{1}+\omega _{2}<\omega _{2}\) . If \(\beta \geq 1\) , then
and \(h\) must be decreasing and in turn negative on \([0,1]\) . If \(\beta < 1\) and \(\alpha \geq -\frac{2\omega _{1}\omega _{2}}{1-\beta}\) , then
and \(h\) must be increasing and thus negative on \([0,1]\) . Finally, if \(\beta <1\) and \(\alpha < -\frac{2\omega _{1}\omega _{2}}{1-\beta}\) , we can compute the discriminant of the quadratic function \(h\) as
and hence \(h(z)<0\) for all \(z\) .
Now, the smallest concave majorant of \(g_{1}\) can be formed by drawing a straight line from \((0,0)\) which touches \(g_{1}\) at some \(\theta ^{*}>(\frac{H}{\gamma})^{\omega _{2}-\omega _{1}}\) . The point of contact is given by the unique \(\theta ^{*}\) satisfying \(\frac{g_{1}(\theta ^{*})}{\theta ^{*}}=g_{1}'(\theta ^{*})\) . The required \(\theta ^{*}\) is thus given by the solution to the equation
which admits the explicit solution
The smallest concave majorant of \(g_{1}\) is then
The value function of the exit problem is thus
From the form of the value function, the optimal exit strategy is to sell the asset whenever its price level is at or above \(p^{*}=s^{-1}(\theta ^{*})= \frac{\omega _{2} H}{\gamma (\omega _{2}-\alpha )}\) .
Now we look at the entry problem. Unlike the problem without discounting whose objective function is ( 2.2 ), the strategy \(\tau \equiv \infty \) now yields a value of zero rather than \(U(-R)\) under utility-discounting. Hence the payoff function for the entry problem is \(\max \left \{V_{1}(P_{\tau};\lambda P_{\tau}+\Psi +R),0\right \}\) rather than \(\max \left \{V_{1}(P_{\tau};\lambda P_{\tau}+\Psi +R),U(-R)\right \}\) . The entry problem is thus
It is also clear that \(V_{1}\) is nonnegative. Then the scaled payoff function of the entry problem is
The last equality holds because
due to the facts that \(R>0\) , \(\Psi \geq 0\) , \(\omega _{1}<\omega _{2}\) and \(\gamma \leq 1\leq \lambda \) , and thus the linear regime of \(\bar{g}_{1}\) always applies when evaluating \(\bar{g_{1}}(\theta ;\lambda \theta ^{ \frac{1}{\omega _{2}-\omega _{1}}}+\Psi +R )\) . It remains to identify the smallest concave majorant \(\bar{g}_{2}\) of \(g_{2}\) . But by similar (and indeed less tedious) calculations as for the exit problem, one can verify that \(\theta \mapsto (\lambda \theta ^{ \frac{1}{\omega _{2}-\omega _{1}}}+ \Psi + R)^{\alpha -\omega _{2}} \theta \) is an increasing concave function for all \(\theta \geq 0\) under the condition \(\alpha <\beta \) . We hence must have \(g_{2}=\bar{g}_{2}\) and the strategy \(\tau \equiv 0\) is optimal. □
Rights and permissions
Open Access This article is licensed under a Creative Commons Attribution 4.0 International License, which permits use, sharing, adaptation, distribution and reproduction in any medium or format, as long as you give appropriate credit to the original author(s) and the source, provide a link to the Creative Commons licence, and indicate if changes were made. The images or other third party material in this article are included in the article’s Creative Commons licence, unless indicated otherwise in a credit line to the material. If material is not included in the article’s Creative Commons licence and your intended use is not permitted by statutory regulation or exceeds the permitted use, you will need to obtain permission directly from the copyright holder. To view a copy of this licence, visit http://creativecommons.org/licenses/by/4.0/ .
Reprints and permissions
About this article
Tse, A.S.L., Zheng, H. Speculative trading, prospect theory and transaction costs. Finance Stoch 27 , 49–96 (2023). https://doi.org/10.1007/s00780-022-00494-7
Download citation
Received : 13 October 2021
Accepted : 05 October 2022
Published : 15 December 2022
Issue Date : January 2023
DOI : https://doi.org/10.1007/s00780-022-00494-7
Share this article
Anyone you share the following link with will be able to read this content:
Sorry, a shareable link is not currently available for this article.
Provided by the Springer Nature SharedIt content-sharing initiative
- Sequential optimal stopping
- S-shaped utility
- Transaction costs
- Entry-and-exit strategies
Mathematics Subject Classification (2020)
Jel classification.
- Find a journal
- Publish with us
- Track your research
You are using an outdated browser. Upgrade your browser today or install Google Chrome Frame to better experience this site.
September 9, 2014 | News
What Does it Really Cost to Buy and Sell Bitcoins?

The total cost to buy and sell bitcoins at an exchange involves more than you might think. It depends not only on trades fees, but also on deposit and withdrawal fees, conversion fees, and slippage from spread and depth. This can make it difficult to know what your true transaction cost is, especially since the effect of spread and depth is a hidden cost.
Below we discuss a way to measure the total cost, which we call the “Complete Cost Index” (CCI). The CCI is a tool that can help you understand your total transaction cost so your assessment isn’t skewed by trade fees alone, which is what most exchanges will try to sell you on. We calculate the CCI for a range of sites to see how Kraken compares to the competition. Kraken does pretty well!
The Complete Cost Index
The Complete Cost Index measures the overall cost efficiency of an exchange by calculating the total round-trip transaction cost starting from your bank to the exchange and back again. Results are expressed as a percentage representing the amount of money remaining after the round-trip transaction.
The calculation starts with a hypothetical deposit amount and any deposit fees charged by the exchange are subtracted. Next, any currency conversion fees are subtracted (say if you deposit euros but have to convert to dollars to trade). Bitcoins are then bought with a market order, and the buy price for the order is calculated using the actual order book. The bitcoins are then sold with a market order again using the order book to determine the sell price.
Bitcoins are bought and sold at market because this provides a way to measure spread and depth. Spread is the difference in price between the best buy and sell offers in the order book. A larger spread means you will pay more to buy bitcoins and receive less to sell bitcoins , creating a hidden transaction cost. Depth is how your price to buy or sell depends on the volume of your order. Weaker depth creates another hidden cost because you pay more for larger buy orders and receive less for larger sell orders.
Finally, fees for withdrawing back to the bank are calculated by subtracting the withdrawal fee and any conversion fees. The amount left in your bank account at the end is then divided by the starting amount, and the percentage left is the CCI. Thus, the higher the number, the lower the cost.
The results
In Figure 1 below, the CCI is calculated for a range of euro deposit amounts at exchanges that support SEPA deposits and withdrawals. As you can see from the chart, Kraken is the lowest in total cost of the group for transactions up to about €25,000.

- The CCI for Kraken vs other exchanges supporting SEPA. Higher number is better (lower cost). Solid lines indicate data at the time the chart was printed, and shading indicates max/min from previous 24 hours. X-axis (volume) is logarithmic. Chart printed on Sept 7th, 2014.
Why is Kraken’s total cost so low?
Because we have reasonable trade fees, very low deposit and withdrawal fees, no conversion fee (since you can trade natively in euros), a tight spread, and solid depth. Compared to BTC-e, for example, Kraken is much lower cost, because BTC-e has weaker depth in the euro market and higher SEPA fees (1% SEPA deposit fee and 1% SEPA withdrawal fee).
The CCI for direct sale sites
Direct sale sites are a convenient way to buy small amounts of bitcoin, but you pay for the convenience. Direct sale sites can seem like they don’t have fees, because they only list buy and sell prices, but the hidden fees are exposed by looking at the CCI.
The CCI for direct sale sites is still a measure of the total cost to do a round trip from your bank and back, but the cost in this case is just the spread between buy and sell prices at the site. In Figure 2 below, the CCI for Kraken is compared to the CCI for direct sale sites that support SEPA. As you can see from the chart, the cost to buy and sell bitcoins at Kraken is much lower compared to the direct sale sites.

- The CCI for Kraken vs direct sale sites supporting SEPA. Higher number is better (lower cost). Solid lines indicate data at the time the chart was printed, and shading indicates max/min from previous 24 hours. X-axis (volume) is logarithmic. Chart printed on Sept 7th, 2014.
Limitations of the CCI
The CCI is a measure of all the major costs to trade bitcoin, but depending on how you trade, you might not have all of these costs. So the CCI should be considered in light of your personal trading style. For example, if you deposit and withdraw from an exchange in bitcoin, then you won’t have bank fees. The fees to deposit or withdraw bitcoins weren’t included in this study because they are negligible at Kraken and most other exchanges. Or, if you use limit orders, you can mitigate the effects of spread and depth to some extent, though it’s generally going to be harder to get limit orders filled at an exchange with a larger spread and weaker depth.
Bitcoin exchanges included in the CCI
Kraken charges €0.09 for SEPA withdrawals, nothing for deposits. The order book is true bitcoin-euro trading, so there are no conversion fees involved. The trade fee schedule is listed here and runs from 0.35% to 0.1%.
bitstamp.net
BitStamp charges €0.90 for SEPA withdrawals, nothing for deposits. The order book only offers bitcoin-dollar trading so conversion fees apply on deposits and withdrawals (the conversion spread can be queried by API). The trade fee schedule is listed on the site and runs from 0.50% to 0.20%. Bitstamp has the strongest depth of the group, so it starts to take the lead in the CCI for volumes above approximately €25,000.
BTC-e charges 1% for both SEPA deposits and withdrawals. The trade fee schedule is a flat 0.20%. BTC-e has a €2,000 deposit limit, so that’s why the chart starts at €2,000.
ANX charges €0.90 for SEPA withdrawals, nothing for deposits. The order book is fundamentally bitcoin-dollar with the conversion fees internalized. This means that you can trade bitcoin-euro, but the spread is pretty large due to the internalized conversion fee. The trade fee schedule is maker/taker with a flat 0.60% taker fee (market orders are taker).
ItBit charges €3.64 for SEPA deposits and €8.03 for SEPA withdrawals. The book is true bitcoin-euro so there are no conversion fees. The fee schedule is maker/taker and the taker fee runs from 0.50% to 0.35% (market orders are taker).
Bitcoin direct sale sites included in the CCI
safello.com
Safello only posts a flat buy and sell price, hence the flat line. The CCI is just a measure of the spread between their buy and sell prices.
bitcoinnordic.com
BitcoinNordic only posts a flat buy and sell price, hence the flat line. The CCI is just a measure of the spread between their buy and sell prices.
Bitonic price quotes depend on the volume you want to buy or sell, so the CCI is a curve. They don’t publish information on how their prices depend on volume, but the CCI can easily be constructed by querying the buy and sell prices at different volumes. The CCI is just a measure of the spread between their buy and sell prices at different volumes. Bitonic has a limit of €10,000, so that’s why the line ends and €10,000.
To the best of our knowledge, the cost information given here is correct at the time of publication.
Share this:
Related posts.

Kraken secures Virtual Asset Service Provider (VASP) registration in Ireland

Trading for Beam (BEAM) starts April 11 – deposit now

Earn up to 28% in yearly rewards* when you stake your DYM

Bitcoin at 15: the relentless resilience of peer-to-peer electronic cash
These materials are for general information purposes only and are not investment advice or a recommendation or solicitation to buy, sell, stake or hold any cryptoasset or to engage in any specific trading strategy. Kraken will not undertake efforts to increase the value of any cryptoasset that you buy. Some crypto products and markets are unregulated, and you may not be protected by government compensation and/or regulatory protection schemes. The unpredictable nature of the cryptoasset markets can lead to loss of funds. Tax may be payable on any return and/or on any increase in the value of your cryptoassets and you should seek independent advice on your taxation position.
Discover more from Kraken Blog
Subscribe now to keep reading and get access to the full archive.
Continue reading
- Daily Notes
- Pivot Point
- Market Profile
- Gann Square of Nine
- Day Trader Millionaire
- All Investment Calculators
- Economic Events
- Round Trip Transaction Costs
Definition of 'Round Trip Transaction Costs'
Do you have a trading or investing definition for our dictionary? Click the Create Definition link to add your own definition. You will earn 150 bonus reputation points for each definition that is accepted.
Is this definition wrong? Let us know by posting to the forum and we will correct it.
Why Do Individual Flights Sometimes Cost More On Their Own Than As Part Of A Round Trip?
- Not all airlines inflate one-way ticket prices; some like JetBlue and Southwest offer transparent pricing by summing up two one-way fares for the round-trip cost.
- Pricing discrepancy between one-way and round-trip fares is particularly evident in premium cabins, driven by the purchasing habits of business travelers who prioritize specific travel dates and times.
- Passengers can explore round-trip options, even if they don't use the return trip, as it may potentially save them from exorbitant one-way fares. Evaluating the value of redemptions against cash fares is also crucial for those using points and miles.
Airfare pricing involves a complex interplay of various factors that can confound travelers seeking consistency and transparency. The common notion that one-way tickets tend to be pricier than round-trip tickets isn't universally true, but there are valid reasons behind this perception. A closer look at the dynamics of airline pricing and passenger behaviors sheds light on this phenomenon.
Not always pricier
To begin with, not all airlines necessarily adhere to the practice of inflating one-way ticket prices. Some carriers, such as JetBlue and Southwest, often offer fairly transparent pricing by simply summing up two one-way fares to create the round-trip cost. Moreover, routes with frequent daily flights from a single airline can exhibit similar pricing symmetry due to increased capacity and a more consistent pricing structure.
Nevertheless, there are instances where the reverse occurs, where one-way tickets appear disproportionately costly compared to round-trip fares.
Driven by business travel
This discrepancy in pricing is particularly evident in premium cabins , attributed to the purchasing habits of business travelers. These passengers often prioritize specific travel dates and times, accommodating their rigid schedules without much sensitivity to cost. Such travelers, compelled by work-related commitments, are more likely to book one-way tickets, often covered by their companies. Although non-business travelers might also seek one-way options for emergencies, their urgency mitigates their price sensitivity.
In practice, actual pricing variations vary based on numerous factors including airline, route, and travel date, but the pronounced price difference between round trip and one-way fares is more observable on long international routes as opposed to on domestic flights. Premium cabins tend to accentuate this pricing differential, although it also occurs in economy class.
Alternate booking methods
To navigate this pricing structure, one strategy for travelers to consider is to explore round-trip options, even if they do not necessarily to utilize the return trip. While this will not always be cheaper than a one-way fare, it is worth looking into. This can lead to cost savings by potentially avoiding exorbitant one-way fares.
This pricing asymmetry presents another strategic challenge for passengers using points and miles for their flights. Evaluating the value of redemptions against cash fares is always crucial. Dividing the flight's cost by the miles or points required provides a simple metric, although there are diverse perspectives in the travel community about calculating this value accurately.
In any event, the rationale behind one-way tickets being consistently pricier than round-trip fares is deeply rooted in the dynamics of airline business and passenger behavior. Business travelers, who frequently require one-way bookings, form a market segment willing to pay for convenience.
Airlines, catering to this demand, capitalize on this by adjusting pricing structures. On the flip side, travelers seeking the flexibility of one-way travel bear the brunt of elevated fares due to the potential revenue loss airlines might incur if return flights aren't utilized. This complex interplay of factors shapes the airfare landscape, prompting travelers to carefully consider their options to optimize both cost and convenience.
What other strategies can passengers who only need a one-way fare utilize to save money? Let us know in the comments below.

IMAGES
VIDEO
COMMENTS
Round trip transaction costs refer to all the costs incurred in a securities or other financial transaction. Round trip transaction costs include commissions , exchange and other fees, bid/ask ...
Definition: Round-trip transactions refer to a series of transactions where a company sells an asset and then repurchases the same or similar asset, often at a similar price and within a short time frame. These transactions can artificially inflate a company's revenue and trading volume, creating a misleading impression of its financial activity and health.
The actual round-trip transaction costs estimates by Aitken and Frino (1996) and Comerton-Forde et al (2005) Aitken and Frino (1996) analysed the magnitude and determinants of execution costs associated with institutional trades on the ASX. In terms of the transaction costs estimate, they used data that extend the period from 1 April 1991 to 30 ...
Moreover, being aware of round trip transaction costs can also help you evaluate the efficiency of your investment strategy. It allows you to compare the costs associated with different investments or trading strategies and choose the most cost-effective approach. In Conclusion. Round trip transaction costs are a crucial aspect of investing.
By Chron Contributor Updated June 17, 2021. The accounting slang term "round tripping" refers to a series of transactions between companies that bolster the revenue of the companies involved but ...
Your round-trip transaction costs amount to: $4;125:00 $4;075:00 = $50 Problem 2.1. The bid-ask spread on a share of stock is $100-$102. A 5% commission is paid for either buying or selling. Calculate the round-trip transaction cost. (a)$14.10 (b)$12.10 (c)$6.10 (d)$4.10 (e)None of the above. Instructor: Milica Cudina
Round Trip Transaction Examples. Examples of the round trip transaction include: Example 1: Exchange fees. Example 2: Commissions. Example 3: Market impact costs. Example 4: Bid or Ask spreads. Example 5: Occasional taxes. Here is a comprehensive article about the round trip transaction.
Round trip transaction costs allude to all the costs incurred in a securities or other financial transaction. Round trip transaction costs include commissions, exchange fees, bid/ask spreads, market impact costs, and occasionally taxes. Since such transaction costs can dissolve a substantial portion of trading profits, traders and investors ...
Barclays Liquidity Cost Scores (LCS®): A Brief Introduction. In 2009, Barclays launched a bond-level liquidity measure, Liquidity Cost Score (LCS), which focuses on cost of trading, an important, and quantifiable, dimension of liquidity. More specifically, LCS measures the cost of an immediate, round-trip transaction of a typical institutional ...
Round-trip transactions costs. Costs of completing a transaction, including commissions, market impact costs, and taxes. Most Popular Terms: Earnings per share (EPS) Beta. Market capitalization ...
What is your round-trip transaction cost? Remember th t the terminology bid and ask is formulated from the market makers per- spective. Theref re, the price at which you can buy is called the ask price. ... Your round-tri transaction costs amount to: $4,075100 $50 2 $20 Problem 2.1. e bid-ask spread on a share of stock is $100-$102. A 5% ...
My round-trip cost - of buying and selling this stock - will then be about 5.5%. Now I figure my expected excess return on an average stock is 4.17% after transaction costs. Why?
An Example of Transaction Costs: Let's say you're an investor looking to buy 100 shares of a company's stock. Each share is valued at $50. Here's a breakdown of the transaction costs involved: Brokerage fee: $10 flat fee per trade. Commission: 1% of the trade value ($50 x 100 x 1% = $50) Bid-Ask Spread: $0.20 per share on both the buy ...
Round-tripping, also known as round-trip transactions or Lazy Susans, is defined by The Wall Street Journal as a form of barter that involves a company selling "an unused asset to another company, while at the same time agreeing to buy back the same or similar assets at about the same price." Swapping assets on a round-trip produces no net ...
LQC is a percentage transaction cost estimate for fixed-income securities based on the Bloomberg's proprietary LQA methodology. LQC is a per-issue percentage round-trip transaction cost estimate for an institutional size trade, i.e., a value of 1 equals to 1% cost as percentage of price; therefore, a lower LQC indicates better liquidity.
An investor who purchased at a premium of 64 basis points and sold at a discount of 61 basis points would incur 125 basis points (1.25 percentage points) of round-trip transaction costs. Selling at a steep discount only adds to the initial transaction cost. Again, a larger but stable premium is often preferable to a lesser, volatile one.
A speculative agent with prospect theory preference chooses the optimal time to purchase and then to sell an indivisible risky asset to maximise the expected utility of the round-trip profit net of transaction costs. The optimisation problem is formulated as a sequential optimal stopping problem, and we provide a complete characterisation of the solution. Depending on the preference and market ...
We do, however, provide an estimate of round-trip transaction costs in Table 10. These costs are broadly consistent with the ones provided in Puckett and Yan (2011) but slightly lower than their estimates. This is because we have a longer and more recent data series, when transaction costs have continued to decline. 5. Biased self-attribution
The Complete Cost Index. The Complete Cost Index measures the overall cost efficiency of an exchange by calculating the total round-trip transaction cost starting from your bank to the exchange and back again. Results are expressed as a percentage representing the amount of money remaining after the round-trip transaction.
My round-trip transaction cost would be a gigantic 7.2%. It's rather difficult to justify such a purchase, since the cost would likely exceed the expected return.
Definition of 'Round Trip Transaction Costs'. Round Trip Transaction Costs refer to the total cost that you will incur in the opening and closing of a futures position. These costs will typically include exchange fees, taxes, broker's commission. See also: Round Trip, Per Side Costs. Do you have a trading or investing definition for our ...
Round Trip Transaction Costs. All commissions and other expenses related to trading a security. When an investor buys or sells a security, he/she must usually pay a fee to his/her investment adviser, who may or may not also be the broker, who is also entitled to a fee. Research and other indirect costs informing investment decisions are not ...
Nevertheless, there are instances where the reverse occurs, where one-way tickets appear disproportionately costly compared to round-trip fares. Driven by business travel
Here are some of the top ways Americans may be able to reduce the overall cost of a trip, according to travel experts. 1. Be flexible with trip timing and location. Being flexible with your plans ...