
An official website of the United States government
The .gov means it’s official. Federal government websites often end in .gov or .mil. Before sharing sensitive information, make sure you’re on a federal government site.
The site is secure. The https:// ensures that you are connecting to the official website and that any information you provide is encrypted and transmitted securely.
- Publications
- Account settings
Preview improvements coming to the PMC website in October 2024. Learn More or Try it out now .
- Advanced Search
- Journal List
- Cogn Res Princ Implic
- v.3; 2018 Dec

Mind-wandering rates fluctuate across the day: evidence from an experience-sampling study
Gabriel king smith.
1 The University of British Columbia, Vancouver, Canada
Caitlin Mills
2 The University of New Hampshire, Durham, USA
Alexandra Paxton
3 University of Connecticut, Storrs, USA
Kalina Christoff
Associated data.
The datasets supporting the conclusions of this article are available in the Open Science Framework repository, https://osf.io/es3gf/ .
Previous research has demonstrated reliable fluctuations in attentional processes during the course of the day. Everyday life experience sampling, during which participants respond to “probes” delivered at random intervals throughout the day on their mobile devices, is an effective tool for capturing such diurnal fluctuations in a naturalistic way. The existence of diurnal fluctuations in the case of mind-wandering, however, has not been examined to date. We did so in two studies. In the first study, we employed everyday experience sampling to obtain self-reports from 146 university students who rated the degree of free movement in their thoughts multiple times per day over five days. These time course data were analyzed using multilevel modelling. Freely moving thought was found to fluctuate reliably over the course of the day, with lower ratings reported in the early morning and afternoon and higher ratings around midday and evening. In the second study, we replicated these effects with a reanalysis of data from a past everyday experience-sampling study. We also demonstrated differences in parameter values for the models representing freely moving thought and two common conceptualizations of mind-wandering: task-unrelated thought and stimulus-independent thought. Taken together, the present results establish and replicate a complex pattern of change over the course of the day in how freely thought moves, while also providing further evidence that freedom of movement is dissociable from other dimensions of thought such as its task-relatedness and stimulus-dependence. Future research should focus on probing possible mechanisms behind circadian fluctuations of thought dynamics.
Electronic supplementary material
The online version of this article (10.1186/s41235-018-0141-4) contains supplementary material, which is available to authorized users.
Significance
Mind-wandering has been linked to crucial parts of our daily lives, including learning, affect, and job productivity. While a number of studies have examined mind-wandering rates in everyday life, an implicit assumption has been that the rate is constant over the course of the day. The current research provides the first evidence suggesting that the extent of free movement in thought fluctuates reliably throughout our day. Thoughts appear to be most constrained in the morning and peaked in freedom of movement midday, increasing throughout the morning, decreasing throughout the afternoon, and finally increasing again in the evening. These findings may have important implications for education and the workplace, since freely moving thought could facilitate performance on tasks that require flexibility of thought (e.g. brain storming, solving novel problems), while leading to performances decrements on tasks that require stability of thought (e.g. algebra, sustained attention). Class and work schedules that adapt to the diurnal patterns of thought may lead to improved efficiency; future work should be done to explore this potential.
The last decade has seen a remarkable surge into mind-wandering research alongside its educational, clinical, and everyday life implications (Callard, Smallwood, & Margulies, 2012 ; Marchetti, Koster, Klinger, & Alloy, 2016 ; Mills, Raffaelli, Irving, Stan, & Christoff, 2017 ; Valdez, Ramírez, & García, 2014 ). Although there is no currently agreed-upon definition of mind-wandering (Christoff et al., 2018 ; Christoff, Irving, Fox, Spreng, & Andrews-Hanna, 2016 ; Irving, 2016 ; Seli et al., 2018 ; Seli, Risko, Smilek, & Schacter, 2016 ), there are a number of dimensions of thought that are being investigated for their presumed connection to mind-wandering. Task-unrelatedness and stimulus independence (i.e. cognition with little/no relation to external events; Schooler et al., 2011 ) have received the lion’s share of researchers’ attention so far (Mills, Raffaelli, et al., 2017 ; Seli et al., 2018 ). An additional dimension—freedom of movement in thought (i.e. the level of constraints on thought as it unfolds over time)—has more recently been highlighted as an important feature of mind-wandering (Christoff et al., 2016 ) and has been shown to be empirically dissociable from task-unrelatedness and stimulus-independence (Mills, Raffaelli, et al., 2017 ). One as-yet-unexplored question, however, is whether thought fluctuates systematically across the day along dimensions relevant to mind-wandering. Here, we provide the first such empirical investigation.
Until recently, a common approach in mind-wandering research was to implicitly equate mind-wandering with task-unrelated thought; however, this practice is no longer tenable due to recent developments in theoretical work on this topic (Christoff et al., 2018 ; Seli et al., 2018 ). Instead, mind-wandering is increasingly being recognized as a phenomenon that has so far evaded a one-to-one correspondence with any particular dimension of thought (Christoff et al., 2018 ). Although no consensus has yet been reached as to how mind-wandering relates to freedom of movement in thought, task-unrelatedness, and stimulus-independence, previous work upholds the relevance of these dimensions to understanding mind-wandering and its implications for everyday life.
In the present work, we chiefly focus on freedom of movement in thought as a dimension of thought relevant to mind-wandering and we offer (to our knowledge) the first empirical examination of its daily fluctuations (Study 1). We also examine how its diurnal fluctuations compare to the fluctuations of task-relatedness and stimulus-independence (Study 2) to present a fuller picture of the different dimensions of thought that have been linked to mind-wandering so far.
Our focus on freedom of movement stems from the potential ability of this dimension to conceptually distinguish between streams of thought that are more constrained (such as ruminative or goal-directed thoughts) and spontaneously unfolding streams of thought—an experience that is often intuitively considered to occur when the mind wanders. Within the Dynamic Framework of Thought (Christoff et al., 2016 ) which emphasizes how thoughts unfold over time, different sources of constraints—for example, automatic (e.g. habits, affect, salient distractors) and deliberate constraints (e.g. goal-directed focus)—dynamically influence the way thoughts unfold over time. In this model, some thought streams are more constrained (or focused on a particular topic) while other thought patterns are more “free,” i.e. they are less likely to be focused on a particular topic and will tend towards greater content variability over time (Mills, Herrera-Bennett, Faber, & Christoff, 2018 ). For example, night-time dreaming is considered to have low levels of constraint and thus higher degrees of freedom that are less bound to reality, compared to creative thinking which involves spontaneity but also elements of topical constraint; however, both can be considered forms of spontaneous thought (Christoff et al., 2016 ). Freedom of movement in thought would vary on a continuum from high to low based on the amount of net constraints that operate on the dynamic flow of thought at any moment, which can come from a variety of sources including (but not limited to) conscious or latent goal pursuits, habits, as well as perceptual or emotional demands.
Thoughts that are considered to have lower levels of constraint – i.e. “freely moving” thoughts – can be distinguished from other thought streams such as focused goal-directed thought and ruminative thoughts (which are influenced by imposing higher levels of constraints). Task-relatedness, despite being the most common thought dimension examined in mind-wandering research so far, does not distinguish whether a train of thought unfolds more spontaneously or in a more constrained manner. For example, rumination occurs in a constrained and fixated manner by definition (Nolen-Hoeksema, Wisco, & Lyubomirsky, 2008 ), which contrasts to the phenomenological experience of daydreaming; yet this contrast cannot be captured by the off-task dimension alone. Because of these distinctions, freedom of movement in thought seems to have particular relevance to mental processes in everyday life, where stimuli and tasks are much less well-defined than in an experimental context.
The majority of empirical studies on mind-wandering to date have taken place in a single session of either experience sampling in the laboratory (Franklin, Smallwood, Zedelius, Broadway, & Schooler, 2016 ; Krawietz, Tamplin, & Radvansky, 2012 ; Mills & D’Mello, 2015 ; Phillips, Mills, D’Mello, & Risko, 2016 ; Smallwood, Fitzgerald, Miles, & Phillips, 2009 ; Smallwood, Nind, & O’Connor, 2009 ; Stawarczyk, Majerus, Maj, Van der Linden, & D’Argembeau, 2011 ; Unsworth & McMillan, 2013 ) or during a single resting state functional magnetic resonance imaging (fMRI) scan (Ellamil et al., 2016 ; Fox, Nijeboer, Solomonova, Domhoff, & Christoff, 2013 ; Fox, Spreng, Ellamil, Andrews-Hanna, & Christoff, 2015 ; Kucyi & Davis, 2014 ; Mills, D’Mello, & Kopp, 2015 ; Phillips et al., 2016 ). However, because many studies are conducted between the hours of 09:00 and 17:00, they may be missing out on consistent variability in the daily cycle by collapsing results over the entire day.
The possibility that mind-wandering rates (as assessed by any of the three dimensions of thought mentioned above) may be influenced by daily cycles has been relatively ignored, despite a large body of work suggesting that time-of-day influences attention (as assessed via vigilance and selective attention tasks) as well as a wide array of cognitive faculties (Carrier & Monk, 2000 ; Folkard & Monk, 1987 ; Gabehart & Dongen, 2017 ). Much research in this field has been devoted to charting performance on attention-demanding cognitive tasks over the course of the day and has been successful in identifying a daily fluctuation pattern that closely parallels the rise and fall of core body temperature. Coincidentally, in parallel with the rising popularity of research on mind-wandering, analysis techniques that expand beyond simply averaging to include dynamic properties over time have also gained popularity in recent years (Allison, 2014 ; Dziak, Li, Tan, Shiffman, & Shiyko, 2015 ; Singer & Willett, 2003 ).
One way to address this gap in the literature is by assessing subjective ratings of thought over time in the real world: participants’ natural everyday life setting. So-called everyday life experience-sampling studies probe at random times throughout the day, typically using a mobile device to allow participants to answer questions (Franklin et al., 2013 ; Jazaieri et al., 2016 ; Killingsworth & Gilbert, 2010 ; McCormick, Rosenthal, Miller, & Maguire, 2017 ; McVay, Kane, & Kwapil, 2009 ; Song & Wang, 2012 ; Spronken, Holland, Figner, & Dijksterhuis, 2016 ). This methodology is frequently used to assess mental states over a period of time, yet longitudinal changes are often ignored in the subsequent analyses.
The present study
Here we investigate the patterns of mind-wandering across the day in two experience-sampling studies. Study 1 focuses specifically on freely moving thought: participants were probed randomly throughout the day for five days on their cell phones as they went about their regular routines. We hypothesized that ratings of freely moving thought would significantly fluctuate throughout the day.
In Study 2, we present a new analysis of a previously published experience-sampling dataset (Mills, Raffaelli, et al., 2017 ) in an attempt to replicate findings from Study 1. We also ask whether different conceptualizations of mind-wandering (i.e. freely moving thought, task-unrelated thought, and stimulus-independent thought) exhibit different fluctuations over the course of the day. This idea is inspired by Mills, Raffaelli, et al.’s ( 2017 ) original finding of low overall correspondence between these distinct forms of mind-wandering; the present study, then, builds on that work to explore whether they share similar dynamics.
In the interest of open science, we have made our code and data freely available on the Open Science Framework ( https://osf.io/es3gf/ ), and our code is additionally available on GitHub ( https://github.com/galagon/mind-wandering-fluctuations ).
Participants
A total of 144 participants were recruited from a large public Canadian university and were compensated for their participation with class credit. All instructions were given in English. The language of the participants was assumed to be the same: proficiency in English is an enrollment requirement for the university and only enrolled students were eligible to participate.
All study procedures were reviewed and approved by the UBC Behavioural Research Ethics Board.
The study included an in-lab component and an experience-sampling component. First, participants underwent a 30-min training session in the lab before beginning, starting with the completion of an informed consent form and a ~ 20-min learning portion. This portion included detailed verbal instructions given via a video-recorded slideshow. The video explained the procedure, gave detailed examples for all relevant definitions, and was paused periodically to enable the experimenters to answer participants’ questions about the study and ask participants to generate novel examples of different kinds of thought. Portions of the exact script used in the video are included in Appendix 1 . Finally, before leaving the lab, participants were asked to keep their phone near them at all times and respond to as many probes as possible unless it was dangerous to do so (e.g. driving).
Participants were prompted to answer probes 20 times per day for five days (yielding up to 100 total responses to probes per participant) via URL links sent directly to their mobile phones in a text message. Each probe included the question “Was your mind moving about freely?”, which was answered on a scale from (1) Not at all to (7) Very much . If it was the first probe answered that day, participants were also asked what time they had awoken that day and what time they had begun to sleep the previous night. Probes were answered from Tuesday through Saturday.
A total of 10,287 responses were collected (M = 71.4 responses per participant, SD = 21.0). Similar to Mills, Raffaelli, et al. ( 2017 ), participants who answered 60 out of 100 probes or fewer (36 participants out of 144) were dropped from the analyses to remove participants who did not follow instructions to answer sufficient thought probes for analysis. The final sample was composed of 108 participants who answered an average of 80.2 probes (SD = 14.1).
Formal and accurate a priori power calculations proved difficult due to the lack of information regarding effect sizes of daily changes, particularly when it came to models that incorporate multiple time terms and mixed effects as described below. In light of this, we aimed to gather as many participants as we could within a limited time window in order to maximize power, resulting in the sample size of 144 participants initially and 108 participants after exclusions. However, post-hoc observed power estimations are reported in the discussion to help with the planning of future research.
To ensure that our results were not driven by exclusion criteria, all analyses reported below were repeated with the complete dataset (i.e. no participant removal). The pattern of results remained unchanged with one exception in Study 2, noted below.
Data preparation
To compress the data into bins, the time at which each probe was received was rounded down to the hour and participants’ ratings of freely moving thought were averaged for each hour of the day, collapsing across different days. For some hours of the day, relatively few probe responses were logged (see Table 1 for a summary of hours with at least one probe response). Fewer than one-quarter of all participants answered a single probe during the hours of 06:00 and 07:00 (all times local and based on the 24-h clock); because of this, data pertaining to those hours were excluded from all analyses. Both Table 1 and Fig. 2 show the average freely moving thought rating for each hour of the data.
Descriptive statistics of the original (Study 1) and reanalyzed (Study 2) datasets

Freedom-of-movement in thought ratings (1–7) ( a ) averaged for each hour of the day and ( b ) averaged for each hour since waking. Error bars represent the standard error. Red lines represent the best-fit cubic models
Approach to data analyses
Our central research question was to determine if ratings of freely moving thought fluctuate in reliable patterns over the course of the day. To answer this question, we modeled ratings of freely moving thought with the hypothesis that they would vary as a function of time. We used a mixed-effect multilevel modelling approach to test this hypothesis because: (1) it assesses change over time while preserving between-participant differences in baseline ratings (intercept) and estimated relationships (slope; Fuller-Tyszkiewicz et al., 2017 ); and (2) it is robust to missing data (Mirman, 2016 ). Below, we describe our analytical approach using current best-practices for growth curve modeling; interested readers can find more detail in Mirman ( 2016 ), Mirman, Dixon, and Magnuson ( 2008 ), and Singer and Willett ( 2003 ).
We tested the patterns of freely moving thought ratings by constructing a mixed-effects model with first- through third-order orthogonal polynomial terms (for comparative examples of the three orders of polynomial models; see Fig. 1 ). All polynomial terms were orthogonalized to avoid multicollinearity and to ensure independence from one another (see Mirman, 2016 ). The significance of each term would shed light on a specific pattern, though none are mutually exclusive (see Fig. 1 ):
- the linear term (first order) would represent a general increase or decrease in freely moving thought over the day;
- the quadratic term (second order) would fit the data if ratings were higher or lower in the middle of the day as compared to morning and evening; and
- the cubic term (third order) would capture trends that changed direction twice over the day—for example, if the ratings rose throughout the morning, fell in early afternoon (i.e. first change in direction), and rose again through the evening (i.e. second change in direction).

Examples of polynomial models representing change across time. The black line represents no change across time (intercept-only); the blue line represents linear change; the green line represents quadratic change; the red line represents cubic change
We used polynomials to model change across time for three reasons, aside from such models being commonly used in the analysis of temporal dynamics (Hartmann et al., 2016 ; Mirman et al., 2008 ; Papageorghiou et al., 2014 ; Shin, 2012 ; White, Xie, Thompson, Loeber, & Stouthamer-Loeber, 2001 ). First, they can easily be orthogonalized with respect to one another, allowing us to interpret the individual contributions of each polynomial without the issue of collinearity. Second, they represent increasingly complex forms of change over time, which is important as we did not believe that simple patterns of change (e.g. linear) were likely given the literature on daily fluctuations of other cognitive variables (Burke, Scheer, Ronda, Czeisler, & Wright, 2015 ; Fimm & Blankenheim, 2016 ; Folkard & Monk, 1987 ; Giambra, Rosenberg, Kasper, Yee, & Sack, 1989 ; Goel, Basner, Rao, & Dinges, 2013 ; Riley, Esterman, Fortenbaugh, & DeGutis, 2017 ; Silva, Wang, Ronda, Wyatt, & Duffy, 2010 ; Valdez et al., 2005 ).
Finally, modeling data with these three polynomials served as a first step in understanding the dynamics of mind-wandering using some of the simplest and most commonly used forms of change found in other diurnal cognitive studies. Given the lack of previous work in this domain, we had no reason to suspect that any more complicated time-varying forms of change would provide a better fit; therefore, the choice of more specific and nuanced models would have been arbitrary. While some past studies have attempted to precisely and mechanistically model daily change in a cognitive outcome variable (Jewett & Kronauer, 1999 ), such models were based upon a deep and established literature that does not yet exist for freely moving thought.
Model construction
All analyses were performed in R (R Core Team, 2017 ). Models were constructed using the lme4 package (Bates, Mächler, Bolker, & Walker, 2015 ) and parameters were tested for significance with the lmerTest package (Kuznetsova, Brockhoff, & Christensen, 2017 ). All variables were centered and standardized before entry into the model, allowing us to interpret the resulting standardized estimates as effect sizes (Keith, 2005 ).
We constructed a model predicting freely moving thought ratings using each orthogonal polynomial as fixed effects without interaction terms. We used participants as our sole random intercept with the maximal slope structures that permitted model convergence (Barr, Levy, Scheepers, & Tily, 2013 ). All models were estimated using unrestricted maximum likelihood estimation, with an unstructured covariance structure.
For completeness, we also compared nested models to identify which model provided the best “fit” for the data. In this step, we constructed hierarchical models with successively higher-order time terms (i.e. intercept-only, linear, quadratic, and cubic) and tested them for improved fit over the previous iteration using chi-square tests. Ultimately, the full model (i.e. cubic model) best accounted for the data. Results from these hierarchical comparisons are reported in our supplementary materials (Additional file 1 ).
Model interpretation
We would find support for our hypothesis that ratings of freely moving thought fluctuate reliably over the course of the day if our model found significance for any time parameter (i.e. linear, quadratic, or cubic; see supplementary materials (Additional file 1 ) for graphical representations of each fitted model). Model parameter values can generally be interpreted as follows:
- positive linear parameters would indicate that ratings increased throughout the day;
- positive quadratic parameters would indicate that ratings were higher at the beginning and end of the day compared to the middle; and
- positive cubic parameters would indicate that ratings increased at the beginning of the day, decreased in the middle of the day, and increased again at the end of the day.
Standardization of ratings meant that the resulting coefficients of the model represent standardized (β) not raw (B) coefficients. This allows different predictors to be directly comparable (Keith, 2005 ). For example, if the coefficient for cubic change is higher than that for the linear change, it indicates the influence of the cubic parameter is greater or that the data conform more to a cubic form of change than a linear one.
Overall, freely moving thought ratings trended towards the center of the scale with a mean rating of 4.11 ( SD = 1.92). As hypothesized, the results indicate that freedom-of-movement in thought did indeed fluctuate in a reliable pattern over the course of the day, as both the quadratic and cubic terms were significant. Table 2 shows the values and significance tests for each of the model parameters.
Fixed effects of the optimal (cubic) model for freely moving thought ratings (original dataset)
β standardized regression coefficient
a Significant at α = 0.05
Figure 2 a shows this change over the course of the day. Ratings of freely moving thought were lowest in the morning (in the 08:00 h) and steadily increased until midday, before declining again. The significance of the negative quadratic term is representative of the parabolic quality of daily change in which ratings start and end low, peaking somewhere in between. The presence of the positive cubic term elaborates on this simple relationship: the peak occurs early in the day (around noon) and is followed by a decreasing pattern that changes direction and increases in the evening.
The data were also analyzed with respect to freely moving thought ratings as a function of hours awake in the day, using the self-reported wake times of participants (Fig. 2 b). A similar cubic relationship was found, although the quadratic term became non-significant. Visual inspection of the form of change indicates a high degree of similarity in the two patterns, strengthening the above findings by indicating that at least the cubic pattern of change cannot be explained by variable sleep habits among participants confounding freely moving thought ratings (for full results, see Appendix 2 ).
Results from Study 1 supported our hypothesis that rates of mind-wandering change in complex patterns throughout the day. We found that participants’ thoughts were more constrained in the morning and gradually became more freely moving across the beginning of the day, peaking around noon, before falling in the afternoon and then rising again in the evening. These findings provide initial support for the idea that mind-wandering dynamically fluctuates in our everyday lives, which is important given its relationship to educational outcomes (Mills, Graesser, Risko, & D’Mello, 2017 ; Mrazek et al., 2017 ; Pachai, Acai, LoGiudice, & Kim, 2016 ; Seli, Wammes, Risko, & Smilek, 2016 ; Sousa, Carriere, & eSmilek, 2013 ; Valdez et al., 2014 ) and workplace productivity (Dane, 2011 ; Dust, 2015 ; Hyland, Lee, & Mills, 2015 ).
Study 2: reanalysis of Mills, Raffaelli, et al. ( 2017 )
Demonstrating replicability of the results from Study 1 would be highly desirable given the novelty of the research question and the exploratory nature of interpreting polynomial models assessing changes over time. Towards this goal, we conducted a novel analysis of data from Mills, Raffaelli, et al. ( 2017 ), a published experience-sampling study in everyday life that included probe timestamp information that had never been analyzed. Reanalyzing this dataset also enabled us to address the question of whether three distinct conceptualizations of mind-wandering (freely moving thought, task-unrelated thought, and stimulus-independent thought) displayed dissociable fluctuations across the day.
A complete description of the method from the original study can be found in Mills, Raffaelli, et al. ( 2017 ), but we provide a very brief overview here. The methodology was identical to that used in the Study 1 with four exceptions. First, the sample included 226 people initially, with 165 participants retained after removing those who answered 60% or fewer probes completely. Second, probes were given 10 times a day for a period of 10 days, yielding a maximum of 100 probes per participant (the same per-participant maximum as Study 1). Third, the questions concerning sleep and wake time were not included; instead, every probe asked participants to rate their task-unrelated thought (“Were you thinking about something other than what you were doing?”) and stimulus-independent thought (reverse-coded; “Were you aware of your surroundings?”) on a scale of 1 to 7. (Probes also included other questions that were not relevant to the present study; see Mills, Raffaelli, et al., 2017 .) Fourth, participants received probes all days of the week but only between the hours of 08:00 and 23:00 (all times local and in the 24-h clock).
Data preparation and analysis
Similar to the analytical approach in Study 1, probes were rounded down to the hour and aggregated by person and hour. Unlike the original study, the probed hours were in the range of 08:00 to 23:00 (Mills, Raffaelli, et al., 2017 ). Only hour 23:00 was removed as it contained responses from < 25% of participants (Table 1 ). Figure 3 displays the mean ratings for each hour of the day for each dimension.

Thought dimension ratings displayed by the hour of the day. Error bars represent standard errors. Red lines represent predictions of the optimal model for each dimension (cubic in all cases): ( a ) freedom-of-movement in thought, ( b ) task-unrelatedness of thought, ( c ) stimulus-independence of thought
Statistical analyses were conducted with the same packages in R (lme4, Bates et al., 2015 ; lmerTest, Kuznetsova et al., 2017 ), the same fixed and random effect constraints, the same maximum likelihood effect estimation, and the same unstructured covariance structure as used with the original data. Again, all data were standardized before entry in the model, resulting in standardized (β) rather than unstandardized (B) coefficients. As with Study 1, hierarchical models were created with increasing orders of polynomial time-varying factors and compared on goodness of fit; the results of these comparisons as well as graphics representing the fitted values for each model can be found in our supplementary materials (Additional file 1 ).
The average rating (per participant) for each of the three definitions clustered toward the center of the scale: the mean freely moving thought rating was 4.03 (SD = 1.73), the mean task-unrelated thought rating was 4.08 (SD = 1.74), and the mean stimulus-independent thought rating was 4.19 (SD = 1.64).
We present each model’s results below. For simplicity, we do not include test statistics in the text; instead, model results are provided in Tables 3 , ,4, 4 , ,5 5 and and6 6 .
Fixed effects of the optimal (cubic) model for freely moving thought ratings (reanalyzed dataset)
β standardized regression coefficient
Fixed effects of the optimal (cubic) model for task-unrelated thought ratings (reanalyzed dataset)
Fixed effects of the optimal (cubic) model for stimulus-independent thought ratings (reanalyzed dataset)
Fixed effects of the optimal (cubic) model for the combined model (reanalyzed dataset)
β standardized regression coefficient, TU task-unrelatedness, SI stimulus-independence
Freely moving thought (Table 3 )
Findings for freely moving thought closely replicated results from Study 1. As in the original dataset, both the quadratic and cubic terms reached significance, but the linear term did not. Notably, when the analyses were run without the removal of participants who did not answer a sufficient number of probes, the linear term became significantly positive, likely as the result of higher freedom-movement ratings in the latest hours (for more, see supplementary materials (Additional file 1 ).
Task-unrelated thought
Ratings of task-unrelated thought showed linear change over the course of the day, but no other time-varying model parameters achieved significance (Table 4 ). The positive linear parameter indicates that the ratings increased significantly between the beginning and the end of the day. This contrasts with ratings of freely-moving thoughts, which showed quadratic and cubic change but not linear.
Stimulus-independent thought
Ratings of stimulus-independent thought showed similar patterns of change over the day as freely moving thought, exhibiting quadratic and cubic fluctuation without linear change (Table 5 ).
Comparing different dimensions of thought
Mills, Raffaelli, et al. ( 2017 ) found that freely moving thought could be distinguished from content-based definitions of mind-wandering (i.e. task-unrelated thought and stimulus-independent thought). In the present study, we provide a novel test of this claim by assessing whether these three dimensions of thought exhibit differential patterns of change across the day. Although we found that two of the dimensions (freedom-of-movement and stimulus-independence) showed similar patterns of change with the same model parameters being significant for each dimension, the individual slopes that make up the equations may be significantly different. In other words, the steepness of the curves may differ.
We tested this possibility by combining responses to all three questions in a dataset with a three-level categorical variable that we call thought dimension (Table 6 ). We then created a cubic model that included the main effect of thought dimension and its interactions with each of the three time terms. A non-significant interaction between thought dimension and a time term indicates that the given slope does not differ substantially between the three dimensions of thought, whereas a significant interaction indicates that the dimensions have dissociable slopes.
By default, the lmer function of the lme4 package in R uses treatment contrasts, meaning that it treats interactions involving categorical variables as pairwise comparisons between the first entered level of the variable (in this case, ratings of freely moving thought) and each level after that (task-unrelatedness and stimulus-independence). An analysis comparing task-unrelatedness and stimulus-independence directly can be found in the supplementary materials (Additional file 1 ), though we chose not to include it in the main text both to minimize the number of tables and to focus on our main research question: does freely moving thought have a distinct daily pattern compared to the dominant, content-based conceptualizations of mind-wandering?
Although there were no significant interactions between dimensions of thought and the linear or cubic terms, the results show an interaction between the quadratic term and the dimension variable with both comparisons reaching significance. The quadratic slope for ratings of freely moving thought was significantly steeper (i.e. more negative) than that of task-unrelated thought yet significantly shallower (i.e. less negative) than that of stimulus-independence thought. This pattern can be observed through visual inspection of the data (Fig. 3 ): freely moving thought ratings experience a rise and fall pattern over the course of the day and peak close to the middle of the day, a relationship that is exaggerated for stimulus-independent thought and absent for task-unrelated thought.
These differences in changes across the day further highlight the dissociability of the three dimensions and indicate that they may be associated with only partially overlapping neural processes or may arise from distinct constraints on agent-environment interactions.
Weekend-only analysis
The greatest advantage of everyday life experience sampling—the fact that ratings come from participants living their daily lives and not conducting artificial laboratory tasks—comes with its own set of limitations. Without controlling the conditions under which participants answer, it is difficult to know whether the observed fluctuations are caused by intrinsic mechanisms such as diurnal rhythms or whether they are simply the product of the activities in which participants were more or less likely to be engaged in at different points in the day. For example, ratings of freely moving thought were highest around midday, a time when the study’s participants—undergraduate students—would be more likely to be in the middle of classes as compared to the evening or early morning. Aside from classes, this pattern could be driven by participants taking lunch breaks around the noon hour or, conversely, by exhaustion after long periods of intensive effort exerted in morning classes.
Although the primary goal of the study was to determine whether reliable daily fluctuations exist and not to determine their source, the reanalyzed dataset affords the opportunity to examine weekdays and weekend days separately. This exploratory analysis rests on the tentative assumption that daily activities on weekdays (e.g. classes, work) are scheduled differently than weekends for most participants. Thus, if daily fluctuations in thought are identical across weekdays and weekend days despite those days containing different sets of activities, there is evidence that activities alone may not account for these daily patterns of thought.
After removing probes answered during weekdays, 4305 of the original 12,997 probes (33.12%) remained. In order to keep the results comparable, the same participants in the full analyses were used for the weekend-only analyses, regardless of the number of probes answered after the removal of weekday probes. The analysis was limited to the reanalyzed dataset from Mills, Raffaelli, et al. ( 2017 ) due to an insufficient number of probes answered over weekend days in the dataset from Study 1.
All model results are available in Appendix 3 . For brevity and clarity, we do not include most test statistics in the text, but graphical representations of the data and optimal cubic models can be seen in Fig. 4 . The model for freely moving thought revealed a highly similar pattern of results, but neither the quadratic nor the cubic term quite reached statistical significance (quadratic: p = 0.056, cubic: p = 0.054; Fig. Fig.4a). 4 a). Although the lack of significance at α = 0.05 may indeed indicate a different pattern of fluctuation across the day, the loss of power accompanying a threefold reduction in the number of probes entered into the model may be an alternative reason for this disparity, especially given that the p values in question, while no longer significant, are still < 0.06. While we cautiously interpret the pattern for freely moving thought to be similar for the weekend-only analysis, future work with greater statistical power may be able to shed more light on this possibility.

Thought dimension ratings for only weekend probes displayed by the hour of the day. Error bars represent standard errors. Red lines represent predictions of the optimal model for each dimension (cubic in all cases): ( a ) freedom-of-movement in thought, ( b ) task-unrelatedness of thought, ( c ) stimulus-independence of thought
On the other hand, we were unable to replicate the findings for the two content-based dimensions of thought. In the model predicting task-unrelatedness of thought, no time-varying parameters reached significance. In the model predicting stimulus-independence of thought, the positive linear term reached significance and the positive cubic term no longer reached significance (although it trended toward significance in the same direction), while the quadratic term remained negative and significant.
We note, however, that visual inspection of the weekend-only data indicates a highly similar pattern for task-unrelatedness of thought (Fig. (Fig.4b). 4 b). This again suggests that the difference from the original analysis is possibly the result of power loss (though it is worth noting that in this case the linear term did not trend towards significance). The stimulus-independence data, on the other hand, does appear to exhibit a different relationship (Fig. (Fig.4c). 4 c). Visual inspection indicates that stimulus-independence scores are higher in the evenings, which would account for the increase in the strength of the linear parameter (since the scores at the end of the day are now higher than the those at the beginning) as well as the attenuation of the cubic parameter (since the scores remain comparatively stable after midday and do not dip in the afternoon or evening).
The reanalysis of Mills, Raffaelli, et al. ( 2017 ) in Study 2 provided three major conclusions. First, ratings of freely moving thought followed a pattern of quadratic and cubic change across the day with an absence of linear change, replicating the findings from Study 1. This pattern was also observed visually —although, crucially, it failed to reach statistical significance—when analyzing only weekend data; the compatible visual and statistical trends in the data, however, suggest that the lack of statistical significance may have been due to a severe drop in statistical power by focusing only on two out of seven days of the week.
Second, two dominant, content-based dimensions of thought were also found to fluctuate across the day. Stimulus-independent thought exhibited a form of change similar to freely moving thought, while task-unrelated thought showed a simple increase in ratings throughout the day. These patterns were less reliable when analyzing weekend-only data, with stimulus-independent thought in particular appearing to exhibit a different form of daily change.
Third, the three dimensions of mind-wandering were empirically dissociable based on their quadratic parameters. Specifically, stimulus-independent thought exhibited a much stronger rise-and-fall pattern of higher scores in the midday compared to the beginning and end of the day, in comparison with freely moving thought. On the other hand, such a pattern was completely absent for task-unrelated thought.
General discussion
Here, we presented—for the first time—evidence that thought patterns fluctuate across the day. Capturing the “wandering” mind by measuring freely moving thought, we probed people on their smartphones during their daily lives to understand the daily rhythms of thought in the “wild.” We found that freely moving thought occurs at lower rates early in the day, rises until midday, and declines gradually before rising once more in the evening. By reanalyzing a dataset from Mills, Raffaelli, et al. ( 2017 ), we were able to replicate these results and investigate novel questions about the dynamics of specific kinds of mind-wandering—in particular, we found that freely moving thought showed distinct daily patterns from two other dominant conceptualizations of mind-wandering. Such findings are consistent with a host of previous research showing that human cognition exhibits diurnal dynamics, not uniform properties over time.
What might drive diurnal dynamics?
Although these results provide novel evidence that mind-wandering does dynamically fluctuate throughout the day, they do not speak to why these changes occur. We describe a few possible—but not mutually exclusive—explanations for the patterns of change. However, we recognize that much more work is required to elucidate the mechanisms driving these results, and we present these as testable hypotheses for additional future work.
The first potential mechanism is autonomic arousal. Arousal may bias attentional selectivity by way of the locus coeruleus, a remarkably well-connected norepinephrinergic brain region (Aston-Jones & Cohen, 2005 ; Aston-Jones & Waterhouse, 2016 ; Gilzenrat, Nieuwenhuis, Jepma, & Cohen, 2010 ; Mather, Clewett, Sakaki, & Harley, 2016 ). Although a direct link between arousal and the freely moving thought conceptualization of mind wandering has not been established, Mittner, Hawkins, Boekel, and Forstmann ( 2016 ) recently suggested that levels of norepinephrine originating from the locus coeruleus may differentiate between mental states that are focused on a single subject (regardless of whether the subject is the task at hand) and “exploratory,” unstable states—similar to the distinction between constrained and freely moving thought.
Indeed, diurnal fluctuations in norepinephrine align well with the pattern of results found here: an increase early in the day, a lunchtime peak, and a gradual afternoon/evening decline (Fibiger, Singer, Miller, Armstrong, & Datar, 1984 ; Hansen, Garde, Skovgaard, & Christensen, 2001 ). Previously observed fluctuations do not map perfectly onto the cubic structure of mind-wandering observed in the present dataset, given that levels of norepinephrine generally do not increase over the course of the evening. However, both share noticeable similarities throughout the rest of the day. The same pattern exists for attentional performance as well (Carrier & Monk, 2000 ; Silva et al., 2010 ; Valdez et al., 2005 ), suggesting that arousal may be a common mechanism behind these daily changes.
The arousal-based account may also be able to partially explain a somewhat-paradoxical finding from our study: mind-wandering rates tended to be at their highest during the times when past studies have indicated attentional abilities are at their peak and they tended to be at their lowest during the times when attentional performance is purported to suffer (Carrier & Monk, 2000 ; Folkard & Monk, 1987 ; Valdez et al., 2005 ). This co-fluctuation seems counter-intuitive, as the relationship between mind-wandering and performance on sustained attention tasks is often considered antithetical (McVay & Kane, 2009 ; Smallwood, Fitzgerald, et al., 2009 ; Stawarczyk et al., 2011 ). However, it is important to note that the majority of the studies that have found evidence of such an antithetical relationship have used task-unrelated thought as their definition of mind-wandering (oftentimes quite literally operationalized as the inverse of attentional performance); by contrast, freedom-of-movement of thought and attentional performance may not exhibit such a marked negative relationship. In light of this, the observed results would be perfectly in line with an arousal mechanism that impacts both attentional performance and thought dynamics but has no direct impact on the content of thought (or, at the least, not its task-relevance).
A second, simpler explanation for the observed daily fluctuations in mind-wandering may be circumstance or environmental pressure. Ratings of freely moving thought first peaked at the time of day when many participants (all of whom were students) would be in class and ratings dipped at times when they were would more likely to be home. It is therefore possible that the classroom environment may loosen constraints on thought or that other activities or states experienced at certain times of day produce the patterns seen here. Since we did not ask students to report their current activity at the time of each probe, this possibility cannot be completely discounted. However, findings for freely moving thought were partially replicated in a subset of the reanalyzed data that included only weekend days, suggesting that this pattern of change is likely independent of the day of the week, which one would not expect if it was driven by activities.
Finally, mind-wandering fluctuations may be tied to humans’ circadian rhythm in some other way. In two out of three dimensions of thought, we observed a rise-and-fall pattern that mirrors circadian body temperature changes that, in the past, have frequently been found to correlate with cognitive performance in a variety of domains (Carrier & Monk, 2000 ; Fimm, Brand, & Spijkers, 2016 ; Goel et al., 2013 ; Valdez, Reilly, & Waterhouse, 2008 ; Wright, Hull, & Czeisler, 2002 ). Although it would have been impractical to record temperature in an everyday life experience-sampling study, there is room for future experimental research to elaborate on the current findings by determining whether freely moving thought exhibits the same correlation with core body temperature as attention, spatial awareness, and other cognitive variables.
We recognize that our study is unable to answer these questions definitively. Perhaps some, all, or none of these explanations even work together: for example, arousal’s influence on mind-wandering could be dependent on current activity or level of motivation. As a result, we call for future work to collect the information that would be able to disentangle these effects, including concurrent measures of arousal, motivation, physiological states, and information about what participants are doing at the time of the probe responses. Such work should also continue to explore the dynamics of the three types of mind-wandering to see how, whether, and when they differ from one another.
Implications
This paper lays the groundwork for understanding daily fluctuations in mind-wandering—a topic that may have implications for shaping real-world policy. Indeed, there is already ample evidence linking one dimension of thought related to mind-wandering (task-unrelatedness) to affect (Killingsworth & Gilbert, 2010 ), creativity (Baird et al., 2012 ), and—perhaps most compellingly for public policy—impaired comprehension and classroom performance (Mills et al., 2017 ; Mrazek et al., 2017 ; Pachai et al., 2016 ; Seli, Wammes, et al., 2016 ; Sousa et al., 2013 ; Valdez et al., 2014 ).
As a result of the link between traditional task-unrelated mind-wandering and learning, educational policy has an enormous potential to be shaped by this and other mind-wandering research. The negative association between task-unrelated thought and text comprehension has been extensively replicated (Franklin, Smallwood, & Schooler, 2011 ; Mrazek et al., 2017 ; Pachai et al., 2016 ; Seli, Wammes, et al., 2016 ; Smallwood, McSpadden, & Schooler, 2008 ; Sousa et al., 2013 ). Additional work suggests that this relationship may be partially explained by mind-wandering’s interference with students’ ability to adaptively adjust their cognitive resources to the current task demands (Mills et al., 2017 ).
The dynamic fluctuations in mind-wandering found here raise the question of whether students’ schedules can or should be adapted around their cognitive cycles. For example, our findings suggest that early afternoon classes may coincide with peak rates of freely moving thought and stimulus-independence—perhaps making them poor times at which to hold class. However, it is currently unclear what an optimal schedule could be, given that past research has demonstrated learning deficits in early morning classes as well due to factors such as sleepiness (see Valdez et al., 2014 ).
A contrasting point of view is that mind-wandering is not a hindrance to all educational outcomes, particularly ones that require creativity (Baird et al., 2012 ; Dijksterhuis & Meurs, 2006 ; Ellamil, Dobson, Beeman, & Christoff, 2012 ; Pachai et al., 2016 ). From this standpoint, school administrators may actually wish to structure class times to take advantage of the benefits of mind-wandering (freely moving thought, in particular), not just avoid the downsides. For example, writing and artistic classes may benefit from being held around midday, when freely moving thought is highest and constraints are lowest. Classes that require a narrower focus of attention (e.g. STEM courses) might be more effective if they are held when freely moving and task-unrelated thought are at their lowest.
It is also important to consider how daily fluctuations in thought influence performance in the workplace. Industrial-organizational research has highlighted the detrimental effects of task-unrelated thought on workplace performance (Dane, 2011 ;Dust, 2015 ; Hyland et al., 2015 ) as well as its potentially positive effects (Dust, 2015 ; Hyland et al., 2015 ). In driving simulations, task-unrelated thought has been associated variously with poorer speed control (Baldwin et al., 2017 ; Yanko & Spalek, 2014 ), slower reaction times (Yanko & Spalek, 2014 ), and a lack of peripheral awareness (He, Becic, Lee, & McCarley, 2011 ). Similarly, in real-world correlational studies, this kind of mind-wandering has been associated with risky or aggressive driving patterns (Qu et al., 2015 ) and responsibility for car accidents (Galéra et al., 2012 ). Understanding the times of day we are most likely go off-task, have unconstrained thoughts, or be unaware of our surroundings, could be a critical consideration for occupational schedules as well.
Limitations and future directions
Of course, as an early and exploratory study, our work has a number of limitations and we see many of them are prime opportunities for future work. First, we asked people to introspectively report on their thoughts; participants’ introspective abilities have been the subject of much debate in the past (Hurlburt, Alderson-Day, Fernyhough, & Kühn, 2017 ; Nisbett & Wilson, 1977 ). However, replication of results across two independent datasets provides some evidence that individuals were responding reliably to the introspective questions. Additionally, extensive training and instructions were included during the training session to compensate for the novelty of the freely moving thought definition. Future work should strive to complement these studies by assessing the phenomenological experience of freely moving thought through its contents over time, as well as its objective signatures through physiological signals (Faber, Bixler, & D’Mello, 2018 ; Mills, Bixler, Wang, & D’Mello, 2016 ; Mills & D’Mello, 2015 ).
Second, we had no experimental control over participants, since the study was conducted in everyday life. This was necessary to maximize ecological validity but means that we had no way of knowing whether participants answered accurately and no way to compel them to answer rapidly. While this provided us with valuable insight into real-world processes, future work should complement naturalistic research (like the present study) with experimental designs that could shed light on processes, mediations, and causal mechanisms.
Third, in Study 1 only 71.4% of all probes were answered on average in the original dataset (80.2% after discarding low responders), leading to some variability in response rates across the hours of the day (see Table 1 ). This is particularly a problem for hour 23:00, as only 53 participants (fewer than half) answered a single probe during this time period, resulting in a high standard error. As a consequence, it is unclear whether the sharp drop in freely moving thought ratings from 22:00 to 23:00 represents a true effect, a problem made worse by the lack of any 23:00 probes in Study 2. We are nevertheless confident in the pattern of results leading up to 22:00 h, particularly the replicated finding that freely moving thought steadily increased throughout the morning.
Fourth, in order to ensure consistency with the existing literature, 7-point Likert scales were used to measure all three dimensions of thought. While this simplifies the process and allows participants to make quick and intuitive decisions, Likert scales have the disadvantage of being unmoored from common reference points. Put simply, even with verbal anchors, there is no objective meaning of a 4 out of 7 on the scale; as a result, each rating may mean something entirely different to each participant. Once again, we attempted to mitigate this by providing participants with extensive instructions. These instructions even included prompting participants to come up with their own examples of different levels of freely moving thought, ensuring that their ideas were as close as possible both to their cohort’s perceptions and to the experimenters’ expectations.
Fifth, past studies have found evidence that task-unrelated thought may demonstrate fluctuations (Carciofo, Du, Song, & Zhang, 2014 ; Giambra et al., 1989 ) similar to those we observed for freely moving thought or stimulus-independent thought. We did not find similar patterns for task-unrelated thought in the present study, but this may be due to significant methodological differences among the studies. Giambra et al. ( 1989 ) conducted a sleep-deprivation study intended more to explore natural circadian rhythms of mind-wandering in isolation than fluctuations in real-world environments. Because they used a lab-based task, their participants were judged on whether they were paying attention to a particular narrow assignment they were given. On the other hand, Carciofo et al. ( 2014 ) had participants estimate when they were most likely to mind-wander instead of probing them throughout the day; they used a multi-item scale that is related to but different than task-unrelated thought to judge mind-wandering. By contrast, the current study presumably assessed participants’ attention to a variety of tasks performed in their everyday life—and did so while they were engaged in those tasks. Taken together, it is difficult to know whether the results of this study represent a true conflict with the literature or simply a difference between experience-sampling studies and lab-based alternatives.
Finally, it is also possible that the unanswered probes differed systematically from the answered probes. Participants were instructed not to answer probes if doing so would put them in danger (e.g. if they were driving a vehicle). The fact that certain activities were un- or underrepresented may mean that ratings across the three dimensions could be biased in one direction. Of course, given the concern for participant safety, it is impossible to address this concern sufficiently in natural data; instead, experimental paradigms could induce similar settings within controlled environments (e.g. risky driving simulations used by Watson et al., 2016 ) to empirically test whether and how these underrepresented activities affect the dynamics.
Another important future step beyond the current work is to better understand the mechanisms that may influence the levels of freedom of movement in thought across the day. For example, how do current concerns and other conscious or latent goal pursuits influence our thought patterns, especially when affectively charged? Additionally, it may be fruitful to assess thought patterns in clinical populations that display more fixated thoughts (e.g. individuals with ruminative tendencies). Studies such as these may provide some key insights about the relationship between affect and constraints and how those features influence our freedom of movement in thought throughout our daily lives.
Post-hoc power analyses
The lack of readily available standardized effect size estimates for mixed-effects polynomial models prevented us from determining our desired sample size based on a calculation of required statistical power. We nevertheless conducted post-hoc observed power analyses in order to inform future research about what types of sample sizes are appropriate for such effects. As observed power analyses are only conceptually meaningful when applied to tests of true effects, we conducted them only on significant parameters in order to estimate each study’s ability to detect the fixed effect in question. Although there is not consensus for how to calculate power exactly for multiple-predictor random-effects models (see Brysbaert & Stevens, 2018 ), we adopted a Monte Carlo simulation approach using the simR package in R (Green & MacLeod, 2015 ). It should be noted that due to their reliance on simulations, these numbers are not exact; however, setting a seed state before executing the analyses ensures that each analysis is replicable. Our shared code includes a fixed seed to allow readers to completely reproduce our analyses.
Table 7 lists the two significant parameters for Study 1 and the five significant parameters for Study 2, along with their corresponding observed power estimates. The sample size of 108 participants (after exclusions) in Study 1 yielded sub-optimal observed power for the quadratic parameter: 60.5% power means that if this study were replicated 100 times, over one-third of the replications would not have found the quadratic parameter to be significant. Although power for the cubic parameter was much higher (82%), future work should ideally set the sample size to ensure sufficient power for the weakest effect of interest. The sample size of 165 participants (after exclusions) used in Study 2 appears to be a little more appropriate; the power to detect the same quadratic parameter in the daily pattern of change for freely moving thought was bolstered to 67%; all other effects achieved a minimum of 80% power.
Observed power for the five significant parameters of Study 2
Observed power determined through simulation as opposed to calculation; 100% power is a statistical impossibility and is the product of simulation-based estimates
In sum, although observed power was lower for Study 1 (which solely focused on freely moving thought), we are encouraged that the patterns for freely moving thought were consistent across both datasets, especially given that observed power was much higher for Study 2. Moreover, based on these analyses, similar research on this topic may want to collect > 165 participants when aiming for > 80% power to detect the smallest effect.
Here, we find the first evidence that mind-wandering is not stable across the day – an important implication for both educational and occupational policy, as well as psychological research. Taken together, our findings emphasize the need to consider distinct dimensions of thought related to mind-wandering independently, a concept that has recently gained support in this research area (Mills, Raffaelli, et al., 2017 ; Seli, Risko, et al., 2016 ). A better understanding of the diurnal patterns of freely moving thought, task-unrelated thought, and stimulus-independent thought will contribute to a richer understanding of how our mental states dynamically shift over the course of our daily lives, perhaps even allowing us to optimize the structure of our days.
Additional files
Supplementary materials. Additional file 1. Model Comparison Results. Additional file 2. Complete Dataset Analyses. Additional file 3. Differentiation Analysis Comparing TUT and SIT. (ZIP 92 kb)
Acknowledgements
This work was supported by NSERC (RGPIN 327317-11) and CIHR (MOP-115197) grants to KC. Any opinions, findings and conclusions, or recommendations expressed in this paper are those of the authors and do not necessarily reflect the views of NSERC or CIHR.
This work was supported by NSERC (RGPIN 327317-11) and CIHR (MOP-115197) grants to KC. Any opinions, findings and conclusions, or recommendations expressed in this paper are those of the authors and do not necessarily reflect the views of NSERC or CIHR. This project was funded in part by a Moore-Sloan Data Science Environments Fellowship to AP, thanks to the Gordon and Betty Moore Foundation through Grant GBMF3834 and the Alfred P. Sloan Foundation through Grant 2013-10-27 to the University of California, Berkeley.
Availability of data and materials
Instructions.
Notes: Every participant was trained in a small group people. The same experimenter was in the room for the entire question and answer question. The same training video was shown to all participants.
Introducing the study and explaining how the probes work Verbal instructions given via video
So, in this short video, we’re going to tell you everything you need to know. There’s a lot of information, so pay close attention. But we’re going to pause throughout to make sure that you’re grasping it correctly and we’re going to answer any questions. Keep those in mind. And here we go.
The basics is that we’re going to get you to rate your mental activity in a survey that you’re going to be answering a number of times throughout the day. You’re going to get text messages at random times with a web link in them, and you’re going to click that, and you’re going to go to the survey, and it’s really important that you answer right away when you get the text. Actually, you have to answer within 10 seconds. If you’re driving, or if you’re in a situation during which you absolutely can’t answer right then and there, you should just not answer at all. Don’t set it aside and try to answer later because we can see that. That being said, try to interrupt whatever you’re doing. It’s really important that you answer as many of these as possible.
Now, as soon as you hear the beep, you’re going to click on the link and go to the survey. And we’re going to get you to save the number into your phone and customize the text message tone. We’ll give you instructions later on how to do that. But it’s very important that these text messages stand out from any other text messages for reasons we’re about to tell you. You need to know right away, when you hear the tone, that it is one of these important text messages. So, when you’re answering the survey, we want you to finish within 40 seconds. Now, I mean, that may seem like a short amount of time, but once you’ve practiced a couple of times actually it’s going to get very fast. You’ll probably be able to do it within 30 seconds. So, answering quickly and intuitively is important as well. And it’s also very important that you keep your phone around you. Obviously, if you’re going to be answering quickly, and you need to answer a lot of these, keep your phone on, keep the sound up loud, and keep it within arm’s reach at all times as much as possible. And finally, you need to answer at least 80% of these probes.
So, this is a survey about your thought activity. What do we mean by thoughts? By thoughts, we mean anything that is going on in your awareness. That can include internal stuff like memories, emotions, imagining things. It can also mean external things. So, if you’re aware of sensations in your body, or things that you see or hear or smell. You get the picture.
So, when you hear that special tone on your phone, you’re going to take a mental snapshot of what’s going on in your awareness at the time. So, you hear the tone, you pause briefly, your take a snapshot, and then you’re going to open the text, click the link, and you’re going to go and answer all the questions keeping that mental snapshot in mind.
Explanation of free movement
Was your mind moving about freely?
Your thoughts move freely when:
- They seem to wander around, flowing from one thing to another
- There is no overarching purpose or direction to your thinking. Although there may still be some connection between one thought and the next
- Images and memories seem to spontaneously come into your mind
- Your attention lands spontaneously on things in your environment
- Your mind may spontaneously drift between things in the external environment and internal images so it may go back and forth.
- Your thoughts move freely when it feels like your thoughts could land on pretty much anything
- Or that your thoughts seem to flow with ease
For example, you know, you’re on the bus going home. You might take the following snapshot of your thoughts: You picture yourself having dinner that evening, then wonder if you’ve been eating too much fast food recently, then notice the faint music playing from another passenger’s headphones and that reminds you of a song you’ve heard at a party the night before.
This is sort of an example of your thoughts are moving freely. You know, they can also move freely around a particular topic such as a current event or, you know, something you’re currently interested in. For example, you think of the bike you just bought, then think yourself biking down a trail next weekend, then picture your friend riding next to you, then remember the first bike you got for your 10th birthday, and so on. So, this is an example where you know, it’s sharing the same topic but it’s still moving freely in that range. Thought can also move freely in the external environment. So, you might be hiking on a forest trail, you notice your mind may shift from the gravel on the path in front of you, to a slug crawling up a stump, to a leaf floating in a puddle. You understand.
So, here we’re going to pause the video, and we’re going to get you to come up with your own example of thoughts when your mind was moving about freely. The example should be different from the ones that were just mentioned.
Freely moving thought ratings as a function of hours since waking
The same participants who had been excluded from the main analysis were excluded from the time-since-waking analysis, and an additional five of the remaining participants had no probes answered on days for which there was wake-up information, leaving a sample size of 104. Only probes answered between 0 and 16 h after waking were kept, as none of the hours after 16 contained responses from 25% or more of the sample.
The pattern of results was similar to the main analysis (Table 8 ), featuring a significant positive cubic parameter and no evidence of linear change over time. The main exception is the negative quadratic term, which trended toward but did not reach statistical significance. Although visual inspection of the data (Fig. 2 b) suggests a highly similar pattern (with the peak occurring 5 hafter waking, dropping sharply until 8 h after waking, and rising afterward), future work should continue to tests this effect.
Fixed effects of the optimal model for freely moving thought ratings by hour since waking (original dataset)
a Significant at alpha = 0.05
Weekend-only analyses
Full model results for the reanalysis of Mills, Raffaelli, et al. ( 2017 ) using only data points collected over weekends.
Fixed effects of the optimal (cubic) model for freely moving thought ratings (reanalyzed dataset, weekends only)
Fixed effects of the optimal (cubic) model for task-unrelated thought ratings (reanalyzed dataset, weekends only)
* Significant at alpha = 0.05
Fixed effects of the optimal (cubic) model for stimulus-independent thought ratings (reanalyzed dataset, weekends only)
Authors’ contributions
CM and KC contributed to the design and coordination of the study. GS and CM decided upon the analysis plan. GS and AP performed the analyses. GS, CM, and AP interpreted the results. GS wrote the initial draft of the manuscript with advisement from CM and KC. All authors read, edited, and approved the final manuscript.
Authors’ information
Not applicable.
Ethics approval and consent to participate
Ethical approval for this study was obtained from the University of British Columbia Behavioural Research Ethics Board, approval no. H16–01247.
Consent for publication
Competing interests.
The authors declare that they have no competing interests.
Publisher’s Note
Springer Nature remains neutral with regard to jurisdictional claims in published maps and institutional affiliations.
Contributor Information
Gabriel King Smith, Email: ac.cbu.hcysp@htimskg .
Caitlin Mills, Email: [email protected] .
Alexandra Paxton, Email: [email protected] .
Kalina Christoff, Email: ac.cbu.hcysp@ffotsirhck .
- Aston-Jones G, Cohen JD. An integrative theory of locus coeruleus-norepinephrine function: Adaptive gain and optimal performance. Annual Review of Neuroscience. 2005; 28 :403–450. doi: 10.1146/annurev.neuro.28.061604.135709. [ PubMed ] [ CrossRef ] [ Google Scholar ]
- Aston-Jones, Waterhouse Locus coeruleus: From global projection system to adaptive regulation of behavior. Brain Research. 2016; 1645 :75–78. doi: 10.1016/j.brainres.2016.03.001. [ PMC free article ] [ PubMed ] [ CrossRef ] [ Google Scholar ]
- Baird B, Smallwood J, Mrazek MD, Kam JWY, Franklin MS, Schooler JW. Inspired by distraction: Mind wandering facilitates creative incubation. Psychological Science. 2012; 23 (10):1117–1122. doi: 10.1177/0956797612446024. [ PubMed ] [ CrossRef ] [ Google Scholar ]
- Baldwin CL, Roberts DM, Barragan D, Lee JD, Lerner N, Higgins JS. Detecting and quantifying mind wandering during simulated driving. Frontiers in Human Neuroscience. 2017; 11 :406. doi: 10.3389/fnhum.2017.00406. [ PMC free article ] [ PubMed ] [ CrossRef ] [ Google Scholar ]
- Barr Dale J., Levy Roger, Scheepers Christoph, Tily Harry J. Random effects structure for confirmatory hypothesis testing: Keep it maximal. Journal of Memory and Language. 2013; 68 (3):255–278. doi: 10.1016/j.jml.2012.11.001. [ PMC free article ] [ PubMed ] [ CrossRef ] [ Google Scholar ]
- Bates, D., Mächler, M., Bolker, B., & Walker, S. (2015). Fitting linear mixed-effects models using lme4. Journal of Statistical Software , 67 (1). 10.18637/jss.v067.i01.
- Brysbaert, M., & Stevens, M. (2018). Power analysis and effect size in mixed effects models: a tutorial. Journal of Cognition , 1, 9 (1). 10.5334/joc.10. [ PMC free article ] [ PubMed ]
- Burke TM, Scheer FAJL, Ronda JM, Czeisler CA, Wright KPJ. Sleep inertia, sleep homeostatic and circadian influences on higher-order cognitive functions. Journal of Sleep Research. 2015; 24 (4):364–371. doi: 10.1111/jsr.12291. [ PMC free article ] [ PubMed ] [ CrossRef ] [ Google Scholar ]
- Callard F, Smallwood J, Margulies DS. Default positions: how neuroscience’s historical legacy has hampered investigation of the resting mind. Frontiers in Psychology. 2012; 3 :321. doi: 10.3389/fpsyg.2012.00321. [ PMC free article ] [ PubMed ] [ CrossRef ] [ Google Scholar ]
- Carciofo R, Du F, Song N, Zhang K. Chronotype and time-of-day correlates of mind wandering and related phenomena. Biological Rhythm Research. 2014; 45 (1):37–49. doi: 10.1080/09291016.2013.790651. [ CrossRef ] [ Google Scholar ]
- Carrier J, Monk TH. Circadian rhythms of performance: New trends. Chronobiology International. 2000; 17 (6):719–732. doi: 10.1081/CBI-100102108. [ PubMed ] [ CrossRef ] [ Google Scholar ]
- Christoff K, Irving ZC, Fox KCR, Spreng RN, Andrews-Hanna JR. Mind-wandering as spontaneous thought: a dynamic framework. Nature Reviews Neuroscience. 2016; 17 :718–731. doi: 10.1038/nrn.2016.113. [ PubMed ] [ CrossRef ] [ Google Scholar ]
- Christoff K, Mills C, Andrews-Hanna JR, Irving ZC, Thompson E, Fox KCR, Kam JWY. Mind-wandering as a scientific concept: cutting through the definitional haze. Trends in Cognitive Sciences. 2018; 22 (11):957–959. doi: 10.1016/j.tics.2018.07.004. [ PubMed ] [ CrossRef ] [ Google Scholar ]
- Dane E. Paying attention to mindfulness and its effects on task performance in the workplace. Journal of Management. 2011; 37 (4):997–1018. doi: 10.1177/0149206310367948. [ CrossRef ] [ Google Scholar ]
- Dijksterhuis A, Meurs T. Where creativity resides: The generative power of unconscious thought. Consciousness and Cognition. 2006; 15 (1):135–146. doi: 10.1016/j.concog.2005.04.007. [ PubMed ] [ CrossRef ] [ Google Scholar ]
- Dust SB. Mindfulness, flow, and mind wandering: The role of trait-based mindfulness in state-task alignment. Industrial and Organizational Psychology; Bowling Green. 2015; 8 (4):609–614. doi: 10.1017/iop.2015.87. [ CrossRef ] [ Google Scholar ]
- Ellamil M, Dobson C, Beeman M, Christoff K. Evaluative and generative modes of thought during the creative process. NeuroImage. 2012; 59 (2):1783–1794. doi: 10.1016/j.neuroimage.2011.08.008. [ PubMed ] [ CrossRef ] [ Google Scholar ]
- Ellamil M, Fox KCR, Dixon ML, Pritchard S, Todd RM, Thompson E, Christoff K. Dynamics of neural recruitment surrounding the spontaneous arising of thoughts in experienced mindfulness practitioners. NeuroImage. 2016; 136 :186–196. doi: 10.1016/j.neuroimage.2016.04.034. [ PubMed ] [ CrossRef ] [ Google Scholar ]
- Faber M, Bixler R, D’Mello SK. An automated behavioral measure of mind wandering during computerized reading. Behavior Research Methods. 2018; 50 (1):134–150. doi: 10.3758/s13428-017-0857-y. [ PubMed ] [ CrossRef ] [ Google Scholar ]
- Fibiger W, Singer G, Miller AJ, Armstrong S, Datar M. Cortisol and catecholamines changes as functions of time-of-day and self-reported mood. Neuroscience & Biobehavioral Reviews. 1984; 8 (4):523–530. doi: 10.1016/0149-7634(84)90009-5. [ PubMed ] [ CrossRef ] [ Google Scholar ]
- Fimm B, Blankenheim A. Effect of sleep deprivation and low arousal on eye movements and spatial attention. Neuropsychologia. 2016; 92 (Supplement C):115–128. doi: 10.1016/j.neuropsychologia.2016.03.021. [ PubMed ] [ CrossRef ] [ Google Scholar ]
- Fimm B, Brand T, Spijkers W. Time-of-day variation of visuo-spatial attention. British Journal of Psychology. 2016; 107 (2):299–321. doi: 10.1111/bjop.12143. [ PubMed ] [ CrossRef ] [ Google Scholar ]
- Folkard S, Monk TH. The measurement of circadian rhythms in psychological functions. In: Scheving LE, Halberg F, Ehret C, editors. Chronobiotechnology and Chronobiological Engineering. Dordrecht: Springer; 1987. pp. 189–201. [ Google Scholar ]
- Fox KCR, Nijeboer S, Solomonova E, Domhoff GW, Christoff K. Dreaming as mind wandering: evidence from functional neuroimaging and first-person content reports. Frontiers in Human Neuroscience. 2013; 7 :412. doi: 10.3389/fnhum.2013.00412. [ PMC free article ] [ PubMed ] [ CrossRef ] [ Google Scholar ]
- Fox KCR, Spreng RN, Ellamil M, Andrews-Hanna JR, Christoff K. The wandering brain: Meta-analysis of functional neuroimaging studies of mind-wandering and related spontaneous thought processes. NeuroImage. 2015; 111 :611–621. doi: 10.1016/j.neuroimage.2015.02.039. [ PubMed ] [ CrossRef ] [ Google Scholar ]
- Franklin MS, Mrazek MD, Anderson CL, Smallwood J, Kingstone A, Schooler JW. The silver lining of a mind in the clouds: Interesting musings are associated with positive mood while mind-wandering. Frontiers in Psychology. 2013; 4 :583. doi: 10.3389/fpsyg.2013.00583. [ PMC free article ] [ PubMed ] [ CrossRef ] [ Google Scholar ]
- Franklin MS, Smallwood J, Schooler JW. Catching the mind in flight: Using behavioral indices to detect mindless reading in real time. Psychonomic Bulletin & Review. 2011; 18 (5):992–997. doi: 10.3758/s13423-011-0109-6. [ PubMed ] [ CrossRef ] [ Google Scholar ]
- Franklin MS, Smallwood J, Zedelius CM, Broadway JM, Schooler JW. Unaware yet reliant on attention: Experience sampling reveals that mind-wandering impedes implicit learning. Psychonomic Bulletin & Review. 2016; 23 (1):223–229. doi: 10.3758/s13423-015-0885-5. [ PubMed ] [ CrossRef ] [ Google Scholar ]
- Fuller-Tyszkiewicz M, Hartley-Clark L, Cummins RA, Tomyn AJ, Weinberg MK, Richardson B. Using dynamic factor analysis to provide insights into data reliability in experience sampling studies. Psychological Assessment. 2017; 29 (9):1120–1128. doi: 10.1037/pas0000411. [ PubMed ] [ CrossRef ] [ Google Scholar ]
- Gabehart RJ, Dongen HPAV. Principles and Practice of Sleep Medicine. 6. Philadelphia: Elsevier; 2017. Circadian rhythms in sleepiness, alertness, and performance; pp. 388–395.e5. [ Google Scholar ]
- Galéra C, Orriols L, M’Bailara K, Laborey M, Contrand B, Ribéreau-Gayon R, et al. Mind wandering and driving: responsibility case-control study. BMJ. 2012; 345 :e8105. doi: 10.1136/bmj.e8105. [ PMC free article ] [ PubMed ] [ CrossRef ] [ Google Scholar ]
- Giambra LM, Rosenberg EH, Kasper S, Yee W, Sack DA. A circadian rhythm in the frequency of spontaneous task-tnrelated images and thoughts. Imagination, Cognition and Personality. 1989; 8 (4):309–314. doi: 10.2190/1ECY-9JDW-MXNW-KMWC. [ CrossRef ] [ Google Scholar ]
- Gilzenrat MS, Nieuwenhuis S, Jepma M, Cohen JD. Pupil diameter tracks changes in control state predicted by the adaptive gain theory of locus coeruleus function. Cognitive, Affective, & Behavioral Neuroscience. 2010; 10 (2):252–269. doi: 10.3758/CABN.10.2.252. [ PMC free article ] [ PubMed ] [ CrossRef ] [ Google Scholar ]
- Goel N, Basner M, Rao H, Dinges DF. Circadian rhythms, sleep deprivation, and human performance. Progress in Molecular Biology and Translational Science. 2013; 119 :155–190. doi: 10.1016/B978-0-12-396971-2.00007-5. [ PMC free article ] [ PubMed ] [ CrossRef ] [ Google Scholar ]
- Green P, MacLeod CJ. SIMR: an R package for power analysis of generalized linear mixed models by simulation. Methods in Ecology and Evolution. 2015; 7 (4):493–498. doi: 10.1111/2041-210X.12504. [ CrossRef ] [ Google Scholar ]
- Hansen ÅM, Garde AH, Skovgaard LT, Christensen JM. Seasonal and biological variation of urinary epinephrine, norepinephrine, and cortisol in healthy women. Clinica Chimica Acta. 2001; 309 (1):25–35. doi: 10.1016/S0009-8981(01)00493-4. [ PubMed ] [ CrossRef ] [ Google Scholar ]
- Hartmann A, Zeeck A, Herzog W, Wild B, de Zwaan M, Herpertz S, et al. The intersession process in psychotherapy for anorexia nervosa: Characteristics and relation to outcome. Journal of Clinical Psychology. 2016; 72 (9):861–879. doi: 10.1002/jclp.22293. [ PubMed ] [ CrossRef ] [ Google Scholar ]
- He J, Becic E, Lee Y-C, McCarley JS. Mind wandering behind the wheel: Performance and oculomotor correlates. Human Factors. 2011; 53 (1):13–21. doi: 10.1177/0018720810391530. [ PubMed ] [ CrossRef ] [ Google Scholar ]
- Hurlburt RT, Alderson-Day B, Fernyhough C, Kühn S. Can inner experience be apprehended in high fidelity? Examining brain activation and experience from multiple perspectives. Frontiers in Psychology. 2017; 8 :628. doi: 10.3389/fpsyg.2017.00043. [ PMC free article ] [ PubMed ] [ CrossRef ] [ Google Scholar ]
- Hyland PK, Lee RA, Mills MJ. Mindfulness at work: A new approach to improving individual and organizational performance. Industrial and Organizational Psychology; Bowling Green. 2015; 8 (4):576–602. doi: 10.1017/iop.2015.41. [ CrossRef ] [ Google Scholar ]
- Irving ZC. Mind-wandering is unguided attention: accounting for the “purposeful” wanderer. Philosophical Studies. 2016; 173 (2):547–571. doi: 10.1007/s11098-015-0506-1. [ CrossRef ] [ Google Scholar ]
- Jazaieri H, Lee IA, McGonigal K, Jinpa T, Doty JR, Gross JJ, Goldin PR. A wandering mind is a less caring mind: Daily experience sampling during compassion meditation training. The Journal of Positive Psychology. 2016; 11 (1):37–50. doi: 10.1080/17439760.2015.1025418. [ CrossRef ] [ Google Scholar ]
- Jewett ME, Kronauer RE. Interactive mathematical models of subjective alertness and cognitive throughput in humans. Journal of Biological Rhythms. 1999; 14 (6):588–597. doi: 10.1177/074873099129000920. [ PubMed ] [ CrossRef ] [ Google Scholar ]
- Keith TZ. Multiple Regression and Beyond. Boston: Pearson; 2005. [ Google Scholar ]
- Killingsworth MA, Gilbert DT. A wandering mind is an unhappy mind. Science. 2010; 330 (6006):932–932. doi: 10.1126/science.1192439. [ PubMed ] [ CrossRef ] [ Google Scholar ]
- Krawietz SA, Tamplin AK, Radvansky GA. Aging and mind wandering during text comprehension. Psychology and Aging. 2012; 27 (4):951–958. doi: 10.1037/a0028831. [ PubMed ] [ CrossRef ] [ Google Scholar ]
- Kucyi A, Davis KD. Dynamic functional connectivity of the default mode network tracks daydreaming. NeuroImage. 2014; 100 :471–480. doi: 10.1016/j.neuroimage.2014.06.044. [ PubMed ] [ CrossRef ] [ Google Scholar ]
- Kuznetsova, A., Brockhoff, P. B., & Christensen, R. H. B. (2017). lmerTest Package: Tests in Linear Mixed Effects Models. Journal of Statistical Software , 82 (13). 10.18637/jss.v082.i13.
- Marchetti I, Koster EHW, Klinger E, Alloy LB. Spontaneous thought and vulnerability to mood disorders: The dark side of the wandering mind. Clinical Psychological Science. 2016; 4 (5):835–857. doi: 10.1177/2167702615622383. [ PMC free article ] [ PubMed ] [ CrossRef ] [ Google Scholar ]
- Mather M, Clewett D, Sakaki M, Harley CW. Norepinephrine ignites local hotspots of neuronal excitation: How arousal amplifies selectivity in perception and memory. Behavioral and Brain Sciences. 2016; 39 :e200. doi: 10.1017/S0140525X15000667. [ PMC free article ] [ PubMed ] [ CrossRef ] [ Google Scholar ]
- McCormick, C., Rosenthal, C. R., Miller, T. D., & Maguire, E. (2017). Mind-wandering in people with hippocampal amnesia. BioRxiv. 10.1101/159681. [ PMC free article ] [ PubMed ]
- McVay JC, Kane MJ. Conducting the train of thought: Working memory capacity, goal neglect, and mind wandering in an executive-control task. Journal of Experimental Psychology: Learning, Memory, and Cognition. 2009; 35 (1):196–204. doi: 10.1037/a0014104. [ PMC free article ] [ PubMed ] [ CrossRef ] [ Google Scholar ]
- McVay JC, Kane MJ, Kwapil TR. Tracking the train of thought from the laboratory into everyday life: An experience-sampling study of mind wandering across controlled and ecological contexts. Psychonomic Bulletin & Review. 2009; 16 (5):857–863. doi: 10.3758/PBR.16.5.857. [ PMC free article ] [ PubMed ] [ CrossRef ] [ Google Scholar ]
- Mills, C., Bixler, R., Wang, X., & D’Mello, S. K. (2016). Automatic Gaze-Based Detection of Mind Wandering during Film Viewing. (pp. 30–37). Presented at the International Conference on Educational Data Mining, Raleigh, NC: International Educational Data Mining Society.
- Mills C, D’Mello S. Toward a Real-Time (Day) Dreamcatcher: Sensor-Free Detection of Mind Wandering during Online Reading. 2015. [ Google Scholar ]
- Mills C, D’Mello SK, Kopp K. The influence of consequence value and text difficulty on affect, attention, and learning while reading instructional texts. Learning and Instruction. 2015; 40 :9–20. doi: 10.1016/j.learninstruc.2015.07.003. [ CrossRef ] [ Google Scholar ]
- Mills C, Graesser A, Risko EF, D’Mello SK. Cognitive coupling during reading. Journal of Experimental Psychology: General. 2017; 146 (6):872–883. doi: 10.1037/xge0000309. [ PubMed ] [ CrossRef ] [ Google Scholar ]
- Mills C, Herrera-Bennett A, Faber M, Christoff K. The Oxford Handbook of Spontaneous Thought: Mind-wandering, Creativity, Dreaming, and Clinical Conditions. New York: Oxford University Press; 2018. Why the mind wanders: How spontaneous thought’s default variability may support episodic efficiency and semantic optimization; pp. 11–22. [ Google Scholar ]
- Mills C, Raffaelli Q, Irving ZC, Stan D, Christoff K. Is an off-task mind a freely-moving mind? Examining the relationship between different dimensions of thought. Consciousness and Cognition. 2018; 58 :20–33. doi: 10.1016/j.concog.2017.10.003. [ PubMed ] [ CrossRef ] [ Google Scholar ]
- Mirman D. Growth curve analysis and visualization using R. Bocan Raton: CRC Press; 2016. [ Google Scholar ]
- Mirman D, Dixon JA, Magnuson JS. Statistical and computational models of the visual world paradigm: Growth curves and individual differences. Journal of Memory and Language. 2008; 59 (4):475–494. doi: 10.1016/j.jml.2007.11.006. [ PMC free article ] [ PubMed ] [ CrossRef ] [ Google Scholar ]
- Mittner M, Hawkins GE, Boekel W, Forstmann BU. A neural model of mind wandering. Trends in Cognitive Sciences. 2016; 20 (8):570–578. doi: 10.1016/j.tics.2016.06.004. [ PubMed ] [ CrossRef ] [ Google Scholar ]
- Mrazek MD, Zedelius CM, Gross ME, Mrazek AJ, Phillips DT, Schooler JW. Mindfulness in education: Enhancing academic achievement and student well-being by reducing mind-wandering. In: Karremans JC, Papies EK, Karremans JC, Papies EK, editors. Mindfulness in social psychology. New York: Routledge/Taylor & Francis Group; 2017. pp. 139–152. [ Google Scholar ]
- Nisbett RE, Wilson TD. Telling more than we can know: Verbal reports on mental processes. Psychological Review. 1977; 84 (3):231–259. doi: 10.1037/0033-295X.84.3.231. [ CrossRef ] [ Google Scholar ]
- Nolen-Hoeksema S, Wisco BE, Lyubomirsky S. Rethinking rumination. Perspectives on Psychological Science. 2008; 3 (5):400–424. doi: 10.1111/j.1745-6924.2008.00088.x. [ PubMed ] [ CrossRef ] [ Google Scholar ]
- Pachai AA, Acai A, LoGiudice AB, Kim JA. The mind that wanders: Challenges and potential benefits of mind wandering in education. Scholarship of Teaching and Learning in Psychology. 2016; 2 (2):134–146. doi: 10.1037/stl0000060. [ CrossRef ] [ Google Scholar ]
- Papageorghiou AT, Ohuma EO, Altman DG, Todros T, Ismail LC, Lambert A, et al. International standards for fetal growth based on serial ultrasound measurements: the Fetal Growth Longitudinal Study of the INTERGROWTH-21st Project. The Lancet. 2014; 384 (9946):869–879. doi: 10.1016/S0140-6736(14)61490-2. [ PubMed ] [ CrossRef ] [ Google Scholar ]
- Phillips NE, Mills C, D’Mello S, Risko EF. On the influence of re-reading on mind wandering. Quarterly Journal of Experimental Psychology. 2016; 69 (12):2338–2357. doi: 10.1080/17470218.2015.1107109. [ PubMed ] [ CrossRef ] [ Google Scholar ]
- Qu W, Ge Y, Xiong Y, Carciofo R, Zhao W, Zhang K. The relationship between mind wandering and dangerous driving behavior among Chinese drivers. Safety Science. 2015; 78 :41–48. doi: 10.1016/j.ssci.2015.04.016. [ CrossRef ] [ Google Scholar ]
- Riley E, Esterman M, Fortenbaugh FC, DeGutis J. Time-of-day variation in sustained attentional control. Chronobiology International. 2017; 34 (7):993–1001. doi: 10.1080/07420528.2017.1308951. [ PubMed ] [ CrossRef ] [ Google Scholar ]
- Schooler JW, Smallwood J, Christoff K, Handy TC, Reichle ED, Sayette MA. Meta-awareness, perceptual decoupling and the wandering mind. Trends in Cognitive Sciences. 2011; 15 (7):319–326. doi: 10.1016/j.tics.2011.05.006. [ PubMed ] [ CrossRef ] [ Google Scholar ]
- Seli P, Kane MJ, Smallwood J, Schacter DL, Maillet D, Schooler JW, Smilek D. Mind-Wandering as a Natural Kind: A Family-Resemblances View. Trends in Cognitive Sciences. 2018; 22 (6):479–490. doi: 10.1016/j.tics.2018.03.010. [ PMC free article ] [ PubMed ] [ CrossRef ] [ Google Scholar ]
- Seli P, Risko EF, Smilek D, Schacter DL. Mind-Wandering with and without intention. Trends in Cognitive Sciences. 2016; 20 (8):605–617. doi: 10.1016/j.tics.2016.05.010. [ PMC free article ] [ PubMed ] [ CrossRef ] [ Google Scholar ]
- Seli P, Wammes JD, Risko EF, Smilek D. On the relation between motivation and retention in educational contexts: The role of intentional and unintentional mind wandering. Psychonomic Bulletin & Review. 2016; 23 (4):1280–1287. doi: 10.3758/s13423-015-0979-0. [ PubMed ] [ CrossRef ] [ Google Scholar ]
- Shin T. The application of various nonlinear models to describe academic growth trajectories: an empirical analysis using four-wave longitudinal achievement data from a large urban school district. Asia Pacific Education Review. 2012; 13 (1):65–76. doi: 10.1007/s12564-011-9168-7. [ CrossRef ] [ Google Scholar ]
- Silva EJ, Wang W, Ronda JM, Wyatt JK, Duffy JF. Circadian and wake-dependent influences on subjective sleepiness, cognitive throughput, and reaction time performance in older and young adults. Sleep. 2010; 33 (4):481. doi: 10.1093/sleep/33.4.481. [ PMC free article ] [ PubMed ] [ CrossRef ] [ Google Scholar ]
- Singer JD, Willett JB. Applied longitudinal data analysis: modeling change and event occurrence. Oxford: Oxford University Press; 2003. [ Google Scholar ]
- Smallwood J, Fitzgerald A, Miles LK, Phillips LH. Shifting moods, wandering minds: Negative moods lead the mind to wander. Emotion. 2009; 9 (2):271–276. doi: 10.1037/a0014855. [ PubMed ] [ CrossRef ] [ Google Scholar ]
- Smallwood J, McSpadden M, Schooler JW. When attention matters: The curious incident of the wandering mind. Memory & Cognition. 2008; 36 (6):1144–1150. doi: 10.3758/MC.36.6.1144. [ PubMed ] [ CrossRef ] [ Google Scholar ]
- Smallwood J, Nind L, O’Connor RC. When is your head at? An exploration of the factors associated with the temporal focus of the wandering mind. Consciousness and Cognition. 2009; 18 (1):118–125. doi: 10.1016/j.concog.2008.11.004. [ PubMed ] [ CrossRef ] [ Google Scholar ]
- Song X, Wang X. Mind wandering in Chinese daily lives—An experience sampling study. PLoS One. 2012; 7 (9):e44423. doi: 10.1371/journal.pone.0044423. [ PMC free article ] [ PubMed ] [ CrossRef ] [ Google Scholar ]
- Sousa TLV, Carriere JSA, eSmilek D. The way we encounter reading material influences how frequently we mind wander. Frontiers in Psychology. 2013; 4 :892. doi: 10.3389/fpsyg.2013.00892. [ PMC free article ] [ PubMed ] [ CrossRef ] [ Google Scholar ]
- Spronken M, Holland RW, Figner B, Dijksterhuis A. Temporal focus, temporal distance, and mind-wandering valence: Results from an experience sampling and an experimental study. Consciousness and Cognition. 2016; 41 :104–118. doi: 10.1016/j.concog.2016.02.004. [ PubMed ] [ CrossRef ] [ Google Scholar ]
- Stawarczyk D, Majerus S, Maj M, Van der Linden M, D’Argembeau A. Mind-wandering: Phenomenology and function as assessed with a novel experience sampling method. Acta Psychologica. 2011; 136 (3):370–381. doi: 10.1016/j.actpsy.2011.01.002. [ PubMed ] [ CrossRef ] [ Google Scholar ]
- Unsworth N, McMillan BD. Mind wandering and reading comprehension: Examining the roles of working memory capacity, interest, motivation, and topic experience. Journal of Experimental Psychology: Learning, Memory, and Cognition. 2013; 39 (3):832–842. doi: 10.1037/a0029669. [ PubMed ] [ CrossRef ] [ Google Scholar ]
- Valdez P, Ramírez C, García A. Circadian rhythms in cognitive processes: Implications for school learning. Mind, Brain, and Education. 2014; 8 (4):161–168. doi: 10.1111/mbe.12056. [ CrossRef ] [ Google Scholar ]
- Valdez P, Ramírez C, García A, Talamantes J, Armijo P, Borrani J. Circadian rhythms in components of attention. Biological Rhythm Research. 2005; 36 (1–2):57–65. doi: 10.1080/09291010400028633. [ CrossRef ] [ Google Scholar ]
- Valdez P, Reilly T, Waterhouse J. Rhythms of mental performance. Mind, Brain, and Education. 2008; 2 (1):7–16. doi: 10.1111/j.1751-228X.2008.00023.x. [ CrossRef ] [ Google Scholar ]
- Watson JM, Memmott MG, Moffitt CC, Coleman J, Turrill J, Fernández Á, Strayer DL. On Working Memory and a Productivity Illusion in Distracted Driving. Journal of Applied Research in Memory and Cognition. 2016; 5 (4):445–453. doi: 10.1016/j.jarmac.2016.06.008. [ CrossRef ] [ Google Scholar ]
- White HR, Xie M, Thompson W, Loeber R, Stouthamer-Loeber M. Psychopathology as a predictor of adolescent drug use trajectories. Psychology of Addictive Behaviors. 2001; 15 (3):210–218. doi: 10.1037/0893-164X.15.3.210. [ PubMed ] [ CrossRef ] [ Google Scholar ]
- Wright KP, Hull JT, Czeisler CA. Relationship between alertness, performance, and body temperature in humans. American Journal of Physiology - Regulatory, Integrative and Comparative Physiology. 2002; 283 (6):R1370–R1377. doi: 10.1152/ajpregu.00205.2002. [ PubMed ] [ CrossRef ] [ Google Scholar ]
- Yanko MR, Spalek TM. Driving with the wandering mind: The effect that mind-wandering has on driving performance. Human Factors. 2014; 56 (2):260–269. doi: 10.1177/0018720813495280. [ PubMed ] [ CrossRef ] [ Google Scholar ]
- Allison, P. D. (2014). Event history and survival analysis: regression for longitudinal event data . SAGE Publications.
- Dziak JJ, Li R, Tan X, Shiffman S, Shiyko MP. Modeling intensive longitudinal data with mixtures of nonparametric trajectories and time-varying effects., Modeling Intensive Longitudinal Data With Mixtures of Nonparametric Trajectories and Time-Varying Effects. Psychological Methods, Psychological Methods. 2015; 20 (4):444–469. doi: 10.1037/met0000048. [ PMC free article ] [ PubMed ] [ CrossRef ] [ Google Scholar ]
- R Core Team . R: A language and environment for statistical computing. Vienna, Austria: R Foundation for Statistical Computing; 2017. [ Google Scholar ]
The Current State of Mind: a Systematic Review of the Relationship Between Mindfulness and Mind-Wandering
- Published: 25 November 2021
- Volume 6 , pages 272–294, ( 2022 )
Cite this article
- Lynley Turkelson ORCID: orcid.org/0000-0002-2735-1105 1 &
- Quintino Mano ORCID: orcid.org/0000-0001-8968-1735 1
1058 Accesses
3 Citations
124 Altmetric
18 Mentions
Explore all metrics
Mind-wandering—defined as off-task thinking—can be disruptive to daily functioning. Mindfulness is considered a potential method for reducing mind-wandering; however, no study has systematically reviewed findings on this topic. The present systematic review synthesizes current findings from this literature, examining whether results vary as a function of study methodology. Our final sample included n = 15 peer-reviewed studies, with 14 studies describing at least one significant relationship between the two constructs. Study results varied as a function of how constructs were operationalized and type of active control. Mindfulness appears most consistently related to reductions in probe-caught mind-wandering, as well as fewer commission errors and less response time variability on sustained attention tasks. Self-report measures of both constructs were the least consistent in their relation to other measures. Future research should focus on increasing methodological rigor to confirm results and on identifying facets of mindfulness most effective for decreasing mind-wandering.
This is a preview of subscription content, log in via an institution to check access.
Access this article
Price includes VAT (Russian Federation)
Instant access to the full article PDF.
Rent this article via DeepDyve
Institutional subscriptions
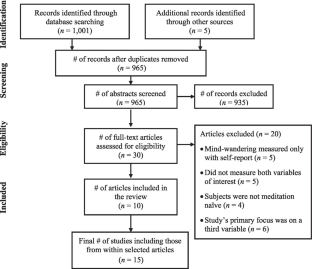
Similar content being viewed by others
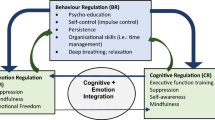
The positive aspects of attention deficit hyperactivity disorder: a qualitative investigation of successful adults with ADHD
Jane Ann Sedgwick, Andrew Merwood & Philip Asherson
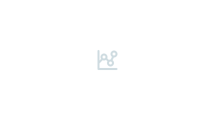
Emotional Well-Being: What It Is and Why It Matters
Crystal L. Park, Laura D. Kubzansky, … Kuan Hong Wang
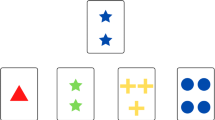
Considerations for using the Wisconsin Card Sorting Test to assess cognitive flexibility
Stephanie Miles, Caitlin A. Howlett, … Andrea Phillipou
Availability of Data and Material
Information was obtained from previously published studies. Coding and search strategy are detailed within the document and the authors do not have additional materials to share.
Code Availability
Not applicable.
Daydreaming and perceptual decoupling are closely related terms that are sometimes used to refer to mind-wandering. However, these constructs are considered distinct from mind-wandering by some. For additional details, see Mrazek, Phillips et al., ( 2013b ) and Schooler et al. ( 2011 ).
RTV is the standard deviation of reaction time divided by mean reaction time.
Studies in this review which used the FFMQ noted that scores on multiple facets of mindfulness increased as a result of mindfulness intervention, suggesting attentional awareness is not the only contributing facet.
Baer, R. A. (2006). Mindfulness-based treatment approaches. Elsevier Academic Press . https://doi.org/10.1016/B978-0-12-088519-0.X5000-X
Article Google Scholar
Baer, R. A., Smith, G. T., Lykins, E., Button, D., Krietemeyer, J., Sauer, S., Walsh, E., Duggan, D., & Williams, J. M. G. (2008). Construct validity of the five facet mindfulness questionnaire in meditating and nonmeditating samples. Assessment, 15 (3), 329–342. https://doi.org/10.1177/1073191107313003
Article PubMed Google Scholar
Banks, J. B., Welhaf, M. S., & Srour, A. (2015). The protective effects of brief mindfulness meditation training. Consciousness and Cognition, 33 , 277–285. https://doi.org/10.1016/j.concog.2015.01.016
Basner, M., & Dinges, D. F. (2011). Maximizing sensitivity of the psychomotor vigilance test (PVT) to sleep loss. Sleep, 34 (5), 581–591. https://doi.org/10.1093/sleep/34.5.581
Article PubMed PubMed Central Google Scholar
Bastian, M., & Sackur, J. (2013). Mind wandering at the fingertips: Automatic parsing of subjective states based on response time variability. Frontiers in Psychology, 4 , 573–583. https://doi.org/10.3389/fpsyg.2013.00573
Bellgrove, M. A., Hawi, Z., Kirley, A., Gill, M., & Robertson, I. H. (2005). Dissecting the attention deficit hyperactivity disorder (ADHD) phenotype: Sustained attention, response variability and spatial attentional asymmetries in relation to dopamine transporter (DAT1) genotype. Neuropsychologia, 43 (13), 1847–1857. https://doi.org/10.1016/j.neuropsychologia.2005.03.011
Bennike, I. H., Wieghorst, A., & Kirk, U. (2017). Online-based mindfulness training reduces behavioral markers of mind wandering. Journal of Cognitive Enhancement, 1 (2), 172–181. https://doi.org/10.1007/s41465-017-0020-9
Blanck, P., Perleth, S., Heidenreich, T., Kröger, P., Ditzen, B., Bents, H., & Mander, J. (2018). Effects of mindfulness exercises as stand-alone intervention on symptoms of anxiety and depression: Systematic review and meta-analysis. Behaviour Research and Therapy, 102 , 25–35. https://doi.org/10.1016/j.brat.2017.12.002
Bond, F. W., Hayes, S. C., Baer, R. A., Carpenter, K. M., Guenole, N., Orcutt, H. K., Waltz, T., & Zettle, R. D. (2011). Preliminary psychometric properties of the acceptance and action questionnaire–II: A revised measure of psychological inflexibility and experiential avoidance. Behavior Therapy, 42 (4), 676–688. https://doi.org/10.1016/j.beth.2011.03.007
Brown, K. W., & Ryan, R. M. (2003). The benefits of being present: Mindfulness and its role in psychological well-being. Journal of Personality and Social Psychology, 84 (4), 822–848. https://doi.org/10.1037/0022-3514.84.4.822
Carriere, J. S. A., Cheyne, J. A., & Smilek, D. (2008). Everyday attention lapses and memory failures: The affective consequences of mindlessness. Consciousness and Cognition, 17 (3), 835–847. https://doi.org/10.1016/j.concog.2007.04.008
Cheyne, J. A., Solman, G. J. F., Carriere, J. S. A., & Smilek, D. (2009). Anatomy of an error: A bidirectional state model of task engagement/disengagement and attention-related errors. Cognition, 111 (1), 98–113. https://doi.org/10.1016/j.cognition.2008.12.009
Cheyne, J. A., Carriere, J. S. A., & Smilek, D. (2006). Absent-mindedness: Lapses of conscious awareness and everyday cognitive failures. Consciousness and Cognition, 15 (3), 578–592. https://doi.org/10.1016/j.concog.2005.11.009
Davidson, R. J. (2010). Empirical explorations of mindfulness: Conceptual and methodological conundrums. Emotion, 10 (1), 8–11. https://doi.org/10.1037/a0018480
Davidson, R. J., & Kaszniak, A. W. (2015). Conceptual and methodological issues in research on mindfulness and meditation. American Psychologist, 70 (7), 581–592. https://doi.org/10.1037/a0039512
Dockree, P. M., Kelly, S. P., Roche, R. A. P., Hogan, M. J., Reilly, R. B., & Robertson, I. H. (2004). Behavioural and physiological impairments of sustained attention after traumatic brain injury. Cognitive Brain Research, 20 (3), 403–414. https://doi.org/10.1016/j.cogbrainres.2004.03.019
Dreyfus, G. (2011). Is mindfulness present-centred and non-judgmental? A discussion of the cognitive dimensions of mindfulness. Contemporary Buddhism, 12 (1), 41–54. https://doi.org/10.1080/14639947.2011.564815
Esterman, M., Noonan, S. K., Rosenberg, M., & Degutis, J. (2013). In the zone or zoning out? Tracking behavioral and neural fluctuations during sustained attention. Cerebral Cortex, 23 (11), 2712–2723. https://doi.org/10.1093/cercor/bhs261
Giambra, L. M. (1980). A factor analysis of the items of the imaginal processes inventory. Journal of Clinical Psychology, 36 (2), 383–409. https://doi.org/10.1002/jclp.6120360203
Giambra, L. M. (1993). The influence of aging on spontaneous shifts of attention from external stimuli to the contents of consciousness. Experimental Gerontology, 28 (4), 485–492. https://doi.org/10.1016/0531-5565(93)90073-M
Goldberg, S. B., Tucker, R. P., Greene, P. A., Davidson, R. J., Wampold, B. E., Kearney, D. J., & Simpson, T. L. (2018). Mindfulness-based interventions for psychiatric disorders: A systematic review and meta-analysis. Clinical Psychology Review, 59 , 52–60. https://doi.org/10.1016/j.cpr.2017.10.011
Grossman, P. (2011). Defining mindfulness by how poorly I think I pay attention during everyday awareness and other intractable problems for psychology’s (re)invention of mindfulness: Comment on Brown et al. (2011) . PubMed , 23 (4), 1034–1040. https://doi.org/10.1037/a0022713
Grossman, P., & Van Dam, N. T. (2011). Mindfulness, by any other name…: Trials and tribulations of sati in western psychology and science. Contemporary Buddhism, 12 (1), 219–239. https://doi.org/10.1080/14639947.2011.564841
Hilton, L., Hempel, S., Ewing, B., Apaydin, E., Xenakis, L., Newberry, S., Colaiaco, B., Maher, A., & Shanman, R. (2017). Mindfulness meditation for chronic pain: Systematic review and meta-analysis. Annals of Behavioral Medicine, 51 (2), 199–213. https://doi.org/10.1007/s12160-016-9844-2
Hopwood, T. L., & Schutte, N. S. (2017). A meta-analytic investigation of the impact of mindfulness-based interventions on post-traumatic stress. Clinical Psychology Review, 57 , 12–20. https://doi.org/10.1016/j.cpr.2017.08.002
Jackson, J. D., & Balota, D. A. (2012). Mind-wandering in younger and older adults: Converging evidence from the sustained attention to response task and reading for comprehension. Psychology and Aging, 27 (1), 106–119. https://doi.org/10.1037/a0023933
Jain, S., Shapiro, S. L., Swanick, S., Roesch, S. C., Mills, P. J., Bell, I., & Schwartz, G. E. R. (2007). A randomized controlled trial of mindfulness meditation versus relaxation training: Effects on distress, positive states of mind, rumination, and distraction. Annals of Behavioral Medicine, 33 (1), 11–21. https://doi.org/10.1207/s15324796abm3301_2
Jun, J., Remington, R. W., Koutstaal, W., & Jiang, Y. V. (2019). Characteristics of sustaining attention in a gradual-onset continuous performance task. Journal of Experimental Psychology. Human Perception and Performance , 45 (3), 386–401. https://doi.org/10.1037/xhp0000604
Khoury, B., Sharma, M., Rush, S. E., & Fournier, C. (2015). Mindfulness-based stress reduction for healthy individuals: A meta-analysis. Journal of Psychosomatic Research, 78 (6), 519–528. https://doi.org/10.1016/j.jpsychores.2015.03.009
Killingsworth, M. A., & Gilbert, D. T. (2010). A wandering mind is an unhappy mind. Science, 330 (6006), 932. https://doi.org/10.1126/science.1192439
Klinger, E., & Cox, W. M. (1987). Dimensions of thought flow in everyday life. Imagination, Cognition and Personality, 7 (2), 105–128. https://doi.org/10.2190/7K24-G343-MTQW-115V
Levinson, D. B., Stoll, E. L., Kindy, S. D., Merry, H. L., & Davidson, R. J. (2014). A mind you can count on: Validating breath counting as a behavioral measure of mindfulness. Frontiers in Psychology, 5 , 1202. https://doi.org/10.3389/fpsyg.2014.01202
Lim, J., & Dinges, D. F. (2008). Sleep deprivation and vigilant attention. Annals of the New York Academy of Sciences, 1129 (1), 305–322. https://doi.org/10.1196/annals.1417.002
Lomas, T., Medina, J. C., Ivtzan, I., Rupprecht, S., & Eiroa-Orosa, F. J. (2019). Mindfulness-based interventions in the workplace: An inclusive systematic review and meta-analysis of their impact upon wellbeing. The Journal of Positive Psychology, 14 (5), 625–640. https://doi.org/10.1080/17439760.2018.1519588
Lutz, A., Slagter, H. A., Dunne, J. D., & Davidson, R. J. (2008). Attention regulation and monitoring in meditation. Trends in Cognitive Sciences, 12 (4), 163–169. https://doi.org/10.1016/j.tics.2008.01.005
Matthews, G., Joyner, L., Campbell, S. E., Gilliland, K., Huggins, J., & Falconer, S. (1999). Validation of a comprehensive stress state questionnaire: Towards a state big three? In I. Mervielde, I. J. Deary, F. De Fruyt, & F. Ostendorf (Eds.), Personality psychology in Europe (pp. 335–350). Tilburg University Press.
Google Scholar
Mooneyham, B. W., & Schooler, J. W. (2013). The costs and benefits of mind-wandering. Canadian Journal of Experimental Psychology, 67 (1), 11–18. https://doi.org/10.1037/a0031569
Moher, D., Liberati, A., Tetzlaff, J., Altman, D. G., for the PRISMA Group, & PRISMA Group. (2009). Preferred reporting items for systematic reviews and meta-analyses: The PRISMA statement. BMJ, 339 , b2535–b2535. https://doi.org/10.1136/bmj.b2535
Morrison, A. B., Goolsarran, M., Rogers, S. L., & Jha, A. P. (2014). Taming a wandering attention: Short-form mindfulness training in student cohorts. Frontiers in Human Neuroscience, 7 , 897. https://doi.org/10.3389/fnhum.2013.00897
Mrazek, M. D., Franklin, M. S., Phillips, D. T., Baird, B., & Schooler, J. W. (2013a). Mindfulness training improves working memory capacity and GRE performance while reducing mind wandering. Psychological Science, 24 (5), 776–781. https://doi.org/10.1177/0956797612459659
Mrazek, M. D., Phillips, D. T., Franklin, M. S., Broadway, J. M., & Schooler, J. W. (2013b). Young and restless: Validation of the mind-wandering questionnaire (MWQ) reveals disruptive impact of mind-wandering for youth. Frontiers in Psychology, 4 , 560. https://doi.org/10.3389/fpsyg.2013.00560
Mrazek, M. D., Smallwood, J., & Schooler, J. W. (2012). Mindfulness and mind-wandering: Finding convergence through opposing constructs. Emotion, 12 (3), 442–448. https://doi.org/10.1037/a0026678
Poissant, H., Mendrek, A., Talbot, N., Khoury, B., & Nolan, J. (2019). Behavioral and cognitive impacts of mindfulness-based interventions on adults with attention-deficit hyperactivity disorder: A systematic review. Behavioural Neurology, 2019 , 1–16. https://doi.org/10.1155/2019/5682050
Poldrack, R. A. (2011). Inferring mental states from neuroimaging data: From reverse inference to large-scale decoding. Neuron, 72 (5), 692–697. https://doi.org/10.1016/j.neuron.2011.11.001
Rahl, H. A., Lindsay, E. K., Pacilio, L. E., Brown, K. W., & Creswell, J. D. (2017). Brief mindfulness meditation training reduces mind wandering: The critical role of acceptance. Emotion, 17 (2), 224–230. https://doi.org/10.1037/emo0000250
Reichle, E. D., Reineberg, A. E., & Schooler, J. W. (2010). Eye movements during mindless reading. Psychological Science, 21 (9), 1300–1310. https://doi.org/10.1177/0956797610378686
Robertson, I. H., Manly, T., Andrade, J., Baddeley, B. T., & Yiend, J. (1997). Oops!’: Performance correlates of everyday attentional failures in traumatic brain injured and normal subjects. Neuropsychologia, 35 (6), 747–758. https://doi.org/10.1016/S0028-3932(97)00015-8
Rosenberg, M. D., Finn, E. S., Scheinost, D., Papademetris, X., Shen, X., Constable, R. T., & Chun, M. M. (2016). A neuromarker of sustained attention from whole-brain functional connectivity. Nature Neuroscience, 19 (1), 165–171. https://doi.org/10.1038/nn.4179
Rosenberg, M., Noonan, S., DeGutis, J., & Esterman, M. (2013). Sustaining visual attention in the face of distraction: A novel gradual-onset continuous performance task. Attention, Perception, & Psychophysics, 75 (3), 426–439. https://doi.org/10.3758/s13414-012-0413-x
Ruffault, A., Czernichow, S., Hagger, M. S., Ferrand, M., Erichot, N., Carette, C., Boujut, E., & Flahault, C. (2016). The effects of mindfulness training on weight-loss and health-related behaviours in adults with overweight and obesity: A systematic review and meta-analysis. Obesity Research & Clinical Practice, 11 (5), 90–111. https://doi.org/10.1016/j.orcp.2016.09.002
Sauer, S., Walach, H., Schmidt, S., Hinterberger, T., Lynch, S., Büssing, A., & Kohls, N. (2013). Assessment of mindfulness: Review on state of the art. Mindfulness, 4 (1), 3–17. https://doi.org/10.1007/s12671-012-0122-5
Sayette, M. A., Reichle, E. D., & Schooler, J. W. (2009). Lost in the sauce: The effects of alcohol on mind wandering. Psychological Science, 20 (6), 747–752. https://doi.org/10.1111/j.1467-9280.2009.02351.x
Schooler, J. W., Mrazek, M. D., Franklin, M. S., Baird, B., Mooneyham, B. W., Zedelius, C., & Broadway, J. M. (2014). The middle way: Finding the balance between mindfulness and mind-wandering. Psychology of Learning and Motivation, 60 , 1–33. https://doi.org/10.1016/B978-0-12-800090-8.00001-9
Schooler, J. W., Reichle, E. D., & Halpern, D. V. (2004). Zoning out while reading: Evidence for dissociations between experience and meta-consciousness. In D. T. Levin (Ed.), Thinking and seeing: Visual metacognition in adults and children (pp. 203–226). MIT Press.
Schooler, J. W., Smallwood, J., Christoff, K., Handy, T. C., Reichle, E. D., & Sayette, M. A. (2011). Meta-awareness, perceptual decoupling and the wandering mind. Trends in Cognitive Sciences, 15 (7), 319–326. https://doi.org/10.1016/j.tics.2011.05.006
Selchen, S., Hawley, L., Regev, R., Richter, P., & Rector, N. (2018). Mindfulness-based cognitive therapy for OCD: Stand-alone and post-CBT augmentation approaches. International Journal of Cognitive Therapy, 11 (1), 58–79. https://doi.org/10.1007/s41811-018-0003-3
Seli, P., Carriere, J. S. A., & Smilek, D. (2015). Not all mind wandering is created equal: Dissociating deliberate from spontaneous mind wandering. Psychological Research Psychologische Forschung, 79 (5), 750–758. https://doi.org/10.1007/s00426-014-0617-x
Singer, J. L., & Antrobus, J. S. (1963). A factor-analytic study of daydreaming and conceptually-related cognitive and personality variables. Perceptual and Motor Skills, 17 , 187–209. https://doi.org/10.2466/pms.1963.17.1.187
Smallwood, J., Fitzgerald, A., Miles, L. K., & Phillips, L. H. (2009). Shifting moods, wandering minds. Emotion, 9 (2), 271–276. https://doi.org/10.1037/a0014855
Smallwood, J., & Schooler, J. W. (2006). The restless mind. Psychological Bulletin, 132 (6), 946–958. https://doi.org/10.1037/0033-2909.132.6.946
Sterne, J. A. C., Savović, J., Page, M. J., Elbers, R. G., Blencowe, N. S., Boutron, I., Cates, C. J., Cheng, H.-Y., Corbett, M. S., Eldridge, S. M., Emberson, J. R., Hernán, M. A., Hopewell, S., Hróbjartsson, A., Junqueira, D. R., Jüni, P., Kirkham, J. J., Lasserson, T., Li, T., & Higgins, J. P. T. (2019). RoB 2: A revised tool for assessing risk of bias in randomised trials. BMJ, 366 , l4898. https://doi.org/10.1136/bmj.l4898
Van Dam, N. T., Earleywine, M., & Borders, A. (2010). Measuring mindfulness? An Item Response Theory analysis of the Mindful Attention Awareness Scale. Personality and Individual Differences, 49 (7), 805–810. https://doi.org/10.1016/j.paid.2010.07.020
Wegner, D. M. (1994). Ironic processes of mental control. Psychological Review, 101 (1), 34–52. https://doi.org/10.1037//0033-295X.101.1.34
Weinstein, Y. (2018). Mind-wandering, how do I measure thee with probes? Let me count the ways. Behavior Research Methods, 50 (2), 642–661. https://doi.org/10.3758/s13428-017-0891-9
Wong, K. F., Massar, S. A. A., Chee, M., & Lim, J. (2018). Towards an objective measure of mindfulness: Replicating and extending the features of the breath-counting task. Mindfulness, 9 (5), 1402–1410. https://doi.org/10.1007/s12671-017-0880-1
Download references
Acknowledgements
I would like to thank everyone who assisted in the editing and publication of this article. To Kristen Mano and Cathy Stough, thank you for your helpful feedback early on and your support of this project. Thank you to my research assistant, Qingyi Zhang, for her diligence and patience helping me edit and prepare this manuscript. Thank you also to my wonderful friend and colleague, Miguel Nuñez, who spent countless late nights helping me proofread and edit. And lastly, to my research mentor and co-author, Quintino Mano. None of this would be possible without his ongoing academic support, mentorship, and wisdom.
Author information
Authors and affiliations.
Psychology Department, University of Cincinnati, PO Box 210376, Cincinnati, OH, 4522, USA
Lynley Turkelson & Quintino Mano
You can also search for this author in PubMed Google Scholar
Contributions
Conceptualization, Lynley Turkelson and Quintino Mano; investigation, Lynley Turkelson and Quintino Mano; formal analysis, Lynley Turkelson; writing, original draft, Lynley Turkelson; writing, review and editing, Lynley Turkelson and Quintino Mano.
Corresponding author
Correspondence to Lynley Turkelson .
Ethics declarations
Ethics approval.
No ethics approval was required as this study did not collect any human subject data and none of the original data from included studies was accessed.
Consent to Participate
For all included studies, we verified that researchers obtained informed consent from subjects before they participated in the study.
Consent for Publication
All information used to conduct this review was previously published and thus does not require additional permission.
Competing Interests
The authors declare no competing interests.
Additional information
Publisher's note.
Springer Nature remains neutral with regard to jurisdictional claims in published maps and institutional affiliations.
Rights and permissions
Reprints and permissions
About this article
Turkelson, L., Mano, Q. The Current State of Mind: a Systematic Review of the Relationship Between Mindfulness and Mind-Wandering. J Cogn Enhanc 6 , 272–294 (2022). https://doi.org/10.1007/s41465-021-00231-6
Download citation
Received : 20 May 2021
Accepted : 29 October 2021
Published : 25 November 2021
Issue Date : June 2022
DOI : https://doi.org/10.1007/s41465-021-00231-6
Share this article
Anyone you share the following link with will be able to read this content:
Sorry, a shareable link is not currently available for this article.
Provided by the Springer Nature SharedIt content-sharing initiative
- Mindfulness
- Mind-wandering
- Task-unrelated thought
- Mindfulness training
- Cognitive training
- Find a journal
- Publish with us
- Track your research
- Original article
- Open access
- Published: 29 December 2018
Mind-wandering rates fluctuate across the day: evidence from an experience-sampling study
- Gabriel King Smith ORCID: orcid.org/0000-0002-9164-1027 1 ,
- Caitlin Mills 1 , 2 ,
- Alexandra Paxton 3 &
- Kalina Christoff 1
Cognitive Research: Principles and Implications volume 3 , Article number: 54 ( 2018 ) Cite this article
6253 Accesses
18 Citations
29 Altmetric
Metrics details
Previous research has demonstrated reliable fluctuations in attentional processes during the course of the day. Everyday life experience sampling, during which participants respond to “probes” delivered at random intervals throughout the day on their mobile devices, is an effective tool for capturing such diurnal fluctuations in a naturalistic way. The existence of diurnal fluctuations in the case of mind-wandering, however, has not been examined to date. We did so in two studies. In the first study, we employed everyday experience sampling to obtain self-reports from 146 university students who rated the degree of free movement in their thoughts multiple times per day over five days. These time course data were analyzed using multilevel modelling. Freely moving thought was found to fluctuate reliably over the course of the day, with lower ratings reported in the early morning and afternoon and higher ratings around midday and evening. In the second study, we replicated these effects with a reanalysis of data from a past everyday experience-sampling study. We also demonstrated differences in parameter values for the models representing freely moving thought and two common conceptualizations of mind-wandering: task-unrelated thought and stimulus-independent thought. Taken together, the present results establish and replicate a complex pattern of change over the course of the day in how freely thought moves, while also providing further evidence that freedom of movement is dissociable from other dimensions of thought such as its task-relatedness and stimulus-dependence. Future research should focus on probing possible mechanisms behind circadian fluctuations of thought dynamics.
Significance
Mind-wandering has been linked to crucial parts of our daily lives, including learning, affect, and job productivity. While a number of studies have examined mind-wandering rates in everyday life, an implicit assumption has been that the rate is constant over the course of the day. The current research provides the first evidence suggesting that the extent of free movement in thought fluctuates reliably throughout our day. Thoughts appear to be most constrained in the morning and peaked in freedom of movement midday, increasing throughout the morning, decreasing throughout the afternoon, and finally increasing again in the evening. These findings may have important implications for education and the workplace, since freely moving thought could facilitate performance on tasks that require flexibility of thought (e.g. brain storming, solving novel problems), while leading to performances decrements on tasks that require stability of thought (e.g. algebra, sustained attention). Class and work schedules that adapt to the diurnal patterns of thought may lead to improved efficiency; future work should be done to explore this potential.
The last decade has seen a remarkable surge into mind-wandering research alongside its educational, clinical, and everyday life implications (Callard, Smallwood, & Margulies, 2012 ; Marchetti, Koster, Klinger, & Alloy, 2016 ; Mills, Raffaelli, Irving, Stan, & Christoff, 2017 ; Valdez, Ramírez, & García, 2014 ). Although there is no currently agreed-upon definition of mind-wandering (Christoff et al., 2018 ; Christoff, Irving, Fox, Spreng, & Andrews-Hanna, 2016 ; Irving, 2016 ; Seli et al., 2018 ; Seli, Risko, Smilek, & Schacter, 2016 ), there are a number of dimensions of thought that are being investigated for their presumed connection to mind-wandering. Task-unrelatedness and stimulus independence (i.e. cognition with little/no relation to external events; Schooler et al., 2011 ) have received the lion’s share of researchers’ attention so far (Mills, Raffaelli, et al., 2017 ; Seli et al., 2018 ). An additional dimension—freedom of movement in thought (i.e. the level of constraints on thought as it unfolds over time)—has more recently been highlighted as an important feature of mind-wandering (Christoff et al., 2016 ) and has been shown to be empirically dissociable from task-unrelatedness and stimulus-independence (Mills, Raffaelli, et al., 2017 ). One as-yet-unexplored question, however, is whether thought fluctuates systematically across the day along dimensions relevant to mind-wandering. Here, we provide the first such empirical investigation.
Until recently, a common approach in mind-wandering research was to implicitly equate mind-wandering with task-unrelated thought; however, this practice is no longer tenable due to recent developments in theoretical work on this topic (Christoff et al., 2018 ; Seli et al., 2018 ). Instead, mind-wandering is increasingly being recognized as a phenomenon that has so far evaded a one-to-one correspondence with any particular dimension of thought (Christoff et al., 2018 ). Although no consensus has yet been reached as to how mind-wandering relates to freedom of movement in thought, task-unrelatedness, and stimulus-independence, previous work upholds the relevance of these dimensions to understanding mind-wandering and its implications for everyday life.
In the present work, we chiefly focus on freedom of movement in thought as a dimension of thought relevant to mind-wandering and we offer (to our knowledge) the first empirical examination of its daily fluctuations (Study 1). We also examine how its diurnal fluctuations compare to the fluctuations of task-relatedness and stimulus-independence (Study 2) to present a fuller picture of the different dimensions of thought that have been linked to mind-wandering so far.
Our focus on freedom of movement stems from the potential ability of this dimension to conceptually distinguish between streams of thought that are more constrained (such as ruminative or goal-directed thoughts) and spontaneously unfolding streams of thought—an experience that is often intuitively considered to occur when the mind wanders. Within the Dynamic Framework of Thought (Christoff et al., 2016 ) which emphasizes how thoughts unfold over time, different sources of constraints—for example, automatic (e.g. habits, affect, salient distractors) and deliberate constraints (e.g. goal-directed focus)—dynamically influence the way thoughts unfold over time. In this model, some thought streams are more constrained (or focused on a particular topic) while other thought patterns are more “free,” i.e. they are less likely to be focused on a particular topic and will tend towards greater content variability over time (Mills, Herrera-Bennett, Faber, & Christoff, 2018 ). For example, night-time dreaming is considered to have low levels of constraint and thus higher degrees of freedom that are less bound to reality, compared to creative thinking which involves spontaneity but also elements of topical constraint; however, both can be considered forms of spontaneous thought (Christoff et al., 2016 ). Freedom of movement in thought would vary on a continuum from high to low based on the amount of net constraints that operate on the dynamic flow of thought at any moment, which can come from a variety of sources including (but not limited to) conscious or latent goal pursuits, habits, as well as perceptual or emotional demands.
Thoughts that are considered to have lower levels of constraint – i.e. “freely moving” thoughts – can be distinguished from other thought streams such as focused goal-directed thought and ruminative thoughts (which are influenced by imposing higher levels of constraints). Task-relatedness, despite being the most common thought dimension examined in mind-wandering research so far, does not distinguish whether a train of thought unfolds more spontaneously or in a more constrained manner. For example, rumination occurs in a constrained and fixated manner by definition (Nolen-Hoeksema, Wisco, & Lyubomirsky, 2008 ), which contrasts to the phenomenological experience of daydreaming; yet this contrast cannot be captured by the off-task dimension alone. Because of these distinctions, freedom of movement in thought seems to have particular relevance to mental processes in everyday life, where stimuli and tasks are much less well-defined than in an experimental context.
The majority of empirical studies on mind-wandering to date have taken place in a single session of either experience sampling in the laboratory (Franklin, Smallwood, Zedelius, Broadway, & Schooler, 2016 ; Krawietz, Tamplin, & Radvansky, 2012 ; Mills & D’Mello, 2015 ; Phillips, Mills, D’Mello, & Risko, 2016 ; Smallwood, Fitzgerald, Miles, & Phillips, 2009 ; Smallwood, Nind, & O’Connor, 2009 ; Stawarczyk, Majerus, Maj, Van der Linden, & D’Argembeau, 2011 ; Unsworth & McMillan, 2013 ) or during a single resting state functional magnetic resonance imaging (fMRI) scan (Ellamil et al., 2016 ; Fox, Nijeboer, Solomonova, Domhoff, & Christoff, 2013 ; Fox, Spreng, Ellamil, Andrews-Hanna, & Christoff, 2015 ; Kucyi & Davis, 2014 ; Mills, D’Mello, & Kopp, 2015 ; Phillips et al., 2016 ). However, because many studies are conducted between the hours of 09:00 and 17:00, they may be missing out on consistent variability in the daily cycle by collapsing results over the entire day.
The possibility that mind-wandering rates (as assessed by any of the three dimensions of thought mentioned above) may be influenced by daily cycles has been relatively ignored, despite a large body of work suggesting that time-of-day influences attention (as assessed via vigilance and selective attention tasks) as well as a wide array of cognitive faculties (Carrier & Monk, 2000 ; Folkard & Monk, 1987 ; Gabehart & Dongen, 2017 ). Much research in this field has been devoted to charting performance on attention-demanding cognitive tasks over the course of the day and has been successful in identifying a daily fluctuation pattern that closely parallels the rise and fall of core body temperature. Coincidentally, in parallel with the rising popularity of research on mind-wandering, analysis techniques that expand beyond simply averaging to include dynamic properties over time have also gained popularity in recent years (Allison, 2014 ; Dziak, Li, Tan, Shiffman, & Shiyko, 2015 ; Singer & Willett, 2003 ).
One way to address this gap in the literature is by assessing subjective ratings of thought over time in the real world: participants’ natural everyday life setting. So-called everyday life experience-sampling studies probe at random times throughout the day, typically using a mobile device to allow participants to answer questions (Franklin et al., 2013 ; Jazaieri et al., 2016 ; Killingsworth & Gilbert, 2010 ; McCormick, Rosenthal, Miller, & Maguire, 2017 ; McVay, Kane, & Kwapil, 2009 ; Song & Wang, 2012 ; Spronken, Holland, Figner, & Dijksterhuis, 2016 ). This methodology is frequently used to assess mental states over a period of time, yet longitudinal changes are often ignored in the subsequent analyses.
The present study
Here we investigate the patterns of mind-wandering across the day in two experience-sampling studies. Study 1 focuses specifically on freely moving thought: participants were probed randomly throughout the day for five days on their cell phones as they went about their regular routines. We hypothesized that ratings of freely moving thought would significantly fluctuate throughout the day.
In Study 2, we present a new analysis of a previously published experience-sampling dataset (Mills, Raffaelli, et al., 2017 ) in an attempt to replicate findings from Study 1. We also ask whether different conceptualizations of mind-wandering (i.e. freely moving thought, task-unrelated thought, and stimulus-independent thought) exhibit different fluctuations over the course of the day. This idea is inspired by Mills, Raffaelli, et al.’s ( 2017 ) original finding of low overall correspondence between these distinct forms of mind-wandering; the present study, then, builds on that work to explore whether they share similar dynamics.
In the interest of open science, we have made our code and data freely available on the Open Science Framework ( https://osf.io/es3gf/ ), and our code is additionally available on GitHub ( https://github.com/galagon/mind-wandering-fluctuations ).
Participants
A total of 144 participants were recruited from a large public Canadian university and were compensated for their participation with class credit. All instructions were given in English. The language of the participants was assumed to be the same: proficiency in English is an enrollment requirement for the university and only enrolled students were eligible to participate.
All study procedures were reviewed and approved by the UBC Behavioural Research Ethics Board.
The study included an in-lab component and an experience-sampling component. First, participants underwent a 30-min training session in the lab before beginning, starting with the completion of an informed consent form and a ~ 20-min learning portion. This portion included detailed verbal instructions given via a video-recorded slideshow. The video explained the procedure, gave detailed examples for all relevant definitions, and was paused periodically to enable the experimenters to answer participants’ questions about the study and ask participants to generate novel examples of different kinds of thought. Portions of the exact script used in the video are included in Appendix 1 . Finally, before leaving the lab, participants were asked to keep their phone near them at all times and respond to as many probes as possible unless it was dangerous to do so (e.g. driving).
Participants were prompted to answer probes 20 times per day for five days (yielding up to 100 total responses to probes per participant) via URL links sent directly to their mobile phones in a text message. Each probe included the question “Was your mind moving about freely?”, which was answered on a scale from (1) Not at all to (7) Very much . If it was the first probe answered that day, participants were also asked what time they had awoken that day and what time they had begun to sleep the previous night. Probes were answered from Tuesday through Saturday.
A total of 10,287 responses were collected (M = 71.4 responses per participant, SD = 21.0). Similar to Mills, Raffaelli, et al. ( 2017 ), participants who answered 60 out of 100 probes or fewer (36 participants out of 144) were dropped from the analyses to remove participants who did not follow instructions to answer sufficient thought probes for analysis. The final sample was composed of 108 participants who answered an average of 80.2 probes (SD = 14.1).
Formal and accurate a priori power calculations proved difficult due to the lack of information regarding effect sizes of daily changes, particularly when it came to models that incorporate multiple time terms and mixed effects as described below. In light of this, we aimed to gather as many participants as we could within a limited time window in order to maximize power, resulting in the sample size of 144 participants initially and 108 participants after exclusions. However, post-hoc observed power estimations are reported in the discussion to help with the planning of future research.
To ensure that our results were not driven by exclusion criteria, all analyses reported below were repeated with the complete dataset (i.e. no participant removal). The pattern of results remained unchanged with one exception in Study 2, noted below.
Data preparation
To compress the data into bins, the time at which each probe was received was rounded down to the hour and participants’ ratings of freely moving thought were averaged for each hour of the day, collapsing across different days. For some hours of the day, relatively few probe responses were logged (see Table 1 for a summary of hours with at least one probe response). Fewer than one-quarter of all participants answered a single probe during the hours of 06:00 and 07:00 (all times local and based on the 24-h clock); because of this, data pertaining to those hours were excluded from all analyses. Both Table 1 and Fig. 2 show the average freely moving thought rating for each hour of the data.
Approach to data analyses
Our central research question was to determine if ratings of freely moving thought fluctuate in reliable patterns over the course of the day. To answer this question, we modeled ratings of freely moving thought with the hypothesis that they would vary as a function of time. We used a mixed-effect multilevel modelling approach to test this hypothesis because: (1) it assesses change over time while preserving between-participant differences in baseline ratings (intercept) and estimated relationships (slope; Fuller-Tyszkiewicz et al., 2017 ); and (2) it is robust to missing data (Mirman, 2016 ). Below, we describe our analytical approach using current best-practices for growth curve modeling; interested readers can find more detail in Mirman ( 2016 ), Mirman, Dixon, and Magnuson ( 2008 ), and Singer and Willett ( 2003 ).
We tested the patterns of freely moving thought ratings by constructing a mixed-effects model with first- through third-order orthogonal polynomial terms (for comparative examples of the three orders of polynomial models; see Fig. 1 ). All polynomial terms were orthogonalized to avoid multicollinearity and to ensure independence from one another (see Mirman, 2016 ). The significance of each term would shed light on a specific pattern, though none are mutually exclusive (see Fig. 1 ):
the linear term (first order) would represent a general increase or decrease in freely moving thought over the day;
the quadratic term (second order) would fit the data if ratings were higher or lower in the middle of the day as compared to morning and evening; and
the cubic term (third order) would capture trends that changed direction twice over the day—for example, if the ratings rose throughout the morning, fell in early afternoon (i.e. first change in direction), and rose again through the evening (i.e. second change in direction).
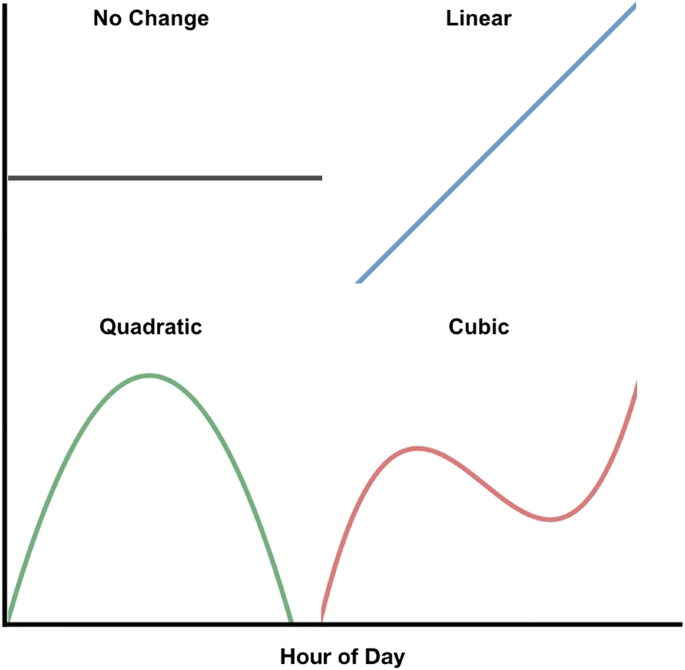
Examples of polynomial models representing change across time. The black line represents no change across time (intercept-only); the blue line represents linear change; the green line represents quadratic change; the red line represents cubic change
We used polynomials to model change across time for three reasons, aside from such models being commonly used in the analysis of temporal dynamics (Hartmann et al., 2016 ; Mirman et al., 2008 ; Papageorghiou et al., 2014 ; Shin, 2012 ; White, Xie, Thompson, Loeber, & Stouthamer-Loeber, 2001 ). First, they can easily be orthogonalized with respect to one another, allowing us to interpret the individual contributions of each polynomial without the issue of collinearity. Second, they represent increasingly complex forms of change over time, which is important as we did not believe that simple patterns of change (e.g. linear) were likely given the literature on daily fluctuations of other cognitive variables (Burke, Scheer, Ronda, Czeisler, & Wright, 2015 ; Fimm & Blankenheim, 2016 ; Folkard & Monk, 1987 ; Giambra, Rosenberg, Kasper, Yee, & Sack, 1989 ; Goel, Basner, Rao, & Dinges, 2013 ; Riley, Esterman, Fortenbaugh, & DeGutis, 2017 ; Silva, Wang, Ronda, Wyatt, & Duffy, 2010 ; Valdez et al., 2005 ).
Finally, modeling data with these three polynomials served as a first step in understanding the dynamics of mind-wandering using some of the simplest and most commonly used forms of change found in other diurnal cognitive studies. Given the lack of previous work in this domain, we had no reason to suspect that any more complicated time-varying forms of change would provide a better fit; therefore, the choice of more specific and nuanced models would have been arbitrary. While some past studies have attempted to precisely and mechanistically model daily change in a cognitive outcome variable (Jewett & Kronauer, 1999 ), such models were based upon a deep and established literature that does not yet exist for freely moving thought.
Model construction
All analyses were performed in R (R Core Team, 2017 ). Models were constructed using the lme4 package (Bates, Mächler, Bolker, & Walker, 2015 ) and parameters were tested for significance with the lmerTest package (Kuznetsova, Brockhoff, & Christensen, 2017 ). All variables were centered and standardized before entry into the model, allowing us to interpret the resulting standardized estimates as effect sizes (Keith, 2005 ).
We constructed a model predicting freely moving thought ratings using each orthogonal polynomial as fixed effects without interaction terms. We used participants as our sole random intercept with the maximal slope structures that permitted model convergence (Barr, Levy, Scheepers, & Tily, 2013 ). All models were estimated using unrestricted maximum likelihood estimation, with an unstructured covariance structure.
For completeness, we also compared nested models to identify which model provided the best “fit” for the data. In this step, we constructed hierarchical models with successively higher-order time terms (i.e. intercept-only, linear, quadratic, and cubic) and tested them for improved fit over the previous iteration using chi-square tests. Ultimately, the full model (i.e. cubic model) best accounted for the data. Results from these hierarchical comparisons are reported in our supplementary materials (Additional file 1 ).
Model interpretation
We would find support for our hypothesis that ratings of freely moving thought fluctuate reliably over the course of the day if our model found significance for any time parameter (i.e. linear, quadratic, or cubic; see supplementary materials (Additional file 1 ) for graphical representations of each fitted model). Model parameter values can generally be interpreted as follows:
positive linear parameters would indicate that ratings increased throughout the day;
positive quadratic parameters would indicate that ratings were higher at the beginning and end of the day compared to the middle; and
positive cubic parameters would indicate that ratings increased at the beginning of the day, decreased in the middle of the day, and increased again at the end of the day.
Standardization of ratings meant that the resulting coefficients of the model represent standardized (β) not raw (B) coefficients. This allows different predictors to be directly comparable (Keith, 2005 ). For example, if the coefficient for cubic change is higher than that for the linear change, it indicates the influence of the cubic parameter is greater or that the data conform more to a cubic form of change than a linear one.
Overall, freely moving thought ratings trended towards the center of the scale with a mean rating of 4.11 ( SD = 1.92). As hypothesized, the results indicate that freedom-of-movement in thought did indeed fluctuate in a reliable pattern over the course of the day, as both the quadratic and cubic terms were significant. Table 2 shows the values and significance tests for each of the model parameters.
Figure 2 a shows this change over the course of the day. Ratings of freely moving thought were lowest in the morning (in the 08:00 h) and steadily increased until midday, before declining again. The significance of the negative quadratic term is representative of the parabolic quality of daily change in which ratings start and end low, peaking somewhere in between. The presence of the positive cubic term elaborates on this simple relationship: the peak occurs early in the day (around noon) and is followed by a decreasing pattern that changes direction and increases in the evening.
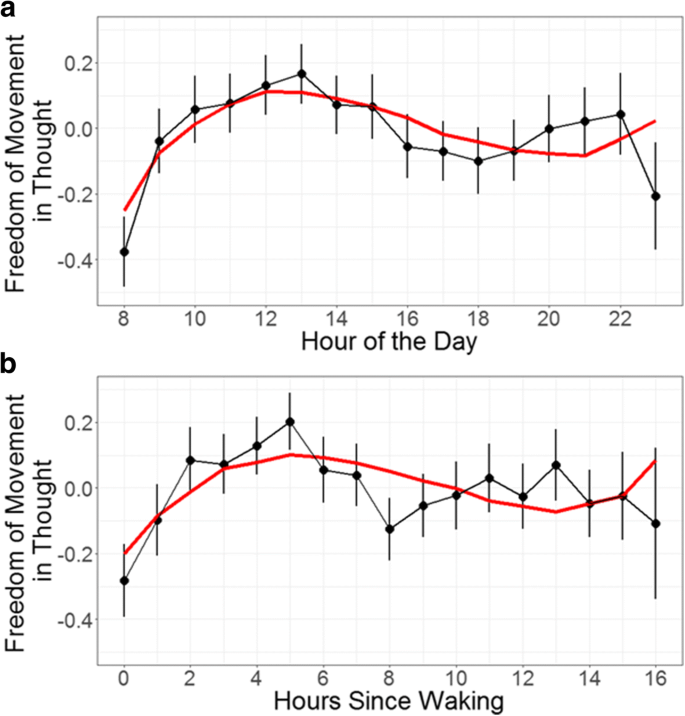
Freedom-of-movement in thought ratings (1–7) ( a ) averaged for each hour of the day and ( b ) averaged for each hour since waking. Error bars represent the standard error. Red lines represent the best-fit cubic models
The data were also analyzed with respect to freely moving thought ratings as a function of hours awake in the day, using the self-reported wake times of participants (Fig. 2 b). A similar cubic relationship was found, although the quadratic term became non-significant. Visual inspection of the form of change indicates a high degree of similarity in the two patterns, strengthening the above findings by indicating that at least the cubic pattern of change cannot be explained by variable sleep habits among participants confounding freely moving thought ratings (for full results, see Appendix 2 ).
Results from Study 1 supported our hypothesis that rates of mind-wandering change in complex patterns throughout the day. We found that participants’ thoughts were more constrained in the morning and gradually became more freely moving across the beginning of the day, peaking around noon, before falling in the afternoon and then rising again in the evening. These findings provide initial support for the idea that mind-wandering dynamically fluctuates in our everyday lives, which is important given its relationship to educational outcomes (Mills, Graesser, Risko, & D’Mello, 2017 ; Mrazek et al., 2017 ; Pachai, Acai, LoGiudice, & Kim, 2016 ; Seli, Wammes, Risko, & Smilek, 2016 ; Sousa, Carriere, & eSmilek, 2013 ; Valdez et al., 2014 ) and workplace productivity (Dane, 2011 ; Dust, 2015 ; Hyland, Lee, & Mills, 2015 ).
Study 2: reanalysis of Mills, Raffaelli, et al. ( 2017 )
Demonstrating replicability of the results from Study 1 would be highly desirable given the novelty of the research question and the exploratory nature of interpreting polynomial models assessing changes over time. Towards this goal, we conducted a novel analysis of data from Mills, Raffaelli, et al. ( 2017 ), a published experience-sampling study in everyday life that included probe timestamp information that had never been analyzed. Reanalyzing this dataset also enabled us to address the question of whether three distinct conceptualizations of mind-wandering (freely moving thought, task-unrelated thought, and stimulus-independent thought) displayed dissociable fluctuations across the day.
A complete description of the method from the original study can be found in Mills, Raffaelli, et al. ( 2017 ), but we provide a very brief overview here. The methodology was identical to that used in the Study 1 with four exceptions. First, the sample included 226 people initially, with 165 participants retained after removing those who answered 60% or fewer probes completely. Second, probes were given 10 times a day for a period of 10 days, yielding a maximum of 100 probes per participant (the same per-participant maximum as Study 1). Third, the questions concerning sleep and wake time were not included; instead, every probe asked participants to rate their task-unrelated thought (“Were you thinking about something other than what you were doing?”) and stimulus-independent thought (reverse-coded; “Were you aware of your surroundings?”) on a scale of 1 to 7. (Probes also included other questions that were not relevant to the present study; see Mills, Raffaelli, et al., 2017 .) Fourth, participants received probes all days of the week but only between the hours of 08:00 and 23:00 (all times local and in the 24-h clock).
Data preparation and analysis
Similar to the analytical approach in Study 1, probes were rounded down to the hour and aggregated by person and hour. Unlike the original study, the probed hours were in the range of 08:00 to 23:00 (Mills, Raffaelli, et al., 2017 ). Only hour 23:00 was removed as it contained responses from < 25% of participants (Table 1 ). Figure 3 displays the mean ratings for each hour of the day for each dimension.
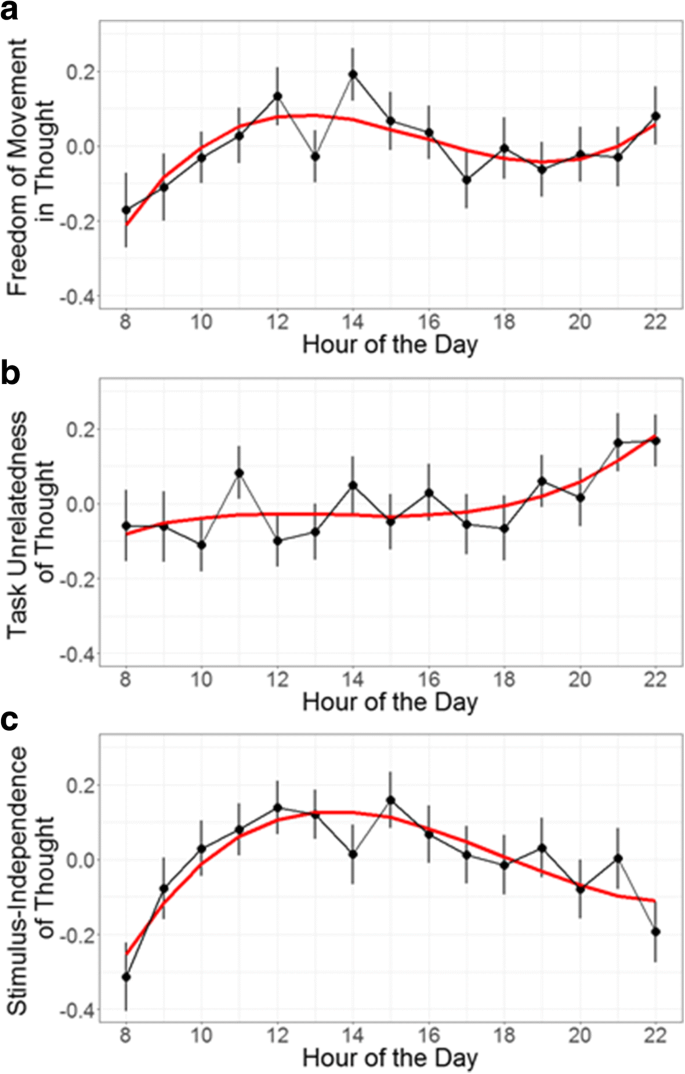
Thought dimension ratings displayed by the hour of the day. Error bars represent standard errors. Red lines represent predictions of the optimal model for each dimension (cubic in all cases): ( a ) freedom-of-movement in thought, ( b ) task-unrelatedness of thought, ( c ) stimulus-independence of thought
Statistical analyses were conducted with the same packages in R (lme4, Bates et al., 2015 ; lmerTest, Kuznetsova et al., 2017 ), the same fixed and random effect constraints, the same maximum likelihood effect estimation, and the same unstructured covariance structure as used with the original data. Again, all data were standardized before entry in the model, resulting in standardized (β) rather than unstandardized (B) coefficients. As with Study 1, hierarchical models were created with increasing orders of polynomial time-varying factors and compared on goodness of fit; the results of these comparisons as well as graphics representing the fitted values for each model can be found in our supplementary materials (Additional file 1 ).
The average rating (per participant) for each of the three definitions clustered toward the center of the scale: the mean freely moving thought rating was 4.03 (SD = 1.73), the mean task-unrelated thought rating was 4.08 (SD = 1.74), and the mean stimulus-independent thought rating was 4.19 (SD = 1.64).
We present each model’s results below. For simplicity, we do not include test statistics in the text; instead, model results are provided in Tables 3 , 4 , 5 and 6 .
Freely moving thought (Table 3 )
Findings for freely moving thought closely replicated results from Study 1. As in the original dataset, both the quadratic and cubic terms reached significance, but the linear term did not. Notably, when the analyses were run without the removal of participants who did not answer a sufficient number of probes, the linear term became significantly positive, likely as the result of higher freedom-movement ratings in the latest hours (for more, see supplementary materials (Additional file 1 ).
- Task-unrelated thought
Ratings of task-unrelated thought showed linear change over the course of the day, but no other time-varying model parameters achieved significance (Table 4 ). The positive linear parameter indicates that the ratings increased significantly between the beginning and the end of the day. This contrasts with ratings of freely-moving thoughts, which showed quadratic and cubic change but not linear.
- Stimulus-independent thought
Ratings of stimulus-independent thought showed similar patterns of change over the day as freely moving thought, exhibiting quadratic and cubic fluctuation without linear change (Table 5 ).

Comparing different dimensions of thought
Mills, Raffaelli, et al. ( 2017 ) found that freely moving thought could be distinguished from content-based definitions of mind-wandering (i.e. task-unrelated thought and stimulus-independent thought). In the present study, we provide a novel test of this claim by assessing whether these three dimensions of thought exhibit differential patterns of change across the day. Although we found that two of the dimensions (freedom-of-movement and stimulus-independence) showed similar patterns of change with the same model parameters being significant for each dimension, the individual slopes that make up the equations may be significantly different. In other words, the steepness of the curves may differ.
We tested this possibility by combining responses to all three questions in a dataset with a three-level categorical variable that we call thought dimension (Table 6 ). We then created a cubic model that included the main effect of thought dimension and its interactions with each of the three time terms. A non-significant interaction between thought dimension and a time term indicates that the given slope does not differ substantially between the three dimensions of thought, whereas a significant interaction indicates that the dimensions have dissociable slopes.
By default, the lmer function of the lme4 package in R uses treatment contrasts, meaning that it treats interactions involving categorical variables as pairwise comparisons between the first entered level of the variable (in this case, ratings of freely moving thought) and each level after that (task-unrelatedness and stimulus-independence). An analysis comparing task-unrelatedness and stimulus-independence directly can be found in the supplementary materials (Additional file 1 ), though we chose not to include it in the main text both to minimize the number of tables and to focus on our main research question: does freely moving thought have a distinct daily pattern compared to the dominant, content-based conceptualizations of mind-wandering?
Although there were no significant interactions between dimensions of thought and the linear or cubic terms, the results show an interaction between the quadratic term and the dimension variable with both comparisons reaching significance. The quadratic slope for ratings of freely moving thought was significantly steeper (i.e. more negative) than that of task-unrelated thought yet significantly shallower (i.e. less negative) than that of stimulus-independence thought. This pattern can be observed through visual inspection of the data (Fig. 3 ): freely moving thought ratings experience a rise and fall pattern over the course of the day and peak close to the middle of the day, a relationship that is exaggerated for stimulus-independent thought and absent for task-unrelated thought.
These differences in changes across the day further highlight the dissociability of the three dimensions and indicate that they may be associated with only partially overlapping neural processes or may arise from distinct constraints on agent-environment interactions.
Weekend-only analysis
The greatest advantage of everyday life experience sampling—the fact that ratings come from participants living their daily lives and not conducting artificial laboratory tasks—comes with its own set of limitations. Without controlling the conditions under which participants answer, it is difficult to know whether the observed fluctuations are caused by intrinsic mechanisms such as diurnal rhythms or whether they are simply the product of the activities in which participants were more or less likely to be engaged in at different points in the day. For example, ratings of freely moving thought were highest around midday, a time when the study’s participants—undergraduate students—would be more likely to be in the middle of classes as compared to the evening or early morning. Aside from classes, this pattern could be driven by participants taking lunch breaks around the noon hour or, conversely, by exhaustion after long periods of intensive effort exerted in morning classes.
Although the primary goal of the study was to determine whether reliable daily fluctuations exist and not to determine their source, the reanalyzed dataset affords the opportunity to examine weekdays and weekend days separately. This exploratory analysis rests on the tentative assumption that daily activities on weekdays (e.g. classes, work) are scheduled differently than weekends for most participants. Thus, if daily fluctuations in thought are identical across weekdays and weekend days despite those days containing different sets of activities, there is evidence that activities alone may not account for these daily patterns of thought.
After removing probes answered during weekdays, 4305 of the original 12,997 probes (33.12%) remained. In order to keep the results comparable, the same participants in the full analyses were used for the weekend-only analyses, regardless of the number of probes answered after the removal of weekday probes. The analysis was limited to the reanalyzed dataset from Mills, Raffaelli, et al. ( 2017 ) due to an insufficient number of probes answered over weekend days in the dataset from Study 1.
All model results are available in Appendix 3 . For brevity and clarity, we do not include most test statistics in the text, but graphical representations of the data and optimal cubic models can be seen in Fig. 4 . The model for freely moving thought revealed a highly similar pattern of results, but neither the quadratic nor the cubic term quite reached statistical significance (quadratic: p = 0.056, cubic: p = 0.054; Fig. 4 a). Although the lack of significance at α = 0.05 may indeed indicate a different pattern of fluctuation across the day, the loss of power accompanying a threefold reduction in the number of probes entered into the model may be an alternative reason for this disparity, especially given that the p values in question, while no longer significant, are still < 0.06. While we cautiously interpret the pattern for freely moving thought to be similar for the weekend-only analysis, future work with greater statistical power may be able to shed more light on this possibility.
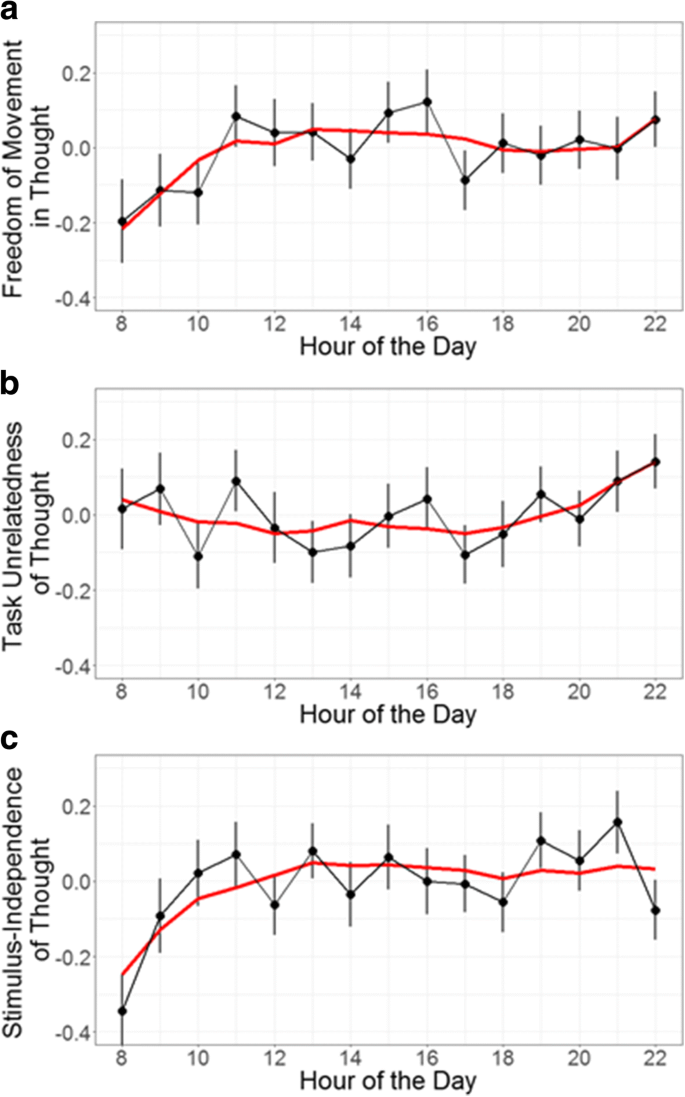
Thought dimension ratings for only weekend probes displayed by the hour of the day. Error bars represent standard errors. Red lines represent predictions of the optimal model for each dimension (cubic in all cases): ( a ) freedom-of-movement in thought, ( b ) task-unrelatedness of thought, ( c ) stimulus-independence of thought
On the other hand, we were unable to replicate the findings for the two content-based dimensions of thought. In the model predicting task-unrelatedness of thought, no time-varying parameters reached significance. In the model predicting stimulus-independence of thought, the positive linear term reached significance and the positive cubic term no longer reached significance (although it trended toward significance in the same direction), while the quadratic term remained negative and significant.
We note, however, that visual inspection of the weekend-only data indicates a highly similar pattern for task-unrelatedness of thought (Fig. 4 b). This again suggests that the difference from the original analysis is possibly the result of power loss (though it is worth noting that in this case the linear term did not trend towards significance). The stimulus-independence data, on the other hand, does appear to exhibit a different relationship (Fig. 4 c). Visual inspection indicates that stimulus-independence scores are higher in the evenings, which would account for the increase in the strength of the linear parameter (since the scores at the end of the day are now higher than the those at the beginning) as well as the attenuation of the cubic parameter (since the scores remain comparatively stable after midday and do not dip in the afternoon or evening).
The reanalysis of Mills, Raffaelli, et al. ( 2017 ) in Study 2 provided three major conclusions. First, ratings of freely moving thought followed a pattern of quadratic and cubic change across the day with an absence of linear change, replicating the findings from Study 1. This pattern was also observed visually —although, crucially, it failed to reach statistical significance—when analyzing only weekend data; the compatible visual and statistical trends in the data, however, suggest that the lack of statistical significance may have been due to a severe drop in statistical power by focusing only on two out of seven days of the week.
Second, two dominant, content-based dimensions of thought were also found to fluctuate across the day. Stimulus-independent thought exhibited a form of change similar to freely moving thought, while task-unrelated thought showed a simple increase in ratings throughout the day. These patterns were less reliable when analyzing weekend-only data, with stimulus-independent thought in particular appearing to exhibit a different form of daily change.
Third, the three dimensions of mind-wandering were empirically dissociable based on their quadratic parameters. Specifically, stimulus-independent thought exhibited a much stronger rise-and-fall pattern of higher scores in the midday compared to the beginning and end of the day, in comparison with freely moving thought. On the other hand, such a pattern was completely absent for task-unrelated thought.
General discussion
Here, we presented—for the first time—evidence that thought patterns fluctuate across the day. Capturing the “wandering” mind by measuring freely moving thought, we probed people on their smartphones during their daily lives to understand the daily rhythms of thought in the “wild.” We found that freely moving thought occurs at lower rates early in the day, rises until midday, and declines gradually before rising once more in the evening. By reanalyzing a dataset from Mills, Raffaelli, et al. ( 2017 ), we were able to replicate these results and investigate novel questions about the dynamics of specific kinds of mind-wandering—in particular, we found that freely moving thought showed distinct daily patterns from two other dominant conceptualizations of mind-wandering. Such findings are consistent with a host of previous research showing that human cognition exhibits diurnal dynamics, not uniform properties over time.
What might drive diurnal dynamics?
Although these results provide novel evidence that mind-wandering does dynamically fluctuate throughout the day, they do not speak to why these changes occur. We describe a few possible—but not mutually exclusive—explanations for the patterns of change. However, we recognize that much more work is required to elucidate the mechanisms driving these results, and we present these as testable hypotheses for additional future work.
The first potential mechanism is autonomic arousal. Arousal may bias attentional selectivity by way of the locus coeruleus, a remarkably well-connected norepinephrinergic brain region (Aston-Jones & Cohen, 2005 ; Aston-Jones & Waterhouse, 2016 ; Gilzenrat, Nieuwenhuis, Jepma, & Cohen, 2010 ; Mather, Clewett, Sakaki, & Harley, 2016 ). Although a direct link between arousal and the freely moving thought conceptualization of mind wandering has not been established, Mittner, Hawkins, Boekel, and Forstmann ( 2016 ) recently suggested that levels of norepinephrine originating from the locus coeruleus may differentiate between mental states that are focused on a single subject (regardless of whether the subject is the task at hand) and “exploratory,” unstable states—similar to the distinction between constrained and freely moving thought.
Indeed, diurnal fluctuations in norepinephrine align well with the pattern of results found here: an increase early in the day, a lunchtime peak, and a gradual afternoon/evening decline (Fibiger, Singer, Miller, Armstrong, & Datar, 1984 ; Hansen, Garde, Skovgaard, & Christensen, 2001 ). Previously observed fluctuations do not map perfectly onto the cubic structure of mind-wandering observed in the present dataset, given that levels of norepinephrine generally do not increase over the course of the evening. However, both share noticeable similarities throughout the rest of the day. The same pattern exists for attentional performance as well (Carrier & Monk, 2000 ; Silva et al., 2010 ; Valdez et al., 2005 ), suggesting that arousal may be a common mechanism behind these daily changes.
The arousal-based account may also be able to partially explain a somewhat-paradoxical finding from our study: mind-wandering rates tended to be at their highest during the times when past studies have indicated attentional abilities are at their peak and they tended to be at their lowest during the times when attentional performance is purported to suffer (Carrier & Monk, 2000 ; Folkard & Monk, 1987 ; Valdez et al., 2005 ). This co-fluctuation seems counter-intuitive, as the relationship between mind-wandering and performance on sustained attention tasks is often considered antithetical (McVay & Kane, 2009 ; Smallwood, Fitzgerald, et al., 2009 ; Stawarczyk et al., 2011 ). However, it is important to note that the majority of the studies that have found evidence of such an antithetical relationship have used task-unrelated thought as their definition of mind-wandering (oftentimes quite literally operationalized as the inverse of attentional performance); by contrast, freedom-of-movement of thought and attentional performance may not exhibit such a marked negative relationship. In light of this, the observed results would be perfectly in line with an arousal mechanism that impacts both attentional performance and thought dynamics but has no direct impact on the content of thought (or, at the least, not its task-relevance).
A second, simpler explanation for the observed daily fluctuations in mind-wandering may be circumstance or environmental pressure. Ratings of freely moving thought first peaked at the time of day when many participants (all of whom were students) would be in class and ratings dipped at times when they were would more likely to be home. It is therefore possible that the classroom environment may loosen constraints on thought or that other activities or states experienced at certain times of day produce the patterns seen here. Since we did not ask students to report their current activity at the time of each probe, this possibility cannot be completely discounted. However, findings for freely moving thought were partially replicated in a subset of the reanalyzed data that included only weekend days, suggesting that this pattern of change is likely independent of the day of the week, which one would not expect if it was driven by activities.
Finally, mind-wandering fluctuations may be tied to humans’ circadian rhythm in some other way. In two out of three dimensions of thought, we observed a rise-and-fall pattern that mirrors circadian body temperature changes that, in the past, have frequently been found to correlate with cognitive performance in a variety of domains (Carrier & Monk, 2000 ; Fimm, Brand, & Spijkers, 2016 ; Goel et al., 2013 ; Valdez, Reilly, & Waterhouse, 2008 ; Wright, Hull, & Czeisler, 2002 ). Although it would have been impractical to record temperature in an everyday life experience-sampling study, there is room for future experimental research to elaborate on the current findings by determining whether freely moving thought exhibits the same correlation with core body temperature as attention, spatial awareness, and other cognitive variables.
We recognize that our study is unable to answer these questions definitively. Perhaps some, all, or none of these explanations even work together: for example, arousal’s influence on mind-wandering could be dependent on current activity or level of motivation. As a result, we call for future work to collect the information that would be able to disentangle these effects, including concurrent measures of arousal, motivation, physiological states, and information about what participants are doing at the time of the probe responses. Such work should also continue to explore the dynamics of the three types of mind-wandering to see how, whether, and when they differ from one another.
Implications
This paper lays the groundwork for understanding daily fluctuations in mind-wandering—a topic that may have implications for shaping real-world policy. Indeed, there is already ample evidence linking one dimension of thought related to mind-wandering (task-unrelatedness) to affect (Killingsworth & Gilbert, 2010 ), creativity (Baird et al., 2012 ), and—perhaps most compellingly for public policy—impaired comprehension and classroom performance (Mills et al., 2017 ; Mrazek et al., 2017 ; Pachai et al., 2016 ; Seli, Wammes, et al., 2016 ; Sousa et al., 2013 ; Valdez et al., 2014 ).
As a result of the link between traditional task-unrelated mind-wandering and learning, educational policy has an enormous potential to be shaped by this and other mind-wandering research. The negative association between task-unrelated thought and text comprehension has been extensively replicated (Franklin, Smallwood, & Schooler, 2011 ; Mrazek et al., 2017 ; Pachai et al., 2016 ; Seli, Wammes, et al., 2016 ; Smallwood, McSpadden, & Schooler, 2008 ; Sousa et al., 2013 ). Additional work suggests that this relationship may be partially explained by mind-wandering’s interference with students’ ability to adaptively adjust their cognitive resources to the current task demands (Mills et al., 2017 ).
The dynamic fluctuations in mind-wandering found here raise the question of whether students’ schedules can or should be adapted around their cognitive cycles. For example, our findings suggest that early afternoon classes may coincide with peak rates of freely moving thought and stimulus-independence—perhaps making them poor times at which to hold class. However, it is currently unclear what an optimal schedule could be, given that past research has demonstrated learning deficits in early morning classes as well due to factors such as sleepiness (see Valdez et al., 2014 ).
A contrasting point of view is that mind-wandering is not a hindrance to all educational outcomes, particularly ones that require creativity (Baird et al., 2012 ; Dijksterhuis & Meurs, 2006 ; Ellamil, Dobson, Beeman, & Christoff, 2012 ; Pachai et al., 2016 ). From this standpoint, school administrators may actually wish to structure class times to take advantage of the benefits of mind-wandering (freely moving thought, in particular), not just avoid the downsides. For example, writing and artistic classes may benefit from being held around midday, when freely moving thought is highest and constraints are lowest. Classes that require a narrower focus of attention (e.g. STEM courses) might be more effective if they are held when freely moving and task-unrelated thought are at their lowest.
It is also important to consider how daily fluctuations in thought influence performance in the workplace. Industrial-organizational research has highlighted the detrimental effects of task-unrelated thought on workplace performance (Dane, 2011 ;Dust, 2015 ; Hyland et al., 2015 ) as well as its potentially positive effects (Dust, 2015 ; Hyland et al., 2015 ). In driving simulations, task-unrelated thought has been associated variously with poorer speed control (Baldwin et al., 2017 ; Yanko & Spalek, 2014 ), slower reaction times (Yanko & Spalek, 2014 ), and a lack of peripheral awareness (He, Becic, Lee, & McCarley, 2011 ). Similarly, in real-world correlational studies, this kind of mind-wandering has been associated with risky or aggressive driving patterns (Qu et al., 2015 ) and responsibility for car accidents (Galéra et al., 2012 ). Understanding the times of day we are most likely go off-task, have unconstrained thoughts, or be unaware of our surroundings, could be a critical consideration for occupational schedules as well.
Limitations and future directions
Of course, as an early and exploratory study, our work has a number of limitations and we see many of them are prime opportunities for future work. First, we asked people to introspectively report on their thoughts; participants’ introspective abilities have been the subject of much debate in the past (Hurlburt, Alderson-Day, Fernyhough, & Kühn, 2017 ; Nisbett & Wilson, 1977 ). However, replication of results across two independent datasets provides some evidence that individuals were responding reliably to the introspective questions. Additionally, extensive training and instructions were included during the training session to compensate for the novelty of the freely moving thought definition. Future work should strive to complement these studies by assessing the phenomenological experience of freely moving thought through its contents over time, as well as its objective signatures through physiological signals (Faber, Bixler, & D’Mello, 2018 ; Mills, Bixler, Wang, & D’Mello, 2016 ; Mills & D’Mello, 2015 ).
Second, we had no experimental control over participants, since the study was conducted in everyday life. This was necessary to maximize ecological validity but means that we had no way of knowing whether participants answered accurately and no way to compel them to answer rapidly. While this provided us with valuable insight into real-world processes, future work should complement naturalistic research (like the present study) with experimental designs that could shed light on processes, mediations, and causal mechanisms.
Third, in Study 1 only 71.4% of all probes were answered on average in the original dataset (80.2% after discarding low responders), leading to some variability in response rates across the hours of the day (see Table 1 ). This is particularly a problem for hour 23:00, as only 53 participants (fewer than half) answered a single probe during this time period, resulting in a high standard error. As a consequence, it is unclear whether the sharp drop in freely moving thought ratings from 22:00 to 23:00 represents a true effect, a problem made worse by the lack of any 23:00 probes in Study 2. We are nevertheless confident in the pattern of results leading up to 22:00 h, particularly the replicated finding that freely moving thought steadily increased throughout the morning.
Fourth, in order to ensure consistency with the existing literature, 7-point Likert scales were used to measure all three dimensions of thought. While this simplifies the process and allows participants to make quick and intuitive decisions, Likert scales have the disadvantage of being unmoored from common reference points. Put simply, even with verbal anchors, there is no objective meaning of a 4 out of 7 on the scale; as a result, each rating may mean something entirely different to each participant. Once again, we attempted to mitigate this by providing participants with extensive instructions. These instructions even included prompting participants to come up with their own examples of different levels of freely moving thought, ensuring that their ideas were as close as possible both to their cohort’s perceptions and to the experimenters’ expectations.
Fifth, past studies have found evidence that task-unrelated thought may demonstrate fluctuations (Carciofo, Du, Song, & Zhang, 2014 ; Giambra et al., 1989 ) similar to those we observed for freely moving thought or stimulus-independent thought. We did not find similar patterns for task-unrelated thought in the present study, but this may be due to significant methodological differences among the studies. Giambra et al. ( 1989 ) conducted a sleep-deprivation study intended more to explore natural circadian rhythms of mind-wandering in isolation than fluctuations in real-world environments. Because they used a lab-based task, their participants were judged on whether they were paying attention to a particular narrow assignment they were given. On the other hand, Carciofo et al. ( 2014 ) had participants estimate when they were most likely to mind-wander instead of probing them throughout the day; they used a multi-item scale that is related to but different than task-unrelated thought to judge mind-wandering. By contrast, the current study presumably assessed participants’ attention to a variety of tasks performed in their everyday life—and did so while they were engaged in those tasks. Taken together, it is difficult to know whether the results of this study represent a true conflict with the literature or simply a difference between experience-sampling studies and lab-based alternatives.
Finally, it is also possible that the unanswered probes differed systematically from the answered probes. Participants were instructed not to answer probes if doing so would put them in danger (e.g. if they were driving a vehicle). The fact that certain activities were un- or underrepresented may mean that ratings across the three dimensions could be biased in one direction. Of course, given the concern for participant safety, it is impossible to address this concern sufficiently in natural data; instead, experimental paradigms could induce similar settings within controlled environments (e.g. risky driving simulations used by Watson et al., 2016 ) to empirically test whether and how these underrepresented activities affect the dynamics.
Another important future step beyond the current work is to better understand the mechanisms that may influence the levels of freedom of movement in thought across the day. For example, how do current concerns and other conscious or latent goal pursuits influence our thought patterns, especially when affectively charged? Additionally, it may be fruitful to assess thought patterns in clinical populations that display more fixated thoughts (e.g. individuals with ruminative tendencies). Studies such as these may provide some key insights about the relationship between affect and constraints and how those features influence our freedom of movement in thought throughout our daily lives.
Post-hoc power analyses
The lack of readily available standardized effect size estimates for mixed-effects polynomial models prevented us from determining our desired sample size based on a calculation of required statistical power. We nevertheless conducted post-hoc observed power analyses in order to inform future research about what types of sample sizes are appropriate for such effects. As observed power analyses are only conceptually meaningful when applied to tests of true effects, we conducted them only on significant parameters in order to estimate each study’s ability to detect the fixed effect in question. Although there is not consensus for how to calculate power exactly for multiple-predictor random-effects models (see Brysbaert & Stevens, 2018 ), we adopted a Monte Carlo simulation approach using the simR package in R (Green & MacLeod, 2015 ). It should be noted that due to their reliance on simulations, these numbers are not exact; however, setting a seed state before executing the analyses ensures that each analysis is replicable. Our shared code includes a fixed seed to allow readers to completely reproduce our analyses.
Table 7 lists the two significant parameters for Study 1 and the five significant parameters for Study 2, along with their corresponding observed power estimates. The sample size of 108 participants (after exclusions) in Study 1 yielded sub-optimal observed power for the quadratic parameter: 60.5% power means that if this study were replicated 100 times, over one-third of the replications would not have found the quadratic parameter to be significant. Although power for the cubic parameter was much higher (82%), future work should ideally set the sample size to ensure sufficient power for the weakest effect of interest. The sample size of 165 participants (after exclusions) used in Study 2 appears to be a little more appropriate; the power to detect the same quadratic parameter in the daily pattern of change for freely moving thought was bolstered to 67%; all other effects achieved a minimum of 80% power.
In sum, although observed power was lower for Study 1 (which solely focused on freely moving thought), we are encouraged that the patterns for freely moving thought were consistent across both datasets, especially given that observed power was much higher for Study 2. Moreover, based on these analyses, similar research on this topic may want to collect > 165 participants when aiming for > 80% power to detect the smallest effect.
Here, we find the first evidence that mind-wandering is not stable across the day – an important implication for both educational and occupational policy, as well as psychological research. Taken together, our findings emphasize the need to consider distinct dimensions of thought related to mind-wandering independently, a concept that has recently gained support in this research area (Mills, Raffaelli, et al., 2017 ; Seli, Risko, et al., 2016 ). A better understanding of the diurnal patterns of freely moving thought, task-unrelated thought, and stimulus-independent thought will contribute to a richer understanding of how our mental states dynamically shift over the course of our daily lives, perhaps even allowing us to optimize the structure of our days.
Aston-Jones, G., & Cohen, J. D. (2005). An integrative theory of locus coeruleus-norepinephrine function: Adaptive gain and optimal performance. Annual Review of Neuroscience , 28 , 403–450. https://doi.org/10.1146/annurev.neuro.28.061604.135709 .
Article PubMed Google Scholar
Aston-Jones, & Waterhouse (2016). Locus coeruleus: From global projection system to adaptive regulation of behavior. Brain Research , 1645 , 75–78. https://doi.org/10.1016/j.brainres.2016.03.001 .
Article PubMed PubMed Central Google Scholar
Baird, B., Smallwood, J., Mrazek, M. D., Kam, J. W. Y., Franklin, M. S., & Schooler, J. W. (2012). Inspired by distraction: Mind wandering facilitates creative incubation. Psychological Science , 23 (10), 1117–1122. https://doi.org/10.1177/0956797612446024 .
Baldwin, C. L., Roberts, D. M., Barragan, D., Lee, J. D., Lerner, N., & Higgins, J. S. (2017). Detecting and quantifying mind wandering during simulated driving. Frontiers in Human Neuroscience , 11 , 406. https://doi.org/10.3389/fnhum.2017.00406 .
Barr, D. J., Levy, R., Scheepers, C., & Tily, H. J. (2013). Random effects structure for confirmatory hypothesis testing: Keep it maximal. Journal of Memory and Language , 68 (3). https://doi.org/10.1016/j.jml.2012.11.001 .
Article Google Scholar
Bates, D., Mächler, M., Bolker, B., & Walker, S. (2015). Fitting linear mixed-effects models using lme4. Journal of Statistical Software , 67 (1). https://doi.org/10.18637/jss.v067.i01 .
Brysbaert, M., & Stevens, M. (2018). Power analysis and effect size in mixed effects models: a tutorial. Journal of Cognition , 1, 9 (1). https://doi.org/10.5334/joc.10 .
Burke, T. M., Scheer, F. A. J. L., Ronda, J. M., Czeisler, C. A., & Wright, K. P. J. (2015). Sleep inertia, sleep homeostatic and circadian influences on higher-order cognitive functions. Journal of Sleep Research , 24 (4), 364–371. https://doi.org/10.1111/jsr.12291 .
Callard, F., Smallwood, J., & Margulies, D. S. (2012). Default positions: how neuroscience’s historical legacy has hampered investigation of the resting mind. Frontiers in Psychology , 3 , 321. https://doi.org/10.3389/fpsyg.2012.00321 .
Carciofo, R., Du, F., Song, N., & Zhang, K. (2014). Chronotype and time-of-day correlates of mind wandering and related phenomena. Biological Rhythm Research , 45 (1), 37–49. https://doi.org/10.1080/09291016.2013.790651 .
Carrier, J., & Monk, T. H. (2000). Circadian rhythms of performance: New trends. Chronobiology International , 17 (6), 719–732. https://doi.org/10.1081/CBI-100102108 .
Christoff, K., Irving, Z. C., Fox, K. C. R., Spreng, R. N., & Andrews-Hanna, J. R. (2016). Mind-wandering as spontaneous thought: a dynamic framework. Nature Reviews Neuroscience , 17 , 718–731. https://doi.org/10.1038/nrn.2016.113 .
Christoff, K., Mills, C., Andrews-Hanna, J. R., Irving, Z. C., Thompson, E., Fox, K. C. R., & Kam, J. W. Y. (2018). Mind-wandering as a scientific concept: cutting through the definitional haze. Trends in Cognitive Sciences , 22 (11), 957–959. https://doi.org/10.1016/j.tics.2018.07.004 .
Dane, E. (2011). Paying attention to mindfulness and its effects on task performance in the workplace. Journal of Management , 37 (4), 997–1018. https://doi.org/10.1177/0149206310367948 .
Dijksterhuis, A., & Meurs, T. (2006). Where creativity resides: The generative power of unconscious thought. Consciousness and Cognition , 15 (1), 135–146. https://doi.org/10.1016/j.concog.2005.04.007 .
Dust, S. B. (2015). Mindfulness, flow, and mind wandering: The role of trait-based mindfulness in state-task alignment. Industrial and Organizational Psychology; Bowling Green , 8 (4), 609–614. https://doi.org/10.1017/iop.2015.87 .
Ellamil, M., Dobson, C., Beeman, M., & Christoff, K. (2012). Evaluative and generative modes of thought during the creative process. NeuroImage , 59 (2), 1783–1794. https://doi.org/10.1016/j.neuroimage.2011.08.008 .
Ellamil, M., Fox, K. C. R., Dixon, M. L., Pritchard, S., Todd, R. M., Thompson, E., & Christoff, K. (2016). Dynamics of neural recruitment surrounding the spontaneous arising of thoughts in experienced mindfulness practitioners. NeuroImage , 136 , 186–196. https://doi.org/10.1016/j.neuroimage.2016.04.034 .
Faber, M., Bixler, R., & D’Mello, S. K. (2018). An automated behavioral measure of mind wandering during computerized reading. Behavior Research Methods , 50 (1), 134–150. https://doi.org/10.3758/s13428-017-0857-y .
Fibiger, W., Singer, G., Miller, A. J., Armstrong, S., & Datar, M. (1984). Cortisol and catecholamines changes as functions of time-of-day and self-reported mood. Neuroscience & Biobehavioral Reviews , 8 (4), 523–530. https://doi.org/10.1016/0149-7634(84)90009-5 .
Fimm, B., & Blankenheim, A. (2016). Effect of sleep deprivation and low arousal on eye movements and spatial attention. Neuropsychologia , 92 (Supplement C), 115–128. https://doi.org/10.1016/j.neuropsychologia.2016.03.021 .
Fimm, B., Brand, T., & Spijkers, W. (2016). Time-of-day variation of visuo-spatial attention. British Journal of Psychology , 107 (2), 299–321. https://doi.org/10.1111/bjop.12143 .
Folkard, S., & Monk, T. H. (1987). The measurement of circadian rhythms in psychological functions. In L. E. Scheving, F. Halberg, & C. Ehret (Eds.), Chronobiotechnology and Chronobiological Engineering , (pp. 189–201). Dordrecht: Springer. https://doi.org/10.1007/978-94-009-3547-1_15 .
Chapter Google Scholar
Fox, K. C. R., Nijeboer, S., Solomonova, E., Domhoff, G. W., & Christoff, K. (2013). Dreaming as mind wandering: evidence from functional neuroimaging and first-person content reports. Frontiers in Human Neuroscience , 7 , 412. https://doi.org/10.3389/fnhum.2013.00412 .
Fox, K. C. R., Spreng, R. N., Ellamil, M., Andrews-Hanna, J. R., & Christoff, K. (2015). The wandering brain: Meta-analysis of functional neuroimaging studies of mind-wandering and related spontaneous thought processes. NeuroImage , 111 , 611–621. https://doi.org/10.1016/j.neuroimage.2015.02.039 .
Franklin, M. S., Mrazek, M. D., Anderson, C. L., Smallwood, J., Kingstone, A., & Schooler, J. W. (2013). The silver lining of a mind in the clouds: Interesting musings are associated with positive mood while mind-wandering. Frontiers in Psychology , 4 , 583. https://doi.org/10.3389/fpsyg.2013.00583 .
Franklin, M. S., Smallwood, J., & Schooler, J. W. (2011). Catching the mind in flight: Using behavioral indices to detect mindless reading in real time. Psychonomic Bulletin & Review , 18 (5), 992–997. https://doi.org/10.3758/s13423-011-0109-6 .
Franklin, M. S., Smallwood, J., Zedelius, C. M., Broadway, J. M., & Schooler, J. W. (2016). Unaware yet reliant on attention: Experience sampling reveals that mind-wandering impedes implicit learning. Psychonomic Bulletin & Review , 23 (1), 223–229. https://doi.org/10.3758/s13423-015-0885-5 .
Fuller-Tyszkiewicz, M., Hartley-Clark, L., Cummins, R. A., Tomyn, A. J., Weinberg, M. K., & Richardson, B. (2017). Using dynamic factor analysis to provide insights into data reliability in experience sampling studies. Psychological Assessment , 29 (9), 1120–1128. https://doi.org/10.1037/pas0000411 .
Gabehart, R. J., & Dongen, H. P. A. V. (2017). Circadian rhythms in sleepiness, alertness, and performance. In Principles and Practice of Sleep Medicine , (6th ed., pp. 388–395.e5). Philadelphia: Elsevier https://www.clinicalkey.com/#!/content/book/3-s2.0-B9780323242882000374 .
Galéra, C., Orriols, L., M’Bailara, K., Laborey, M., Contrand, B., Ribéreau-Gayon, R., … Lagarde, E. (2012). Mind wandering and driving: responsibility case-control study. BMJ , 345 , e8105. https://doi.org/10.1136/bmj.e8105 .
Giambra, L. M., Rosenberg, E. H., Kasper, S., Yee, W., & Sack, D. A. (1989). A circadian rhythm in the frequency of spontaneous task-tnrelated images and thoughts. Imagination, Cognition and Personality , 8 (4), 309–314. https://doi.org/10.2190/1ECY-9JDW-MXNW-KMWC .
Gilzenrat, M. S., Nieuwenhuis, S., Jepma, M., & Cohen, J. D. (2010). Pupil diameter tracks changes in control state predicted by the adaptive gain theory of locus coeruleus function. Cognitive, Affective, & Behavioral Neuroscience , 10 (2), 252–269. https://doi.org/10.3758/CABN.10.2.252 .
Goel, N., Basner, M., Rao, H., & Dinges, D. F. (2013). Circadian rhythms, sleep deprivation, and human performance. Progress in Molecular Biology and Translational Science , 119 , 155–190. https://doi.org/10.1016/B978-0-12-396971-2.00007-5 .
Green, P., & MacLeod, C. J. (2015). SIMR: an R package for power analysis of generalized linear mixed models by simulation. Methods in Ecology and Evolution , 7 (4), 493–498. https://doi.org/10.1111/2041-210X.12504 .
Hansen, Å. M., Garde, A. H., Skovgaard, L. T., & Christensen, J. M. (2001). Seasonal and biological variation of urinary epinephrine, norepinephrine, and cortisol in healthy women. Clinica Chimica Acta , 309 (1), 25–35. https://doi.org/10.1016/S0009-8981(01)00493-4 .
Hartmann, A., Zeeck, A., Herzog, W., Wild, B., de Zwaan, M., Herpertz, S., … Zipfel, S. (2016). The intersession process in psychotherapy for anorexia nervosa: Characteristics and relation to outcome. Journal of Clinical Psychology , 72 (9), 861–879. https://doi.org/10.1002/jclp.22293 .
He, J., Becic, E., Lee, Y.-C., & McCarley, J. S. (2011). Mind wandering behind the wheel: Performance and oculomotor correlates. Human Factors , 53 (1), 13–21. https://doi.org/10.1177/0018720810391530 .
Hurlburt, R. T., Alderson-Day, B., Fernyhough, C., & Kühn, S. (2017). Can inner experience be apprehended in high fidelity? Examining brain activation and experience from multiple perspectives. Frontiers in Psychology , 8 , 628. https://doi.org/10.3389/fpsyg.2017.00043 .
Hyland, P. K., Lee, R. A., & Mills, M. J. (2015). Mindfulness at work: A new approach to improving individual and organizational performance. Industrial and Organizational Psychology; Bowling Green , 8 (4), 576–602. https://doi.org/10.1017/iop.2015.41 .
Irving, Z. C. (2016). Mind-wandering is unguided attention: accounting for the “purposeful” wanderer. Philosophical Studies , 173 (2), 547–571. https://doi.org/10.1007/s11098-015-0506-1 .
Jazaieri, H., Lee, I. A., McGonigal, K., Jinpa, T., Doty, J. R., Gross, J. J., & Goldin, P. R. (2016). A wandering mind is a less caring mind: Daily experience sampling during compassion meditation training. The Journal of Positive Psychology , 11 (1), 37–50. https://doi.org/10.1080/17439760.2015.1025418 .
Jewett, M. E., & Kronauer, R. E. (1999). Interactive mathematical models of subjective alertness and cognitive throughput in humans. Journal of Biological Rhythms , 14 (6), 588–597. https://doi.org/10.1177/074873099129000920 .
Keith, T. Z. (2005). Multiple Regression and Beyond . Boston: Pearson.
Google Scholar
Killingsworth, M. A., & Gilbert, D. T. (2010). A wandering mind is an unhappy mind. Science , 330 (6006), 932–932. https://doi.org/10.1126/science.1192439 .
Krawietz, S. A., Tamplin, A. K., & Radvansky, G. A. (2012). Aging and mind wandering during text comprehension. Psychology and Aging , 27 (4), 951–958. https://doi.org/10.1037/a0028831 .
Kucyi, A., & Davis, K. D. (2014). Dynamic functional connectivity of the default mode network tracks daydreaming. NeuroImage , 100 , 471–480. https://doi.org/10.1016/j.neuroimage.2014.06.044 .
Kuznetsova, A., Brockhoff, P. B., & Christensen, R. H. B. (2017). lmerTest Package: Tests in Linear Mixed Effects Models. Journal of Statistical Software , 82 (13). https://doi.org/10.18637/jss.v082.i13 .
Marchetti, I., Koster, E. H. W., Klinger, E., & Alloy, L. B. (2016). Spontaneous thought and vulnerability to mood disorders: The dark side of the wandering mind. Clinical Psychological Science , 4 (5), 835–857. https://doi.org/10.1177/2167702615622383 .
Mather, M., Clewett, D., Sakaki, M., & Harley, C. W. (2016). Norepinephrine ignites local hotspots of neuronal excitation: How arousal amplifies selectivity in perception and memory. Behavioral and Brain Sciences , 39 , e200. https://doi.org/10.1017/S0140525X15000667 .
McCormick, C., Rosenthal, C. R., Miller, T. D., & Maguire, E. (2017). Mind-wandering in people with hippocampal amnesia. BioRxiv. https://doi.org/10.1101/159681 .
McVay, J. C., & Kane, M. J. (2009). Conducting the train of thought: Working memory capacity, goal neglect, and mind wandering in an executive-control task. Journal of Experimental Psychology: Learning, Memory, and Cognition , 35 (1), 196–204. https://doi.org/10.1037/a0014104 .
McVay, J. C., Kane, M. J., & Kwapil, T. R. (2009). Tracking the train of thought from the laboratory into everyday life: An experience-sampling study of mind wandering across controlled and ecological contexts. Psychonomic Bulletin & Review , 16 (5), 857–863. https://doi.org/10.3758/PBR.16.5.857 .
Mills, C., Bixler, R., Wang, X., & D’Mello, S. K. (2016). Automatic Gaze-Based Detection of Mind Wandering during Film Viewing. (pp. 30–37). Presented at the International Conference on Educational Data Mining, Raleigh, NC: International Educational Data Mining Society.
Mills, C., & D’Mello, S. (2015). Toward a Real-Time (Day) Dreamcatcher: Sensor-Free Detection of Mind Wandering during Online Reading. Presented at the International Conference on Educational Data Mining, Madrid, Spain.
Mills, C., D’Mello, S. K., & Kopp, K. (2015). The influence of consequence value and text difficulty on affect, attention, and learning while reading instructional texts. Learning and Instruction , 40 , 9–20. https://doi.org/10.1016/j.learninstruc.2015.07.003 .
Mills, C., Graesser, A., Risko, E. F., & D’Mello, S. K. (2017). Cognitive coupling during reading. Journal of Experimental Psychology: General , 146 (6), 872–883. https://doi.org/10.1037/xge0000309 .
Mills, C., Herrera-Bennett, A., Faber, M., & Christoff, K. (2018). Why the mind wanders: How spontaneous thought’s default variability may support episodic efficiency and semantic optimization. In The Oxford Handbook of Spontaneous Thought: Mind-wandering, Creativity, Dreaming, and Clinical Conditions , (pp. 11–22). New York: Oxford University Press.
Mills, C., Raffaelli, Q., Irving, Z. C., Stan, D., & Christoff, K. (2018). Is an off-task mind a freely-moving mind? Examining the relationship between different dimensions of thought. Consciousness and Cognition , 58 , 20–33. https://doi.org/10.1016/j.concog.2017.10.003 .
Mirman, D. (2016). Growth curve analysis and visualization using R . Bocan Raton: CRC Press.
Mirman, D., Dixon, J. A., & Magnuson, J. S. (2008). Statistical and computational models of the visual world paradigm: Growth curves and individual differences. Journal of Memory and Language , 59 (4), 475–494. https://doi.org/10.1016/j.jml.2007.11.006 .
Mittner, M., Hawkins, G. E., Boekel, W., & Forstmann, B. U. (2016). A neural model of mind wandering. Trends in Cognitive Sciences , 20 (8), 570–578. https://doi.org/10.1016/j.tics.2016.06.004 .
Mrazek, M. D., Zedelius, C. M., Gross, M. E., Mrazek, A. J., Phillips, D. T., & Schooler, J. W. (2017). Mindfulness in education: Enhancing academic achievement and student well-being by reducing mind-wandering. In J. C. Karremans, E. K. Papies, J. C. Karremans, & E. K. Papies (Eds.), Mindfulness in social psychology , (pp. 139–152). New York: Routledge/Taylor & Francis Group http://ezproxy.library.ubc.ca/login?url=http://search.ebscohost.com/login.aspx?direct=true&db=psyh&AN=2017-21402-010&login.asp&site=ehost-live&scope=site .
Nisbett, R. E., & Wilson, T. D. (1977). Telling more than we can know: Verbal reports on mental processes. Psychological Review , 84 (3), 231–259. https://doi.org/10.1037/0033-295X.84.3.231 .
Nolen-Hoeksema, S., Wisco, B. E., & Lyubomirsky, S. (2008). Rethinking rumination. Perspectives on Psychological Science , 3 (5), 400–424. https://doi.org/10.1111/j.1745-6924.2008.00088.x .
Pachai, A. A., Acai, A., LoGiudice, A. B., & Kim, J. A. (2016). The mind that wanders: Challenges and potential benefits of mind wandering in education. Scholarship of Teaching and Learning in Psychology , 2 (2), 134–146. https://doi.org/10.1037/stl0000060 .
Papageorghiou, A. T., Ohuma, E. O., Altman, D. G., Todros, T., Ismail, L. C., Lambert, A., … Villar, J. (2014). International standards for fetal growth based on serial ultrasound measurements: the Fetal Growth Longitudinal Study of the INTERGROWTH-21st Project. The Lancet , 384 (9946), 869–879. https://doi.org/10.1016/S0140-6736(14)61490-2 .
Phillips, N. E., Mills, C., D’Mello, S., & Risko, E. F. (2016). On the influence of re-reading on mind wandering. Quarterly Journal of Experimental Psychology , 69 (12), 2338–2357. https://doi.org/10.1080/17470218.2015.1107109 .
Qu, W., Ge, Y., Xiong, Y., Carciofo, R., Zhao, W., & Zhang, K. (2015). The relationship between mind wandering and dangerous driving behavior among Chinese drivers. Safety Science , 78 , 41–48. https://doi.org/10.1016/j.ssci.2015.04.016 .
Riley, E., Esterman, M., Fortenbaugh, F. C., & DeGutis, J. (2017). Time-of-day variation in sustained attentional control. Chronobiology International , 34 (7), 993–1001. https://doi.org/10.1080/07420528.2017.1308951 .
Schooler, J. W., Smallwood, J., Christoff, K., Handy, T. C., Reichle, E. D., & Sayette, M. A. (2011). Meta-awareness, perceptual decoupling and the wandering mind. Trends in Cognitive Sciences , 15 (7), 319–326. https://doi.org/10.1016/j.tics.2011.05.006 .
Seli, P., Kane, M. J., Smallwood, J., Schacter, D. L., Maillet, D., Schooler, J. W., & Smilek, D. (2018). Mind-Wandering as a Natural Kind: A Family-Resemblances View. Trends in Cognitive Sciences , 22 (6), 479–490. https://doi.org/10.1016/j.tics.2018.03.010 .
Seli, P., Risko, E. F., Smilek, D., & Schacter, D. L. (2016). Mind-Wandering with and without intention. Trends in Cognitive Sciences , 20 (8), 605–617. https://doi.org/10.1016/j.tics.2016.05.010 .
Seli, P., Wammes, J. D., Risko, E. F., & Smilek, D. (2016). On the relation between motivation and retention in educational contexts: The role of intentional and unintentional mind wandering. Psychonomic Bulletin & Review , 23 (4), 1280–1287. https://doi.org/10.3758/s13423-015-0979-0 .
Shin, T. (2012). The application of various nonlinear models to describe academic growth trajectories: an empirical analysis using four-wave longitudinal achievement data from a large urban school district. Asia Pacific Education Review , 13 (1), 65–76. https://doi.org/10.1007/s12564-011-9168-7 .
Silva, E. J., Wang, W., Ronda, J. M., Wyatt, J. K., & Duffy, J. F. (2010). Circadian and wake-dependent influences on subjective sleepiness, cognitive throughput, and reaction time performance in older and young adults. Sleep , 33 (4), 481.
Singer, J. D., & Willett, J. B. (2003). Applied longitudinal data analysis: modeling change and event occurrence . Oxford: Oxford University Press.
Book Google Scholar
Smallwood, J., Fitzgerald, A., Miles, L. K., & Phillips, L. H. (2009). Shifting moods, wandering minds: Negative moods lead the mind to wander. Emotion , 9 (2), 271–276. https://doi.org/10.1037/a0014855 .
Smallwood, J., McSpadden, M., & Schooler, J. W. (2008). When attention matters: The curious incident of the wandering mind. Memory & Cognition , 36 (6), 1144–1150. https://doi.org/10.3758/MC.36.6.1144 .
Smallwood, J., Nind, L., & O’Connor, R. C. (2009). When is your head at? An exploration of the factors associated with the temporal focus of the wandering mind. Consciousness and Cognition , 18 (1), 118–125. https://doi.org/10.1016/j.concog.2008.11.004 .
Song, X., & Wang, X. (2012). Mind wandering in Chinese daily lives—An experience sampling study. PLoS One , 7 (9), e44423. https://doi.org/10.1371/journal.pone.0044423 .
Sousa, T. L. V., Carriere, J. S. A., & eSmilek, D. (2013). The way we encounter reading material influences how frequently we mind wander. Frontiers in Psychology , 4 , 892. https://doi.org/10.3389/fpsyg.2013.00892 .
Spronken, M., Holland, R. W., Figner, B., & Dijksterhuis, A. (2016). Temporal focus, temporal distance, and mind-wandering valence: Results from an experience sampling and an experimental study. Consciousness and Cognition , 41 , 104–118. https://doi.org/10.1016/j.concog.2016.02.004 .
Stawarczyk, D., Majerus, S., Maj, M., Van der Linden, M., & D’Argembeau, A. (2011). Mind-wandering: Phenomenology and function as assessed with a novel experience sampling method. Acta Psychologica , 136 (3), 370–381. https://doi.org/10.1016/j.actpsy.2011.01.002 .
Unsworth, N., & McMillan, B. D. (2013). Mind wandering and reading comprehension: Examining the roles of working memory capacity, interest, motivation, and topic experience. Journal of Experimental Psychology: Learning, Memory, and Cognition , 39 (3), 832–842. https://doi.org/10.1037/a0029669 .
Valdez, P., Ramírez, C., & García, A. (2014). Circadian rhythms in cognitive processes: Implications for school learning. Mind, Brain, and Education , 8 (4), 161–168. https://doi.org/10.1111/mbe.12056 .
Valdez, P., Ramírez, C., García, A., Talamantes, J., Armijo, P., & Borrani, J. (2005). Circadian rhythms in components of attention. Biological Rhythm Research , 36 (1–2), 57–65. https://doi.org/10.1080/09291010400028633 .
Valdez, P., Reilly, T., & Waterhouse, J. (2008). Rhythms of mental performance. Mind, Brain, and Education , 2 (1), 7–16. https://doi.org/10.1111/j.1751-228X.2008.00023.x .
Watson, J. M., Memmott, M. G., Moffitt, C. C., Coleman, J., Turrill, J., Fernández, Á., & Strayer, D. L. (2016). On Working Memory and a Productivity Illusion in Distracted Driving. Journal of Applied Research in Memory and Cognition , 5 (4), 445–453. https://doi.org/10.1016/j.jarmac.2016.06.008 .
White, H. R., Xie, M., Thompson, W., Loeber, R., & Stouthamer-Loeber, M. (2001). Psychopathology as a predictor of adolescent drug use trajectories. Psychology of Addictive Behaviors , 15 (3), 210–218. https://doi.org/10.1037/0893-164X.15.3.210 .
Wright, K. P., Hull, J. T., & Czeisler, C. A. (2002). Relationship between alertness, performance, and body temperature in humans. American Journal of Physiology - Regulatory, Integrative and Comparative Physiology , 283 (6), R1370–R1377. https://doi.org/10.1152/ajpregu.00205.2002 .
Yanko, M. R., & Spalek, T. M. (2014). Driving with the wandering mind: The effect that mind-wandering has on driving performance. Human Factors , 56 (2), 260–269. https://doi.org/10.1177/0018720813495280 .
Allison, P. D. (2014). Event history and survival analysis: regression for longitudinal event data . SAGE Publications.
Dziak, J. J., Li, R., Tan, X., Shiffman, S., & Shiyko, M. P. (2015). Modeling intensive longitudinal data with mixtures of nonparametric trajectories and time-varying effects., Modeling Intensive Longitudinal Data With Mixtures of Nonparametric Trajectories and Time-Varying Effects. Psychological Methods, Psychological Methods , 20 (4), 444–469. https://doi.org/10.1037/met0000048 .
R Core Team (2017). R: A language and environment for statistical computing . Vienna, Austria: R Foundation for Statistical Computing Retrieved from https://www.R-project.org/ .
Download references
Acknowledgements
This work was supported by NSERC (RGPIN 327317-11) and CIHR (MOP-115197) grants to KC. Any opinions, findings and conclusions, or recommendations expressed in this paper are those of the authors and do not necessarily reflect the views of NSERC or CIHR.
This work was supported by NSERC (RGPIN 327317-11) and CIHR (MOP-115197) grants to KC. Any opinions, findings and conclusions, or recommendations expressed in this paper are those of the authors and do not necessarily reflect the views of NSERC or CIHR. This project was funded in part by a Moore-Sloan Data Science Environments Fellowship to AP, thanks to the Gordon and Betty Moore Foundation through Grant GBMF3834 and the Alfred P. Sloan Foundation through Grant 2013-10-27 to the University of California, Berkeley.
Availability of data and materials
The datasets supporting the conclusions of this article are available in the Open Science Framework repository, https://osf.io/es3gf/ .
Author information
Authors and affiliations.
The University of British Columbia, Vancouver, Canada
Gabriel King Smith, Caitlin Mills & Kalina Christoff
The University of New Hampshire, Durham, USA
Caitlin Mills
University of Connecticut, Storrs, USA
Alexandra Paxton
You can also search for this author in PubMed Google Scholar
Contributions
CM and KC contributed to the design and coordination of the study. GS and CM decided upon the analysis plan. GS and AP performed the analyses. GS, CM, and AP interpreted the results. GS wrote the initial draft of the manuscript with advisement from CM and KC. All authors read, edited, and approved the final manuscript.
Corresponding author
Correspondence to Gabriel King Smith .
Ethics declarations
Authors’ information.
Not applicable.
Ethics approval and consent to participate
Ethical approval for this study was obtained from the University of British Columbia Behavioural Research Ethics Board, approval no. H16–01247.
Consent for publication
Competing interests.
The authors declare that they have no competing interests.
Publisher’s Note
Springer Nature remains neutral with regard to jurisdictional claims in published maps and institutional affiliations.
Additional files
Additional file 1:.
Supplementary materials. Additional file 1. Model Comparison Results. Additional file 2. Complete Dataset Analyses. Additional file 3. Differentiation Analysis Comparing TUT and SIT. (ZIP 92 kb)
Instructions
Notes: Every participant was trained in a small group people. The same experimenter was in the room for the entire question and answer question. The same training video was shown to all participants.
Introducing the study and explaining how the probes work Verbal instructions given via video
So, in this short video, we’re going to tell you everything you need to know. There’s a lot of information, so pay close attention. But we’re going to pause throughout to make sure that you’re grasping it correctly and we’re going to answer any questions. Keep those in mind. And here we go.
The basics is that we’re going to get you to rate your mental activity in a survey that you’re going to be answering a number of times throughout the day. You’re going to get text messages at random times with a web link in them, and you’re going to click that, and you’re going to go to the survey, and it’s really important that you answer right away when you get the text. Actually, you have to answer within 10 seconds. If you’re driving, or if you’re in a situation during which you absolutely can’t answer right then and there, you should just not answer at all. Don’t set it aside and try to answer later because we can see that. That being said, try to interrupt whatever you’re doing. It’s really important that you answer as many of these as possible.
Now, as soon as you hear the beep, you’re going to click on the link and go to the survey. And we’re going to get you to save the number into your phone and customize the text message tone. We’ll give you instructions later on how to do that. But it’s very important that these text messages stand out from any other text messages for reasons we’re about to tell you. You need to know right away, when you hear the tone, that it is one of these important text messages. So, when you’re answering the survey, we want you to finish within 40 seconds. Now, I mean, that may seem like a short amount of time, but once you’ve practiced a couple of times actually it’s going to get very fast. You’ll probably be able to do it within 30 seconds. So, answering quickly and intuitively is important as well. And it’s also very important that you keep your phone around you. Obviously, if you’re going to be answering quickly, and you need to answer a lot of these, keep your phone on, keep the sound up loud, and keep it within arm’s reach at all times as much as possible. And finally, you need to answer at least 80% of these probes.
So, this is a survey about your thought activity. What do we mean by thoughts? By thoughts, we mean anything that is going on in your awareness. That can include internal stuff like memories, emotions, imagining things. It can also mean external things. So, if you’re aware of sensations in your body, or things that you see or hear or smell. You get the picture.
So, when you hear that special tone on your phone, you’re going to take a mental snapshot of what’s going on in your awareness at the time. So, you hear the tone, you pause briefly, your take a snapshot, and then you’re going to open the text, click the link, and you’re going to go and answer all the questions keeping that mental snapshot in mind.
Explanation of free movement
Was your mind moving about freely?
Your thoughts move freely when:
They seem to wander around, flowing from one thing to another
There is no overarching purpose or direction to your thinking. Although there may still be some connection between one thought and the next
Images and memories seem to spontaneously come into your mind
Your attention lands spontaneously on things in your environment
Your mind may spontaneously drift between things in the external environment and internal images so it may go back and forth.
Your thoughts move freely when it feels like your thoughts could land on pretty much anything
Or that your thoughts seem to flow with ease
For example, you know, you’re on the bus going home. You might take the following snapshot of your thoughts: You picture yourself having dinner that evening, then wonder if you’ve been eating too much fast food recently, then notice the faint music playing from another passenger’s headphones and that reminds you of a song you’ve heard at a party the night before.
This is sort of an example of your thoughts are moving freely. You know, they can also move freely around a particular topic such as a current event or, you know, something you’re currently interested in. For example, you think of the bike you just bought, then think yourself biking down a trail next weekend, then picture your friend riding next to you, then remember the first bike you got for your 10th birthday, and so on. So, this is an example where you know, it’s sharing the same topic but it’s still moving freely in that range. Thought can also move freely in the external environment. So, you might be hiking on a forest trail, you notice your mind may shift from the gravel on the path in front of you, to a slug crawling up a stump, to a leaf floating in a puddle. You understand.
So, here we’re going to pause the video, and we’re going to get you to come up with your own example of thoughts when your mind was moving about freely. The example should be different from the ones that were just mentioned.
Freely moving thought ratings as a function of hours since waking
The same participants who had been excluded from the main analysis were excluded from the time-since-waking analysis, and an additional five of the remaining participants had no probes answered on days for which there was wake-up information, leaving a sample size of 104. Only probes answered between 0 and 16 h after waking were kept, as none of the hours after 16 contained responses from 25% or more of the sample.
The pattern of results was similar to the main analysis (Table 8 ), featuring a significant positive cubic parameter and no evidence of linear change over time. The main exception is the negative quadratic term, which trended toward but did not reach statistical significance. Although visual inspection of the data (Fig. 2 b) suggests a highly similar pattern (with the peak occurring 5 hafter waking, dropping sharply until 8 h after waking, and rising afterward), future work should continue to tests this effect.
Weekend-only analyses
Full model results for the reanalysis of Mills, Raffaelli, et al. ( 2017 ) using only data points collected over weekends.
Rights and permissions
Open Access This article is distributed under the terms of the Creative Commons Attribution 4.0 International License ( http://creativecommons.org/licenses/by/4.0/ ), which permits unrestricted use, distribution, and reproduction in any medium, provided you give appropriate credit to the original author(s) and the source, provide a link to the Creative Commons license, and indicate if changes were made.
Reprints and permissions
About this article
Cite this article.
Smith, G.K., Mills, C., Paxton, A. et al. Mind-wandering rates fluctuate across the day: evidence from an experience-sampling study. Cogn. Research 3 , 54 (2018). https://doi.org/10.1186/s41235-018-0141-4
Download citation
Received : 18 January 2018
Accepted : 07 November 2018
Published : 29 December 2018
DOI : https://doi.org/10.1186/s41235-018-0141-4
Share this article
Anyone you share the following link with will be able to read this content:
Sorry, a shareable link is not currently available for this article.
Provided by the Springer Nature SharedIt content-sharing initiative
- Mind wandering
- Freely moving thought
- Experience sampling
- Daily change
- Circadian rhythms
The power of daydreams: 4 studies on the surprising science of mind-wandering
[ted id=1607 width=560 height=315]
What makes us happy? It’s one of the most complicated puzzles of human existence — and one that, so far, 87 speakers have explored in TEDTalks .
In today’s talk , Matt Killingsworth (who studied under Dan Gilbert at Harvard) shares a novel approach to the study of happiness — an app, Track Your Happiness , which allows people to chart their feelings on a moment-by-moment basis. As they go about their day, app users get random pings, asking them to share their current activity and note their mood. When Killingsworth gave this talk at TEDxCambridge in 2011, the app had collected data from more than 15,000 people in 80 countries, representing a wide range of ages, education levels and occupations. In this talk, Killingsworth reveals a very surprising finding: that mind-wandering appears to factor heavily into this happiness equation.
“As human beings, we have this unique ability to have our minds stray,” says Killingsworth on the TEDx stage . “This ability to focus our attention on something other than the present is amazing — it allows us to learn and plan and reason.”
While most people think of mind-wandering as a lifting escape from daily drudgery, the Track Your Happiness data shows that this may not the case. In fact, mind-wandering appears to be correlated with unhappiness . When people were mind-wandering, they reported feeling happy only 56% of the time. Meanwhile, when they were focused on the present moment, they reported feeling happy 66% of the time. This effect was true regardless of the activity the person was doing — be it waiting in a traffic jam or eating a delicious dinner. (Read Killingsworth’s study, published in the journal Science in 2010 , to see a breakdown of mind-wandering rates by activity.)
According to Killingsworth’s data, people mind-wander most when in the shower and least when they are having sex. But, still, mind-wandering is a constant. Overall, people mind-wander 47% of the time. Perhaps not such a good thing if it relates to unhappiness,
To hear more about mind-wandering — and about the importance of studying happiness in general — watch Killingsworth’s talk . And after the jump, read several more fascinating studies on the psychology of mind-wandering — some of which will make you feel better about your daydreaming.
A relationship to working memory Mind-wandering might make us feel less content, but it could also have a functional purpose. A recent study published in the journal Psychological Science suggests that mind-wandering might be a sign of a high capacity working memory — in other words, the ability to think about multiple things at once. Researchers asked study participants to press a button and, as they went, checked in to see if their minds were wandering. After the task was complete, researchers gave participants a measure of their working memory. Interestingly, those who were found to be frequent mind-wanderers during the first task showed a greater capacity of working memory. Researcher Jonathan Smallwood of the Max Planck Institute for Human Cognitive and Brain Science explains, “Our results suggest that the sorts of planning that people do quite often in daily life — when they’re on the bus, when they’re cycling to work, when they’re in the shower — are probably supported by working memory. Their brains are trying to allocate resources to the most pressing problems.”
A key to memory formation Mind-wandering might also play a vital function in helping us form memories. New York University neuroscientist Arielle Tambini looked at memory consolidation in this study published in the journal Neuron in 2010 . Participants in the study were asked to look at pairs of images and, in between, were instructed to take a break to think about anything they wanted. Using fMRI, the researchers looked at the activity in the hippocampus cortical regions while they did both. The study showed that these two areas of the brain appear to work together — and that the greater the levels of brain activity in both areas, the stronger the subjects’ recall of the image pairing was. Explains Lila Davichi, who oversaw the study , “Your brain is working for you when you’re resting, so rest is important for memory and cognitive function. This is something we don’t appreciate much, especially when today’s information technologies keep us working round-the-clock … Taking a coffee break after class can actually help you retain that information you just learned.”
A creative boost As the cliché goes, the best ideas usually come when you are least expecting them. A recent study published in the journal Psychological Science gives a clue as to why. A research team led by Benjamin Baird and Jonathan Schooler of the University of California at Santa Barbara asked participants to take “unusual uses” tests — brainstorming alternate ways to use an everyday object like a toothpick for two minutes. Study participants did two of these sessions, and then were given a 12-minute break, during which they were asked to rest, perform a demanding memory exercise or do a reaction time activity designed to maximize their mind-wandering. After the break, they did four more unusual uses tests — two of them repeats. While all of the groups performed comparably on the two new unusual uses lists, the group that had performed the mind-wandering tasks performed 41% better then the other groups on the unusual uses lists they were repeating. “The implication is that mind-wandering was only helpful for problems that were already being mentally chewed on. It didn’t seem to lead to a general increase in creative problem-solving ability,” says Baird .
- Subscribe to TED Blog by email
Comments (69)
Pingback: Tracking Mind-Wandering – Resonance
Pingback: 4 studies on the surprising science of mind-wandering | TED Blog | Miquel Blanch
Pingback: 4 Tips For Better Daydreaming / R.S. Mollison-Read
Pingback: TED Talks Pinned: Speaking of HapPINness | The English Learners' Blog
Pingback: momentary happiness please… Ted Talk | Hunt FOR Truth on wordpress
Pingback: On the science of Mind-wandering | Learning Sciences of Change
Pingback: Poetics Serendipity | Margo Roby: Wordgathering
Pingback: Matt Killingsworthy:想要快樂嗎?專注於當下吧! | TEDxTaipei
Pingback: Issue 27 – Happy Thanksgiving! — TLN
Pingback: Abundance. | Cindy Mantai Writing & Editing
ORIGINAL RESEARCH article
Young and restless: validation of the mind-wandering questionnaire (mwq) reveals disruptive impact of mind-wandering for youth.

- Department of Psychological and Brain Sciences, University of California Santa Barbara, Santa Barbara, CA, USA
Mind-wandering is the focus of extensive investigation, yet until recently there has been no validated scale to directly measure trait levels of task-unrelated thought. Scales commonly used to assess mind-wandering lack face validity, measuring related constructs such as daydreaming or behavioral errors. Here we report four studies validating a Mind-Wandering Questionnaire (MWQ) across college, high school, and middle school samples. The 5-item scale showed high internal consistency, as well as convergent validity with existing measures of mind-wandering and related constructs. Trait levels of mind-wandering, as measured by the MWQ, were correlated with task-unrelated thought measured by thought sampling during a test of reading comprehension. In both middle school and high school samples, mind-wandering during testing was associated with worse reading comprehension. By contrast, elevated trait levels of mind-wandering predicted worse mood, less life-satisfaction, greater stress, and lower self-esteem. By extending the use of thought sampling to measure mind-wandering among adolescents, our findings also validate the use of this methodology with younger populations. Both the MWQ and thought sampling indicate that mind-wandering is a pervasive—and problematic—influence on the performance and well-being of adolescents.
Introduction
An extensive empirical literature has demonstrated that adults mind-wander as much as 30–50% of their waking lives, often at considerable expense to ongoing performance and quality of life ( Kane et al., 2007 ; Killingsworth and Gilbert, 2010 ; Schooler et al., 2011 ). Mind-wandering is characteristically described as the interruption of task-focus by task-unrelated thought (TUT; Smallwood and Schooler, 2006 ). Consistent with this usage, the most common and direct assessment of mind-wandering involves periodically interrupting individuals during a task and asking them to report the extent to which their attention was either on-task or on task-unrelated concerns. Yet despite the growing recognition that variation in mind-wandering represents an important individual difference measure ( Kane et al., 2007 ; McVay and Kane, 2012 ; Mrazek et al., 2012a , b ), research has until recently proceeded without a validated scale to directly measure trait levels of task-unrelated thought. Here we report the creation and validation of a Mind-Wandering Questionnaire (MWQ), along with novel insights made possible by the use of this scale regarding the impact of mind-wandering on the academic performance and well-being of adolescents.
Scales commonly used to assess mind-wandering lack face validity, measuring related but distinct constructs like daydreaming or behavioral errors. Perhaps the most widely used scale to assess trait levels of mind-wandering is the Daydream Frequency Scale (DDFS), also known as the daydream subscale of the Imaginal Processes Inventory ( Giambra, 1995 ). This scale was central to one of the initial investigations linking mind-wandering to the default network (a collection of brain regions that show greater activation at rest; Mason et al., 2007 ). Mason and colleagues argued that recruitment of the default network during practiced tasks reflected mind-wandering in part because this activation was correlated with individuals' propensity to mind-wander as measured by the DDFS. More recently, the DDFS has been used as a measure of mind-wandering in investigations linking task-unrelated thought with the opposing construct of mindfulness ( Mrazek et al., 2012b ; Stawarczyk et al., 2012 ) and with creativity ( Baird et al., 2012 ).
Despite the widespread tendency to use the DDFS as a measure of mind-wandering, a review of the scale's items indicates that rather than focusing on task unrelated thought, it predominantly focuses on stimulus-independent thought (e.g., When I have time on my hands I daydream … ; On a long bus, train, or airplane ride, I daydream … ; When I am not paying close attention to some job, book or TV, I tend to be daydreaming.). The DDFS therefore lacks face validity as a measure of mind-wandering, even though scores on the DDFS correlate with other measures of task-unrelated thought ( Mrazek et al., 2012a , b ). The key difference between the constructs of daydreaming and mind-wandering relates to the relevance of a primary task from which attention is diverted. Daydreaming refers to stimulus-independent thought that does not occur during a primary task, while mind-wandering involves a redirection of attention away from a task. Although setting precise criteria for what exactly constitutes a primary task is challenging (e.g., could taking a walk be classified as a task?), the DDFS is clearly not designed to measure stimulus-independent thought that occurs during a competing task. This distinction may hold considerable importance for ongoing research given that some individuals might daydream extensively but still have the ability to focus their attention on a primary task as needed.
Although less commonly treated as a direct measure of mind-wandering, another questionnaire used to measure lapses of attention is the Attention Related Cognitive Errors Scale (ARCES; Cheyne et al., 2006 ). This scale assesses the frequency of everyday mistakes that are likely to be caused by failures of attention. The ARCES has been correlated with several performance markers of inattention in the Sustained Attention to Response Task (SART), and has been interpreted as a measure of the consequences of mind-wandering ( Cheyne et al., 2006 , 2009 ). Although the ARCES provides a useful measurement of attention-related failures, it cannot provide a direct assessment of trait levels of mind-wandering because not all task-unrelated thought necessarily impairs performance and not all mistakes result from lapses of attention.
A third questionnaire that has been used to assess mind-wandering is the Mindful Attention and Awareness Scale (MAAS; Brown and Ryan, 2003 ). This scale measures the presence or absence of attention to and awareness of what is occurring in the present. The MAAS is used as a measure of mind-wandering based on the (often implicit) logic that mindfulness and mind-wandering are opposing constructs. Indeed, research indicates that those who report high levels of mindfulness on the MAAS tend to mind-wander less during laboratory tasks ( Mrazek et al., 2012b ) and that mindfulness training can reduce mind-wandering ( Mrazek et al., 2013 ).
Although the MAAS has some ability to capture variability in dispositional mind-wandering, it also has limited face validity for at least two reasons. First, the MAAS measures inattention in contexts without a clearly delineated primary task (e.g., “I find myself preoccupied with the future or the past.”) This sort of preoccupation is not mind-wandering if it occurs in the absence of a primary task. Second, the MAAS emphasizes not only attention, but also awareness. The majority of scale items target one's tendency for reflective self-awareness or meta-awareness (e.g., “I do jobs or tasks automatically, without being aware of what I'm doing”). It is possible to complete a task without meta-awareness yet with unwavering attention.
Another related challenge associated with using the MAAS as a measure of mind-wandering is that the word mindfulness is ultimately varied in its meaning. Some meditative traditions have defined mindfulness as sustained non-distraction ( Wallace and Shapiro, 2006 ; Dreyfus, 2011 ), whereas multifactor conceptualizations of mindfulness emphasize additional qualities as well, such as an orientation toward one's experiences characterized by curiosity, openness, and acceptance ( Bishop et al., 2004 ; Baer et al., 2006 ). In fact, some specifically argue that mindfulness cannot be defined as simply the absence of mind-wandering ( Grossman and Van Dam, 2011 ). Given this disagreement, the MAAS has limitations in its use as a trait measure of mind-wandering.
In the absence of a scale specifically designed to measure mind-wandering, researchers have relied on alternative measures of related constructs like daydreaming, attention-related errors, and mindfulness. While we developed and validated a new scale to provide researchers with a direct tool for rapidly assessing trait levels of the frequency of mind-wandering, Carriere et al. (2013) developed and conducted an initial validation of two additional mind-wandering scales: the Mind Wandering-Deliberate (MW-D) scale and the Mind Wandering-Spontaneous (MW-S) scale. These scales revealed that fidgeting is associated with spontaneous but not deliberate mind-wandering ( Carriere et al., 2013 ). Used separately, neither the MW-D nor the MW-S reflects the overall frequency of mind-wandering. Whether their combination can be used for this purpose is still undetermined.
The MWQ presented here is intended to measure the frequency of mind-wandering, irrespective of whether mind-wandering is deliberate or spontaneous. Items for the MWQ were written in simple language, with the intention that this scale could also be used with adolescents. Despite the wide recognition that mind-wandering is a pervasive influence among adults, the role of mind-wandering among adolescents has received little attention. This stands in contrast to strong evidence that attention problems more generally among youth are both widespread and significant. A recent meta-analysis reviewing research linking attention problems and academic achievement found that attention problems—defined according to clinical and sub-clinical levels of symptoms associated with Attention Deficit Hyperactivity Disorder (ADHD)—were associated with a variety of academic challenges ( Polderman et al., 2010 ). Attention problems predicted grade repetition, the need for special education, and lower scores on achievement tests.
Given that attention problems are a major challenge for young students, existing and new methodologies used to study mind-wandering might facilitate a more complete understanding of inattention among youth. Here we examine the usefulness of both the MWQ and existing measures of mind-wandering in adolescent populations. This allows us to extend the use of these methods to younger populations while also investigating whether mind-wandering—which is known to be extensive and disruptive among adults—is an equally pervasive and problematic influence among middle school and high school students.
Experimental Overview
Study 1 describes the development of the MWQ and the determination of its reliability and conceptual homogeneity in a large sample of college undergraduates. Study 2 used a separate undergraduate sample to explore the convergent validity of the MWQ with thought sampling of mind-wandering during a test of working memory capacity. In Studies 3–4, we extended the validation of the MWQ to younger populations by measuring middle school and high school students' mind-wandering using the MWQ and also with thought sampling during a test of reading comprehension. We then examined how mind-wandering was associated with reading ability, stress, self-esteem, mood, and life satisfaction. Studies 3–4 therefore provided the opportunity to (1) extend the validation of the MWQ to younger populations, (2) establish the usefulness of thought sampling as a measure of mind-wandering with younger populations, (3) investigate whether mind-wandering during testing is associated with impaired performance among adolescents, (4) examine the link between mind-wandering and several markers of quality of life, and (5) explore the hypothesis that there is a marked difference in the implications of mind-wandering that occurs during testing vs. that which occurs during daily life.
Materials and Methods
Three researchers independently reviewed the DDFS, ARCES, and MAAS to select items that corresponded to the following definition of mind-wandering: the interruption of task-focus by task-unrelated thought. Each researcher also contributed three novel items, and the resulting pool of items was then reviewed independently by all researchers. Five items were unanimously regarded as acceptable assessments of mind-wandering (1: I have difficulty maintaining focus on simple or repetitive work; 2: While reading, I find I haven't been thinking about the text and must therefore read it again; 3: I do things without paying full attention; 4: I find myself listening with one ear, thinking about something else at the same time; 5: I mind-wander during lectures or presentations) 1 . Following the MAAS, response options were designated along a 6-point Likert scale (1-almost never, 2-very infrequently; 3-somewhat infrequently; 4-somewhat frequently; 5-very frequently; 6-almost always).
Six hundred and sixty three undergraduates (262 male, 401 female) from the University of California Santa Barbara (UCSB) participated in exchange for course credit (mean age = 19.48 years, SD = 2.29, range = 18–58). As part of a larger 1-h online survey, participants completed the 5-item MWQ. For this and all following studies, participants provided written informed consent and all procedures were approved by the Human Subjects Committee of the UCSB.
Results and Discussion
Descriptive statistics for each of the five items are included in Table 1 . A reliability analysis revealed a Cronbach's alpha of 0.850. This indicates good internal consistency among the items, particularly for a 5-item scale (reliability estimates tend to become larger as the number of items increases).
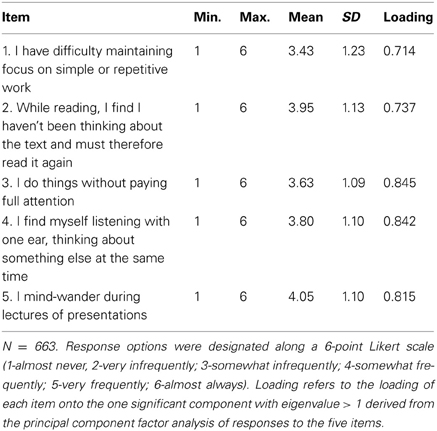
Table 1. Descriptive statistics for each Mind-Wandering Scale item .
To further test the internal consistency, we next examined the inter-item correlations. Consistently moderate inter-item correlations are preferred, as they indicate good internal reliability without highly redundant items ( Clark and Watson, 1995 ). According to these criteria, the MWQ had good inter-item correlations (Mean: 0.540, Min: 0.439, Max: 0.684).
Given that the scale showed high internal reliability, we next examined the scale's homogeneity—the degree to which the items assess a single underlying construct—using factor analysis. Principal component factor analysis of responses to the five items revealed one significant component with eigenvalue > 1 (eigenvalue: 3.58), accounting for 63.16% of total variance. Each of the five scale items had a high loading on this component (0.714, 0.737, 0.845, 0.842, 0.815). No other significant components were extracted. These results indicate that the MWQ showed good homogeneity and that the set of items assessed a single underlying construct.
Having established the internal consistency and homogeneity of the MWQ in Study 1, we next examined the convergent validity of the scale among a sample of college undergraduates, specifically in relation to thought sampling of mind-wandering during a task. This widely used measure of mind-wandering involves periodically interrupting individuals during a task and asking them to report the extent to which their attention was on the task or task-unrelated concerns. There is a broad literature validating self-reported mind-wandering obtained through thought-sampling by using behavioral ( Smallwood et al., 2004 ), event-related potential (ERP; Smallwood et al., 2008 ), and fMRI methodologies ( Christoff et al., 2009 ). Such studies suggest that individuals are able to accurately report whether they have been mind-wandering as revealed by distinct patterns of task performance and neural activation in association with self-reported mind-wandering. Having previously established the relationship between mind-wandering measured via thought sampling and performance on the operation span task ( Mrazek et al., 2012a , b ) we selected this measure of working memory capacity for the initial validation of the MWQ.
Seventy-seven undergraduate students (26 male, 51 female) from the UCSB participated in exchange for course credit (mean age = 18.84, SD = 0.93, range = 18–22). Participants completed an automated version of the operation span task (OSPAN; Unsworth et al., 2005 ) with embedded thought sampling, followed by the MWQ. The OSPAN is a complex span task that presents to-be-remembered letters in alternation with an unrelated processing task (verifying the accuracy of an equation). In each trial, the to-be-remembered items were sets of 3–7 letters chosen from a pool of 12 with each individual letter presented for 250 ms. At the end of each trial, participants selected the presented items in the serial order in which they appeared. However, to allow for thought sampling during the OSPAN, five trials ended with a thought sampling probe rather than an opportunity to select the items that had appeared. Thus, there were a total of 15 complete trials and 5 trials that ended with thought probes. The thought probe asked participants to indicate to what extent their attention was either on-task or on task-unrelated concerns using a 1–5 Likert scale (1:completely on-task; 2:mostly on-task; 3:both on the task and unrelated concerns; 4:mostly on unrelated concerns; 5:completely on unrelated concerns).
Following standard procedure for this task ( Conway et al., 2005 ), one participant with an accuracy rate of less than 85% on the unrelated processing task (including errors caused by failing to respond within a response deadline based on latencies (M + 2.5 SDs) for 15 practice items) was excluded from the analysis. Span scores were calculated as the total number of items recalled in correct serial order across all trials ( Conway et al., 2005 ).
At unpredictable intervals during the OSPAN, five trial response screens were replaced with thought sampling probes which asked participants to indicate to what extent their attention was either on-task or on task-unrelated concerns using a 1–5 Likert scale (1:completely on-task; 2:mostly on-task; 3:both on the task and unrelated concerns; 4:mostly on unrelated concerns; 5:completely on unrelated concerns). After answering the thought probe, participants were instructed that they would begin a new trial. A mind-wandering score was computed by calculating the mean of the five thought probe responses.
Although trait levels of mind-wandering are conceptually distinct from levels of mind-wandering during challenging laboratory tasks, a positive correlation between these measures would establish the convergent validity of the MWQ with the most commonly used methodology for assessing task-unrelated thought. Indeed, MWQ scores predicted probe-caught mind-wandering during the OSPAN ( r = 0.229, p = 0.047). MWQ scores also predicted OSPAN performance ( r = −0.283, p = 0.013). This is consistent with the well-established negative correlation between mind-wandering and working memory capacity ( Kane et al., 2007 ; McVay and Kane, 2009 ; Mrazek et al., 2012a , b ) which was also true of probe-caught mind-wandering and OSPAN scores in this sample ( r = −0.271, p = 0.018).
Study 3 sought to establish the convergent validity of the MWQ in adolescent populations while also addressing a number of unanswered questions about mind-wandering among youth. Is thought sampling an effective measure of mind-wandering with younger populations? Although this measure is widely used among adults, adolescents might not be able to monitor or report their conscious experience as effectively. Is mind-wandering during testing associated with impaired performance among adolescents? This relationship is well-established among adults, but has not yet been demonstrated among adolescents. Is mind-wandering among youth associated with mood or quality of life ? Although mind-wandering predicts lower mood in adults ( Smallwood et al., 2005 , 2009 ; Killingsworth and Gilbert, 2010 ), its impact on adolescents' levels of mood, stress, self-esteem, and life satisfaction are unknown. Finally, are there different implications for trait levels of mind-wandering vs. task-unrelated thought that occurs during testing? We examined each of these questions in a large sample of high school students by integrating thought sampling into a test of reading comprehension and administering a set of questionnaires to assess mind-wandering and several facets of well-being.
One hundred and six female high school students from Dos Pueblos High School in Goleta, CA participated in exchange for gift cards. As the pre-testing for a larger training study, participants completed a 20-min reading comprehension test with thought sampling and a battery of scales described below. Test passages and questions were selected from standardized reading comprehension testing materials used in Alaska and Florida. Two versions matched for length, number of questions, and difficulty were created for each grade.
At unpredictable intervals during the reading test, participants were interrupted 8 times and asked to answer thought sampling probes identical to those in Study 2. After answering each thought probe, participants were instructed to resume their reading test. A mind-wandering score was computed by calculating the mean of the eight thought probe responses.
Following the reading test, participants completed the following scales: MWQ; MAAS; Satisfaction with Life Scale (SWLS); Perceived Stress Scale (PSS); Positive and Negative Affect Schedule (PANAS); and the Rosenberg Self-Esteem Scale (RSES). A composite mood score was computed for the PANAS by subtracting the negative affect sub-scale from the positive affect sub-scale.
Correlations among all measures are reported in Table 2 . Mind-wandering during testing as assessed by thought sampling was negatively correlated with reading comprehension. This result indicates that high school students are able to monitor and report the focus of their attention with sufficient accuracy to predict their test performance. Trait levels of mind-wandering as measured by the MWQ were correlated with task-unrelated thought during the test of reading comprehension. Whereas mind-wandering during testing was significantly associated with worse reading comprehension, high scores on the MWQ significantly predicted worse mood, greater stress, and lower self-esteem.
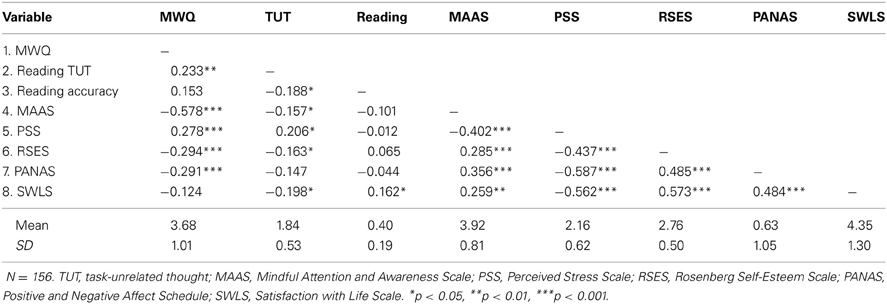
Table 2. Correlations among MWQ, performance measures, and scales for high school students .
Having administered both the MWQ and the MAAS, we next examined which of these scales was a better predictor of mind-wandering during testing. We conducted a simultaneous regression analysis predicting mind-wandering during testing from MWQ and MAAS scores. We examined the collinearity statistics of the predictor variables and found that the tolerance of both the MWQ and MAAS were 0.67 and the VIF were 1.50, indicating that there was no evidence of a multicollinearity problem. The overall regression model was significant, F (1, 153) = 4.467, p = 0.013. Only the MWQ was a significant predictor of mind-wandering during testing. An inspection of the standardized partial regression coefficients (β) and semi-partial correlations ( sr 2 ) revealed that the MWQ explained a significant amount of unique variance in mind-wandering (β = 0.214, p = 0.028, sr 2 = 0.177), whereas the MAAS did not (β = 0.033, p = 0.729, sr 2 = 0.028).
Given that the MWQ measured trait levels of mind-wandering among high school students in a way that led to a predicted and informative pattern of relationships with other measures, we sought to extend the use of this scale to a middle school sample.
Seventy-eight middle school students (35 male, 43 female) from Santa Barbara Middle School and Crane Country Day School in Santa Barbara, CA participated in exchange for gift cards (68 students in Grade 7, 10 students in Grade 6). As the pre-testing for a larger training study, participants completed the same experimental procedure as in Study 3. Test passages and questions were selected from standardized state reading comprehension testing materials used in California. Two versions matched for length, number of questions, and difficulty were created for each grade. Participants completed the five questionnaires in a counterbalanced order.
The pattern of correlations among measures in this sample of middle school students (Table 3 ) closely resembled results from Study 3. Mind-wandering during testing was associated with impaired reading comprehension. High scores on the MWQ predicted mind-wandering during testing, but not reading comprehension. Finally, high levels of mind-wandering on the MWQ predicted worse mood, greater stress, and lower self-esteem. These results suggest that even middle school students of 11–13 years of age can report the focus of their attention with reasonable accuracy. The similarity in findings across high school and middle school samples indicates that mind-wandering may hold similar implications across these age groups.
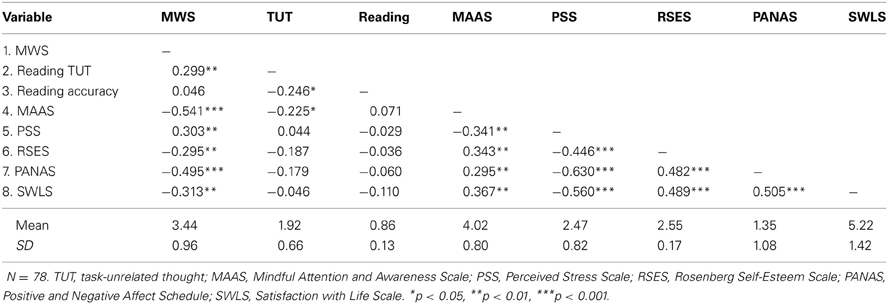
Table 3. Correlations among MWS, performance measures, and scales for middle school students .
General Discussion
The MWQ is a face-valid tool for rapidly assessing trait levels of mind-wandering. The scale showed high internal consistency and good homogeneity. It predicted mind-wandering assessed via thought sampling in college, high school, and middle school populations. The current research therefore suggests that the MWQ is a suitable tool for researchers to use when they are specifically interested in mind-wandering, as opposed to related constructs assessed by the DDFS, ARCES, or MAAS.
Further validation of the MWQ would be useful, particularly with respect to additional populations of interest and greater specification of the task contexts in which performance can be predicted by trait levels of mind-wandering. The present research focused on the use of this scale with undergraduate, high school, and middle school students. Further research across cultures or with more heterogeneous adult samples or special populations like youth or adults with ADHD could determine additional domains in which the MWQ could be used. Another remaining question is whether trait levels of mind-wandering assessed via the MWQ will be useful in predicting performance on laboratory tasks. High scores on the MWQ were associated with worse working memory performance among undergraduates, but the MWQ did not predict worse reading comprehension among high school or middle school students. In fact, there was a marginally significant association between more mind-wandering on the MWQ and better reading comprehension among high school students (though not in the middle school sample). It is unclear whether this discrepancy is due to the age of participants, the type of task, or some additional variable. Regardless, the present findings suggest that the MWQ cannot be used in place of thought sampling for researchers specifically interested in mind-wandering that occurs during laboratory tests of cognitive performance.
Children develop more explicit and effective metacognition as they age ( Kuhn, 2000 ), yet the current findings suggest that children as young as 11 years of age (in 6th grade) are able to report the focus of their attention in a way that meaningfully correlates with their reading comprehension and well-being. Given the usefulness of thought sampling in characterizing the causes and consequences of mind-wandering among adults ( Schooler et al., 2011 ), continued investigation of inattention among youth may be facilitated by the present demonstration that thought sampling can also be an appropriate method with adolescents. Both existing research and the present findings suggest that attention problems among youth are both widespread and significant ( Polderman et al., 2010 ). More comprehensive analysis of the prevalence and impact of mind-wandering in schools are clearly warranted. For instance, mind-wandering during lectures is associated with impaired learning among college students ( Risko et al., 2012 ), suggesting that the use of thought sampling in other high school and middle school contexts might reveal that the link between mind-wandering and impaired reading comprehension has parallels in other educational outcomes.
High levels of mind-wandering as assessed by the MWQ were associated with worse mood, greater stress, and lower self-esteem among adolescents. These results indicate that efforts to enhance the well-being of youth might benefit from greater recognition and understanding of the impact of mind-wandering on social and emotional dimensions of students' lives. The correlational findings in the present research do not indicate that mind-wandering leads to lower quality of life, but research does suggest that mind-wandering may cause worse mood in adults ( Smallwood et al., 2009 ; Killingsworth and Gilbert, 2010 ). This suggests that additional research examining the social and emotional impact of mind-wandering among adolescents would be worthwhile. Even if mind-wandering is not directly causing stress, low mood, and poor self-esteem, it is still possible that interventions targeting any one of these outcomes might affect the others. Given that levels of mind-wandering can be influenced by interventions among adults ( Mrazek et al., 2013 ), similar interventions for reducing mind-wandering early in life might help improve both academic performance and quality of life. Nevertheless, future research should also keep the potential benefits of mind-wandering in view. It may be that individuals are best off not eliminating mind-wandering altogether, but instead mind-wandering at the right times (e.g., when primary task demands are relatively modest) and on the right topics (e.g., on productive issues that can foster future planning or creativity).
Author's Note
Michael D. Mrazek, Michael S. Franklin, James M. Broadway, Dawa T. Phillips, and Jonathan W. Schooler are supported through United States Department of Education grant R305A110277 awarded to Jonathan W. Schooler. The content of this article does not necessarily reflect the position or policy of the US Government, and no official endorsement should be inferred.
Conflict of Interest Statement
The authors declare that the research was conducted in the absence of any commercial or financial relationships that could be construed as a potential conflict of interest.
- ^ Items 3 and 4 were adapted from MAAS items “I find myself doing things without paying attention” and “I find myself listening to someone with one ear, doing something else at the same time.”
Baer, R. A., Smith, G. T., Hopkins, J., Krietemeyer, J., and Toney, L. (2006). Using self-report assessment methods to explore facets of mindfulness. Assessment 13, 27–45. doi: 10.1177/1073191105283504
Pubmed Abstract | Pubmed Full Text | CrossRef Full Text
Baird, B., Smallwood, J., Mrazek, M. D., Kam, J. W. Y., Franklin, M. S., and Schooler, J. W. (2012). Inspired by distraction: mind-wandering facilitates creative incubation. Psychol. Sci . 23, 1117–1122. doi: 10.1177/0956797612446024
Bishop, S. R., Lau, M., Shapiro, S., Carlson, L., Anderson, N. D., Carmody, J., et al. (2004). Mindfulness: a proposed operational definition. Clin. Psychol. Sci. Pract . 11, 230–241. doi: 10.1093/clipsy.bph077
CrossRef Full Text
Brown, K. W., and Ryan, R. M. (2003). The benefits of being present: mindfulness and its role in psychological well-being. J. Pers. Soc. Psychol . 84, 822–848. doi: 10.1037/0022-3514.84.4.822
Carriere, J. S. A., Seli, P., and Smilek, D. (2013). Wandering in both mind and body: individual differences in mind wandering and inattention predict fidgeting. Can. J. Exp. Psychol . 67, 19–31. doi: 10.1037/a0031438
Cheyne, J. A., Carriere, J. S. A., and Smilek, D. (2006). Absent-mindedness: lapses of conscious awareness and everyday cognitive failures. Conscious. Cogn . 15, 578–592. doi:10.1016/j.concog.2005.11.009
Cheyne, J. A., Solman, G. J. F., Carriere, J. S. A., and Smilek, D. (2009). Anatomy of an error: a bidirectional state model of task engagement/disengagement and attention-related errors. Cognition 111, 98–113. doi: 10.1016/j.cognition.2008.12.009
Christoff, K., Gordon, A. M., Smallwood, J., Smith, R., and Schooler, J. W. (2009). Experience sampling during fMRI reveals default network and executive system contributions to mind wandering. Proc. Natl. Acad. Sci. U.S.A . 106, 8719–8724. doi: 10.1073/pnas.0900234106
Clark, L., and Watson, D. (1995). Constructing validity: basic issues in objective scale development. Psychol. Assess . 7, 309–319. doi: 10.1037/1040-3590.7.3.309
Conway, A. R. A., Kane, M. J., Bunting, M. F., Hambrick, D. Z., Wilhelm, O., and Engle, R. W. (2005). Working memory span tasks: a methodological review and user's guide. Psychon. Bull. Rev . 12, 769–786. doi: 10.3758/BF03196772
Dreyfus, G. (2011). Is mindfulness present-centred and non-judgmental? a discussion of the cognitive dimensions of mindfulness. Contemp. Buddhism 12, 41–54. doi: 10.1080/14639947.2011.564815
Giambra, L. M. (1995). A laboratory method for investigating influences on switching attention to task-unrelated imagery and thought. Conscious. Cogn . 4, 1–21. doi: 10.1006/ccog.1995.1001
Grossman, P., and Van Dam, N. T. (2011). Mindfulness, by any other name … :trials and tribulations of sati in western psychology and science. Contemp. Buddhism 12, 219–239. doi: 10.1080/14639947.2011.564841
Kane, M. J., Brown, L. H., McVay, J. C., Silvial, P. J., Myin-Germeys, I., and Kwapil, T. R. (2007). For whom the mind wanders, and when: an experience-sampling study of working memory and executive control in daily life. Psychol. Sci . 18, 614–621. doi: 10.1111/j.1467-9280.2007.01948.x
Killingsworth, M. A., and Gilbert, D. T. (2010). A wandering mind is an unhappy mind. Science 330, 932. doi: 10.1126/science.1192439
Kuhn, D. (2000). Metacognitive development. Perspect. Psychol. Sci . 9, 178–181. doi: 10.1111/1467-8721.00088
Mason, M. F., Norton, M. I., Van Horn, J. D., Wegner, D. M., Grafton, S. T., and Macrae, C. N. (2007). Wandering minds: the default network and stimulus-independent thought. Science 315, 393–395. doi: 10.1111/j.1467-9280.2007.01948.x
McVay, J. C., and Kane, M. J. (2009). Conducting the train of thought: working memory capacity, goal neglect, and mind wandering in an executive-control task. journal of experimental psychology. Learn. Mem. Cogn . 35, 196–204. doi: 10.1037/a0014104
McVay, J. C., and Kane, M. J. (2012). Drifting from slow to “d'oh!”: working memory capacity and mind wandering predict extreme reaction times and executive control errors. J. Exp. Psychol. Learn. Mem. Cogn . 38, 525–549. doi: 10.1037/a0025896
Mrazek, M. D., Franklin, M. S., Phillips, D., Baird, B., and Schooler, J. (2013). Mindfulness training improves working memory and GRE performance while reducing mind-wandering. Psychol. Sci . 24, 776–781. doi: 10.1177/0956797612459659
Mrazek, M. D., Smallwood, J., Franklin, M. S., Baird, B., Chin, J., and Schooler, J. W. (2012a). The role of mind-wandering in measurements of general aptitude. J. Exp. Psychol. Gen . 141, 788–798. doi: 10.1037/a0027968
Mrazek, M. D., Smallwood, J., and Schooler, J. W. (2012b). Mindfulness and mind-wandering: finding convergence through opposing constructs. Emotion 12, 442–448. doi: 10.1037/a0026678
Polderman, T. J. C., Boomsma, D. I., Bartels, M., Verhulst, F. C., and Huizink, A. C. (2010). A systematic review of prospective studies on attention problems and academic achievement. Acta Psychiatric. Scand . 122, 271–284. doi: 10.1111/j.1600-0447.2010.01568.x
Risko, E. F., Anderson, N., Sarwal, A., Engelhardt, M., and Kingstone, A. (2012). Everyday attention: variation in mind-wandering and attention in a lecture. Appl. Cogn. Psychol . 26, 234–242. doi: 10.1002/acp.1814
Schooler, J. W., Smallwood, J., Christoff, K., Handy, T. C., Reichle, E. D., and Sayette, M. A. (2011). Meta-awareness, perceptual decoupling and the wandering mind. Trends Cogn. Sci . 15, 319–326. doi: 10.1016/j.tics.2011.05.006
Smallwood, J., Beach, E., Schooler, J. W., and Handy, T. C. (2008). Going AWOL in the brain: mind wandering reduces cortical analysis of external events. J. Cogn. Neurosci . 20, 458–469. doi: 10.1162/jocn.2008.20037
Smallwood, J., Davies, J. B., Heim, D., Finnigan, F., Sudberry, M., O'Connor, R., et al. (2004). Subjective experience and the attentional lapse: task engagement and disengagement during sustained attention. Conscious. Cogn . 13, 657–690. doi: 10.1016/j.concog.2004.06.003
Smallwood, J., Fitzgerald, A., Miles, L. K., and Phillips, L. H. (2009). Shifting moods, wandering minds: negative moods lead the mind to wander. Emotion 9, 271. doi: 10.1037/a0014855
Smallwood, J., O'Connor, R. C., and Heim, D. (2005). Rumination, dysphoria, and subjective experience. Imagin . Cogn. Pers . 24, 355–367. doi: 10.2190/AE18-AD1V-YF7L-EKBX
Smallwood, J., and Schooler, J. W. (2006). The restless mind. Psychol. Bull . 132, 946–958. doi: 10.1037/0033-2909.132.6.946
Stawarczyk, D., Majerus, S., Van der Linden, M., and D'Argembeau, A. (2012). Using the daydreaming frequency scale to investigate the relationships between mind-wandering, psychological well-being, and present-moment awareness. Front. Psychol . 3:363. doi: 10.3389/fpsyg.2012.00363
Unsworth, N., Heitz, R. P., Schrock, J. C., and Engle, R. W. (2005). An automated version of the operation span task. Behav. Res. Methods 37, 498–505. doi: 10.3758/BF03192720
Wallace, B. A., and Shapiro, S. L. (2006). Mental balance and well-being: building bridges between Buddhism and Western psychology. Am. Psychol . 61, 690–701. doi: 10.1037/0003-066X.61.7.690
Keywords: mind-wandering, attention, reading comprehension, K-12 education, well-being
Citation: Mrazek MD, Phillips DT, Franklin MS, Broadway JM and Schooler JW (2013) Young and restless: validation of the Mind-Wandering Questionnaire (MWQ) reveals disruptive impact of mind-wandering for youth. Front. Psychol . 4 :560. doi: 10.3389/fpsyg.2013.00560
Received: 29 May 2013; Paper pending published: 14 June 2013; Accepted: 06 August 2013; Published online: 27 August 2013.
Reviewed by:
Copyright © 2013 Mrazek, Phillips, Franklin, Broadway and Schooler. This is an open-access article distributed under the terms of the Creative Commons Attribution License (CC BY) . The use, distribution or reproduction in other forums is permitted, provided the original author(s) or licensor are credited and that the original publication in this journal is cited, in accordance with accepted academic practice. No use, distribution or reproduction is permitted which does not comply with these terms.
*Correspondence: Michael D. Mrazek, Department of Psychological and Brain Sciences, University of California, Building 251 Psychology East, UCEN Rd., Santa Barbara, CA 93106, USA e-mail: [email protected]
Disclaimer: All claims expressed in this article are solely those of the authors and do not necessarily represent those of their affiliated organizations, or those of the publisher, the editors and the reviewers. Any product that may be evaluated in this article or claim that may be made by its manufacturer is not guaranteed or endorsed by the publisher.
Thank you for visiting nature.com. You are using a browser version with limited support for CSS. To obtain the best experience, we recommend you use a more up to date browser (or turn off compatibility mode in Internet Explorer). In the meantime, to ensure continued support, we are displaying the site without styles and JavaScript.
- View all journals
- My Account Login
- Explore content
- About the journal
- Publish with us
- Sign up for alerts
- Open access
- Published: 18 February 2023
Ecological momentary assessment of mind-wandering: meta-analysis and systematic review
- Issaku Kawashima 1 ,
- Tomoko Hinuma 1 &
- Saori C. Tanaka 1 , 2
Scientific Reports volume 13 , Article number: 2873 ( 2023 ) Cite this article
2388 Accesses
1 Citations
4 Altmetric
Metrics details
- Human behaviour
Mind-wandering (MW) is a universal human phenomenon and revealing its nature contributes to understanding consciousness. The ecological momentary assessment (EMA), in which subjects report a momentary mental state, is a suitable method to investigate MW in a natural environment. Previous studies employed EMA to study MW and attempted to answer the most fundamental question: How often do we let our minds wander? However, reported MW occupancies vary widely among studies. Further, while some experimental settings may induce bias in MW reports, these designs have not been explored. Therefore, we searched PubMed and Web of Science for articles published until the end of 2020 and systematically reviewed 25 articles, and performed meta-analyses on 17 of them. Our meta-analysis found that people spend 34.504% of daily life in mind-wandering, and meta-regression revealed that using subject smartphones for EMA, frequent sampling, and long experimental duration significantly affect MW reports. This result indicates that EMA using subject smartphones may tend to collect sampling under habitual smartphone use. Furthermore, these results indicate the existence of reactivity, even in MW research. We provide fundamental knowledge of MW and discuss rough standards for EMA settings in future MW studies.
Similar content being viewed by others
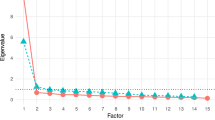
On the relationship between mind wandering and mindfulness
Angelo Belardi, Leila Chaieb, … Thomas P. Reber
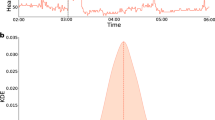
Real-time sensing of war’s effects on wellbeing with smartphones and smartwatches
Merav Mofaz, Matan Yechezkel, … Erez Shmueli
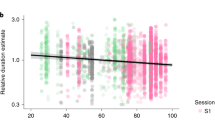
The Blursday database as a resource to study subjective temporalities during COVID-19
Maximilien Chaumon, Pier-Alexandre Rioux, … Virginie van Wassenhove
Introduction
Today, ecological momentary assessment (EMA) is an indispensable method to investigate the nature of mind-wandering (MW 1 ). MW, a phenomenon in which one’s attention drifts away from the current task or situation, has received attention from psychologists and cognitive neuroscientists. People spend much of the time allowing their minds to wander 2 , and during MW, they incubate ideas, which contributes to creativity 2 , 3 , MW is a worthy theme of cognitive psychology and neuroscience. Furthermore, clinical psychologists also focus on MW because it may worsen mood and cause depression 4 , 5 , 6 . It is thought to be related to a powerful psychotherapy mechanism called “mindfulness-based intervention” 7 , 8 . While some behavioral 9 , 10 , neural 11 , 12 , and other physiological indices 11 , 13 , 14 have been suggested as indirect approaches, the only direct assessment tool for private MW experience is self-reporting 15 . It is widely thought that EMA should be used when assessing MW in daily life, because it is a self-reporting method that can capture MW that is progressing unconsciously. In EMA, questions are delivered to participants using smartphones or similar devices while they are spending their time normally, and participants respond to these inquiries about their current mental states 16 . While MW often progresses unconsciously, abruptly delivered probes make participants aware of their MW and enable researchers to observe unconsciously progressing MW. Moreover, while retrospective reports of MW have the risk of recall bias 17 , EMA asking about momentary states can avoid such risk 15 , 18 .
Indeed, many previous studies employed EMA to investigate MW and reported major findings, including the amount of time spent in MW during normal activities. Though EMA is the best way to address this issue, reported MW rates vary widely between articles. We hypothesized that differences in two aspects of study design may explain this inconsistency. First, we hypothesized that differences in the number of questions asked between scenarios when MW exists and when it does not, reduce MW reporting. In some MW EMA studies, contents of sampling were branched, based on the answer. In a typical design with branching questions, the first question asks whether participants are absorbed in MW. If they answer “Yes”, then they are required to answer additional questions about characteristics of MW, e.g., emotional value, temporal orientation, etc. However, if they answer “No”, additional questions are skipped and the sampling ends immediately or soon thereafter. While such branching eliminates meaningless questions and allows subjects to save time, it may encourage subjects to answer “No” in order to avoid answering so many questions. Second, we hypothesized that utilizing participant smartphones biases the reported MW rate, because sampling may concentrate on the smartphone-use context. Some MW EMA studies lent subjects an electronic device designed for EMA and probes were submitted to it, and others sent probes through subject smartphones using an app for EMA or messages. When smartphones are employed, subjects are sometimes unaware of probe notifications, but this is not possible if the probe arrives while participants are actively using their smartphones. Hence, EMA may be prone to gathering answers when participants are using their smartphones. Because the phones provide interesting content for users, people may experience less MW while using them. Hence, this overrepresentation of smartphone-use may underestimate the MW rate.
Therefore, in this study, we systematically reviewed designs of MW EMA studies and meta-analyzed them. First, we statistically synthesized reported MW rates and calculated the average MW occupancy. Then, we performed meta-regression analyses and investigated experimental settings that altered MW reports. As mentioned above, we hypothesized that question branching and smartphone use affect reported MW. Moreover, though this was not originally planned, we explored other experimental parameters having a risk of bias. Finally, based on results of meta-regression and systematic review, we discussed and suggested a rough standard of experimental design when using EMA for MW research.
Even though there are articles reviewing EMA studies and summarizing their designs 19 , 20 , 21 , 22 , 23 and other studies empirically exploring experimental parameters 24 , 25 , 26 , this study, which targeted MW studies regarding healthy people, has clear originality. As EMA designs can be tailored for individual study purposes 20 , different trends regarding experimental parameters can be seen in MW EMA studies. Moreover, most importantly, this is the first study to investigate the risk of bias due to specific EMA design settings in MW reports.
Eligibility criteria
All articles published in English through 2020, and fulfilling the following criteria were included in this systematic review: (1) reporting studies conducting an EMA and (2) EMAs targeting MW, including task-unrelated thought, self-generated thought, spontaneous thought, stimulus-independent thought, and day-dreaming.
From these, articles matching any of the following exclusion criteria were excluded from the meta-analysis: (1) The mean and SD of MW rate were not provided by authors, and the reviewer could not calculate them from the report or the available data; (2) the MW rate was reported only from an atypical subject group, e.g., those with specific disorders or with special training; or (3) subjects who reported few or excessive MW were rejected and MW rate before rejection was not provided.
To check the publication date, we referred only to the date on which the issue of the journal was published (coded as PD in PubMed) and not other types of publication dates, such as the date of electronic publication.
Reviewing process
We searched PubMed and Web of Science on 21 July 2021 (and repeated the search on 8 February 2022) for articles using the following terms: '(mind-wandering OR "mind wandering" OR "task-unrelated thought" OR "task unrelated thought" OR "self-generated thought" OR "spontaneous thought" OR "day-dreaming" OR "stimulus-independent thought" OR "stimulus independent thought") AND ("experience sampling" OR "ecological momentary assessment" OR "ambulatory assessment")'.
Reviewers independently checked abstracts and examined eligibility for systematic reviews and meta-analyses in duplicate. Articles judged ineligible by both reviewers were excluded. We retrieved the full text of articles that passed the first triage. Two reviewers independently reviewed full texts and extracted data items. Their evaluations were compared and conflicts were resolved by discussions.
We extracted the duration of the experiment, sampling frequency, counts of question items per probe, rewards, characteristics of subjects, numbers of analyzed and removed subjects, criteria for subject removal, EMA tool, mean compliance rate, and mean MW rate. The MW rate is the total number of MW answers per subject divided by the total number of probes per subject, and the mean MW rate is its average. When the sampling frequency varied by subject or by experiment day, or when the question items in one probe varied because of branching, we calculated their expected value. For example, in McVay et al. (2009) 27 , a probe contained 24 items if MW existed and 19 if it did not, and the mean MW rate was 30%. In this article, we calculated the expected value as (24 × 0.3) + (19 × 0.7) = 20.5. If the study examined MW using a Likert scale, the central point or higher was treated as MW 28 . When these data items could not be extracted, we acquired the raw data and calculated them if the data were publicly available. Otherwise, we asked the investigators about unclear items by email. We excluded articles with unclear or missing items from the analysis, if efforts to clarify or recover them were unsuccessful.
Though we had planned during the pre-registration to summarize the time limit for responses and instructions for subjects on what to do when they could not answer immediately, we abandoned these objectives because few articles reported them. Additionally, we did not list all question items because they were more numerous than expected.
Data synthesis
We investigated homogeneity between studies with Q and I 2 and conducted a random-model, meta-analysis using the DerSimonian-Laird method. The primary outcome was the MW rate, that is, the ratio of affirmative answers regarding engaging in MW. The reported mean MW rates and their confidence intervals were displayed in a forest plot. To determine the average, we performed meta-regressions and investigated the relation between MW rate and some variables. The main independent variables were differences in the number of questions between “if MW exists” and “if it does not”, and the EMA tool, i.e., whether subject smartphones were used for EMA, and both were study-level variables. Though the first independent variable was not part of the original design, we decided to investigate it during the review of articles.
Along with the originally planned hypothesis testing, we performed exploratory analyses. First, we conducted additional meta-regression and explored other factors that increase or decrease MW answers. We used the duration, sampling frequency, and the number of questions per probe. p values for estimated slopes were corrected using the Benjamini–Hochberg procedure. Second, we repeated the same analyses on the reported compliance rate to help researchers decide on parameters for avoiding bias in MW reports. That is, we summarized the compliance rate in a table (Supplementary Table S1 ) and a forest plot, conducted meta-regressions, and explored whether the foregoing experimental parameters affect compliance.
We assumed that the risk of publication bias was quite small, because the extracted data, e.g., the MW rate, was not thought to affect publication. Similarly, because the extracted data were fundamental EMA data, they are not likely to be selectively reported. Hence, we did not assess meta-bias. We did not assess biases in individual studies because this review does not target intervention effects, and there are no suggestions regarding possible risk of bias for extracted data.
For statistical synthesis, we used R version 4.1.0 (2021-05-18) and the metafor 3.0.2 package. This study was conducted based on the Preferred Reporting Items for Systematic Reviews and Meta-Analysis (PRISMA, 2020 29 ) guidelines, and our protocols were pre-registered on OSF ( https://osf.io/m8qk3/?view_only=e2e0a9be1f1f47328635df2331989d7a ).
Additional analyses
After completing the above-mentioned review and data synthesis, we reviewed the articles again and extracted some additional items. We extracted the type of scale asking about MW and the reported ages of participants. Further, we extracted the definition of MW that was given to participants. In other words, we summarized what rate we extracted as the “MW rate.” We extracted this information from questions presented to participants in EMA. However, if authors stated that they introduced the definition of MW to the participants, we ignored the question. Instead, we extracted the definition from the introduction, or if it was unavailable, we extracted the definition described in the paper.
Afterward, we found that almost all articles defined MW as task-unrelated thought (TUT) or stimulus-independent (SI) TUT. Therefore, during supplementary analyses, we conducted the same data synthesis of MW rate with only those articles, from which we extracted TUT or SI TUT as MW.
In the meta-regression of duration and sampling frequency, we found two articles (one in each analysis) that appeared to be outliers. Therefore, as a sensitivity analysis, we repeated these analyses excluding the article that appeared to be an outlier.
Study selection
Our flow of study selection is shown in Fig. 1 . We found 32 articles in the Web of Science and 70 in PubMed. Of these, 28 were duplicates and were excluded. We also excluded 40 articles for not performing EMA (laboratory experiments only), two that did not target MW, and three that were review papers. Furthermore, a paper 13 that shared data with an included article 30 , an abstract for a conference 31 , a paper targeting only a clinical sample 32 , and a corrigendum 33 were rejected (“Other reasons” in Fig. 1 ). Finally, we included 25 articles for the systematic review.
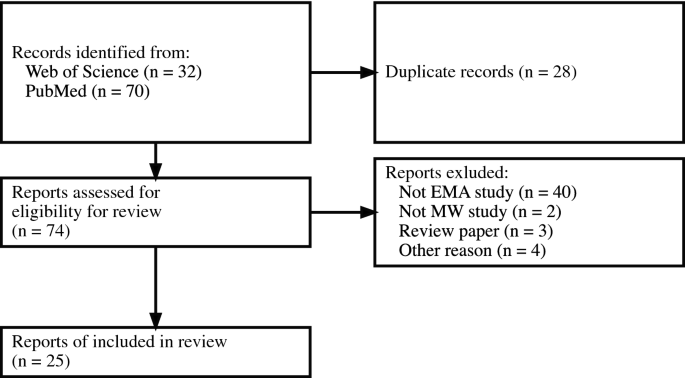
Flow chart of the study selection protocol.
Syntheses of experimental parameters
We summarize experimental duration (number of days), number of probes per day, expected number of questions in a probe, number of questions if MW did not occur and if it did, reward for subjects, number of included and excluded subjects in EMA, exclusion criteria, whether subject smartphones were utilized, and the mean and SD of compliance and MW rates (Supplementary Table S1 ). Distribution of the number of days and probes per day, subject exclusion rate, the threshold of compliance rate for exclusion, and the number of questions if MW occurred and if it did not are plotted in Fig. 2 . The number of included studies, mean, mode in the kernel density estimation, SD , and minimum and maximum values of these parameters are provided in Table 1 . Of all studies, 45.833% used subject smartphones for EMA. From these, we extracted task-unrelated thought from 16 articles, stimulus-independent task-unrelated thought from 4, stimulus-independent thought without rumination and anxiety from 3, freely-moving task-unrelated thought from 1, and freely-moving mind from 1.
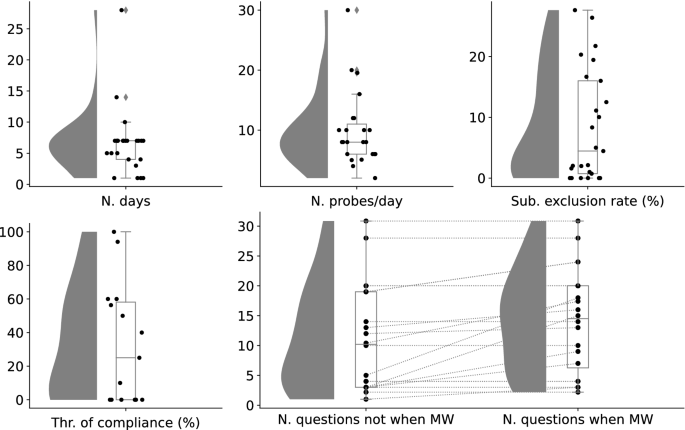
The distribution of experimental parameters of reviewed articles. N. days Number of days (Experiment duration), N. probes/day Expected number of probes per day, Sub. exclusion rate (%) Rate of excluded subjects to recruited subjects, Thr. of compliance (%) Threshold of compliance rate (only if authors used compliance rate as a criterion of subject exclusion), N. questions not when MW Expected number of questions if MW does not exist, N. questions when MW Expected number of questions if MW exists.
Meta-analyses
Sixteen articles reported the mean MW rate and 16 reported the mean compliance rate, and we used them to conduct meta-analyses. We found large heterogeneity between reviewed articles (MW rate: Q = 534.686, p < 0.001, I 2 = 97.195 (95.851–99.075), N = 16; compliance: Q = 1226.747, p < 0.001, I 2 = 98.777 (97.254–99.378), N = 16). The meta-analysis indicated that the mean MW rate was 34.504% ( CI = 29.607–39.401%; Fig. 3 ) and the mean compliance rate was 76.727% ( CI = 69.828–83.625%; Fig. 4 ). The meta-regression showed that utilizing subject smartphones ( Estimate = 10.56; p = 0.03; 95% CI = 1.29–19.82), experiment duration ( Estimate = 1.26; p < 0.001; 95% CI = 0.6–1.92), and number of probes per day ( Estimate = − 0.86; p = 0.01; 95% CI = − 1.51–− 0.2) significantly affected reported MW rates.
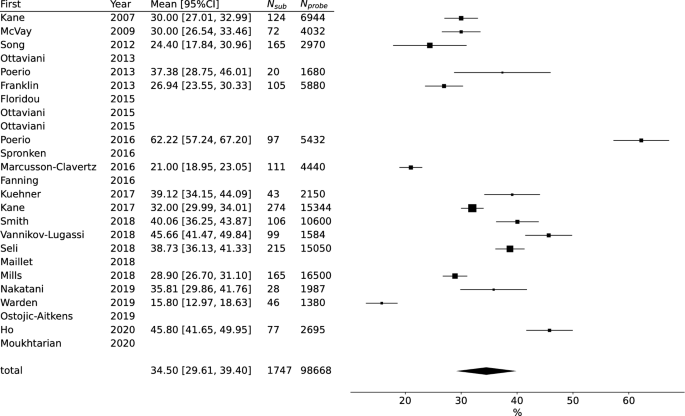
Forest plot of mind-wandering rate. The effect size and corresponding bar were blank if the mean MW rate was unavailable. First First author of studies, Year Year of publication, Mean [95%CI] , Reported mean MW rate and 95% confidence intervals, N sub Included number of subjects, N probe Included total number of probes.
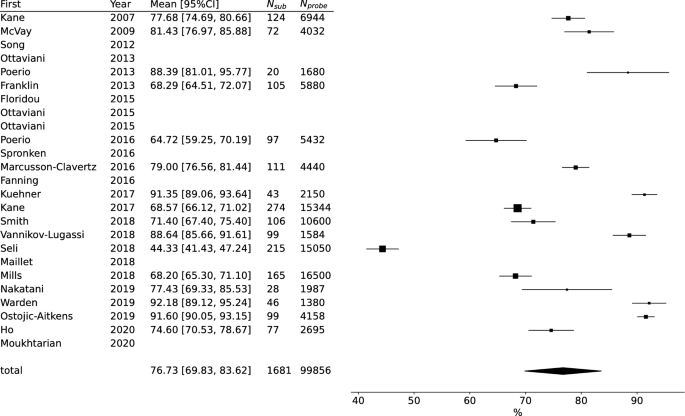
Forest plot of compliance rate. The effect size and corresponding bar are blank if the mean compliance rate was unavailable. First First author of studies, Year Year of publication, Mean [95%CI] Reported mean compliance rate and 95% confidence intervals, N sub Included number of subjects, N probe Included total number of probes.
Results of meta-regression for MW rate are shown in Table 2 and Fig. 5 , and for compliance, in Table 3 and Fig. 6 . The regulating definition of MW (only TUT or SI TUT articles were included) did not cause different results (Supplementary Results and Supplementary Table S2 ). The meta-regression of duration and sampling frequency without the outlier article did not show significant effects on MW rates (Supplementary Results and Figure).
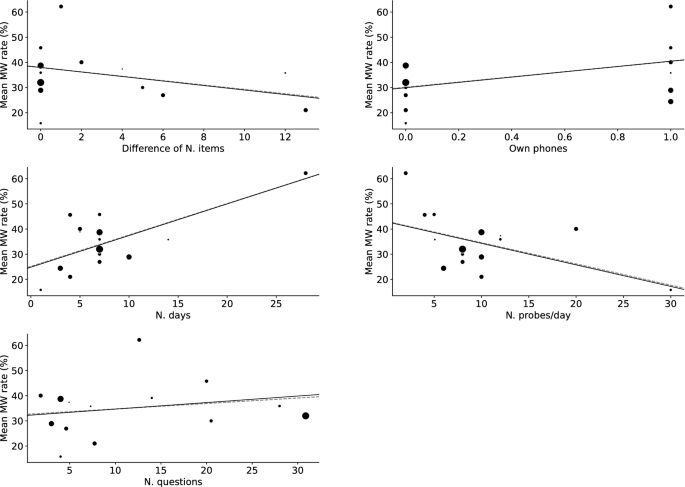
Scatter and meta-regression plot of mind-wandering rate. Marker sizes reflect article sample sizes. The solid line denotes the meta-regression line biased due to article weights. The dashed line denotes the unweighted regression line. N. days Number of days (Experiment duration), N. probes/day Expected number of probes per day, Sub. exclusion rate (%) Rate of excluded subjects to recruited subjects, Thr. of compliance (%) Threshold of compliance rate (only if authors used compliance rate as a criterion of subject exclusion), N. questions not when MW Expected number of questions if MW does not exist. N. questions when MW Expected number of questions if MW exists.
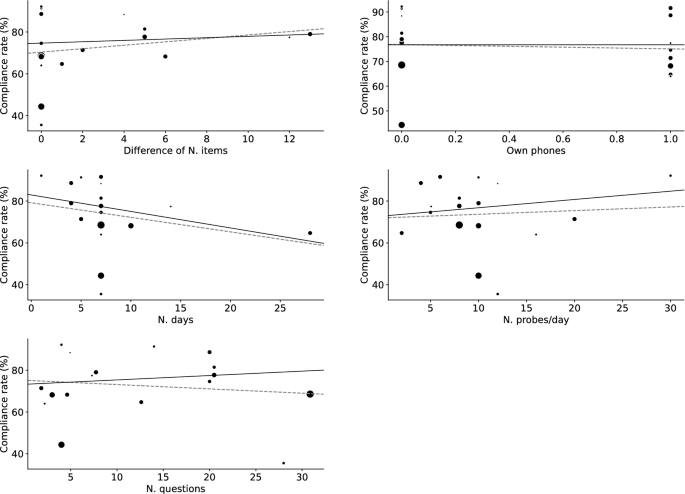
Scatter and meta-regression plot of compliance rate. Marker sizes reflect article sample sizes. The solid line is the meta-regression line biased by article weights. The dashed line denotes the unweighted regression line. N. days Number of days (Experiment duration), N. probes/day Expected number of probes per day, Sub. exclusion rate (%) Rate of excluded subjects to recruited subjects, Thr. of compliance (%) Threshold of compliance rate (only if authors used compliance rate as a criterion of subject exclusion), N. questions not when MW Expected number of questions if MW does not exist, N. questions when MW Expected number of questions if MW exists.
This study systematically collected articles, reviewed them to summarize experimental parameters, calculated the mean proportion of MW, and investigated variables that affect the MW rate.
The definition of MW
A large number of reviewed articles provided the ratio of TUT or SI TUT. Even if an article mainly targeted more narrowly defined MW, e.g., TUT without meta-awareness, in many cases, the first question was about TUT and its details in subsequent questions. TUT was defined as thoughts or images not directed toward one’s current activity 34 , and SI means that it comes from the internal mental process and is not related to the immediate environment 35 . Even if articles that adopted different definitions of MW were rejected from data synthesis, the statistical significance did not differ. Note that this does not imply that the difference in MW type did not affect MW report, since we just tested the result without articles treating freely-moving thought and did not compare MW type thoroughly.
Meta-analysis
While a meta-analysis showed that people engage in MW for one-third of their waking hours, large heterogeneity between articles existed. Not only the hypothesized variables, but also other experimental parameters affected the MW report. The more frequently subjects received probes or the shorter the duration of the experiment, the less they reported MW. These results may indicate that reactivity (observer effect; Hawthorne effect) occurs even in MW EMA research to some extent. Reactivity refers to the effect on research outcomes derived from the study protocol 36 . Receiving constant EMA probes (or expectation of this) could lead subjects be more aware of their MW, and their MW may be shortened. The assumption that reactivity occurred explains the effects of experimental duration and sampling frequency simultaneously. More frequent sampling is thought to reinforce reactivity 37 ; thus, the number of probes per day decreased MW reports in our meta-analysis. Considering that this reactivity ceased as participants became accustomed to it 38 , it is reasonable that longer experiments increased reported MW.
From the scatter plot (Figs. 5 and 6 ), both regressions included one article that appeared to be an outlier 39 , 40 . In meta-analyses, outlier rejection is not recommended 41 . Particularly, rejection of such articles should be carefully conducted. While outliers in measured data indicate potential measurement errors and are usually rejected, in the current case, authors intentionally designed settings are outliers rather than measured data. However, in any case, sensitivity analyses without these articles did not show the same significance and we cannot argue that those were robust results.
Using meta-regression, we found that utilizing subject smartphones for EMA significantly increased the MW rate, contrary to our hypothesis that since smartphones provide users with interesting content, MW would be reduced. We interpret this result to mean that people experience more MW while using smartphones. Previous research indicated that 60% of smartphone use was habitual and absent-minded 42 . It has been strongly suspected that people report MW during habitual smartphone use, and indeed the propensity for habitual use correlates well with the MW trait 43 . In smartphone-based EMA, reports during habitual use may be overrepresented.
Otherwise, given the potential reactivity found in the current meta-analysis, this smartphone effect can also be explained as reactivity. Compared to habitually carried smartphones, additional EMA devices may more readily remind subjects of the measurement. Hence, extra wearable devices to gather additional data, e.g., heart rate, can also enhance reactivity (no articles used subject smartphones or extra devices in our meta-analysis). However, the current systematic review and meta-analysis cannot reveal which interpretation (overrepresentation of smartphone using condition vs. enhancement of reactivity) is correct. Future studies that directly compare EMA protocols with and without subject smartphones should reveal it.
However, we did not find a significant effect from use of branching questions in cases in which participants reported MW. This result did not support the contention that the risk of allowing subjects to skip questions leads to bias in the report. However, it is still possible that branching questions selectively bias MW reports in unmotivated participants, e.g., people participating in an experiment only for cash reward.
Suggestions regarding experimental parameters
Based on the aforementioned meta-analysis and a summary of previous studies, some suggestions about experimental parameters in future MW EMA studies are appropriate. The meta-analysis showed that using subject smartphones affects MW reports; however, it did not support the supposition that using a smartphone or a dedicated device (or both) leads to bias. If using subject smartphones drives bias, it would be effective to include a question asking about momentary smartphone use or collecting use data, so as to consider whether subjects used them when they received probes. To cope with the potential effect of EMA-dedicated devices or extra wearable devices, we can use a trial period to acclimate the user to the device. Future studies are expected to reveal which (or both) approaches are effective for more precise EMA.
The mode value of experimental duration varied, centering on a week, except for a paper performing a 28-day experiment 39 . Given that reactivity indicated by our meta-analysis and previous studies showed that longer experiments did not degrade compliance 21 , 22 , 23 , we recommend a long-term experiment if an assessment in a maximally natural environment is needed. However, from this study, we cannot propose a duration for which the observer effect would cease. At present, we suggest a seven-day experiment for compatibility with previous studies. Notably, when a long-term experiment is performed, researchers need to pay attention to the possibility that the earlier and later parts of individual datasets may not be homogeneous.
Sampling frequency also appears to produce reactivity. Considering that the mode value of frequency in previous research was 8.0/day, and a study reported that the quality and compliance rate were unchanged by fewer than 9 probes/day in previous studies 24 , 25 , we suggest using the minimum required frequency, which ideally, is fewer than 9.
While the numbers of questions varied, all except for one study (33 maximum 44 ) adopted fewer than 30 items. We think that future studies should set the number of questions equal to the number of aspects of MW being investigated; however, to maintain compatibility with previous studies, answer quality 24 , 25 , and compliance 24 , we recommend restricting it to under 30 questions. Though there was no evidence indicating a risk caused by branching questions, further studies are needed. If we want to avoid branching, we can allow subjects to answer based on the latest MW experience if their minds are not wandering when the probe arrives 39 .
When designing an experiment using EMA, it is also beneficial to consider this recommendation driven by lab-based experiments. In the probe-caught paradigm, participants sometimes receive and answer a probe asking about their momentary attentional state while performing a cognitive task demanding relatively simple focusing. We may be able to understand EMA as a probe-caught paradigm extended from the laboratory to daily life (aside from the history of method development). Therefore, it would be beneficial to consider knowledge and recommendations from lab-based probe-caught paradigm studies. One article 15 reviewed probe-caught paradigm experiments and suggested some recommendations about probe framing and response options, etc. Another study 17 experimentally investigated the effect of different probe types and recommended that probes ask about the content of thought, rather than the intensity of MW. These proposals would be compatible with EMA studies.
Limitations and Future Directions
While we tried to comprehensively collect articles with pre-registered search strategies, it is possible that we missed some papers. Particularly, we might have missed several articles published before EMA-related terms such as “EMA” or “experience sampling” became widespread among scientists. However, we think we achieved the study aim, which was to contribute to future EMA MW research, by covering relatively recent papers.
Readers should note that our review and meta-analysis did not include Killingsworth and Gilbert (2010) 45 , the largest EMA MW study conducted, because EMA-relating words were not included. This study includes an enormous number of participants (2,250) so including this study would have heavily biased the results. The design of that study deviated strongly from other studies in that people freely download the app and started and terminated their participation in the study. The enormous contribution of this study notwithstanding, its unmanaged design would have biased our results in ways that would be difficult to control, so, we felt that removing it from the present meta-analysis was prudent.
Since the number of MW EMA studies is still growing, re-performing the meta-analysis of EMA MW articles after decade or more is expected not only to validate our current results, but also to resolve some limitations of the current study. First, such a future study would summarize differences in the occupancy of each MW type. Investigating the effect of MW types is beyond the intent of the current research and it is difficult to determine it from the collected articles because they mainly targeted TUT. However, this is a crucial topic for MW studies, given that TUT has different associations with mental problems 46 and brain activity 47 depending on whether it is stimulus-independent or stimulus-dependent, and future meta-analysis is expected to evaluate it. Moreover, with more articles, it will be possible to statistically control some characteristics of subjects in meta-analyses. For example, since the age of subjects correlates with an MW trait 48 , it can be entered as a co-variate.
Data availability
Data and codes that support the findings of this study are available in the supplementary information.
Smallwood, J. & Schooler, J. W. The restless mind. Psychol. Bull. 132 , 946–958 (2006).
Article PubMed Google Scholar
Smallwood, J. & Schooler, J. W. The science of mind wandering: Empirically navigating the stream of consciousness. Annu. Rev. Psychol. 66 , 487–518 (2015).
Williams, K. J. H. et al. Conceptualising creativity benefits of nature experience: Attention restoration and mind wandering as complementary processes. J. Environ. Psychol. 59 , 36–45 (2018).
Article Google Scholar
Burg, J. M. & Michalak, J. The healthy quality of mindful breathing: Associations with rumination and depression. Cogn. Ther. Res. 35 , 179–185 (2011).
Hoffmann, F., Banzhaf, C., Kanske, P., Bermpohl, F. & Singer, T. Where the depressed mind wanders: Self-generated thought patterns as assessed through experience sampling as a state marker of depression. J. Affect. Disord. 198 , 127–134 (2016).
Smallwood, J., O’Connor, R. C., Sudbery, M. V. & Obonsawin, M. Mind-wandering and dysphoria. Cogn. Emot. 21 , 816–842 (2007).
Kabat-Zinn, J. Mindfulness. Mindfulness 6 , 1481–1483 (2015).
Wang, Y., Xu, W., Zhuang, C. & Liu, X. Does mind wandering mediate the association between mindfulness and negative mood? A preliminary study. Psychol. Rep. 120 , 118–129 (2017).
Robertson, I. H., Manly, T., Andrade, J., Baddeley, B. T. & Yiend, J. ‘Oops!’: Performance correlates of everyday attentional failures in traumatic brain injured and normal subjects. Neuropsychologia 35 , 747–758 (1997).
Article CAS PubMed Google Scholar
Esterman, M., Noonan, S. K., Rosenberg, M. & DeGutis, J. In the zone or zoning out? Tracking behavioral and neural fluctuations during sustained attention. Cereb. Cortex 23 , 2712–2723 (2013).
Madore, K. P. et al. Memory failure predicted by attention lapsing and media multitasking. Nature 587 , 87–91 (2020).
Article ADS CAS PubMed PubMed Central Google Scholar
Kam, J. W. Y. et al. Distinct electrophysiological signatures of task-unrelated and dynamic thoughts. Proc. Natl Acad. Sci. U. S. A. 118 , e2011796118 (2021).
Franklin, M. S. et al. Tracking distraction: The relationship between mind-wandering, meta-awareness, and ADHD symptomatology. J. Atten. Disord. 21 , 475–486 (2017).
Unsworth, N. & Robison, M. K. Pupillary correlates of lapses of sustained attention. Cogn. Affect. Behav. Neurosci. 16 , 601–615 (2016).
Weinstein, Y. Mind-wandering, how do I measure thee with probes? Let me count the ways. Behav. Res. Methods 50 , 642–661 (2018).
Shiffman, S., Stone, A. A. & Hufford, M. R. Ecological momentary assessment. Annu. Rev. Clin. Psychol. 4 , 1–32 (2008).
Kane, M. J., Smeekens, B. A., Meier, M. E., Welhaf, M. S. & Phillips, N. E. Testing the construct validity of competing measurement approaches to probed mind-wandering reports. Behav. Res. Methods 53 , 2372–2411 (2021).
Article PubMed PubMed Central Google Scholar
Schooler, J. W., Smallwood, J., Handy, T. C., Reichle, E. D. & Sayette, M. A. Meta-awareness, perceptual decoupling and the wandering mind. Trends Cogn. Sci. 15 , 319–326 (2011).
PubMed Google Scholar
van den Heuvel, M. I. et al. Tracking infant development with a smartphone: A practical guide to the experience sampling method. Front. Psychol. 12 , 703743 (2021).
Hall, M., Scherner, P. V., Kreidel, Y. & Rubel, J. A. A systematic review of momentary assessment designs for mood and anxiety symptoms. Front. Psychol. 12 , 642044 (2021).
Wen, C. K. F., Schneider, S., Stone, A. A. & Spruijt-Metz, D. Compliance with mobile ecological momentary assessment protocols in children and adolescents: A systematic review and meta-analysis. J. Med. Internet Res. 19 , e132 (2017).
Jones, A. et al. Compliance with ecological momentary assessment protocols in substance users: A meta-analysis. Addiction 114 , 609–619 (2019).
Vachon, H., Viechtbauer, W., Rintala, A. & Myin-Germeys, I. Compliance and retention with the experience sampling method over the continuum of severe mental disorders: Meta-analysis and recommendations. J. Med. Internet Res. 21 , e14475 (2019).
Eisele, G. et al. The effects of sampling frequency and questionnaire length on perceived burden, compliance, and careless responding in experience sampling data in a student population. Assessment 29 , 136–151 (2022).
Hasselhorn, K., Ottenstein, C. & Lischetzke, T. The effects of assessment intensity on participant burden, compliance, within-person variance, and within-person relationships in ambulatory assessment. Behav. Res. Methods 54 (4), 1541–1558. https://doi.org/10.3758/s13428-021-01683-6 (2021).
Smyth, J. M. et al. Influence of ecological momentary assessment study design features on reported willingness to participate and perceptions of potential research studies: An experimental study. BMJ Open 11 , e049154 (2021).
McVay, J. C., Kane, M. J. & Kwapil, T. R. Tracking the train of thought from the laboratory into everyday life: An experience-sampling study of mind wandering across controlled and ecological contexts. Psychon. Bull. Rev. 16 , 857–863 (2009).
Seli, P. et al. How pervasive is mind wandering, really?. Conscious. Cogn. 66 , 74–78 (2018).
Page, M. J. et al. The PRISMA 2020 statement: An updated guideline for reporting systematic reviews. BMJ 372 , n71 (2021).
Franklin, M. S. et al. The silver lining of a mind in the clouds: Interesting musings are associated with positive mood while mind-wandering. Front. Psychol. 4 , 583 (2013).
Bailey, T., Ottaviani, C., Shapiro, D. & Shahabi, L. The relationship between mood and anxiety disorders and cardiovascular risk: The role of rumination. In 75th Annual Scientific Meeting: Mobilizing Technology to Advance Biobehavioral Science and Health A69 (2017).
Marcusson-Clavertz, D., Gušić, S., Bengtsson, H., Jacobsen, H. & Cardeña, E. The relation of dissociation and mind wandering to unresolved/disorganized attachment: An experience sampling study. Attach. Hum. Dev. 19 , 170–190 (2017).
Poerio, G. L., Totterdell, P., Emerson, L.-M. & Miles, E. Corrigendum: Social daydreaming and adjustment: An experience-sampling study of socio-emotional adaptation during a life transition. Front. Psychol. 7 , 174 (2016).
PubMed PubMed Central Google Scholar
Kane, M. J. et al. For whom the mind wanders, and when: An experience-sampling study of working memory and executive control in daily life. Psychol. Sci. 18 , 614–621 (2007).
Song, X. & Wang, X. Mind wandering in Chinese daily lives - an experience sampling study. PLoS ONE 7 , e44423 (2012).
McCambridge, J., Witton, J. & Elbourne, D. R. Systematic review of the Hawthorne effect: New concepts are needed to study research participation effects. J. Clin. Epidemiol. 67 , 267–277 (2014).
McCarthy, D. E., Minami, H., Yeh, V. M. & Bold, K. W. An experimental investigation of reactivity to ecological momentary assessment frequency among adults trying to quit smoking: Reactivity to ecological momentary assessment. Addiction 110 , 1549–1560 (2015).
Clemes, S. A. & Deans, N. K. Presence and duration of reactivity to pedometers in adults. Med. Sci. Sports Exerc. 44 , 1097–1101 (2012).
Poerio, G. L., Totterdell, P., Emerson, L.-M. & Miles, E. Social daydreaming and adjustment: An experience-sampling study of socio-emotional adaptation during a life transition. Front. Psychol. 7 , 13 (2016).
Warden, E. A., Plimpton, B. & Kvavilashvili, L. Absence of age effects on spontaneous past and future thinking in daily life. Psychol. Res. 83 , 727–746 (2019).
Deeks, J., Higgins, J. & Altman, D. Chapter10: Analysing data and undertaking meta-analyses. In Cochrane handbook for systematic reviews of interventions version 6.3 (2022).
Hiniker, A., Patel, S. N., Kohno, T. & Kientz, J. A. Why would you do that? Predicting the uses and gratifications behind smartphone-usage behaviors. In Proceedings of the 2016 ACM International Joint Conference on Pervasive and Ubiquitous Computing 634–645 (ACM, 2016).
Marty-Dugas, J., Ralph, B. C. W., Oakman, J. M. & Smilek, D. The relation between smartphone use and everyday inattention. Psychol. Conscious. Theor. Res. Pract. 5 , 46–62 (2018).
Google Scholar
Kane, M. J. et al. For whom the mind wanders, and when, varies across laboratory and daily-life settings. Psychol. Sci. 28 , 1271–1289 (2017).
Killingsworth, M. A. & Gilbert, D. T. A wandering mind is an unhappy mind. Science 330 , 932–932 (2010).
Article ADS CAS PubMed Google Scholar
Arch, J. J., Wilcox, R. R., Ives, L. T., Sroloff, A. & Andrews-Hanna, J. R. Off-task thinking among adults with and without social anxiety disorder: An ecological momentary assessment study. Cogn. Emot. 35 , 269–281 (2021).
Stawarczyk, D., Majerus, S., Maquet, P. & D’Argembeau, A. Neural correlates of ongoing conscious experience: Both task-unrelatedness and stimulus-independence are related to default network activity. PLoS ONE 6 , e16997 (2011).
Maillet, D. & Schacter, D. L. From mind wandering to involuntary retrieval: Age-related differences in spontaneous cognitive processes. Neuropsychologia 80 , 142–156 (2016).
Download references
Acknowledgements
We would thank the authors of the papers we reviewed who shared or tried to share raw data and/or additional analyzed results with us. This research was supported by a KDDI collaborative research contract. It was also supported by Innovative Science and Technology Initiative for Security Grant Number JPJ004596, ATLA, Japan and JSPS KAKENHI Grant Number JP18K13332.
Author information
Authors and affiliations.
Brain Information Communication Research Laboratory Group, Advanced Telecommunications Research Institute International (ATR), Kyoto, Japan
Issaku Kawashima, Tomoko Hinuma & Saori C. Tanaka
Division of Information Science, Graduate School of Science and Technology, Nara Institute of Science and Technology, Nara, Japan
Saori C. Tanaka
You can also search for this author in PubMed Google Scholar
Contributions
All authors contributed to conceptualization and design of the study. I.K. and T.H. reviewed articles. I.K. and T.H. performed the statistical analysis. I.K. wrote the first draft of the manuscript. All authors contributed to manuscript revision, read, and approved the submitted version.
Corresponding authors
Correspondence to Issaku Kawashima or Saori C. Tanaka .
Ethics declarations
Competing interests.
The authors declare no competing interests.
Additional information
Publisher's note.
Springer Nature remains neutral with regard to jurisdictional claims in published maps and institutional affiliations.
Supplementary Information
Supplementary information 1., supplementary information 2., rights and permissions.
Open Access This article is licensed under a Creative Commons Attribution 4.0 International License, which permits use, sharing, adaptation, distribution and reproduction in any medium or format, as long as you give appropriate credit to the original author(s) and the source, provide a link to the Creative Commons licence, and indicate if changes were made. The images or other third party material in this article are included in the article's Creative Commons licence, unless indicated otherwise in a credit line to the material. If material is not included in the article's Creative Commons licence and your intended use is not permitted by statutory regulation or exceeds the permitted use, you will need to obtain permission directly from the copyright holder. To view a copy of this licence, visit http://creativecommons.org/licenses/by/4.0/ .
Reprints and permissions
About this article
Cite this article.
Kawashima, I., Hinuma, T. & Tanaka, S.C. Ecological momentary assessment of mind-wandering: meta-analysis and systematic review. Sci Rep 13 , 2873 (2023). https://doi.org/10.1038/s41598-023-29854-9
Download citation
Received : 05 October 2022
Accepted : 11 February 2023
Published : 18 February 2023
DOI : https://doi.org/10.1038/s41598-023-29854-9
Share this article
Anyone you share the following link with will be able to read this content:
Sorry, a shareable link is not currently available for this article.
Provided by the Springer Nature SharedIt content-sharing initiative
By submitting a comment you agree to abide by our Terms and Community Guidelines . If you find something abusive or that does not comply with our terms or guidelines please flag it as inappropriate.
Quick links
- Explore articles by subject
- Guide to authors
- Editorial policies
Sign up for the Nature Briefing newsletter — what matters in science, free to your inbox daily.

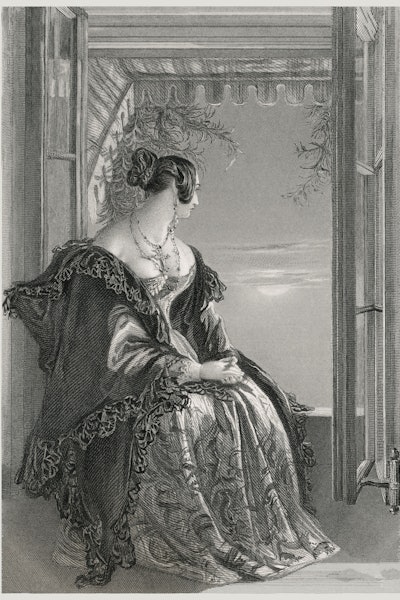
mikroman6/Moment/Getty Images
“Mind-wandering can be a benefit and a curse”
Scientists finally know why we get distracted — and how we can stay on track
More than just a distraction, mind-wandering (and its cousin, daydreaming) may help us prepare for the future
When psychologist Jonathan Smallwood set out to study mind-wandering about 25 years ago, few of his peers thought that was a very good idea. How could one hope to investigate these spontaneous and unpredictable thoughts that crop up when people stop paying attention to their surroundings and the task at hand? Thoughts that couldn’t be linked to any measurable outward behavior?
But Smallwood, now at Queen’s University in Ontario, Canada, forged ahead. He used as his tool a downright tedious computer task that was intended to reproduce the kinds of lapses of attention that cause us to pour milk into someone’s cup when they asked for black coffee. And he started out by asking study participants a few basic questions to gain insight into when and why minds tend to wander, and what subjects they tend to wander toward. After a while, he began to scan participants’ brains as well, to catch a glimpse of what was going on in there during mind-wandering.
Smallwood learned that unhappy minds tend to wander in the past, while happy minds often ponder the future . He also became convinced that wandering among our memories is crucial to help prepare us for what is yet to come. Though some kinds of mind-wandering — such as dwelling on problems that can’t be fixed — may be associated with depression , Smallwood now believes mind-wandering is rarely a waste of time. It is merely our brain trying to get a bit of work done when it is under the impression that there isn’t much else going on.
Smallwood, who coauthored an influential 2015 overview of mind-wandering research in the Annual Review of Psychology, is the first to admit that many questions remain to be answered.
This conversation has been edited for length and clarity.
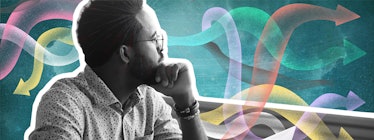
There are good reasons for your mind to wander.
Is mind-wandering the same thing as daydreaming, or would you say those are different?
I think it’s a similar process used in a different context. When you’re on holiday, and you’ve got lots of free time, you might say you’re daydreaming about what you’d like to do next. But when you’re under pressure to perform, you’d experience the same thoughts as mind-wandering.
I think it is more helpful to talk about the underlying processes: spontaneous thought, or the decoupling of attention from perception, which is what happens when our thoughts separate from our perception of the environment. Both these processes take place during mind-wandering and daydreaming.
It often takes us a while to catch ourselves mind-wandering. How can you catch it to study it in other people?
In the beginning, we gave people experimental tasks that were really boring, so that mind-wandering would happen a lot. We would just ask from time to time, “Are you mind-wandering?” while recording the brain’s activity in an fMRI scanner.
But what I’ve realized, after doing studies like that for a long time, is that if we want to know how thinking works in the real world, where people are doing things like watching TV or going for a run, most of the data we have are never going to tell us very much.
So we are now trying to study these situations . And instead of doing experiments where we just ask, “Are you mind-wandering?” we are now asking people a lot of different questions, like: “Are your thoughts detailed? Are they positive? Are they distracting you?”
How and why did you decide to study mind-wandering?
I started studying mind-wandering at the start of my career when I was young and naive.
I didn’t really understand at the time why nobody was studying it. Psychology was focused on measurable, outward behavior then. I thought to myself: That’s not what I want to understand about my thoughts. What I want to know is: Why do they come, where do they come from, and why do they persist even if they interfere with attention to the here and now?
Around the same time, brain imaging techniques were developing, and they were telling neuroscientists that something happens in the brain even when it isn’t occupied with a behavioral task. Large regions of the brain, now called the default mode network , did the opposite: If you gave people a task, the activity in these areas went down.
When scientists made this link between brain activity and mind-wandering, it became fashionable. I’ve been very lucky, because I hadn’t anticipated any of that when I started my Ph.D., at the University of Strathclyde in Glasgow. But I’ve seen it all pan out.
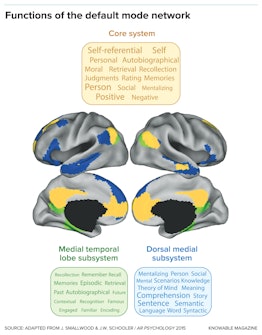
Different subsystems are responsible for different areas of cognition.
Would you say, then, that mind-wandering is the default mode for our brains?
It turns out to be more complicated than that. Initially, researchers were very sure that the default mode network rarely increased its activity during tasks. But these tasks were all externally focused — they involved doing something in the outside world. When researchers later asked people to do a task that doesn’t require them to interact with their environment — like think about the future — that activated the default mode network as well.
More recently, we have identified much simpler tasks that also activate the default mode network. If you let people watch a series of shapes like triangles or squares on a screen, and every so often you surprise them and ask something — like, “In the last trial, which side was the triangle on?”— regions within the default mode network increase activity when they’re making that decision . That’s a challenging observation if you think the default mode network is just a mind-wandering system.
But what both situations have in common is the person is using information from memory. I now think the default mode network is necessary for any thinking based on information from memory — and that includes mind-wandering.
Would it be possible to demonstrate that this is indeed the case?
In a recent study, instead of asking people whether they were paying attention, we went one step further . People were in a scanner reading short factual sentences on a screen. Occasionally, we’d show them a prompt that said, “Remember,” followed by an item from a list of things from their past that they’d provided earlier. So then, instead of reading, they’d remember the thing we showed them. We could cause them to remember.
What we find is that the brain scans in this experiment look remarkably similar to mind-wandering. That is important: It gives us more control over the pattern of thinking than when it occurs spontaneously, like in naturally occurring mind-wandering. Of course, that is a weakness as well, because it’s not spontaneous. But we’ve already done lots of spontaneous studies.
When we make people remember things from the list, we recapitulate quite a lot of what we saw in spontaneous mind-wandering. This suggests that at least some of the activity we see when minds wander is indeed associated with the retrieval of memories. We now think the decoupling between attention and perception happens because people are remembering.
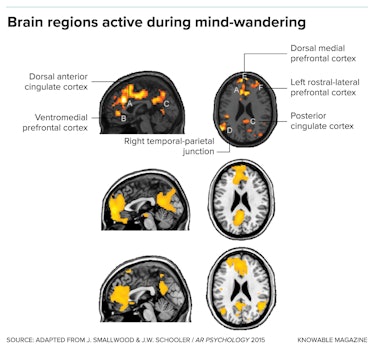
The science of mind wandering is visible in brain scans.
Nowadays, it seems that many of the idle moments in which our minds would previously have wandered are now spent scrolling our phones. How do you think that might change how our brain functions?
The interesting thing about social media and mind-wandering, I think, is that they may have similar motivations. Mind-wandering is very social. In our studies , we’re locking people in small booths and making them do these tasks and they keep coming out and saying, “I’m thinking about my friends.” That’s telling us that keeping up with others is very important to people.
Social groups are so important to us as a species that we spend most of our time trying to anticipate what others are going to do, and I think social media is filling part of the gap that mind-wandering is trying to fill. It’s like mainlining social information: You can try to imagine what your friend is doing, or you can just find out online. Though, of course, there is an important difference: When you’re mind-wandering, you’re ordering your own thoughts. Scrolling social media is more passive.
Could there be a way for us to suppress mind-wandering in situations where it might be dangerous?
Mind-wandering can be a benefit and a curse, but I wouldn’t be confident that we know yet when it would be a good idea to stop it. In our studies at the moment, we are trying to map how people think across a range of different types of tasks. We hope this approach will help us identify when mind-wandering is likely to be useful or not — and when we should try to control it and when we shouldn’t.
For example, in our studies, people who are more intelligent don’t mind wandering so often when the task is hard but can do it more when tasks are easy . It is possible that they are using idle time when the external world is not demanding their attention to think about other important matters. This highlights the uncertainty about whether mind wandering is always a bad thing because this sort of result implies it is likely to be useful under some circumstances.
This map — of how people think in different situations — has become very important in our research. This is the work I’m going to focus on now, probably for the rest of my career.
This article originally appeared in Knowable Magazine , an independent journalistic endeavor from Annual Reviews. Sign up for the newsletter .
This article was originally published on Sep. 10, 2022
- Mental Health
Five mind-blowing facts - New York Central passenger trains
Mind-blowing facts - new york central passenger trains.
We are 50-plus years into the Amtrak era, which began on May 1, 1971. A few Amtrak trains still carry the identity of the conveyances they imitate - California Zephyr, Empire Builder, and Crescent - to mention a few. What lives on today is a contemporary train - traveling a route similar to the original, but not quite - that is a shadow of their forebears. When it comes to American passenger trains, names like the Super Chief, City of New Orleans, or Capitol Limited evoke a hushed reverence and the legend of exceptional service, comfortable accommodations, fine food, and a schedule well maintained.
If these few name trains are the stuff of legend, then the New York Central, its Great Steel Fleet, and trains like the 20th Century Limited are the stuff legends are made of. Many have argued that the 20th Century Limited was the finest passenger train on American rails. Argue how you like and long for the days of yore, but either way come along now for a glimpse behind the legendary standard passenger trains of the New York Central. There is more to the story than meets the eye across all the years. These are five-mind blowing facts about the passenger trains of the New York Central.
No. 1 - The Century by the numbers
The New York Central's top passenger train was the 20th Century Limited . It was an all-Pullman, extra-fare, luxury liner running between New York and Chicago on a 15-hour schedule in 1948. An early-1930 advertisement by the NYC stated that: "The 20th Century Limited long since ceased to be a ‘train.' It is a daily fleet of trains." In the 12 months prior to the ad's appearance, the NYC had dispatched 2,153 trains carrying the 20th Century Limited name. That works out to almost six sections of the train daily. The record day came in January 1929 when seven Century sections traveled eastbound from Chicago to New York carrying 822 passenger.
Each Century section required a crew of nearly 70, which is a ratio of one crew member for every 1.75 passengers. Included among the staff were sleeping car porters, dining car waiters, stewards, cooks, maids, barbers, manicurists, valets, and secretaries.
Equipment-wise, the NYC kept 24 locomotives and 122 identical luxury sleeping cars on standby to meet demand and or mitigate negative situations impacting the Century's schedule. The schedule, by the way, was guaranteed to the point that the NYC refunded each passenger $1 for each hour the train was late. Also, the 122 standby sleepers, as with all cars assigned to the Century, had to be turned so that passenger room windows faced the waters of the Hudson River going into or coming out of New York. The NYC paid attention to the smallest detail so passengers would have the best travel experience.
No. 2 - The Wolverine and My Old School
Grab those stereophonic headphones, it's time for a trip back to 1973 …
I remember the 35 sweet goodbyes
When you put me on The Wolverine up to Annandale
It was still September
When your daddy was quite surprised
To find you with the working girls in the county jail
I was smoking with the boys upstairs when I
Heard about the whole affair, I said oh no
William and Mary won’t do …
Dial the volume down from 10 and let's interpret what you just heard. This is the first verse of the song My Old School from the group Steely Dan's 1973 album Countdown to Ecstasy. Band leaders Donald Fagen and Walter Becker tell the story of getting caught up in a pot bust at their old school - Bard College - in Annandale-on-Hudson, N.Y. The incident happened in May 1969, a time when "long hairs," like Fagen and Becker, were targeted for their behavior - real or imagined.
The Wolverine was a New York Central train running from New York to Chicago, and was popular transportation for Bard students. The train ran an interesting route, ducking into Canada after Buffalo, N.Y., and returning to the U.S. at Detroit, before a final sprint to the Windy City. It carried both sleeper and coach accommodations.
The time visited in My Old School was a time when The Wolverine, like many passenger trains, was in decline. Starting in 1957, the train lost its observation car. By December 1967, The Wolverine was no longer a named train, merely appearing as Nos. 17/8. During the Penn Central period, the train remained numbered only - westbound, Nos. 61/17. Eastbound, No. 14, was truncated at Buffalo, with passengers needing to change trains at 2:30 a.m. in order to reach New York.
Interestingly in all of this, The Wolverine never stopped in Annandale-on-Hudson. The closest NYC station to Bard College was 8 miles away in Rhinecliff, N.Y. Finally, today's Amtrak Wolverine, running between Chicago and Pontiac, Mich., via Detroit, is a direct descendant of the original NYC train … although neither of them would actually get you to my old school.
No. 3 - Would you like butter with that?
The 20th Century Limited epitomized the concept of a passenger train being akin to the finest hotel or steamship, but one that glides along steel rails rather than remaining perched on a foundation or cresting the next wave. During the Century's heyday, its two arch-competitors could be found in the Pennsylvania Railroad's Broadway Limited, and the Baltimore & Ohio Railroad's Capitol Limited , both of which also ran a New York-Chicago route. It has been reported, that all else being equal in the mind of the passenger, the choice of which train to take sometimes came down to the dining car the passenger preferred.
If a passenger liked butter, the 20th Century Limited was the way to go. Beginning with the Century's inauguration in 1902, Dr. William Seward Webb, a Vanderbilt relation and president of the Lake Shore & Michigan Southern Railroad over whose tracks the Century ran west of Buffalo, N.Y., became the official source for the train's butter provision. Webb owned a hobby agricultural venture - Shelburne Farm, located on the shores of Lake Champlain in Vermont. For a hobby farm, the operation was stunning, featuring well-maintained, prized herd of purebred Hereford cattle. From here, Webb produced the luscious creamy butter served aboard the Century.
For each passenger, the Century carried one pound of butter. The butter was served at table in the dining car and also used in the kitchen for cooking. However, whether traveling New York to Chicago or Chicago to New York, a pound of butter was allotted for each passenger. " … the diners of the Century may will have rolled on butter in their journal boxes," commented noted author and photographer Lucius Beebe in his book on the train.
No. 4 – Beer and NYC trains don't mix
New York's Grand Central Terminal stands as an icon in the railroad world. Since opening on Feb. 2, 1913, millions have passed through its spectacular halls, beginning, or ending a long-distance journey or merely as part of a daily commute. The Terminal's construction solved a significant neighborhood problem, by putting the trains underground and converting Park Avenue, running north of the station, into a boulevard befitting the posh uptown neighborhood the area was becoming.
Constructing Grand Central's two levels of track required massive excavations along Park Avenue. From 42nd to 50th street 1.6 million yards of rock were drilled and removed along with 1.2 million cubic yards of earth. More than 200 buildings were razed as the terminal site expanded from 23 to 48 acres.
One of the many construction challenges that arose appeared in the two-block area bounded by Lexington and Park avenues and 50th and 52nd streets. Roughly a third of these two blocks were occupied by two schools and church, another third by businesses and apartments. The last third, and the largest single landholder was the F.&M. Schaefer Brewing Co. The brewery fronted Park Avenue and for many years enjoyed having a street-level siding. With the tracks being moved below street level, the brewery's siding was gone. More disturbing, however, was that the drilling vibrations and the close proximity of the railroad excavations to the brewery threatened underground lagering cellars.
Despite the setbacks and danger, The F.&M. Schaefer Brewing Co. did not protest the planned expansion. On Nov. 22, 1902, according to the New York Times, former city controller Ashbel P. Fitch, representing the brewery, stated, " [F.&M. Schaefer Brewing Co. will] not stand in the way of any great public improvement, and that although they had already suffered by the closing of streets, they were willing to suffer more for the public good."
Ultimately, Schaefer Brewing closed the Manhattan plant, sold the incredibly valuable land, and built a new brewery in Brooklyn.
No. 5 - Late to its own funeral
Into the 1950s, the 20th Century Limited, like other passenger trains was in decline. The allure of faster jetliners and the freedom of the automobile had taken its toll. The last run of the Century was announced for Dec. 2, 1967. The announcement really wasn't a big announcement, but rather a whisper in an attempt to reduce the fanfare around the great train's demise.
Only half full, the Century departed from Grand Central Terminal track 34 at 6 p.m. The fanfare was minimal: a group of railfans gathered on the famous red carpet, taking photos of observation-lounge Wingate Brook, which brought up the rear of the train. Beyond that was the press, taking photos of the railfans. Aboard the train, despite the crew putting on a good face, the equipment was worn, and it was clearly the final trip.
In the dark of night, west of Harbor Creek, Pa., another train had derailed, blocking the Century's path. The train sat still for hours until it was detoured over the Norfolk & Western. In the end, the last run of the 20th Century Limited limped into Chicago around 6:45 p.m. - 9 hours, 50 minutes overdue - late to its own funeral.

IMAGES
VIDEO
COMMENTS
Mind-wandering has been linked to crucial parts of our daily lives, including learning, affect, and job productivity. While a number of studies have examined mind-wandering rates in everyday life, an implicit assumption has been that the rate is constant over the course of the day. The current research provides the first evidence suggesting ...
The debate about mind-wandering. On the depressing side of the debate, Matt Killingsworth's Track Your Happiness project concluded that mind-wandering makes us unhappy. His data showed that our ...
New research led by UC Berkeley has come up with a way to track the flow of our internal thought processes and signal whether our minds are focused, fixated or wandering. Using an electroencephalogram (EEG) to measure brain activity while people performed mundane attention tasks, researchers identified brain signals that reveal when the mind is ...
Mind wandering (MW) and mindfulness have both been reported to be vital moderators of psychological wellbeing. Here, we aim to examine how closely associated these phenomena are and evaluate the ...
Mind wandering, most commonly defined as a shift of attention from a task at hand to task-unrelated thoughts (Smallwood & Schooler, 2006), is a fairly common phenomenon estimated to occupy up to 45% of our daily mental activity (Killingsworth & Gilbert, 2010).This high prevalence of mind wandering has a direct impact on the functioning of cognitive processes.
Mind-wandering—defined as off-task thinking—can be disruptive to daily functioning. Mindfulness is considered a potential method for reducing mind-wandering; however, no study has systematically reviewed findings on this topic. The present systematic review synthesizes current findings from this literature, examining whether results vary as a function of study methodology. Our final sample ...
Mind-wandering is often defined as task-unrelated or stimulus-unrelated thought. In this Review, Christoff and colleagues present a definition for mind-wandering that places more emphasis on the ...
The existence of diurnal fluctuations in the case of mind-wandering, however, has not been examined to date. We did so in two studies. In the first study, we employed everyday experience sampling to obtain self-reports from 146 university students who rated the degree of free movement in their thoughts multiple times per day over five days ...
Mind-wandering (MW) as a research topic has received considerable attention over the last several decades. The recent differentiation between spontaneous and deliberate MW has suggested a particular effect of the former on psychopathology; in that increased spontaneous MW may precede mental illness. The present study sought to explore MW as a ...
But, still, mind-wandering is a constant. Overall, people mind-wander 47% of the time. Perhaps not such a good thing if it relates to unhappiness, To hear more about mind-wandering — and about the importance of studying happiness in general — watch Killingsworth's talk. And after the jump, read several more fascinating studies on the ...
Introduction. An extensive empirical literature has demonstrated that adults mind-wander as much as 30-50% of their waking lives, often at considerable expense to ongoing performance and quality of life (Kane et al., 2007; Killingsworth and Gilbert, 2010; Schooler et al., 2011).Mind-wandering is characteristically described as the interruption of task-focus by task-unrelated thought (TUT ...
Mind-wandering (MW) is a universal human phenomenon and revealing its nature contributes to understanding consciousness. The ecological momentary assessment (EMA), in which subjects report a ...
Conscious experience is fluid; it rarely remains on one topic for an extended period without deviation. Its dynamic nature is illustrated by the experience of mind wandering, in which attention switches from a current task to unrelated thoughts and feelings. Studies exploring the phenomenology of mind wandering highlight the importance of its content and relation to meta-cognition in ...
Mind-wandering refers to moments when this inward focus diverts attention away from the current task-at-hand. Mind-wandering is thought to be ubiquitous, having been estimated to occur between 30% and 50% of our waking moments. Yet, it is unclear whether this frequency is similar within-task performance contexts and unknown whether mind ...
Mind Wandering and Other Lapses. J. Smallwood, in Encyclopedia of Consciousness, 2009 Mind wandering is a universal human experience in which the focus of attention temporarily shifts from what we are doing. This article describes how to conceptualize these shifts in attention as changes in the flow of information through an attentional system and considers the different explanations offered ...
1. Introduction. The human mind has a remarkable capacity to disconnect from the immediate environment and wander off to another time or place. Often characterized as thoughts minimally constrained by the ongoing task or external environment, this phenomenon of mind wandering can occupy a notable portion of our awake mental life.
Scientists finally know why we get distracted — and how we can stay on track. More than just a distraction, mind-wandering (and its cousin, daydreaming) may help us prepare for the future. by ...
University students watched a video lecture on statistics, during which they were periodically probed to report their thought content, which could either be on-task (thoughts about what was being discussed in the video at that time), lecture-related mind wandering (e.g., thoughts on some aspect of the topic but not what was being presented in ...
Mind-blowing facts — New York Central passenger trains We are 50-plus years into the Amtrak era, which began on May 1, 1971. A few Amtrak trains still carry the identity of the conveyances they ...