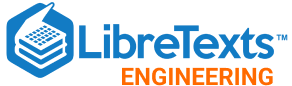
- school Campus Bookshelves
- menu_book Bookshelves
- perm_media Learning Objects
- login Login
- how_to_reg Request Instructor Account
- hub Instructor Commons
- Download Page (PDF)
- Download Full Book (PDF)
- Periodic Table
- Physics Constants
- Scientific Calculator
- Reference & Cite
- Tools expand_more
- Readability
selected template will load here
This action is not available.
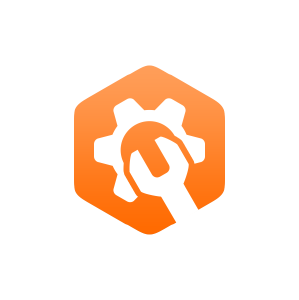

3.4: Trip Generation
- Last updated
- Save as PDF
- Page ID 47326
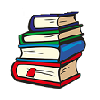
- David Levinson et al.
- Associate Professor (Engineering) via Wikipedia
Trip Generation is the first step in the conventional four-step transportation forecasting process (followed by Destination Choice, Mode Choice, and Route Choice), widely used for forecasting travel demands. It predicts the number of trips originating in or destined for a particular traffic analysis zone.
Every trip has two ends, and we need to know where both of them are. The first part is determining how many trips originate in a zone and the second part is how many trips are destined for a zone. Because land use can be divided into two broad category (residential and non-residential) we have models that are household based and non-household based (e.g. a function of number of jobs or retail activity).
For the residential side of things, trip generation is thought of as a function of the social and economic attributes of households (households and housing units are very similar measures, but sometimes housing units have no households, and sometimes they contain multiple households, clearly housing units are easier to measure, and those are often used instead for models, it is important to be clear which assumption you are using).
At the level of the traffic analysis zone, the language is that of land uses "producing" or attracting trips, where by assumption trips are "produced" by households and "attracted" to non-households. Production and attractions differ from origins and destinations. Trips are produced by households even when they are returning home (that is, when the household is a destination). Again it is important to be clear what assumptions you are using.
People engage in activities, these activities are the "purpose" of the trip. Major activities are home, work, shop, school, eating out, socializing, recreating, and serving passengers (picking up and dropping off). There are numerous other activities that people engage on a less than daily or even weekly basis, such as going to the doctor, banking, etc. Often less frequent categories are dropped and lumped into the catchall "Other".
Every trip has two ends, an origin and a destination. Trips are categorized by purposes , the activity undertaken at a destination location.
Observed trip making from the Twin Cities (2000-2001) Travel Behavior Inventory by Gender
Some observations:
- Men and women behave differently on average, splitting responsibilities within households, and engaging in different activities,
- Most trips are not work trips, though work trips are important because of their peaked nature (and because they tend to be longer in both distance and travel time),
- The vast majority of trips are not people going to (or from) work.
People engage in activities in sequence, and may chain their trips. In the Figure below, the trip-maker is traveling from home to work to shop to eating out and then returning home.

Specifying Models
How do we predict how many trips will be generated by a zone? The number of trips originating from or destined to a purpose in a zone are described by trip rates (a cross-classification by age or demographics is often used) or equations. First, we need to identify what we think the relevant variables are.
The total number of trips leaving or returning to homes in a zone may be described as a function of:
\[T_h = f(housing \text{ }units, household \text{ }size, age, income, accessibility, vehicle \text{ }ownership)\]
Home-End Trips are sometimes functions of:
- Housing Units
- Household Size
- Accessibility
- Vehicle Ownership
- Other Home-Based Elements
At the work-end of work trips, the number of trips generated might be a function as below:
\[T_w=f(jobs(area \text{ }of \text{ } space \text{ } by \text{ } type, occupancy \text{ } rate\]
Work-End Trips are sometimes functions of:
- Area of Workspace
- Occupancy Rate
- Other Job-Related Elements
Similarly shopping trips depend on a number of factors:
\[T_s = f(number \text{ }of \text{ }retail \text{ }workers, type \text{ }of \text{ }retail, area, location, competition)\]
Shop-End Trips are sometimes functions of:
- Number of Retail Workers
- Type of Retail Available
- Area of Retail Available
- Competition
- Other Retail-Related Elements
A forecasting activity conducted by planners or economists, such as one based on the concept of economic base analysis, provides aggregate measures of population and activity growth. Land use forecasting distributes forecast changes in activities across traffic zones.
Estimating Models
Which is more accurate: the data or the average? The problem with averages (or aggregates) is that every individual’s trip-making pattern is different.
To estimate trip generation at the home end, a cross-classification model can be used. This is basically constructing a table where the rows and columns have different attributes, and each cell in the table shows a predicted number of trips, this is generally derived directly from data.
In the example cross-classification model: The dependent variable is trips per person. The independent variables are dwelling type (single or multiple family), household size (1, 2, 3, 4, or 5+ persons per household), and person age.
The figure below shows a typical example of how trips vary by age in both single-family and multi-family residence types.

The figure below shows a moving average.

Non-home-end
The trip generation rates for both “work” and “other” trip ends can be developed using Ordinary Least Squares (OLS) regression (a statistical technique for fitting curves to minimize the sum of squared errors (the difference between predicted and actual value) relating trips to employment by type and population characteristics.
The variables used in estimating trip rates for the work-end are Employment in Offices (\(E_{off}\)), Retail (\(E_{ret}\)), and Other (\(E_{oth}\))
A typical form of the equation can be expressed as:
\[T_{D,k}=a_1E_{off,k}+a_2E_{oth,k}+a_3E_{ret,k}\]
- \(T_{D,k}\) - Person trips attracted per worker in Zone k
- \(E_{off,i}\) - office employment in the ith zone
- \(E_{oth,i}\) - other employment in the ith zone
- \(E_{ret,i}\)- retail employment in the ith zone
- \(a_1,a_2,a_3\) - model coefficients
Normalization
For each trip purpose (e.g. home to work trips), the number of trips originating at home must equal the number of trips destined for work. Two distinct models may give two results. There are several techniques for dealing with this problem. One can either assume one model is correct and adjust the other, or split the difference.
It is necessary to ensure that the total number of trip origins equals the total number of trip destinations, since each trip interchange by definition must have two trip ends.
The rates developed for the home end are assumed to be most accurate,
The basic equation for normalization:
\[T'_{D,j}=T_{D,j} \dfrac{ \displaystyle \sum{i=1}^I T_{O,i}}{\displaystyle \sum{j=1}^J T_{TD,j}}\]
Sample Problems
Planners have estimated the following models for the AM Peak Hour
\(T_{O,i}=1.5*H_i\)
\(T_{D,j}=(1.5*E_{off,j})+(1*E_{oth,j})+(0.5*E_{ret,j})\)
\(T_{O,i}\) = Person Trips Originating in Zone \(i\)
\(T_{D,j}\) = Person Trips Destined for Zone \(j\)
\(H_i\) = Number of Households in Zone \(i\)
You are also given the following data
A. What are the number of person trips originating in and destined for each city?
B. Normalize the number of person trips so that the number of person trip origins = the number of person trip destinations. Assume the model for person trip origins is more accurate.
Solution to Trip Generation Problem Part A
\[T'_{D,j}=T_{D,j} \dfrac{ \displaystyle \sum{i=1}^I T_{O,i}}{\displaystyle \sum{j=1}^J T_{TD,j}}=>T_{D,j} \dfrac{37500}{36750}=T_{D,j}*1.0204\]
Solution to Trip Generation Problem Part B
Modelers have estimated that the number of trips leaving Rivertown (\(T_O\)) is a function of the number of households (H) and the number of jobs (J), and the number of trips arriving in Marcytown (\(T_D\)) is also a function of the number of households and number of jobs.
\(T_O=1H+0.1J;R^2=0.9\)
\(T_D=0.1H+1J;R^2=0.5\)
Assuming all trips originate in Rivertown and are destined for Marcytown and:
Rivertown: 30000 H, 5000 J
Marcytown: 6000 H, 29000 J
Determine the number of trips originating in Rivertown and the number destined for Marcytown according to the model.
Which number of origins or destinations is more accurate? Why?
T_Rivertown =T_O ; T_O= 1(30000) + 0.1(5000) = 30500 trips
T_(MarcyTown)=T_D ; T_D= 0.1(6000) + 1(29000) = 29600 trips
Origins(T_{Rivertown}) because of the goodness of fit measure of the Statistical model (R^2=0.9).
Modelers have estimated that in the AM peak hour, the number of trip origins (T_O) is a function of the number of households (H) and the number of jobs (J), and the number of trip destinations (T_D) is also a function of the number of households and number of jobs.
\(T_O=1.0H+0.1J;R^2=0.9\)
Suburbia: 30000 H, 5000 J
Urbia: 6000 H, 29000 J
1) Determine the number of trips originating in and destined for Suburbia and for Urbia according to the model.
2) Does this result make sense? Normalize the result to improve its accuracy and sensibility?
- \(T_{O,i}\) - Person trips originating in Zone i
- \(T_{D,j}\) - Person Trips destined for Zone j
- \(T_{O,i'}\) - Normalized Person trips originating in Zone i
- \(T_{D,j'}\) - Normalized Person Trips destined for Zone j
- \(T_h\) - Person trips generated at home end (typically morning origins, afternoon destinations)
- \(T_w\) - Person trips generated at work end (typically afternoon origins, morning destinations)
- \(T_s\) - Person trips generated at shop end
- \(H_i\) - Number of Households in Zone i
- \(E_{off,k}\) - office employment in Zone k
- \(E_{ret,k}\) - retail employment in Zone k
- \(E_{oth,k}\) - other employment in Zone k
- \(B_n\) - model coefficients
Abbreviations
- H2W - Home to work
- W2H - Work to home
- W2O - Work to other
- O2W - Other to work
- H2O - Home to other
- O2H - Other to home
- O2O - Other to other
- HBO - Home based other (includes H2O, O2H)
- HBW - Home based work (H2W, W2H)
- NHB - Non-home based (O2W, W2O, O2O)
External Exercises
Use the ADAM software at the STREET website and try Assignment #1 to learn how changes in analysis zone characteristics generate additional trips on the network.
Additional Problems
- the start and end time (to the nearest minute)
- start and end location of each trip,
- primary mode you took (drive alone, car driver with passenger, car passenger, bus, LRT, walk, bike, motorcycle, taxi, Zipcar, other). (use the codes provided)
- purpose (to work, return home, work related business, shopping, family/personal business, school, church, medical/dental, vacation, visit friends or relatives, other social recreational, other) (use the codes provided)
- if you traveled with anyone else, and if so whether they lived in your household or not.
Bonus: Email your professor at the end of everyday with a detailed log of your travel diary. (+5 points on the first exam)
- Are number of destinations always less than origins?
- Pose 5 hypotheses about factors that affect work, non-work trips? How do these factors affect accuracy, and thus normalization?
- What is the acceptable level of error?
- Describe one variable used in trip generation and how it affects the model.
- What is the basic equation for normalization?
- Which of these models (home-end, work-end) are assumed to be more accurate? Why is it important to normalize trip generation models
- What are the different trip purposes/types trip generation?
- Why is it difficult to know who is traveling when?
- What share of trips during peak afternoon peak periods are work to home (>50%, <50%?), why?
- What does ORIO abbreviate?
- What types of employees (ORIO) are more likely to travel from work to home in the evening peak
- What does the trip rate tell us about various parts of the population?
- What does the “T-statistic” value tell us about the trip rate estimation?
- Why might afternoon work to home trips be more or less than morning home to work trips? Why might the percent of trips be different?
- Define frequency.
- Why do individuals > 65 years of age make fewer work to home trips?
- Solve the following problem. You have the following trip generation model:
\[Trips=B_1Off+B_2Ind+B_3Ret\]
And you are given the following coefficients derived from a regression model.
If there are 600 office employees, 300 industrial employees, and 200 retail employees, how many trips are going from work to home?

Model calibration and validation
Past Practices
Current Practice
Additional Information
Trip Based Models
Page categories
Topic Circles
Travel models are typically defined as a set of mathematical formulae and relationships, being used in an attempt to reflect an open system – the real world populated by people who are responding to influences that are constantly changing, do not always make rational decisions, and whose responses to influences affecting travel are not always the same. Since travel models (and travel modelers) cannot be omniscient, there will always be missed information and abstractions resulting in less than perfect models. Travel model calibration can be defined as the approach and methods used to make travel models reasonably reproduce a snapshot of travel in the modeling area. Travel model validation can be defined as the approach and methods used to demonstrate that travel models have reasonable sensitivities and will provide reasonable forecasts of travel based on alternative conditions or assumptions regarding the population or transportation system.
# Background
The Figure below shows the overall travel demand model development and application process. Model calibration and validation take place between model estimation and model application. Once the model is estimated, small adjustments are made until the model accurately replicates observed patterns and behavior. Then the model is validated to a different set of observed data (for example, traffic counts) than was used for model estimation or calibration.
As part of the process, model calibration and validation generally occur in an iterative fashion. Model validation may reveal the need to return to the model estimation or model calibration steps. The application of the model using future year conditions requires that the model forecasts are reasonable and consistent with expectations and also might reveal a need to return to the model estimation or calibration step.

Several definitions are necessary to understand the roles and purposes of travel model calibration and validation:
- Model estimation is the use of statistical analysis techniques and observed data to develop model parameters or coefficients
- Model assertion is the declaration of model forms or parameters without the use of statistical analysis of observed data
- Model calibration is the adjustment of constants and other model parameters in estimated or asserted models in an effort to make the models replicate observed data for a base year or otherwise produce more reasonable results
- Model validation is the application of the calibrated models and comparison of the results against observed data; ideally, the observed data are not the same data used for model estimation or calibration
- Model sensitivity testing is the application of the models and the model set using alternative input data or assumptions to determine if the model results are plausible and reasonable
In the past, model calibration and validation have been used to basically describe the same action. Further, models have been asserted to be "valid" based on achieving a rather arbitrary set of standards, such as obtaining a regional coefficient of determination (R^2^) of 0.85 for the match between modeled and observed traffic volumes. While the use of statistical measures to summarize model results can be very useful, matching a set of arbitrary standards does not guarantee that a model will be useful for travel forecasting. Indeed, a model might be calibrated to closely reproduce observed traffic volumes or transit ridership under base conditions but be so insensitive that it would be useless for analyzing changes in development patterns or the transportation system.
The Travel Model Validation and Reasonableness Checking Manual - Second Edition (revised) , describes improved practices and approaches to travel model calibration and validation. The manual refocuses travel model validation on the sensitivities and reasonableness of travel models as well as their abilities to reproduce observed travel under a base condition.
# Past Practices
Previously the calibration and validation process was focused only on the overall results of the travel model, without detailed checking of interim model steps. However, this approach often leads to the problem of “error propagation”. Error propagation occurs when errors made in each step are compounded and thereby increase the overall modeling errors.
# Current Practice
Due to the nature of travel demand models, it is important that model calibration and validation take place at each step in the travel demand model development process, after estimation and assertion of the model parameters. In best practice modeling, each model component is subjected to a series of checks and reasonableness tests to make sure that each component reasonably reproduces observed travel characteristics. A good way to accomplish this is through a structured, Stepwise Model Calibration/Validation process. The overall model is validated to ensure that each is properly interfaced and that any modeling error is not propagated by chaining the models together. It is very useful to develop a model validation plan at the outset of a model development or update process to guide the model validation and help ensure that necessary validation data are available.
# Additional Information
See the following pages for additional information:
- Validating Input Data
# Input Data
There are two types of input data required for travel demand modeling:
- Socioeconomic data.
- Transportation network data
Since these input data are the basic building blocks along with the travel demand models to forecast travel in a region, the success or failure of the modeling process rest largely on the quality of the input data. Therefore, the checking of input data is extremely important.
Socioeconomic Data
Socioeconomic data includes population, households classified by variables such as number of persons, number of workers, income level, and / or auto ownership, school enrollment, and employment data classified by industry type such as retail, manufacturing, service, etc.
This starts with a simple inspection of the data through the use of thematic mapping. It is usually recommended that checks be performed at the TAZ, district, and regional level. Basic checks include mapping and review of population, households, average household size, proportions of households by income level or auto ownership, employment, and employment by category. Various ratios should also be created and reviewed including persons per household, vehicles per household, workers per household, vehicles per worker, population/employment, and income ratios (zonal average income/regional average income). Anomalies, such as zero population and non-zero households, or very low or non-existent employment in a TAZ surrounded by employment, should be checked. For forecast data, trends in the data and derived statistical measures over time for the region should be reviewed to identify any unusual or extreme changes, and corrections should be made where necessary.
The main sources of socioeconomic data are Census data, American Community Survey (ACS) data, Quarterly Census of Employment and Wages (QCEW) data, and market research listings, such as InfoUSA or Dun & Bradstreet.
Transportation Network Data
Transportation network data represent highway, transit, and other non-motorized mode networks and their attributes.
For the first level of validation testing, color coded plots of the following network attributes should be produced and reviewed:
- Facility type,
- Functional class,
- Number of lanes,
- Posted speed,
- Capacity, and
- Direction of flow.
Other important checks include network connectivity and path testing. This process will identify dangling links, disconnected link segments, incorrectly coded one-way links or ramps, and disconnected nodes. Highway and transit network paths (or skims) are useful for creating time contours between locations of interest. Contour maps can be analyzed to ensure that all centroids with particularly long or short travel times as compared to surrounding centroids are properly connected to the line layer and that all surrounding links are properly coded.
The main sources of transportation network data are Census Bureau Topologically Integrated Geographic Encoding and Referencing (TIGER) files, Regional or local GIS/planning agencies, or commercial distributors.
# Trip Based Models
Model calibration requires household travel survey data to adjust the model to match observed trip generation rates, trip length frequency distributions, aggregate trip movements, and mode shares. Model validation could include some components of calibration if household survey data are available; however, survey data are not required in adjusting the model to match traffic counts.
The following sections describe the common calibration and validation checks for each modeling step.
# Trip Generation Models
Since trip generation models consists of three components: trip production, trip attraction, and trip balancing, calibration and validation checks should be conducted for each of the three components.
Trip Productions
Once the initial trip production model is estimated, the trip productions by purpose are calculated and summarized by zone. The total productions by purpose should be then compared with expanded totals from a household travel survey. Since the household travel survey data is used in the model estimation, it is expected that these two area production totals match. Therefore, it is a good idea to check the model results against other sources such as CTPP or ACS data to ensure that the overall productions are in line with observed aggregate estimates. In addition, overall trip rates per household, trip rates per household by purpose, and percentage of trips by purpose should be compared with results from household travel surveys. If no household travel survey exists, trip rates should be compared to results from other comparable areas or from the National Household Travel Survey (NHTS) for reasonableness.
Trip Attractions
Unlike trip productions, there are few resources for checks of trip attractions, due to a lack of work place surveys that are used to calculate attraction rates. One common check is to compute the number of home-based work attractions to the total employment by zone. Since some workers do not work every workday, the reasonable range is between 1.20 and 1.55 home-based work attractions per employee.
Trip Balancing
Once the trip productions and trip attractions are estimated, their totals by purpose should be compared. In theory, the estimated total trip productions must be equal to the total trip attractions for each trip purpose, because every trip has one production end and one attraction end. However, since trip production and attraction models are independently developed, this equality is not guaranteed. The ratio of area productions to attractions by purpose should be between 0.9 and 1.1 before balancing. If there is not a close match, the reasons for the lack of match should be investigated.
# Trip Distribution Models
There are two types of calibration and validation checks usually conducted for trip distribution models: trip length checks and travel pattern checks. Household travel survey data is usually required for these checks and CTPP journey-to-work data is a supplementary source for home-based work trips.
Trip Length Checks
The average trip length and trip length frequency distributions by purpose are compared between model results and observed data from the household travel survey. Trip lengths should be checked in both distance and time units. Since trip length information reported in household travel survey is not reliable, observed average trip length and trip length frequency distributions are usually computed from the trip table obtained from the expanded household travel survey data using the time and distance skims.
If the modeled and observed average trip lengths by purpose are within 5%, this is typically considered reasonable.
Coincidence ratios are used as a measure of how close the estimated trip frequency distribution is to the observed distribution. The coincidence ratio measures the percent of total area in common between two distributions. The coincidence ratio lies between 0 and 1, where a ratio of 0 indicates two disjoint distributions and 1 indicates identical distributions. It is preferable to have 0.7 or higher coincidence ratio for each purpose.
Travel Pattern Checks
The basic check for travel pattern is the comparison of district-level trip interchanges by purpose. Although zone-level comparison is desirable, limited sample sizes of household travel surveys do not allow a comparison of trip interchanges at a zone level. The estimated trip table from the model is compared to observed trip table from expanded household travel survey at a district level to check origin-destination travel patterns. This comparison can be useful in determining the reasonableness of trip distribution results as well as in assessing the possible need for K-factors. K-factors account for physical or socioeconomic barriers that affect trip making, but are not represented in the model, such as a river crossing. K-factors are usually not recommended and should be used as a last resort after all other possible causes for error and calibration adjustment have been considered.
A validation measure of travel patterns is the orientation ratio. This ratio measures the propensity of trips from a production area to the attraction area. A value of less than 1.0 indicates that the production area is less orientated to the attraction area than is the region as a whole, while a value of greater than 1.0 indicates the opposite. The ratios of the modeled to observed district-level orientation ratios can be used to check trip distribution results. The closer the ratios are to 1.0, the better the model is reproducing observed trip distribution pattern.
Another check of travel patterns is the amount of intrazonal trips. The modeled percentage of intrazonal trips by purpose should be compared to the observed percentages of intrazonal trips from the expanded household survey. If the modeled percentage of intrazonal trips is within 3 percentages of observed for each purpose, it is usually considered reasonable.
# Mode Choice Models
# trip assignment models, # activity based models, # population synthesis, # long term choice models, # statewide travel models, # model system validation.
← Skim Matrix Mode choice →
This site uses cookies to learn which topics interest our readers.
- Experiment List
You have not logged in!!!
please login to perform the experiment.
Trip Generation
Trip Generation is the first step in the Sequential Demand Modelling arrangement which is also called as the Four Step Transportation Planning Process(FSTP) as mentioned earlier. In order to carry out modelling, the variable consists of total number of person-trips generated by a zone as a dependent variable and the independent variable consists of household and socio- economic factors which influence the trip making behaviour of the person. The data for the independent variable should be attained from an analyst. The output thus obtained consists of trip making or trip ends for each zone within a region.
In contemporary transportation planning language, A Trip is defined as a one way person movement by a mechanized mode of transport, having two trip ends. The start of the trip is called as origin and the end of trip is called as destination. Trip is classified as Production or Origin and Attraction or Destination. It should be note that the terminologies used are not identical. To understand with an example consider a single worker on a typical working day making a trip from his house which is in zone P to his office in Zone Q . Thus his trip origin will be zone P and trip destination will be zone Q . For the return trip from office to house his trip origin will be zone Q and trip Destination will be Zone P . Thus from the above Example it can be understood that the term Origin and Destination are defined in terms of direction of the trip while Production and Attraction in terms of land use associated with each trip end. Trip Production is the home end of home based trip and is the origin of trip of non home based trip. Trip Attraction is the non home end of home based trip and is the destination of a non home based trip.

It has been found that better trip generation models can be obtained if the trips by different purpose are identified and modelled separately.The trips can be classified as given below:
1. Home Based Trip: One of the trip end is home.
Example: A trip from home to office.
Following are the list of home based trips that is trip purpose which are classified into five categories:
a. Work Trips
b. School Trips
c. Shopping Trips
d. Social- recreational Trips
e. Other Trips
The first two trips are mandatory trips while other trips are discretional trips. The other trip class encompasses all the trips made for less routine purpose such as health bureaucracy etc.
2. Non Home based trips: None of the trip end is home.
Example: A trip from office to Shopping Mall.
3. Time based trips
The proportion of journey is different by different purposes usually varies with time of the day. Thus the classification is often given as Peak and Off Peak Period Trip.
4. Person-type based trips
The travel behaviour of an individual is mainly dependent on its Socio-Economic attributes. Following are the categories which are usually employed.
a. Income Level- Poor, Middle Class, Rich
b. Car Ownership- 0,1,2,3
c. Household Size- 1,2,3,4... etc
a. No. of workers in a household.
b. No. of Students.
c. Household size and composition.
d. The household income.
e. Some proxy of income such as number of cars etc.
a. Floor area and number of employment opportunities in retail trade, service, offices manufacturing and wholesale areas.
b. School and college enrolment
c. Other activity centres like transport terminals, sports stadium, major recreational/ cultural/religious places
Table below represents base year data of Trip Production for exact zone.
Similarly Trip Attraction Table is obtained with respect to its influencing variables.
Trip generation study typically involves the application of residential trip production which contains variable that defines the demographic makeup of zonal population and trip attraction that captures the activity of non residential activities within the zone.
In the example given below the zones are connected by a two way link. Each zone will have its own demographic and non residential characteristics depending on which the Trip Generation table is represented below.

Modelling basically relates the dependent variable ie trips produced by a zone for aggregated model or household trip production rate for household based models to the corresponding Independent variables characterised by the whole zone or household characteristic respectively. Calibration is done based on the set of observations obtained corresponding to the zones for aggregate model and for disaggregate model employs a number of base year observations corresponding to an individual household in a sample of household drawn randomly from the region. Thus we first need to identify what are the relevant variables: a. Home end b. Work End c. Shop End
Analytical tools used for Trip Generation Modelling are given below: 1. Regression Model (Regression Analysis) 2. Cross Classification Model (Category Analysis)
The purpose of trip generation is to estimate the number of trip ends for each zones for the targeted year. The trip end is calculated for different travel purpose within the zone. These trips are represented as residential trip production obtained from household based cross classification tables or non residential trip attractions which is obtained by projection of land use. Trip generation Models that are often used are Multiple Linear Regression Model or Cross Classification Model or involves combination of both.
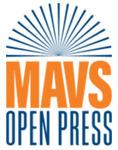
Want to create or adapt books like this? Learn more about how Pressbooks supports open publishing practices.
Part III: Travel Demand Modeling
11 Second Step of Four Step Modeling (Trip Distribution)
Chapter overview.
This chapter describes the second step, trip distribution, of the four-step travel demand modeling (FSM). This step focuses on the procedure that distributes the trips after trip generation has been modeled, meaning after trips generated from or attracted to each zone in the study area are understood. The input for this step of FSM is the output from the previous step discussed in Chapter 10, trip generation plus the interzonal transportation costs introduced in this chapter. Based on the concepts of the gravity model, the trip flows between pairs of zones can be calculated as an origin-to-destination (O-D) matrix. The essential concepts and techniques, such as growth factors and calibration methods, for this step are also discussed in this chapter.
Learning Objectives
- Explain trip distribution and how to relate it to the first step (trip generation) results.
- Summarize the factors that determine the level of attractiveness of zones in a travel demand model.
- Summarize and compare different methods for trip distribution estimation within FSM.
- Complete the trip distribution step by balancing total trip productions and attractions after the trip distribution step.
Introduction
This chapter delves into trip distribution, which is the second step of the Four-Step Model (FSM). After generating trip productions and attractions (P-A trips) by zone in the first step, the next task is to compute the number of trips between each pair of zones, referred to as trip distribution. These outputs are commonly known as Origin-Destination pairs (O-D pairs or Tij, as discussed in Chapter 9), indicating the number of trips between Zone i (origin) and Zone j (destination) (Levine, 2010). Essentially, trip distribution transforms the outcomes of the first FSM step into a comprehensive matrix detailing origins and destinations in Traffic Analysis Zones (TAZs). It also considers travel impedance factors, such as travel time or cost, for each O-D pair. Figure 11.1 illustrates the input (P-A trips) and outputs (trip tables) of this step in the model, highlighting the role of impedance functions initially introduced in Chapter 3 and discussed further in this chapter.
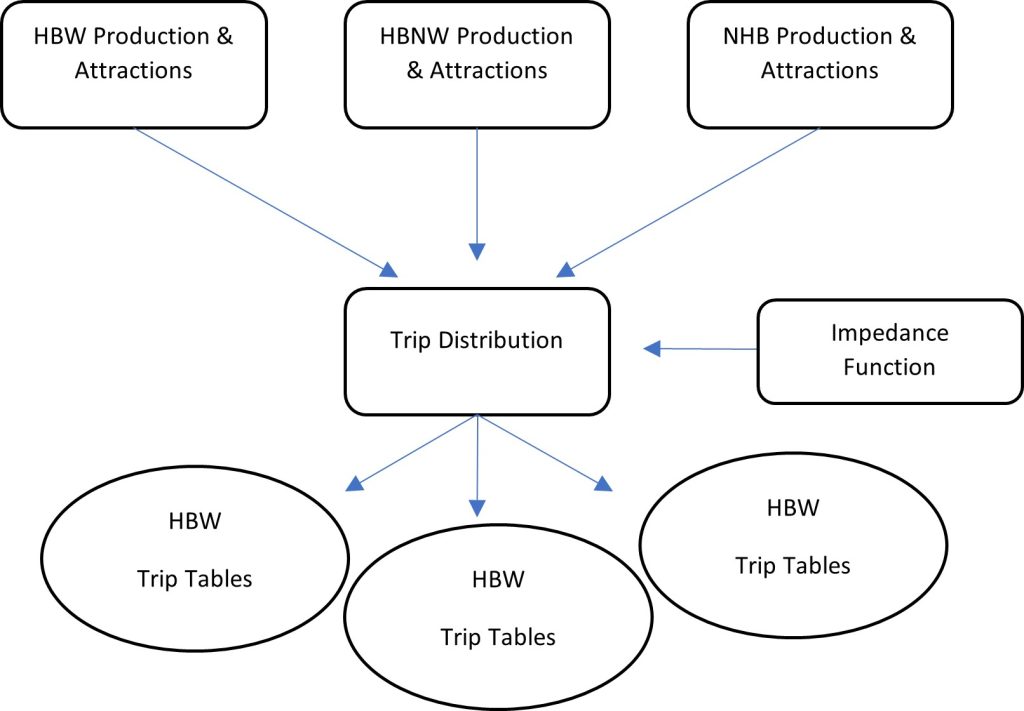
Recall from Chapter 10, that each step of the FSM answers a question specific to the step but central to model determining travel demand in a study area. For the trip distribution step, the main question is “What portion of trips produced in or attracted to a zone would go to each of the other zones?” There are several methods typically used to estimate trip distribution. While growth factor models and the intervening opportunities model are used , the gravity model is the most common.
There are a few foundational components to consider prior to calculating trip distribution. It is important to note that these components are independent of the FSM framework, or the methods used for trip distribution estimation. However, they serve as inputs for estimating trip distribution. As previously mentioned, trip distribution constitutes the second step of the FSM, where trip productions are allocated to all other zones. The outcomes yield a matrix that displays the number of both intrazonal and interzonal trips in a single table (Lincoln MPO, 2011).
The attractiveness of a zone is influenced by several factors (Cesario, 1973):
- Uniqueness : This factor indicates how unique a service or employment center is and thus attracts more trips regardless of distance.
- Distance: The spatial separation, distance, between two zones plays an impedance role, meaning that the further the two zones are from each other, the fewer trips will be distributed between them.
- Closeness to other services: The assumption is that proximity to other desirable services will result in more trips to that zone within an urban area.
- Urban or rural area : The assumption is that the attraction rate for a zone differs based on its urban or rural classification, while controlling for other factors.
In addition to the destination’s attractiveness factors, the origin’ has an emissivity factor, which is usually represented by population, employment, or income (Cesario, 1973). With a general understanding of the factors affecting trip distribution from origin and destination, we can now proceed with an introduction to methodology.
Gravity Model
As we discussed, the gravity model is the most common method used to estimate trip distribution. Gravity models are easy to understand and very accurate, and they can also accommodate varied factors such as population, employment, socio-demographics, and transportation systems. Almost all U.S. Departments of Transportation (DOTs) use gravity models. In contrast, the Growth Factor Model, discussed in a subsequent section, requires additional data about trip distribution in the base year and an estimate of the number of future trips in each zone, which is only sometimes available (Meyer, 2016).
It is important to remember that the Gravity Model is built based on the number of trips made between two zones. The number of trips is directly linked to the total number of attractions in the destination zone and inversely proportional to a function of cost, which may be represented by travel time or trip cost (Council, 2006). The formula gets its name from Newton’s Law of Gravity , which states that the attractiveness between two bodies is related to their mass (positive attraction) and the distance between them (negative attraction) (Verlinde, 2011). In transportation modeling, the two main factors are trip production and attraction, along with the time duration of travel or the cost of travel. While using the gravity model is simple, determining the optimal value for the impedance factor can be difficult. This value is highly dependent on the context and can vary by circumstances.
Equation below shows the fundamental equation of trip distribution:
Trips between TAZ1 and TAZ2=Trips prodduced in TAZ1*(Attractiveness of TAZ2 /Attractiveness of all TAZs
As equation (1) shows, the total trips between zones are equal to the products of the trips produced in a zone, a ratio of the attractiveness of the destination zone, and the total attractiveness of all zones. We can represent the gravity model in several different ways. Remodifying equation the original equation, the gravity model can be rewritten as:
Trips ij =Productions i *(Attractions j *FF ij *k ij /∑Attractions j *FF ij *k ij )
Where Trips ij is the number of trips between zone i and zone j , Prouctionsi is trip production in zone i , Attractionsj is total trips attracted to zone j , FF ij is the friction factor (travel impedance) between i and j , and K ij are the socio-economic factors of zones i and j . These values will be elaborated later in this chapter.
From the above equations, the mathematical format of gravity model can be seen in equation below:
![Rendered by QuickLaTeX.com T_ij=P_i\ [(A_j\ F_ij\ K_ij)/(\sum_l\ A_j\ F_ij\ K_ij\ )]](https://uta.pressbooks.pub/app/uploads/quicklatex/quicklatex.com-233c6adc83febe12db9e4dd8900b6807_l3.png)
T ij = number of trips that are produced in zone i and attracted to zone j
P i = total number of trips produced in zone i
A j = number of trips attracted to zone j
F ij = a value which is an inverse function of travel time
K ij = socioeconomic adjustment factor for interchange ij
Recall that the Pi and Aj values are determined through the trip generation process (refer to Chapter 10), and the sum of all productions and attractions should be equal (PE, 2017). Numerous studies confirm that people value travel time differently based on the purpose of the trip (like work trips vs. recreational trips) (Hansen, 1962; Allen, 1984; Thill & Kim, 2005). Therefore, it is rational to compute the gravity model for each trip purpose using different impedance factors (Meyer, 2016).
Impedance Factor
The impedance factor (aka friction factor) is a value that varies for different trip purposes because, with the FSM model, the assumption is that travel behavior depends on trip purpose. Impedance captures the spatial separation between two zones, represented as travel time or cost.
Friction factors (FF) can be estimated using different measure, as follows:
- A simple measure of friction is the travel time between the zones.
- Another method is adopting an exponential formula with the 1/exp (m × Tij) friction factor, where m is the average travel time calculated using empirical data.
- Gamma distribution uses scaling factors to estimate distribution (Cambridge Systematics, 2010; Meyer, 2016).
The impedance factor reflects the difficulty of traveling between two zones. The friction factor is higher when accessibility between two zones is easy and is zero if no individual is willing to travel between two zones.
There is also a calibration step in the friction factor estimation process. For calibration, trip generation and attraction values are distributed between O-D pairs using the gravity model. Next, the number of trips is compared with a particular amount of time to the results of the O-D survey (observed data). If the numbers do not match, calibration adjusts for the friction factor. When using travel time as the measure of impedance, the relationship between the friction factor and time in the is represented as t-1, t–2, e– t (Ashford & Covault, 1969).
The friction factor is estimated for the entire analysis area. However, such an assumption is limiting because travel costs or time has different implications for different households. For example, a toll on a specific highway may result in disparate use. The cost burden or friction factor may be too high for low-income individuals or households, meaning a factor greater than zero, but negligible for higher-income households. In this case the friction factor for higher-income commuters is zero.
The friction factors for different trip purposes can also be specified. The figure below (Figure 11.2) shows the function of the friction factor appropriate to the time and for different trip purposes. As the figure shows, there is a direct relationship between friction factor and travel time. According to this figure, for each trip purpose, there is a perception about the length or impedance of trip. Beyond certain length, friction factor approaches zero, meaning a high level of disutility of the trip and this threshold is different for each trip purpose. In very general terms, a friction factor Fij that is an inverse function of travel impedance Wij is used in trip distribution to plug in the travelers’ willingness to travel between zone i and zone j .
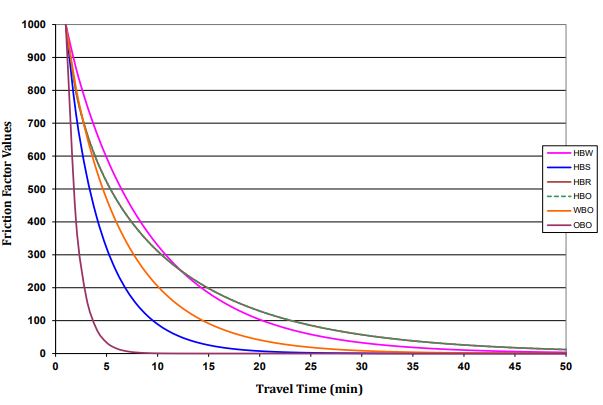
In very general terms, a friction factor F ij that is an inverse function of travel impedance W ij is used in trip distribution to plug in the travelers’ willingness to travel between zone i and zone j .

Travel demand modeling is influenced by various socio-economic factors that affect travel behavior and demand for different purposes. Chapter 10 highlights the most significant factors in travel demand modeling: income, auto ownership, availability of multimodal transportation systems, age, and job type (Pan et al., 2020). Therefore, the K-Factor method was developed and plugged into the gravity model to represent variation in socio-economic factors and adjust interzonal trips accordingly. For example, a blue-collar employee working in a low-income suburb may exhibit different travel behaviors (in terms of mode choice and frequency of travel) compared to a white-collar employee working in the central city with a higher income. The K-factor is determined and plugged into the gravity formula to accommodate such differences.
Recall the classic land use models presented in Chapter 4. Based on the proximity to employment centers or the central business districts, different neighborhoods offer housing and job options tailored to individuals in different income brackets. For example, the earnings of employees in chain restaurants significantly contrast with those working in Central Business District (CBD) headquarters. Prevailing land use policies and the typical American development pattern heighten this disparity. Consequently, these groups are likely to inhabit geographically distant areas in a country like the United States. Furthermore, people of varying income levels or social statuses may respond differently to travel impediments, such as travel time or cost.
Calibration of K values is determined by comparing the estimated results and observed data for the base year (Tawfik & Rakha, 2012). The K numeric value will be above one (>1) if the socio-economic factors contribute to more travel and less than one (< 1) if otherwise (Meyer, 2016). Figure 11.3 shows the mean number of trips for different age groups (K-factor) and various trip purposes. Accordingly, calculating friction factors and K-factors for different purposes and socio-economic groups yields a better fit to the data.
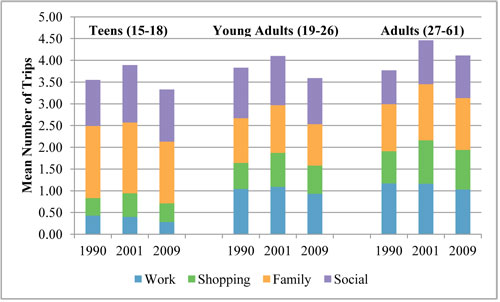
11.2.3 Example 1
Consider a small area with three zones (TAZs). Table 11.1 shows the trip generation results for each zone, and Table 11.2 shows the travel time for each pair of zones. The friction factor is also given in this example as a function of travel time in Table 11.3. The intrazonal travel time for zone 1 is larger than that of most other inter-zone times because of the geographical characteristics of the zone and lack of access within the area. Using this information, please calculate the number of trips for each pair of zones.
For the calculation of trip distribution between the three zones, the trip generation and attraction table from step one of the FSM model is the input data, and then the gravity model is used for calculation. Tables 11.1, 11.2, and 11.3 represent the trip generated and attracted for each zone, travel time between each pair of zones, and friction factor derived from the travel time.
Now with this information, we can start the calculation process. First, we have to estimate the attractiveness of each zone using the equation (1)
For example, for zone 1 we have:
Attractiveness1= 210*26=5460
Attractiveness2= 210*35=7350
Attractiveness3= 350*35=12250
Now, we use the pivotal formula of the gravity model (equation 2). Accordingly, we have (K-factor set to 1):

The result of the calculation is summarized in Table 11.4:
However, our calculations’ results do not match the already existing and observed data. The mentioned mismatch is why calibration and balancing of the matrix are needed. In other words, we must perform more than one iteration of the model to generate more accurate results. For performing a double or triple iteration, we use a formula discussed at the end of this chapter (example adapted from: Garber & Hoel, 2018).
Growth Factor Model
After successfully calibrating and validating the data we have estimated, we can also apply the gravity model to forecast future travel behavior or travel patterns in our study area. Future trip distributions can be predicted by using the change in land-use data, socioeconomic data, or any other changes in the whole system. We can calculate trip distribution from the O-D table for either base or forecasting year when the friction factor and K-factor data are unavailable or unsatisfactorily calibrated. Depending on historical trends and data, growth factor models are limited if an observed O-D table is unavailable. Similar to the trip generation step, growth factor models cannot incorporate updated travel time as the change in travel time between zones can highly affect travel patterns (Qsim, 2016).
Fratar method
One of the most common mathematical formulas of the growth factor model is the Fratar method, shown in the following equation. Through his method, the future distribution of trips from one zone is equal to the present distribution multiplied by the growth factor of the destination zone between now and the forecasting year (Heanue & Pyers, 1966). The formula to calculate future trip values is shown in equation below:

T ij =number of trips estimated from zone to zone t i =present trip generation in zone G x =growth factor of zone T i =future trip generation in zone t ix =number of trips between zone and other zones t ij =present trips between zone and zone G j =growth factor of zone
The following section will discuss an example illustrating the application of the Fratar method.
The case study area of this example consists of four TAZs. Table 11.5 shows the current trip distributions. Assuming the growth rate for each TAZ is shown in Table 11.6, the next step is to calculate the number of trips between each two TAZs in the future year.
To solve this problem, apply the Fratar Method using the required two estimates for each pair. These estimates should be averaged; the resulting value will be the final T ij . Based on the formula, calculations are as follows:

Based on the calculations, the first iteration of the method will yield the following table:
To estimate future trip rates between zones, use the Fratar formula, as shown in Table 11.7. However, there is a problem with the estimated total number of trips generated in each zone not being equal to the actual trip generation. Therefore, a second iteration is necessary. In this second iteration, we use the new O-D matrix as the input to calculate new growth ratios. The trip generation is estimated to occur in the next five years based on the preceding calculation. As an exercise, you can conduct as many iterations as needed to bring the estimated and actual trip generations into alignment.
In a hypothetical area, we are interested in determining the number of trips attracted by three different shopping malls at various distances from a university campus that generates about 2,000 trips per day. In Figure 11.4, the hypothetical area, the number of trips generated by the campus, and the total number of trips attracted for each zone are presented:
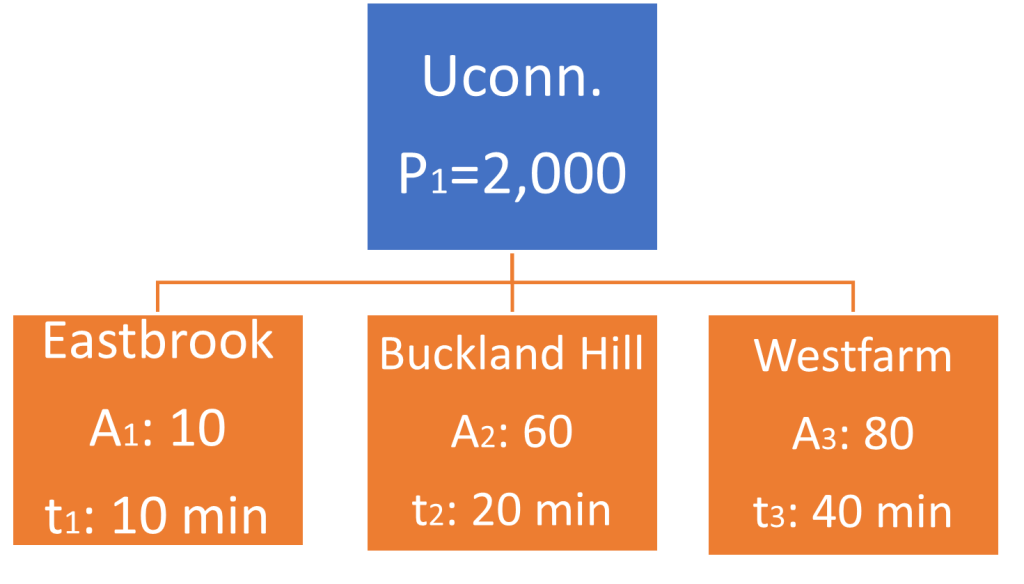
- socioeconomic adj. Factor K=1.0
- Calibration factor C=2.0
As the first step, we need to calculate the friction factor for each pair of zones based on travel time (t). Given is the following formula with which we calculate friction factor:

Next, using the friction factor, we use the gravity model to calculate the relative attractiveness of each zone. In Table 11.8 , you can see how calculations are being carried out for each zone.
Next, with having relative attractiveness of each zone (or probability of attracting trips), we plug in the trip generation rate for the campus (6,000) to finally estimate the number of trips attracted from the campus to each zone. Figure 11.5 shows the final results.
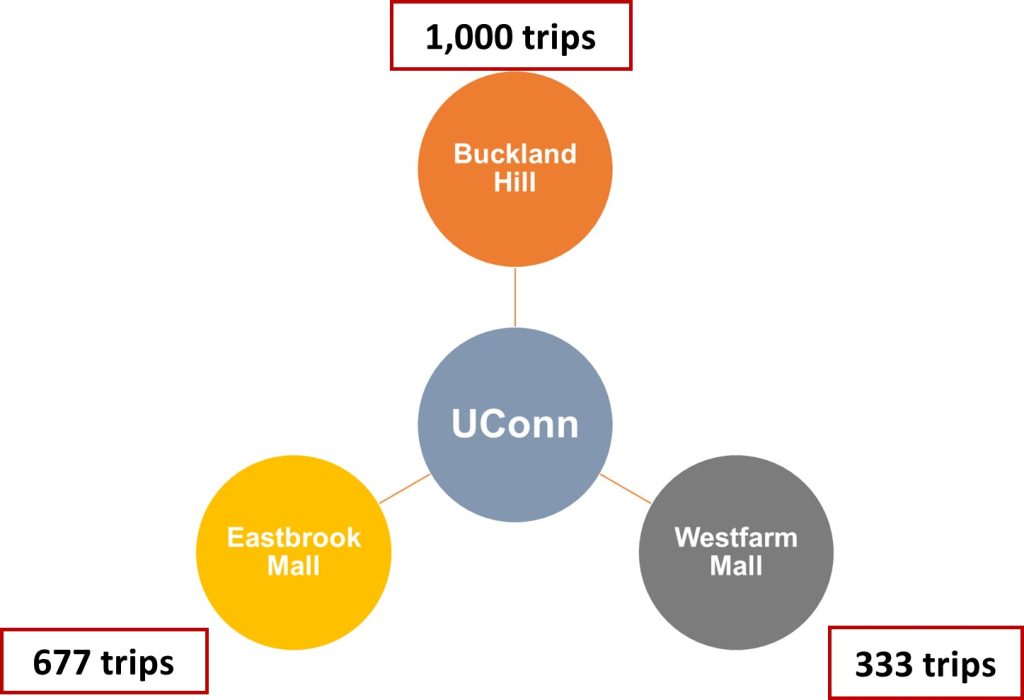
Model Calibration and Validation
Model validation is an integral part of all simulation and modeling procedures. One of the most essential steps in FSM modeling is developing a procedure to calibrate its final outputs (predictions) with actual and observed data. To do this, model parameters are adjusted so that the observed data and estimations have fewer mismatches (Meyer, 2016). After such adjustments, the model with calibrated parameters can help in simulation and future scenario analyses.
After completing the trip distribution step, it is important to compare model calibration and adjustment results in each category (i.e., by trip purpose) with recorded real-world trips from the O-D survey.
If the two values are not identical, model parameters, like FF or K-factors, are reassigned and re-run the gravity model. The process continues until the observed data and estimations are very close (ratio between 0.9 and 1.1).
The following example shows the process of trip distribution step with calibration.

11.4.1 Example 4
This example demonstrates the calibration process. The first step is to identify the model’s inputs, which are the outcomes of the trip generation process. The following tables show the results obtained from surveys and actual trip data, as well as the travel time between each pair of zones (represented by the friction factor (FF)) and the socioeconomic conditions (Tables 11.10, 11.11, and 11.12 ). The column with the heading “A’ “in Table 11.11 represents observed generated trips.
here are several formulas, such as negative exponential or inverse power function, that can be used to calculate friction factors from impeding factors like travel cost or time, as discussed in previous sections. To estimate the number of trips between each pair of zones, we use the gravity formula and input the necessary data. Table 11.14 shows the results of trip distribution for each pair of zones. However, the total number of trips attracted from our calculations is different from observed data.
Now, by looking to the last table we can see that the total number of trips produced is exactly matching to the results of the trip generation table. But the total attractions and actual data have a mismatch. In the next step, we apply the calibration methods in order to make our final results more accurate.
In the first iteration of calibration, we have to generate a value called column factor, which is the result of dividing actual data attraction by estimated attractions. Then we apply this number for each pair in the same column. In Table 11.15, we can observe that the sum of attractions is now the same as the actual data, but the sum of generation amounts is now different from actual data generation. In this step, we perform another iteration, the same as the first iteration but instead of column factor, we plug in row factor value, which is the result of dividing actual data trip generation by estimated generation.
A third iteration is needed because the sum of attraction is still different from the actual data, and we must generate another column factor. The results are shown in Table 11.17.
Based on the results of the third iteration results, we see the attractions are now accurate, and trip generations have very insignificant differences with actual data. At this point, we can stop the calibration. However, the procedure can continue to calibrate results to decrease the difference as much as possible. In other words, the sensitivity of the calibration, the threshold for the row and column factors, can be adjusted by the modeler.
In this chapter, we demonstrated the procedure, application and other details of the second step of FSM modeling framework. Using the concept of gravity-based accessibility, we saw how the production and attraction table can be transformed into a trip distribution matrix. By using simple numerical examples, we showed how different methods can be applied to calculate trips between pair of zones. Assumption of homogenous behavior, assumption of static and sequential behavior, aggregation biases, and less emphasis on lots of social and physical barriers. Dynamic modeling (concurrent mode and destination choice), micro-simulation, agent-based models or newer methods such machine learning have made several enhancement to the traditional model. Collection of real-time data as well as increase in computational capacity has opened such prospects in travel demand modeling and trip distribution studies.
- Emissivity is a quantity that represents the trip production rate of a neighborhood, similar to attractiveness for trip attraction.
- Intra zonal trips are those trips that both ends of the trip is in the same zone.
- Interzonal Trips are those trips where one end of the trip is in a different zone.
- Uniqueness is a quantity defined for a TAZ that indicates how unique that zone or trip attraction center is.
- Gamma distribution is a probability distribution that is used for converting travel times into impedance functions
Blue-collar employee is a worker who usually performs manual and low-skill duties for their work.
White-collar employee is a worker who is high-skill and performs professional, or administrative work.
- Zonal Emissivity refers to a quantity that represents the trip making rates for that zone. Factors affecting this feature can be population, employment, income level, vehicle ownership, etc.
Key Takeaways
In this chapter, we covered:
- What trip distribution is and the factors that determine attractiveness of zones for travel demand.
- Different modeling frameworks appropriate for trip distribution and their mathematical formulation.
- What advantages and disadvantages of different methods and assumptions in trip distribution are.
- How to perform a trip distribution manually using simplified transportation networks.
Prep/quiz/assessments
- What factors affect the attractiveness of the zones in trip distribution, and what input data is needed to measure such attractiveness?
- What are the advantages and disadvantages of the three trip distribution methods (gravity model, intervening opportunities, and Fratar model)?
- What are the friction factor and K-factor in trip distribution, and how do they help to calibrate model results?
- How should we balance trip attraction and production after performing trip distribution? Explain.
Allen, B. (1984). Trip distribution using composite impedance. Transportation Research Record , 944 , 118–127.
Seggerman, KE. (2010). Increasing the integration of TDM into the land use and development process. Fairfax County (Virginia) Department of Transportation, May. Department of Transportation.
Cesario, F. J. (1973). A generalized trip distribution model. Regional Science Journal , 13 (2), 1973-08
Council, A. T. (2006). National guidelines for transport system management in Australia 2006 . Australia Transportation Council. https://www.atap.gov.au/sites/default/files/National_Guidelines_Volume_1.pdf
Garber, N. J., & Hoel, L. A. (2018). Traffic and highway engineering . Cengage Learning.
Hansen, W. G. (1962). Evaluation of gravity model trip distribution procedures . Highway Research Board Bulletin, 347 . https://onlinepubs.trb.org/Onlinepubs/hrbbulletin/347/347-007.pdf
Ned Levine (2015). CrimeStat : A spatial statistics program for the analysis of crime incident locations (v 4.02). Ned Levine & Associates, Houston, Texas, and the National Institute of Justice, Washington, D.C. August.
Lima & Associates. (2011). Lincoln travel demand model . Lincoln Metropolitan Planning Organization. (2011). https://www.lincoln.ne.gov/files/sharedassets/public/planning/mpo/projects-amp-reports/tdm11.pdf
Meyer, M. D. (2016). Transportation planning handbook . John Wiley & Sons.
NHI. (2005). Introduction to Urban Travel Demand Forecasting . In National Highway Administration (Ed.), Introduction to Urban Travel Demand Forecasting. American University. . National Highway Institute : Search for Courses (dot.gov)
Pan, Q., Jin, Z., & Liu, X. (2020). Measuring the effects of job competition and matching on employment accessibility. Transportation Research Part D: Transport and Environment , 87 , 102535. https://doi.org/10.1016/j.trd.2020.102535
PE Lindeburg, M. R. (2017). PPI FE civil review – A comprehensive FE civil review manual (First edition). PPI, a Kaplan Company.
Qasim, G. (2015). Travel demand modeling: AL-Amarah city as a case study . [Unpublished Doctoral dissertation , the Engineering College University of Baghdad]
Tawfik, A. M., & Rakha, H. A. (2012). Human aspects of route choice behavior: Incorporating perceptions, learning trends, latent classes, and personality traits in the modeling of driver heterogeneity in route choice behavior . Virginia Tech Transportation Institute . Blacksburg, Virginia https://vtechworks.lib.vt.edu/handle/10919/55070
Thill, J.-C., & Kim, M. (2005). Trip making, induced travel demand, and accessibility. Journal of Geographical Systems , 7 (2), 229–248. https://doi.org/10.1007/s10109-005-0158-3
Verlinde, E. (2011). On the origin of gravity and the laws of Newton. Journal of High Energy Physics , 2011 (4), 1–27. https://link.springer.com/content/pdf/10.1007/JHEP04(2011)029.pdf
Transportation Land-Use Modeling & Policy Copyright © by Mavs Open Press. All Rights Reserved.
Share This Book
Trip Generation Appendices
Tgm appendices.
Click to download in Excel
Pass-By Data and Rate Tables
Time-of-Day Distribution - Truck
Time-of-Day Distribution - Vehicle
Trip Generation Data Plots - Modal
Click to download in PDF
000s - Port and Terminal - Modal Data Plots 1
200s - Residential - Modal Data Plots
300s - Lodging - Modal Data Plots
400s - Recreational - Modal Data Plots
500s - Institutional - Modal Data Plots
600s - Lodging - Modal Data Plots
700s - Office - Modal Data Plots
800s - Retail - Modal Data Plots
900s - Services - Modal Data Plots
Land Uses with Modal Data Plots
Trip Generation Data Plots - Truck
100s - Industrial - Truck Data Plots
200s - Residential - Truck Data Plots
300s - Lodging - Truck Data Plots
500s - Institutional - Truck Data Plots
600s - Medical - Truck Data Plots
700s - Office - Truck Data Plots
800s - Retail - Truck Data Plots
900s - Services - Truck Data Plots
Land Uses with Truck Data Plots

Estimating Truck Trip Generation for Airport Air Cargo Activity (2017)
Chapter: chapter two - description of air cargo system components.
Below is the uncorrected machine-read text of this chapter, intended to provide our own search engines and external engines with highly rich, chapter-representative searchable text of each book. Because it is UNCORRECTED material, please consider the following text as a useful but insufficient proxy for the authoritative book pages.
5 chapter two Description of Air cArgo system components Air freight is transported in dedicated cargo aircraft and in the cargo space of passenger aircraft (belly cargo). As with passenger baggage handling, cargo on narrow-body and smaller aircraft is loaded individually, whereas cargo on widebody aircraft is usually containerized. International cargo arriving as imports may have been pre-cleared electronically or may be subject to additional inspection by regulators before being cleared to leave the airport. Perhaps one of the most unique attributes of the air cargo industry is the rapid loading and unloading of commodities onto widebody and narrow- body freighter aircraft by means of unit load devices (ULDs), including pallets and containers. Cargo aircraft have large doors and rollers fastened to the deck of the aircraft. These aircraft allow containers and pallets laden with freight and mail to be rolled on and off either manually or through a mechanized system. types of cArgo cArriAge As shown in Table 1, there are four primary air cargo transport business models that affect airport facility planning. These are passenger airlines/combination carriers, all-cargo carriers, integrated express carriers, and freight forwarders. passenger Airlines and combination carriers A passenger airline provides cargo services to the industry by offering for sale the capacity of the âbellyâ compartment of its aircraft that is available after the passenger-related items such as food/ beverages, company material, and passenger luggage are loaded. These airlines can provide the industry with air cargo transport flexibility in the form of frequent flights to destinations. Passenger airlines generally provide airport-to-airport service, with freight and mail carried as belly cargo. Airlines usually offer belly cargo space âas available,â because priority goes to passengers and their luggage. These airlines may also be known as combination carriers, which are scheduled air carriers that transport both passengers and cargo in passenger-configured aircraft, with cargo restricted to the lower deck compartments. All-cargo carriers All-cargo carriers operate airport-to-airport air cargo and freight services for their customers but do not offer passenger service. All-cargo carriers offer scheduled service to major markets throughout the world using widebody or containerized cargo aircraft. Major air cargo carriers operating in North America include Polar Air Cargo and Kalitta Air. integrated express carriers Integrated express operators move the customerâs goods door-to-door, providing shipment collec- tion, transport by air or truck, and delivery. Dominant integrated express operators in North America include FedEx, UPS, and DHL. (DHLâs U.S. domestic pickup and delivery service was discontin- ued in January 2009.) Express companies provide next-day and deferred, time-definite delivery of documents and small packages (up to 70 pounds). Integrated express operators are increasingly transporting heavy freight (more than 70 pounds), which is the next logical step in leveraging the
6 unique scale of operations, network, and other resources that operators can bring to each business sector (Maynard 2015). freight forwarders A freight forwarder is a firm that receives, stores, and/or ships goods on behalf of other companies. It usually provides a range of services including tracking inland transportation, preparation of ship- ping and export documents, warehousing, booking cargo space, negotiating freight charges, freight consolidation, cargo insurance, and filing of insurance claims. Freight forwarders usually ship under their own bills of lading or air waybills; their agents or associates at the destination (overseas freight forwarders) provide document delivery, deconsolidation, and freight collection services (Business Dictionary 2016). UPS Supply Chain Solutions (part of UPS, Inc.) and SPO Logistics are the two largest freight forwarding firms in the United States (Transport Topics 2016). types of Airport Air cArgo fAcilities The consolidation of the domestic air cargo industry in recent years has precipitated a situation in which air cargo facilities at many airports no longer have a wide customer base, as more freight is trucked directly to major air cargo hubs, bypassing smaller, low-volume facilities. This has led to many vacant cargo facilities or space that are not well utilized. For example, the U.S. Postal Service once had airmail sorting facilities at most medium and large airports, but has closed many since 2001 because much of its Express and Priority Mail Service has switched from passenger airlines to con- tract agreements with FedEx and UPS. On the international air cargo front, the passenger gateway airports continue to experience greater tonnage growth than the domestic airports, and more inter- national passenger routes and freighter routes continue to expand into U.S. airports. Figure 1 presents a diagram of a generalized on-airport air cargo facility that provides truck access and parking. Such on-airport cargo terminals are usually multi-tenant. These may be common-user spaces managed by an authorized cargo handler, but, as traffic levels increase, carriers and integrators often want to have their own space. Forwarders/customs agents may occupy a designated storage area or merely place their customersâ consignments in a common area (The World Bank Group 2009). Figure 2 illustrates, in simplified schematic form, the various types of ground movements asso- ciated with air cargo at a major air cargo airport. As shown, cargo may flow between aircraft and numerous facilities, both on and off-airport. Similarly, cargo may flow between these facilities and Type of Carrier Example of Carrier Characteristics Customers Market/ Movement Type of Cargo Combination Carrier Most passenger airlines Baggage hold of passenger aircraft Wholesale businesses, mail, retail Airport-to- airport Mail, freight All-cargo Carrier Polar, Kalitta Air, World Airways* Main deck of all-cargo aircraft Wholesale businesses Airport-to- airport Larger specialized freight Integrated Express Carrier UPS, FedEx Express Main deck of all-cargo aircraft Retail businesses Door-to-door Packages, express Freight Forwarders Panalpina, Forward Air All-cargo and passenger aircraft Wholesale businesses Road feeder service (pickup and delivery) Ocean and air freight pickup and delivery *No longer operating. Source: Air Transport Association and International Air Cargo Association [compiled by the Texas Transportation Institute (TTI)]. TABLE 1 TyPES OF AIr CArGO CArrIErS
FIGURE 1 Simple air cargo facility diagram (Source: Maynard et al. 2015). FIGURE 2 Overview of typical air cargo ground movements at major airport (Source: RSG).
8 customers and suppliers outside of the airport, including shippers and receivers many miles distant (e.g., road feeder services) as well as integrated industrial facilities adjacent to airport property such as the JetPlex Industrial Park at Huntsville, Alabama International Airport. The common charac- teristic shared by all these activities is that they generally involve truck movements, both on-airport and between the airport and external parties. Belly cargo Handling for passenger Airlines Freight on passenger airlines is dropped off at a warehouse at the origination airport by a shipper or shipperâs agency; the freight is then picked up at the destination airport by the customer (or freight forwarder) after arriving on the passenger airline. Inbound belly cargo is unloaded and transported to cargo facilities or from one aircraft to another aircraft, whereas outbound belly cargo is transported from trucks to the cargo terminal and loaded onto the aircraft prior to departure. All-cargo carrier facilities All-cargo airlines (e.g., Polar Air Cargo, Cargolux) typically contract with third-party companies for loading, unloading, and ground-handling of cargo at privately or airport owned warehouse facilities. Typically, the same facility at an airport handles both the belly and all-cargo airlines (e.g., Swissport). integrated express carrier facilities Integrated express operators use a hub-and-spoke transport model, such as that used by passenger airlines. The air cargo hub used for package sorting and aircraft transfer is the backbone of inte- grated express operators. This allows for total product connection to each market in the operatorâs system. Each day of operation, flights from around the world arrive at the hub, where packages are unloaded, sorted by destination market, and loaded onto outbound aircraft. Integrators often make heavy use of automated sorting at their hub terminals to achieve desired turnaround times and deliv- ery commitments. regional air cargo carriers operate smaller turboprop aircraft between origin and destination (OD) or local market stations and smaller or more remote cargo markets, typically in support of a larger integrated express cargo operator such as FedEx, UPS, or DHL. Wiggins Airways and Mountain Air Cargo are examples of feeder airlines contracted to both UPS and FedEx. Feeder flights often transport cargo from a smaller market and feed cargo to an awaiting aircraft for the carrierâs hub. Feeder aircraft may also fly directly to a hub.
TRB's Airport Cooperative Research Program (ACRP) Synthesis 80: Estimating Truck Trip Generation for Airport Air Cargo Activity compiles existing information about air cargo truck trip generation studies. The existing literature and research regarding air cargo facility-related truck trip generation rates is limited in its scope and detail. In addition, the complexity of the modern air cargo industry makes it difficult to obtain the data necessary to develop truck trip generation rates. Access to such information could conceivably help a community plan and invest appropriately by accounting for air cargo’s impacts. Similarly, air cargo operators and airport officials could employ such data to help ensure cargo facility truck access and egress remains reliable and safe.
READ FREE ONLINE
Welcome to OpenBook!
You're looking at OpenBook, NAP.edu's online reading room since 1999. Based on feedback from you, our users, we've made some improvements that make it easier than ever to read thousands of publications on our website.
Do you want to take a quick tour of the OpenBook's features?
Show this book's table of contents , where you can jump to any chapter by name.
...or use these buttons to go back to the previous chapter or skip to the next one.
Jump up to the previous page or down to the next one. Also, you can type in a page number and press Enter to go directly to that page in the book.
To search the entire text of this book, type in your search term here and press Enter .
Share a link to this book page on your preferred social network or via email.
View our suggested citation for this chapter.
Ready to take your reading offline? Click here to buy this book in print or download it as a free PDF, if available.
Get Email Updates
Do you enjoy reading reports from the Academies online for free ? Sign up for email notifications and we'll let you know about new publications in your areas of interest when they're released.
TheJakartaPost
Please Update your browser
Your browser is out of date, and may not be compatible with our website. A list of the most popular web browsers can be found below. Just click on the icons to get to the download page.
- Destinations
- Jakpost Guide to
- Newsletter New
- Mobile Apps
- Tenggara Strategics
- B/NDL Studios
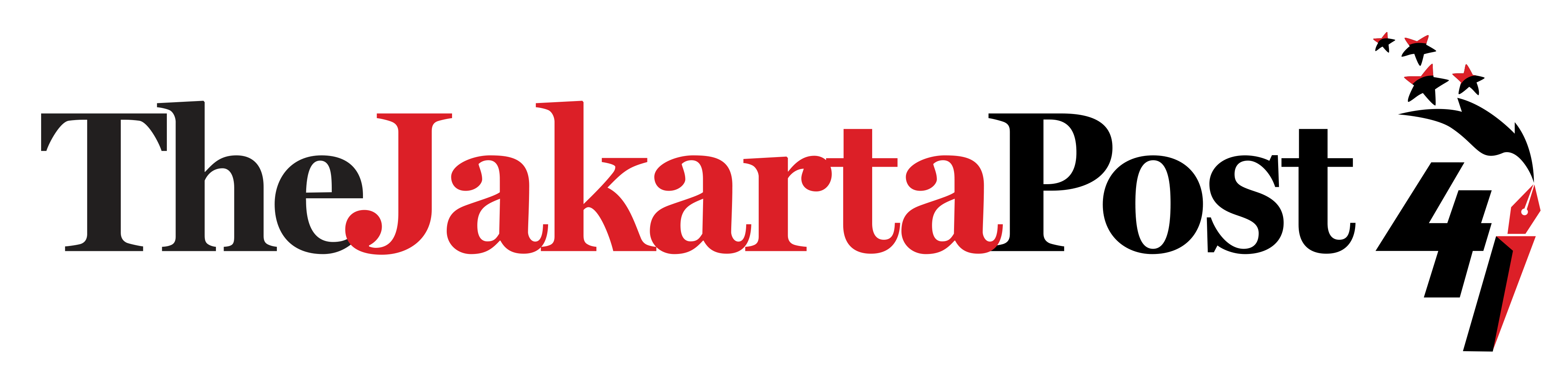
- Archipelago
- Election 2024
- Regulations
- Asia & Pacific
- Middle East & Africa
- Entertainment
- Arts & Culture
- Environment
- Work it Right
- Quick Dispatch
- Longform Biz
Golden generation
The team and the country will need Shin’s touch more than ever after the Asian Cup, whether or not the squad wins the coveted trip to the Paris Olympics.
Share This Article
Change size.
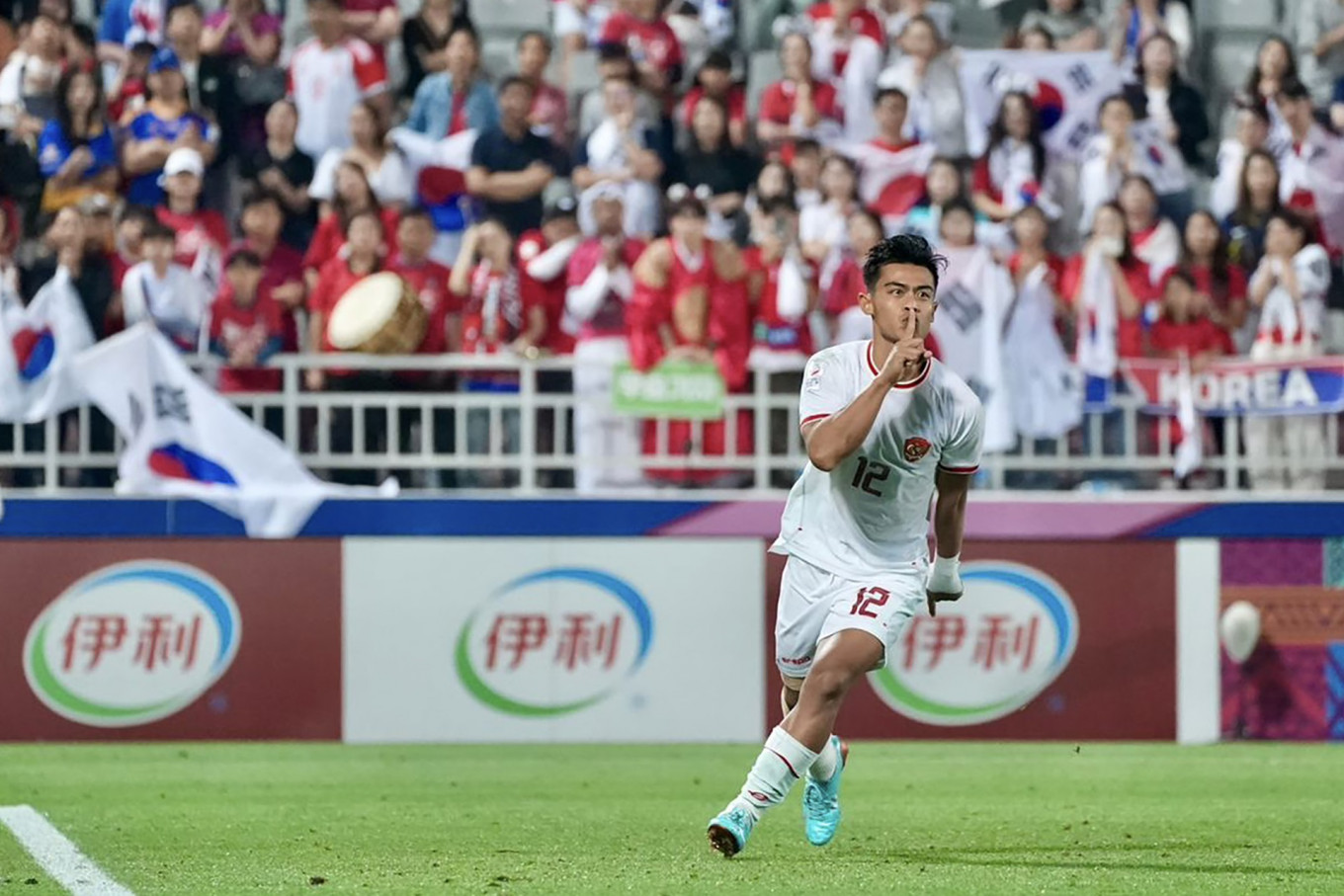
ndonesia’s faith in national soccer team head coach Shin Tae-yong has paid dividends, with the South Korean guiding Young Garuda to the semifinals of the U-23 Asian Cup, bringing the squad one step closer to a historic appearance at the Olympic Games.
Shin was the toast of the town after his lads, in an ironic twist, ended his own national team’s dreams of making it to this summer’s Olympics in Paris. The match, which was held at Doha’s Abdullah bin Khalifa Stadium on Thursday evening, was decided in a dramatic penalty shootout after a pulsating 120 minutes of play resulted in a 2-2 draw.
Indonesian goalkeeper Ernando Ari Sutaryadi saved two penalty kicks and made one of his own to contribute to a 11-10 win against pre-tournament favorite South Korea. He was also responsible for a brilliant tip that denied Australia a point in Indonesia’s 1-0 upset win in their group match on April 18.
Shin’s contract as the national team’s head coach had been extended until 2027 even before the quarterfinal victory against South Korea. It was the right decision at the right time, considering the squad’s convincing performance during his tenure.
The team and the country will need Shin’s touch more than ever after the Asian Cup, whether or not the squad wins the coveted trip to the Paris Olympics. Blessed with a big pool of talent, Indonesia has been longing for a ticket to the World Cup, and with Shin at the helm, the dream may just come true in 2026.
Fans have dubbed the current national team the “golden generation” of Indonesian soccer, especially after they won the country’s first Southeast Asian Games gold medal in the sport for more than three decades. The squad lost to Qatar in the opening match of this year’s Asian Cup but fought back, earning two consecutive wins to qualify for the quarterfinals for the first time as the group runner-up to the host.

Every Thursday
Whether you're looking to broaden your horizons or stay informed on the latest developments, "Viewpoint" is the perfect source for anyone seeking to engage with the issues that matter most.
By registering, you agree with The Jakarta Post 's Privacy Policy
for signing up our newsletter!
Please check your email for your newsletter subscription.
Few would have thought Indonesia capable of defeating South Korea, which had won all three prior group matches. Indonesia has lost seven times to the South Koreans in U-23 encounters, including a 7-0 drubbing in a 2000 Olympics qualifying match.
The upset win against Australia was the turning point for Indonesia. The national team’s confidence grew further with another shocking victory, 4-1 over feisty Jordan.
And having reached the semifinals, the squad may have what it takes to go all the way.
They will take on either Uzbekistan or defending champion Saudi Arabia in their semifinal match on Monday, where one ticket to the Paris Olympics is up for grabs.
If Indonesia loses, it can still qualify for the world’s biggest sporting event if it can beat the other losing semifinalist in the playoff for third place. But not even a defeat in that match will dash Indonesia’s hopes entirely, provided that it can overcome Guinea in an Asian-African playoff later in May.
The last time an Indonesian soccer team competed in an Olympics was in 1956 in Melbourne. After being automatically advanced through the first round, Indonesia lost to the Soviet Union in the quarterfinals.
After a long dormancy, Indonesia has slowly but surely regained its reputation as a regional soccer power to reckon with after its strong showing in Qatar over the past two weeks.
Coach Shin’s contribution has been extraordinary in building a strong team physically and mentally. Some players have drawn attention to their coach’s discipline – the key, perhaps, to the team’s winning run in Qatar. He does not hesitate to criticize his players in public for their lack of discipline, such as turning up late for a training session.
The team faces still greater challenges in its quest for soccer glory. It is our responsibility to help our golden soccer generation grow as strong as it can be.
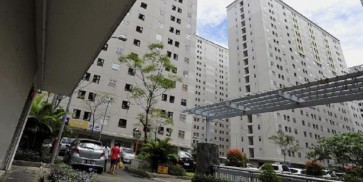
Magnitude 6.5 earthquake strikes off Java island: authorities
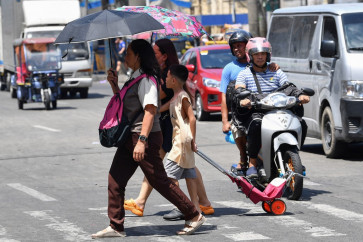
Philippines to endure extreme heat until mid-May
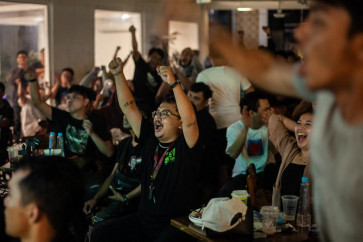
Indonesia one step from Olympics after beating South Korea
Related articles, young garuda makes history by reaching u-23 asia cup quarters after beating jordan 4-1, indonesia, korea can bridge global ai divide, says deputy minister, north korea fires ballistic missiles as blinken visits seoul, genocide should not be synonymous with self defense, jakarta police deploy over 2,000 officers for u-17 world cup, related article, more in opinion.
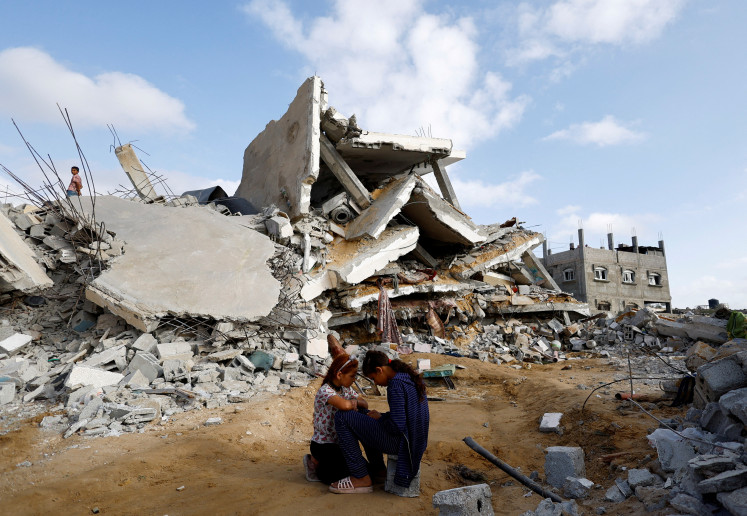
Gaza war tests Indonesia’s free and active foreign policy
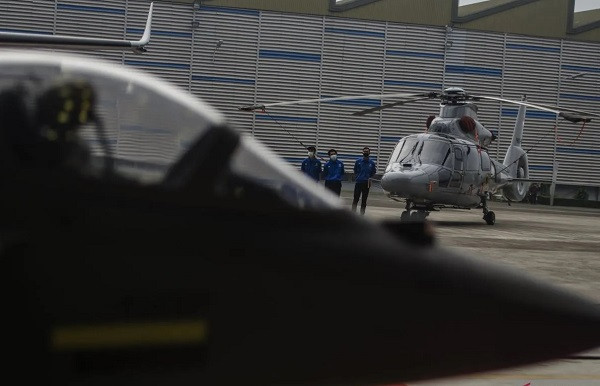
What the next ‘MEF’ for 2025-2045 needs to focus on
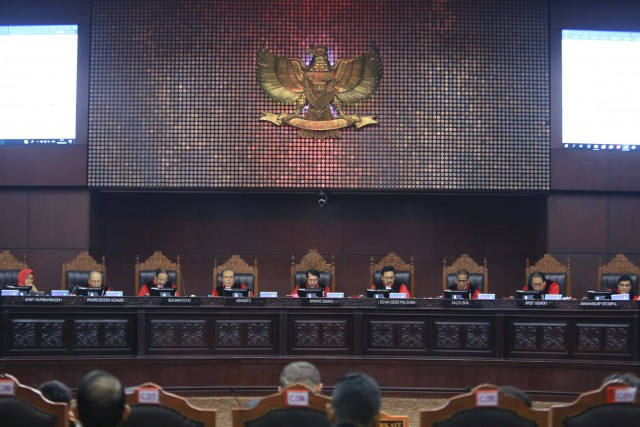
Analysis: Court upholds election results, as expected
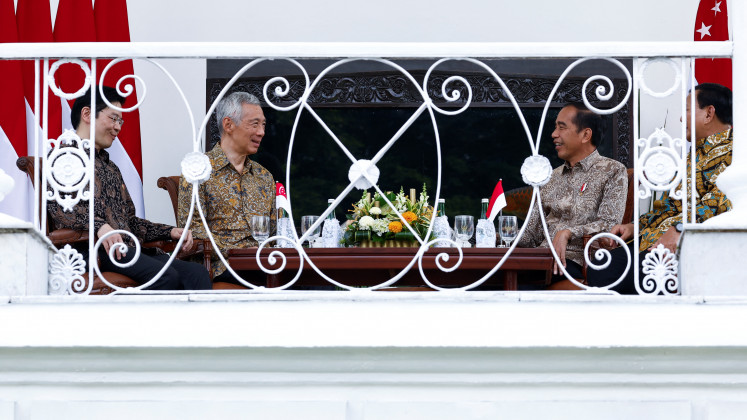
Jokowi, Lee pledge continuity in Indonesia-Singapore ties at their final retreat
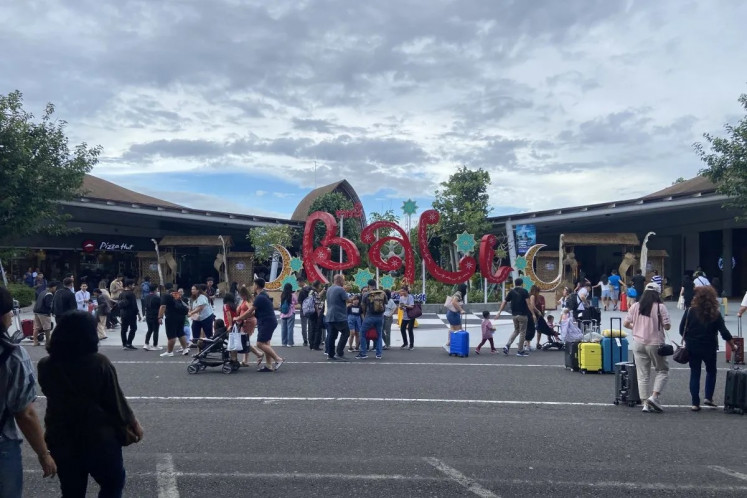
Surcharging air passengers?
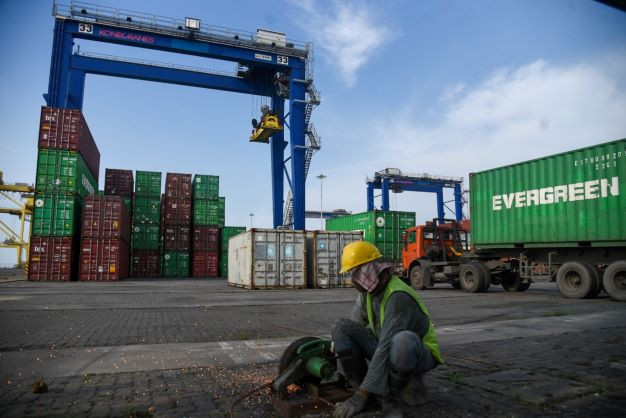
Govt revising import restrictions following business outcry
Goto says on track for profitability despite first-quarter loss, investment growth on track in first quarter, jump in f&b sector, court kicks off hearings for legislative election disputes, idx composite climbs up by 1.7%, retouches 7,100 mark, golkar branch head up against jokowi’s son-in-law for n. sumatra governor, scotland's humza yousaf expected to resign as first minister, 2024 biofuel subsidy funding needs 55% increase: bpdpks, israel concerned over possible icc arrest warrants related to gaza war.

- Jakpost Guide To
- Art & Culture
- Today's Paper
- Southeast Asia
- Cyber Media Guidelines
- Paper Subscription
- Privacy Policy
- Discussion Guideline
- Term of Use
© 2016 - 2024 PT. Bina Media Tenggara
Your Opinion Matters
Share your experiences, suggestions, and any issues you've encountered on The Jakarta Post. We're here to listen.
Thank you for sharing your thoughts. We appreciate your feedback.
Intel issues statement about CPU crashes, blames motherboard makers — BIOSes disable thermal and power protection, causing issues
Intel points out motherboard profiles with disabled protections while exceeding recommended voltages to gain high overclocks.
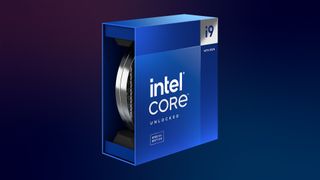
Igor's Lab seems to have obtained a message originally destined for motherboard manufacturers concerning a prolonged stability issue on the company's 13th Generation Raptor Lake and 14th Generation Raptor Lake Refresh chips, which rank among the best CPUs . It made sense for the company to clarify the issue where many blamed the motherboard manufacturers in a race to become 'the fastest' performer by having over-aggressive voltages for allowing higher clock speeds. The company specifically points out the issue with 600/ 700 series motherboard manufacturers that disable thermal and power protection to achieve the highest possible overclocks, even at the cost of instability. The chipmaker said in the message:
Intel has observed that this issue may be related to out of specification operating conditions resulting in sustained high voltage and frequency during periods of elevated heat. Analysis of affected processors shows some parts experience shifts in minimum operating voltages which may be related to operation outside of Intel® specified operating conditions. While the root cause has not yet been identified, Intel® has observed the majority of reports of this issue are from users with unlocked/overclock capable motherboards. Intel has observed 600/700 Series chipset boards often set BIOS defaults to disable thermal and power delivery safeguards designed to limit processor exposure to sustained periods of high voltage and frequency, for example: – Disabling Current Excursion Protection (CEP) – Enabling the IccMax Unlimited bit – Disabling Thermal Velocity Boost (TVB) and/or Enhanced Thermal Velocity Boost (eTVB) – Additional settings which may increase the risk of system instability: – Disabling C-states – Using Windows Ultimate Performance mode – Increasing PL1 and PL2 beyond Intel® recommended limits Intel requests system and motherboard manufacturers to provide end users with a default BIOS profile that matches Intel recommended settings. Intel strongly recommends customer’s default BIOS settings should ensure operation within Intel’s recommended settings. In addition, Intel strongly recommends motherboard manufacturers to implement warnings for end users alerting them to any unlocked or overclocking feature usage. Intel is continuing to actively investigate this issue to determine the root cause and will provide additional updates as relevant information becomes available. Intel will be publishing a public statement regarding issue status and Intel recommended BIOS setting recommendations targeted for May 2024.
Asus was the first to address the issue on its side of the fence as it began rolling out new BIOS revisions with an 'Intel Baseline Profile,' which mitigated the problem by lowering its power limit and using Intel's recommended settings. Eventually, two other motherboards addressed this , with MSI providing a tutorial on how to tune its motherboard BIOS to run Intel's power limit and voltage specifications. Gigabyte rolled out beta BIOS revisions like Asus. It's uncertain why MSI didn't roll out similar firmware for some, if not all, of the company's motherboards. Naturally, these steps will reduce performance, but it's a lot better than having crashes while gaming or during other intense workloads. The problem has mostly been with users of Core i9 SKUs from both generations. The lesson is that overclocking should be a manual and custom attempt, as not all silicon is the same. Enabling extreme settings by default is also not a good idea, especially when increasing the power limit and turning off other specific protection options that are normally enabled out of the box. Intel introduced 'Extreme Power Delivery' with its 13th Generation CPUs, like the Core i9-13900KS , which peaked at 320 watts via this profile. At the same time, Intel already had recommended steps, and motherboard manufacturers should have played it safe so that end-users who would have a tough time navigating manual settings in the BIOS would not be on the receiving end. Perhaps Intel should have stricter guidelines for respective motherboard manufacturers to follow, given that such issues can last a few months before they can be narrowed down.
Stay on the Cutting Edge
Join the experts who read Tom's Hardware for the inside track on enthusiast PC tech news — and have for over 25 years. We'll send breaking news and in-depth reviews of CPUs, GPUs, AI, maker hardware and more straight to your inbox.
Homegrown Chinese CPUs are catching up to AMD and Intel — Loongson 3B6600 and 3B7000 allegedly match Intel 10th Gen CPU performance
AMD's China-exclusive Ryzen CPUs come to the retail market — Ryzen 7 8700F listed for $420 and Ryzen 5 7500F for $296
Modders build PC with coffee machine inside — full roaster and grinder fit in the chassis
- endocine So all of the settings that intel has been OK with, either tacitly or explicitly encouraging motherboard manufacturers to use. Why sell K SKUs and charge a premium for them if they are already at their maximum with intel baseline defaults, and why sell OC chipsets that allow this? Some of these are absurd: "Using windows ultimate performance mode" <--is that a problem even with intel baseline defaults? Really? "Disabling C-States" <--is that a problem even with intel baseline defaults? Really? If those are a problem, then the CPUs can not be run at their baseline spec, its using too much power and generating too much heat, there are workloads that will max them out all the time. PL1 and 2 have been set equal now, with unlimited tau, is that a problem now too? Reply
- Amdlova Memory overclock it's another nightmare... my asrock motherboard says can overclock the ddr4 at 5000+ mhz Think what voltage at IMC and What gear mode... on my test from 3200mhz to 3600mhz incrase almost 10w from RAM and IMC voltage :) (blazing heat speeds) Reply
Perhaps Intel would have stricter guidelines for respective motherboard manufacturers to follow, given that such issues span for a few months before it could be narrowed down.
endocine said: So all of the settings that intel has been OK with, either tacitly or explicitly encouraging motherboard manufacturers to use. Why sell K SKUs and charge a premium for them if they are already at their maximum with intel baseline defaults, and why sell OC chipsets that allow this? Some of these are absurd: "Using windows ultimate performance mode" <--is that a problem even with intel baseline defaults? Really? "Disabling C-States" <--is that a problem even with intel baseline defaults? Really? If those are a problem, then the CPUs can not be run at their baseline spec, its using too much power and generating too much heat, there are workloads that will max them out all the time. PL1 and 2 have been set equal now, with unlimited tau, is that a problem now too?
– Additional settings which may increase the risk of system instability: – Disabling C-states – Using Windows Ultimate Performance mode – Increasing PL1 and PL2 beyond Intel® recommended limits
Intel has observed 600/700 Series chipset boards often set BIOS defaults to disable thermal and power delivery safeguards designed to limit processor exposure to sustained periods of high voltage and frequency, for example:
- D1v1n3D I have to say for competitive reasons it is pretty sad and sucks that Intel keeps fumbling the ball so badly. Releasing the 13th and 14th gen in there state alone is just a disaster waiting to happen, with how hot they run could quite literally cause fires in some situations! But, no one cares to talk about that. Feeding a board with over 300w of power just to get that higher clock that still loses to a much lower clocked CPU that's almost a third the power usage is a tell tail sign Intel doesn't have anything new from core to this hybrid core system still Intel one in the same from core 4th Gen just push the chips to their thermal and frequency limits regardless of risks and when shit hits the fan blame everyone Else. Reply
Admin said: Intel issues a state regarding stability issues concerning 13th and 14th Generation CPUs on 600-and 700-series motherboards made by respective manufacturers. Intel issues statement about CPU crashes, blames motherboard makers — BIOSes disable thermal and power protection, causing issues : Read more
D1v1n3D said: I have to say for competitive reasons it is pretty sad and sucks that Intel keeps fumbling the ball so badly. Releasing the 13th and 14th gen in there state alone is just a disaster waiting to happen, with how hot they run could quite literally cause fires in some situations! But, no one cares to talk about that. Feeding a board with over 300w of power just to get that higher clock that still loses to a much lower clocked CPU that's almost a third the power usage is a tell tail sign Intel doesn't have anything new from core to this hybrid core system still Intel one in the same from core 4th Gen just push the chips to their thermal and frequency limits regardless of risks and when shit hits the fan blame everyone Else.
Digital~Dreams said: How do these crashes appear on folks systems ?. I've had an intermittent (once every few hours) problem where games will suddenly jump to the desktop (like an ALT-TAB). Nothing helpful ever appears in the windows logs and an app that tracked what changed the window focus showed nothing either !?. I can always then select the game on the taskbar and get right back to where I was but still frustrating.
Alvar Miles Udell said: So their guidance should have been "When we say our recommended specs we really mean those are our recommended specs and not our "recommended specs", so you should actually follow them?" Do explain Mr. Shaikh how Intel should have been more strict in their guidance.
endocine said: So all of the settings that intel has been OK with, either tacitly or explicitly encouraging motherboard manufacturers to use.
endocine said: Why sell K SKUs and charge a premium for them if they are already at their maximum with intel baseline defaults, and why sell OC chipsets that allow this?
thestryker said: Intel hasn't done this much since motherboard manufacturers first started messing with default settings with IVB. Intel obviously isn't going to control other profiles and/or the ability to modify settings, but the default behavior is something they clearly need to.
- View All 68 Comments
Most Popular
- View Record
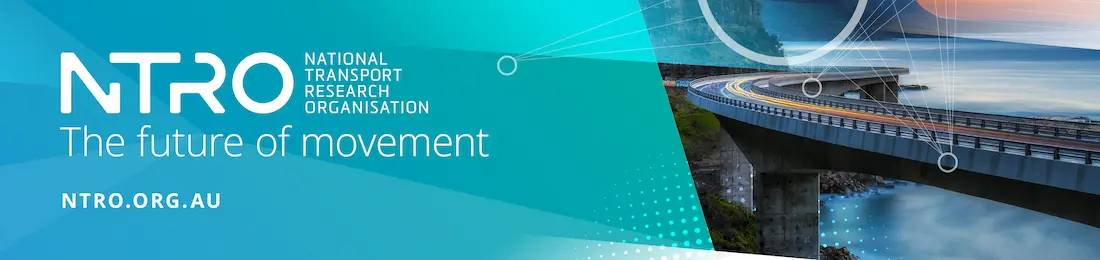
TRID the TRIS and ITRD database
IMPROVED METHODS FOR TRIP GENERATION
The acceptance by the UK Government of the SACTRA recommendation that induced traffic should be taken into account in road scheme assessment has focused modelling interest again on the methods by which the various components of induced traffic can be forecast. The possibility that increases in the number of trips may be among the relevant changes means that appropriate models of trip generation need to be considered. Drawing on recent research for the European Commission and the Department of Transport, as well as on other work, the paper addresses the problems of formulating and estimating appropriate models of trip generation, considering a number of the issues that arise: a) the connection between trip generation and the activity basis of travel demand; b) the contrasts between household and person-based models and between trip and tour modelling; c) the nature of the data that is available for modelling; d) the impact of the inclusion of slow modes in the trip rates; e) the ways in which accessibility can be measured and the incorporation of generation models in hierarchical logit model systems; f) the appropriate form of model to be used and its relationship to demand elasticity; and g) the distinction between generation and attraction modelling. It is shown that quite simple models can be developed which allow an efficient use of data to test the correlation between trip rate and accessibility and in particular that very simple methods can be used in 'pivot point' procedures to predict changes in trip rates resulting from changes in the 'level of service' offered by transport networks. Practical results are given from a number of studies to illustrate the sensitivity of trip generation to accessibility in various contexts. For the covering abstract, see IRRD E101013.
- Find a library where document is available. Order URL: http://worldcat.org/isbn/0860503054
PTRC Education and Research Services Limited
- Transportation planning methods Volume 11. Proceedings of seminar F held at PTRC European Transport Forum, Brunel University, England, 1-5 September 1997. Volume P415
- Publication Date: 1997-9
- Features: References;
- Pagination: p. 207-22
Subject/Index Terms
- TRT Terms: Accessibility ; Conferences ; Demand ; Forecasting ; Households ; Improvements ; Mathematical models ; Measurement ; Methodology ; Planning ; Transportation ; Travel ; Travelers ; Trip generation
- ITRD Terms: 9139 : Accessibility ; 8525 : Conference ; 285 : Demand (econ) ; 132 : Forecast ; 663 : Generated traffic ; 309 : Household ; 9108 : Improvement ; 698 : Journey ; 6473 : Mathematical model ; 6136 : Measurement ; 9102 : Method ; 143 : Planning ; 1755 : Road user ; 1155 : Transport
- Subject Areas: Planning and Forecasting; Transportation (General);
Filing Info
- Accession Number: 00765768
- Record Type: Publication
- Source Agency: Transport Research Laboratory
- ISBN: 0-86050-305-4
- Files: ITRD
- Created Date: Jul 1 1999 12:00AM

COMMENTS
The previous chapter introduces the four-step travel demand model (FSM), provides a real-world application, and outlines the data required to carry out each of the model steps. Chapter 10 focuses on the first step of the FSM, which is trip generation. This step involves predicting the total number of trips generated by each zone in a study area ...
The trip generation rates for both "work" and "other" trip ends can be developed using Ordinary Least Squares (OLS) regression (a statistical technique for fitting curves to minimize the sum of squared errors (the difference between predicted and actual value) relating trips to employment by type and population characteristics. ...
The ITE Trip Generation Manual presents a summary of the trip generation data that have been voluntarily collected and submitted to ITE. The trip generation database includes both vehicle and person trip generation for urban, suburban and rural settings. A Trip Generation web app—ITETripGen allows electronic access to the entire dataset with ...
Trip generation models require some explanatory variables that are related to trip-making behavior and some functions that estimate the number of trips based on these explanatory variables. ... and time spent transferring between vehicles. In some models, components of out-of-vehicle time are considered separately, while in others, a single out ...
To summarize, the model parameters for trip generation are the trip production and attraction rates, represented by Pratepk in Equation 4-7 and Aratepk in Equation 4-8. 4.4.3 Basis for Data Development When sufficient local data are available, best practice for the development of trip generation models is to estimate the model parameters from ...
Trip generation is the first step in the conventional four-step transportation forecasting process used for forecasting travel demands. It predicts the number of trips originating in or destined for a particular traffic analysis zone (TAZ). Trip generation analysis focuses on residences and residential trip generation is thought of as a function of the social and economic attributes of households.
Trip Generation Manual, 11th Edition. This new edition of the Trip Generation Manual enhances the 10th edition's modernized content, data set, and contemporary delivery - making it an invaluable resource. The 11th edition features: (1) All the latest multimodal trip generation data for urban, suburban and rural applications, (2) Reclassified ...
Of the 97 publications reviewed, 49 focused on trip generation based on land use characteristics and 44 on the use of advanced technologies for travel data collection, providing the main basis for the literature review. Fig. 1 presents the various keywords used by the researchers in studies related to trip generation.
Since trip generation models consists of three components: trip production, trip attraction, and trip balancing, calibration and validation checks should be conducted for each of the three components. Trip Productions. Once the initial trip production model is estimated, the trip productions by purpose are calculated and summarized by zone. ...
The main objective of this paper was to provide a comprehensive review of the trip generation model associated with land use characteristics and advanced technologies in travel data collection. Further, various modelling approaches used in the literature were examined. Though socio-demographic, built environment, and land use characteristics ...
Trip Generation. Trip generation is the first step in the FSM model. This step defines the magnitude of daily travel in the study area for different trip purposes. It will also provide us with an estimate of the total trips to and from each zone, creating a trip production and attraction matrix for each trip's purpose. Trip purposes are ...
Trip Generation Introduction. Trip Generation is the first step in the Sequential Demand Modelling arrangement which is also called as the Four Step Transportation Planning Process(FSTP) as mentioned earlier. In order to carry out modelling, the variable consists of total number of person-trips generated by a zone as a dependent variable and ...
Trip Generation. Trip generation involves predicting the total number of trips that are produced by and attracted to each zone in the modelled region. This is generally done using different parameters for each travel purpose, scenario and associated time horizon defined in the model setup. The following code sample is taken from the Toowoomba ...
This chapter describes the second step, trip distribution, of the four-step travel demand modeling (FSM). This step focuses on the procedure that distributes the trips after trip generation has been modeled, meaning after trips generated from or attracted to each zone in the study area are understood. The input for this step of FSM is the ...
Trip Generation Handbook. The principal objectives of Trip Generation Handbook (or the Handbook) are: (1) to provide guidance in the proper techniques for estimating trip generation, both person and vehicle, for potential development sites in urban, suburban, and rural settings; The techniques use data presented in Trip Generation Manual (or the Manual) and in appendices of this Handbook.
The Trip Generation Manual, 10th Edition includes: (1) Volume 1, Desk Reference: Provides a detailed description of new urban and person-based trip data, key instructional information, sample plots, and identifies significant changes from the previous edition. (2) Volume 2, Land Use Data Plots: Includes a complete set of land use descriptions ...
During the development Trip Generation Manual, 10th Edition, an online data submission system was created to facilitate an improved process for submitting data to ITE to be considered for inclusion in its trip generation database. The online data collection site is available at www.itedatasubmission.org. A hard-copy trip generation data form is ...
Trip Generation Data Plots - Modal. Click to download in PDF. 000s - Port and Terminal - Modal Data Plots 1. 200s - Residential - Modal Data Plots. 300s - Lodging - Modal Data Plots. 400s - Recreational - Modal Data Plots. 500s - Institutional - Modal Data Plots. 600s - Lodging - Modal Data Plots. 700s - Office - Modal Data Plots.
Estimate the number of trips a new 4,500 sq ft McDonald's restaurant with a drive-through window will generate during the restaurant's weekday morning peak h...
avoid splitting a complex HBW tour into home and non‐home based components, due to the loss of explanatory power for the non‐home based trips, and the loss of consistency between the choices made ... trip generation stage the model can afford to be expansive in terms of the variables used to cross‐ classify the households, it is also ...
Suggested Citation:"Chapter Two - Description of Air Cargo System Components." National Academies of Sciences, Engineering, and Medicine. 2017. Estimating Truck Trip Generation for Airport Air Cargo Activity. Washington, DC: The National Academies Press. doi: 10.17226/24848.
That part will feature the company's next-generation general-purpose cores and a revamped GPU. Huawei reportedly hopes the new Kirin will compete against Apple's M3 in multi-thread workloads.
Trip Generation Handbook. The principal objectives of Trip Generation Handbook, 3rd Edition (the Handbook) are to provide recommendations for proper techniques for estimating trip generation, both person and vehicle, for potential development sites in urban, suburban, and rural settings; standardization of trip generation data collection efforts; and ethics and objectivity in the use of Trip ...
Golden generation The team and the country will need Shin's touch more than ever after the Asian Cup, whether or not the squad wins the coveted trip to the Paris Olympics. Editorial Board (The ...
Intel issues a state regarding stability issues concerning 13th and 14th Generation CPUs on 600-and 700-series motherboards made by respective manufacturers.
Governor Kathy Hochul today announced a historic agreement as part of the FY 2025 Enacted Budget to address New York's housing crisis by increasing the housing supply, promoting affordability, strengthening protections for New York renters and homeowners, and combatting bias and discrimination in housing.
IMPROVED METHODS FOR TRIP GENERATION. The acceptance by the UK Government of the SACTRA recommendation that induced traffic should be taken into account in road scheme assessment has focused modelling interest again on the methods by which the various components of induced traffic can be forecast. The possibility that increases in the number of ...