Big data in tourism marketing: past research and future opportunities
Spanish Journal of Marketing - ESIC
ISSN : 2444-9695
Article publication date: 9 January 2023
The purpose of this study was to uncover representative emergent areas and to examine the research area of marketing, tourism and big data (BD) to assess how these thematic areas have developed over a 27-year time period from 1996 to 2022. This study analyzed 1,152 studies to identify the principal thematic areas and emergent topics, principal theories used, predominant forms of analysis and the most productive authors in terms of research.

Design/methodology/approach
The articles for this research were all selected from the Web of Science database. A systematic and quantitative literature review was performed. This study used SciMAT software to extract indicators. Specifically, this study analyzed productivity and produced a science map.
The findings suggest that interest in this area has increased gradually. The outputs also reveal the innovative effort of industry in new technologies for developing models for tourism marketing. Ten research areas were identified: “destination marketing,” “mobility patterns,” “co-creation,” “gastronomy,” “sustainability,” “tourist behavior,” “market segmentation,” “artificial neural networks,” “pricing” and “tourist satisfaction.”
Originality/value
This work is unique in proposing an agenda for future research into tourism marketing research with new technologies such as BD and artificial intelligence techniques. In addition, the results presented here fill the current gap in the research since while there have been literature reviews covering tourism with BD or marketing, these areas have not been studied as a whole.
El objetivo de esta investigación fue descubrir nichos representativos de áreas emergentes y examinar el área de Marketing, Turismo y Big Data, evaluando cómo han evolucionado estas áreas temáticas durante un período de 27 años desde 1996–2022. Analizamos 1.152 investigaciones para identificar las principales áreas temáticas y temas emergentes, las principales teorías utilizadas, las formas de análisis predominantes y los autores más productivos en términos de investigación.
Metodología
Todos los artículos para esta investigación fueron seleccionados de la base de datos Web of Science. Realizamos una revisión sistemática y cuantitativa de la literatura. Utilizamos el software SciMAT para extraer indicadores. Específicamente, analizamos la productividad y elaboramos un mapeo científico.
Los hallazgos sugieren que el interés en esta área ha aumentado gradualmente. Los resultados también revelan el esfuerzo innovador de la industria en nuevas tecnologías para desarrollar modelos de marketing turístico. Se identificaron diez áreas de investigación (“marketing de destinos”, “patrones de movilidad”, “co-creación”, “gastronomía”, “sostenibilidad”, “comportamiento turístico”, “segmentación de mercado”, “redes neuronales artificiales”, “precios”, y “satisfacción del turista”).
Este trabajo es único al proponer una agenda para futuras investigaciones en investigación de Marketing Turístico con nuevas tecnologías como Big Data y técnicas de Inteligencia Artificial. Además, los resultados presentados aquí llenan el vacío actual en la investigación ya que si bien se han realizado revisiones de literatura que cubren Turismo con Big Data o Marketing, estas áreas no se han estudiado como un conjunto.
这一特定研究领域的目标是发现具有代表性的新兴领域, 并考察市场营销、旅游和大数据研究领域, 以评估这些主题领域在1996年至2022年的27年间是如何发展的。我们分析了1152项研究, 以确定主要专题领域和新兴主题、使用的主要理论、主要的分析形式以及在研究方面最有成效的作者。
本研究的文章都是从Web of Science数据库中选出的。我们进行了系统化的定量文献审查, 并使用SciMAT软件来提取指标。具体来说, 我们分析了生产力并制作了一个科学研究地图。
研究结果表明, 人们对这一领域的兴趣已经逐渐增加。本文也揭示了工业界在开发旅游营销模式的新技术方面的创新努力。研究确定了十个研究领域:“目的地营销”、“流动模式”、“共同创造”、“美食”、“可持续性”、“游客行为”、“市场细分”、“人工神经网络”、“定价 “和游客满意度”。
这项研究的独特之处在于提出了未来利用大数据和人工智能技术等新技术进行旅游营销研究的议程。此外, 本文的结果填补了目前的研究空白, 因为虽然有文献综述涉及旅游与大数据或市场营销, 但这些领域还没有被作为一个整体来研究。
- Tourism marketing
- Literature review
- Science mapping analysis
- Future research agenda
- Palabras Big data
- Marketing turístico
- Revisión de la literatura
- Análisis de mapeo científico
- Agenda de investigación futura
Blanco-Moreno, S. , González-Fernández, A.M. and Muñoz-Gallego, P.A. (2023), "Big data in tourism marketing: past research and future opportunities", Spanish Journal of Marketing - ESIC , Vol. ahead-of-print No. ahead-of-print. https://doi.org/10.1108/SJME-06-2022-0134
Emerald Publishing Limited
Copyright © 2022, Sofía Blanco-Moreno, Ana M. González-Fernández and Pablo Antonio Muñoz-Gallego.
Published in Spanish Journal of Marketing – ESIC . Published by Emerald Publishing Limited. This article is published under the Creative Commons Attribution (CC BY 4.0) licence. Anyone may reproduce, distribute, translate and create derivative works of this article (for both commercial and non-commercial purposes), subject to full attribution to the original publication and authors. The full terms of this licence maybe seen at http://creativecommons.org/ licences/by/4.0/legalcode
1. Introduction
The field of tourism research is one of the most long-established areas, with more than 175,000 publications listed on the Web of Science (WoS) from 1940 until 2022 ( Kontogianni and Alepis, 2020 ).
Unfortunately, researchers have not always been in possession of sufficiently advanced tools and techniques to process all this information. However, thanks to big data (BD), grounded in facilities for the massive storage of quality structured data, this issue is starting to be resolved.
BD and its tools have changed the ways in which we can analyze and process information. However, there is currently no literature giving a thorough overview of how BD techniques have been used in tourism marketing over the past 27 years of its existence.
In the past decade, several authors have undertaken bibliometric analyses of tourism research literature. Work has concentrated on three key areas in isolation: tourism ( Hall, 2011 ; Köseoglu et al. , 2015 , 2016 ), BD in tourism ( Li et al. , 2018 ; Mariani and Baggio, 2021 ; Samara et al. , 2020 ) and tourism experience ( Kim and So, 2022 ). To our knowledge, no bibliometric analyses exist dealing with BD, tourism and marketing. Such a study has great value enabling researchers to gain an understanding of how key areas of study have evolved over time.
Compared to existing literature reviews on the topic “BD and tourism,” our work is distinctive in three ways. First, while the two previous literature reviews have focused only on BD and tourism, this study performs queries related explicitly to BD, tourism and marketing. We feel that the inclusion of marketing is essential as there is currently a lack of research into the practical applications of BD in tourism product design and marketing.
Second, while previous work has reviewed articles published between 2007 and 2020, we have extended the time span of interest to include all articles published, from 1996 to 2022. In this way, we cover not only the inception of this field but also its most recent evolution, including the two-year period of the COVID-19 crisis.
Third, unlike the present study, none of the previous review articles mentioned application of the bibliometric techniques of productivity analysis and science mapping.
The aim of this study then is to fill the gap identified in the current literature by completing possibly the first exhaustive bibliometric analysis of research output in the combined areas of BD, tourism and marketing.
The scientific database, the WoS, was selected for our analysis of trends and prediction of future research paths in this field. The analysis itself was completed through a complete indexation of articles found and the use of the bibliometric research tool SciMAT (Science Mapping Analysis Tool).
To uncover specific research niches representing emergent areas in the tourism marketing field.
To analyze the body of research in terms of principal authors, volume of publications and most productive categories.
To help academics and professionals gain a better understanding using a schema showing the evolution between 1996 and 2022.
To identify the key thematic areas that have drawn most research interest during the past 27 years.
We believe that one major contribution of this bibliometric analysis is the identification of 10 key themes in the past 27 years of BD research in tourism marketing. Furthermore, this study offers researchers useful information concerning the significance of BD in the development of tourism marketing strategies, both in the present and the future, and it highlights the emerging tendencies on which future investigation should be focused.
Our study begins with an overview of the evolution of BD in tourism marketing and goes on to explain our methods of bibliometric analysis, before giving a detailed explanation of the results of our empirical analysis and future research trends. We conclude with a description of the study’s limitations and its implications for the future.
2. The evolution of big data in tourism marketing
BD first emerged in 1989 with the birth of the World Wide Web. The term refers to the massive volumes of data produced online that are processed at high velocity, have a high level of veracity and comprise huge variety being both complex and diverse.
In the area of tourism, BD enables consumer profiling to create personalized services and make forecasts. Furthermore, recent research shows a clear tendency toward its use in the field of sustainable tourism; thus it has become an essential element in the United Nations plans to achieve its Sustainable Development Goals.
The use of BD in tourism marketing strategies can be explained through the classical resource-based view theory. This arises naturally from the fact that the use of BD requires physical resources such as sufficiently powerful computers; human resources, such as data scientists; and, because it is essential that organizations and corporate processes should be able to adapt to new technologies, intellectual resources like organizational capital.
The three major sources of BD for the tourism industry are as follows ( Li et al. , 2018 ): user data or user-generated content (UGC) like text and photos; device data, including that from the global positioning system (GPS) or Bluetooth; and transaction data such as Web searches and online bookings among others.
In the area of tourism marketing, research is dominated by studies that use online ratings and reviews to measure tourist satisfaction. Indeed, there are numerous studies concerning how hotels use electronic word of mouth (eWOM) due to the importance of this phenomenon in attracting tourists.
Furthermore, while the analysis of textual data is still important, photos are beginning to acquire prominence thanks to the development of web 2.0 and social networking platforms such as Instagram, Pinterest, Flickr and Facebook. These data have a diversity of uses, for example, to analyze the attitudes of tourists toward a particular destination, as well as tourist behavior, given that a photo greatly simplifies the process by which travelers can communicate their tourist experiences online. In this way, industry specialists can make recommendations to potential clients, and design marketing strategies to promote particular services or tourist destinations.
3. Research design and data collection
To gain an understanding of the themes of BD, tourism and marketing, we performed a bibliometric analysis of academic articles indexed in one of the most important academic databases: WoS. Bibliometric analysis was used as it has several advantages and enables the evaluation of academic research according to objective criteria. It is used as a tool, and it facilitates the identification of new lines of research.
Because the aim of our bibliometric analysis was to evaluate key themes explored by researchers, and identify thematic clusters, it was vital to have a holistic overview of the BD, tourism and marketing themes. We selected WoS over other sources for three reasons.
First and foremost, even though WoS and Scopus are the two most commonly used sources for bibliometric analysis, the WoS database is the only large-scale literature database from as early as 1940 ( Calof et al. , 2022 ) and also contains articles from journals identified as having the highest impact factor according to the Journal Citation Report index. Second, WoS, compared to Scopus, has the advantage of having its own tourism category. Third, and finally, the WoS database is the most frequently used source of scientific information ( Kim and So, 2022 ).
Several search criteria were deployed to retrieve the articles. In line with Mariani and Baggio (2021) we developed multiple search queries entailing a combination of the focal keywords “big data,” “artificial intelligence,” “machine learning,” “marketing” and “consumer behavior,” with hospitality and tourism words “travel*,” “touris*” and “hotel” in the text, abstract and keywords.
As the data used for this study was collected between 1996 and 2022, the search was conducted from the beginning of the coverage up to March 31, 2022. We eliminated articles which were not directly related to the topic of the analysis. The final data set used for the analyses contains 1,152 papers for WoS.
To execute the bibliometric analysis, our sample of articles was grouped into four time periods, each addressing a particular era in the evolution of research into BD techniques, tourism and information technology ( Xiang, 2018 ).
The first time period (1996–2006) corresponds to a phase of explosion and digitalization of information. It is composed of 12 papers and 147 keywords. The second time period (2007–2016) corresponds to a phase of acceleration in the use, storage and processing of massive digital data. It is composed of 112 papers and 387 keywords. The third period (2017–2020) constitutes the most recent phase in which this type of data and its associated technologies are established, and the research field has matured. It is composed of 426 papers and 811 keywords. The last period (2020–2022) corresponds to the two years of the COVID-19 pandemic. It is composed of 602 papers and 578 keywords.
The research methodologies used in this work are in line with the other well-known principles used in bibliometric analyses and quantitative literature reviews ( Cobo et al. , 2012 ; Tranfield et al. , 2003 ) ( Figure 1 ).
4. Method: bibliometric analysis using SciMAT
There are two principal methods of bibliometric analysis: productivity analysis , which evaluates the impact of academic research, and science mapping which enables the visualization of the structure and evolution of concepts within an academic field. This investigation combines both types of analysis to present the most important conceptual domains.
The first stage of our investigation involved a retrieval of publications related to BD, tourism and marketing on the WoS database.
Following this, the search was revised for possible errors, and the relevant documents were extracted to begin constructing our thematic network, in this instance using keywords ( Cobo et al. , 2012 ). We then constructed a word-network based on keyword co-occurrence, that is, when words appear together in a document this implies a relationship ( Cobo et al. , 2011a ).
The next step was relationship network normalization via the equivalence index, with the aim of calculating the degree of similarity between keywords. This is deemed to be the most appropriate way to normalize co-occurrence frequencies ( Cobo et al. , 2011b ).
After the normalization process, a science map was constructed to show the knowledge structure of this research area through its key concepts. The present study used an analysis of co-words in a longitudinal framework ( Cobo et al. , 2011a ). A clustering algorithm was applied to the networks of co-words generated for each of our selected time periods, to identify the most significant word in each cluster.
The visualization techniques available in SciMAT enable the representation of the science map with the evolution of thematic areas, through a diagram that allows the representation of two Callon’s centrality and Callon’s density ( Cobo et al. , 2011b ).
Callon’s centrality measures the degree of interaction between one network and other networks. It is defined as: c = 10 × ∑ e kh , where k refers to a keyword belonging to a theme in one network, and h refers to a keyword belonging to themes in other networks. Callon’s density measures the internal strength of the network and is defined as: d = 100 ( ∑ e ij / ω ), where i and j are keywords belonging to a given theme, and ω is the number of keywords in that theme. Two measures can represent the detected networks. On the strategic diagram, centrality and density are represented on the horizontal and vertical axes, respectively ( Figure 2 ). In this way, the diagram is divided into four categories:
Driving themes (upper right quadrant): those that are very interrelated, developed in great depth and highly relevant.
Underlying and transversal themes (lower right quadrant): important general themes in the research field but which are less well developed.
Emerging themes or those in decline (lower left quadrant): under-developed topics.
Specialized or peripheral themes (upper left quadrant): marginal themes having little relevance to the research field as a whole.
The last step is the productivity analysis which incorporates indicators such as the citation number, and the h and g indices. It enables an understanding of which topics are most productive and have the greatest impact.
5. Mapping the co-word analysis
5.1 productivity analysis and science mapping.
BD has made a significant impact in the field of tourism marketing research. Since 2017, the number of academic articles published in this area has seen a fivefold increase. More than 89% of the articles were published in the past six years.
Of the 446 journals included in the database, only 6% are directly related to tourism marketing, that is to say, 26 journals containing 71 articles.
The majority of the articles are not published in tourism marketing journals but are distributed across a variety of journals focusing on other disciplines such as management, sustainability and technology. The category of Hospitality, Leisure, Sport and Tourism itself contains 476 articles and Tourism Management is the second most productive category, with 224 articles published in this area. Finally, the most productive authors are Rob Law (School of Hotel and Tourism Management, Hong Kong) and Zheng Xiang (Virginia Tech, Beijing Union University).
Certain themes have established their intrinsic importance throughout the 27 years studied here and we will discuss their development in what follows (see Table 1 ).
5.2 First period: digitalization of information (1996–2006)
Only 12 relevant articles appear in this 11-year period ( Figure 2a ).
5.2.1 Driving themes: “website,” “photographs,” “performance,” “online reviews” and “tourism patterns.”
The most highly related and most relevant driving themes are “website” and “online reviews” ( Cobo et al. , 2011a ).
The “website” cluster demonstrates the growing importance of three areas of research: traveler experiences recorded on blogs and Facebook; consumer perspectives on the personalization of products and services; and smart cities in Asia via the Internet of Things. The “online reviews” topic is connected with sentiment analysis for segmenting the international tourist market.
“Performance” and “tourism patterns” are concerned with forecasting in the tourism sector which studies segmentation strategies and the results in terms of performance ( Curry et al. , 2001 ) using social networks such as Sina Weibo.
The “photographs” topic is connected with analysis of smart tourism and ecotourism, and how to segment the market through self-organizing maps. Here, investigation predominantly focuses on the tourist motivations which have the greatest weight in buying decisions in the senior-tourist market segment ( Kim et al. , 2003 ).
5.2.2 Underlying and transversal themes: “behavior” and “big data.”
Tourist behavior is the most relevant of all the themes identified. Articles belonging to this cluster focus on environmental behavior, post-buying behavior, and forecasting tourist behavior. In addition, work in this area relies on two cognitive theories: the theory of reasoned action and its extension the theory of planned behavior. These theories are considered to offer the best framework for understanding tourist behavior ( Hsu and Huang, 2012 ).
The “behavior” theme is, in turn, related to others such as loyalty, market segmentation, mobility, demand and tourism forecasting. The majority of this research strand comes from the USA.
The application of human–computer interaction theory is another important topic here. This theory establishes the fundamentals for an understanding of tourists’ behavior in terms of how they search for and plan their trips ( Xiang, 2018 ).
To understand “consumer behavior,” researchers have used BD techniques such as time series ( Pattie and Snyder, 1996 ), and lexicon and text mining or modeling ( Bloom, 2004 ), and have predicted things like loyalty, sales and tourist satisfaction.
5.2.3 Emergent themes: “neural networks” and “tourism and hospitality.”
The theme “neural networks” is associated with predicting trends in “tourism demand” through the use of BD. Specifically, it links to how BD can improve models used in econometric forecasting ( Witt and Witt, 1995 ) through the use of artificial neural networks and so enable the development of improved tourism demand models ( Palmer et al. , 2006 ). Japan, China and Spain are connected to this theme. The most common types of analysis are cluster and multiple linear regression.
5.3 Second period: acceleration (2007–2016)
The total number of articles belonging to this period is 112, so is evidence of the huge growth index for publications in this field ( Figure 2b ). Topics such as “tourist satisfaction,” “big data,” “neural networks,” “China” and “social media” achieved 5,350 citations.
5.3.1 Principal driving theme: “tourist satisfaction”.
This is the most important driving theme in the field, leading in terms of number of documents, citations and values of h and g indices. It is strongly linked to WOM as recorded in reviews left by travelers describing their experiences in hotels, and the impact of these reviews on sales is also a topic of study.
This decade is characterized as an era of acceleration due to the enormous increase in UGC on the internet. This factor, among others, has enabled the in-depth study of eWOM ( Ghose et al. , 2012 ). UGC, comprising any online data either in the form of text or images, makes up almost 50% of BD in connection with tourism ( Li et al. , 2018 ). The reason for its extensive use lies in the fact that it can be easily accessed and processed, and indeed, it is very low cost ( Karimi et al. , 2020 ).
The predominant theoretical frameworks applied in this era include sign theory, attribution theory, transaction cost theory and expectancy theory. This demonstrates the impact of reviews in the description of consumer experience.
Online reviews are one of the significant elements in eWOM which can influence future demand from other clients, and as a result, has important commercial value ( Xie et al. , 2014 ). This is due to the way it can enable forecasting of future profits for hotels, decisions concerning the location of accommodation and room rates, as well as the improvement of results based on performance ( Pan and Yang, 2017 ).
A predominant trend here is articles addressing new ways of categorizing hotels based on the mean perceived utility of specific hotel features ( Berezina et al. , 2016 ). Other important work involves identification of which sorts of messages posted on social media enabled the greatest user interaction or the possibility of virality ( Mariani et al. , 2016 ). In this respect, Facebook and Twitter stand out.
5.3.2 Driving and transversal themes: “big data” and “neural networks.”
Alongside “tourist satisfaction,” these are the other driving themes in the second period. Both these concepts are cornerstones of marketing, due to their capacity to positively influence the performance of an organization. In this way, they are very interrelated terms and, in addition, are linked to the themes “perceived quality of service” and “loyalty,” which in turn are strongly connected to “tourist satisfaction.”
A large proportion of articles addresses the theme of “performance” and analyzes which variables affect tourism-business outcomes within a competitive environment. Among the areas that have received most attention in this regard are the quality of hotel services, and hotel attributes and efficiency, in addition to the identification of factors determining tourist satisfaction and appropriate strategic decision-making ( Moutinho et al. , 2015 ). The most common types of analysis are spatial ( Supak et al. , 2015 ), cluster ( Brida et al. , 2012 ), textual ( Krawczyk and Xiang, 2016 ), time series ( Claveria and Torra, 2014 ), fuzzy system ( Shahrabi et al. , 2013 ) and photo-sharing analysis ( García-Palomares et al. , 2015 ).
5.3.3 Secondary underlying and transversal themes: “administration and management,” “destination marketing” and “social media analyses.”
These three topics constitute the underlying transversal themes of research in this second period.
“Administration and management,” which began as a driving theme moves to being a transversal theme, that is, we see its consolidation. In the course of this theme’s evolution, BD research can be seen to undergo significant development, enabling it to encompass the problems of tourism management ( Xiang, 2018 ). In addition, this topic is aligned with the evolution in tourism demand. In this area, three big powers stand out: China, the USA and Europe, specifically Spain. In fact, “Europe” moves from being an emergent theme to become integrated into an essential cluster.
The topic of “destination marketing” is linked to the study of tourism destinations and traveler motivations. Of great importance here is the use of images and websites that guide traveler management ( Xiang, 2018 ). It is a fundamental theme from the resource-based theory, because online visibility is a differentiating factor leading to superior business performance because it potentially helps attract more tourists enabling increased rates of occupancy ( Smithson et al. , 2011 ).
Finally, the “analysis of social media” appears as an underlying theme. Understanding clients through the reviews left on social media platforms such as Twitter constitutes a key factor for success in the era of BD ( Park et al. , 2016 ). The principal techniques used in this field include neural networks and data mining.
5.3.4 Emergent areas: “pricing” and “geo-tagged data.”
These two themes are considered emergent areas. In contrast to the first period, these terms are now important, and they will have importance in the following (third) time period.
The “pricing” theme shows strong links to airlines through revenue management, pricing strategies and tourist satisfaction with low-cost or full-service carriers ( Leong et al. , 2015 ).
Through the use of geographic information systems, “geo-tagged data” has enabled the use of photos obtained principally from the Flickr social media platform ( Levin et al. , 2015 ).
5.4 Third period: consolidation (2017–2020)
Over these four years, the research field has grown with 426 articles ( Figure 2c ). Over this time period, tourism research undergoes a dramatic change as BD becomes a fundamental knowledge creation tool. This transformation is without precedent in academic research, and is thanks to ever more efficient management of the millions of bytes of data generated ( Batista e Silva et al. , 2018 ).
5.4.1 Principal driving theme: “tourist satisfaction.”
This is the highest central theme in the third period and is a topic that has gained importance with respect to the previous period. Tourism literature establishes general tourist satisfaction, and indeed tourists’ intention to return to a given destination is effected by many different destination attributes ( Alegre and Garau, 2010 ). For instance, consumers gain a specific degree of satisfaction as a function of their perceptions concerning the various attributes of hotels, thus perceptions represent one dimension of satisfaction ( Guo et al. , 2017 ).
This topic is strongly related to themes in the “tourist satisfaction” cluster from the second period, such as online and offline reviews, hotels and tourist intentions. Topics such as loyalty, and hotel attributes and service quality that were previously related to perceptions are now linked with satisfaction. Furthermore, terms such as “Twitter” and “UGC” have disappeared. Research is no longer so focused on general social networks, but rather on those that are specifically concerned with tourism such as TripAdvisor.
Data from reviews and blogs are now principally used in studies of satisfaction, recommendations and tourist opinion ( Deng and Li, 2018 ).
5.4.2 Secondary driving themes: “management,” “mobility,” “trust” and “destination marketing.”
Together with “tourist satisfaction,” these are among the driving themes of the third period. “Management” is a topic of relatively high importance in all the periods studied and, in the third period is once again a driving theme.
This cluster is related to other topics such as “social networks,” “Facebook” and “engagement.” The investigations in which these terms appear focus on the strategic use of Facebook to promote and market destinations ( Mariani et al. , 2018 ); on the analysis of opinions using texts ( Zola et al. , 2019 ); and the generation of commitment ( Villamediana-Pedrosa et al. , 2019 ).
The topic of “mobility” involves examples of the use of data obtained from GPS, social media and mobile telephones used between cities, and at open-air venues hosting sporting events or festivals ( Salas-Olmedo et al. , 2018 ). The theme of “trust,” on the other hand, exemplifies the growth of concerns and problems associated with engagement in the so-called trust economy ( Xiang, 2018 ), specifically Airbnb and Booking.com. Variables such as reputation, communication and pricing strategies are found to be moderating factors in the “trust” theme.
With respect to the “destination marketing” theme, here UGC predominates, as do marketing strategies on social networks and their analysis. In this way, organizations can understand the perceptions of users and develop strategies to promote revisiting.
In all, 73% of the articles look at tourist destination image. This theme has evolved from being dominated by the destination marketers, to become a dynamic process of interaction between tourists and promotion, before finally reaching a new era in which destination management organizations examine and modify their projected destination image based principally on behavior, perceptions, experiences and the diffusion of information by tourists on social networking platforms.
“Destination marketing” is related to heritage too, as well as rural tourism in protected areas and National Parks. Two basic objectives dominate: developing branding strategies and extracting trends in this area of tourism, with sustainability and ecological protection high on the agenda. The most common type of analysis is content analysis.
5.4.3 Underlying and transversal themes: “tourism destinations” and “photographs.”
Besides tourist satisfaction, these constitute the most important underlying and transversal themes in this period. Both are related to the analysis of geo-tagged text and images obtained from social media platforms such as Facebook, Twitter, TripAdvisor and Sina Weibo.
To improve their business intelligence, “tourist destinations” are supported by tools such as customer relationship management (CRM). The surge in social networks challenges traditional notions of how to manage client relationships, and thus social-CRM has appeared on the scene ( Chan et al. , 2018 ).
In terms of size, the “social networks” cluster clearly stands out. Current literature concerning CRM focuses on the analysis of BD and the use of social networking platforms to capture huge amounts of data and take advantage of customers’ improved interactivity to personalize services ( Sota et al. , 2020 ). TripAdvisor appears as the most widely used platform in terms of marketing strategies. Another area of high research activity is applied studies concerning China and sport tourism.
“Photographs” in conjunction with “tourism destinations” constitute the underlying and transversal themes of the third period of study.
This topic is highly related to the management and promotion of hotel rooms and online bookings, as well as attempts to better understand client profiling via BD ( Liu et al. , 2019 ). Furthermore, the availability of large sets of photos from trips shared online provides an accessible source of data for tourism researchers ( Ma et al. , 2020 ). This type of content can be interpreted through semiotic theory. The principal origin of online photographic content is social media such as Twitter, Instagram and Flickr, as well as blogs. These enable study of the discovery and development of tourist routes, marketing strategies and tourism patterns, and can be differentiated into two types: concerning travelers or trips. At present, tourism research related to photos is dominated by Flickr, despite the fact that Instagram has more users and contains more images.
5.4.4 Emergent themes: “market segmentation” and “internet.”
The “internet,” understood as the tool that provides the raw data on which the techniques of BD can operate, is starting to manifest as an emergent theme in the context of tourism marketing because it enables accommodation providers to adapt, for example, room characteristics and pricing strategies.
A further area of high interest is “market segmentation,” related to recommendation systems via the “internet” cluster. Both of these themes are themselves strongly linked to co-creation which enables, among other things, the personalization of products through market segmentation using traveler preference data and geo-localized data extracted from mobile phones. The use of BD techniques to segment the tourism market, in fact, continues to be recognized as a key source of value creation in the fourth time period.
5.5 Fourth period: COVID-19 (2020–2022)
To supplement this investigation in the wake of the global COVID-19 pandemic, a further 602 articles published during the pandemic were added to our database. This additional, newly published work constitutes 50% of our database ( Figure 2d ).
5.5.1 Principal driving themes: “tourist satisfaction,” “social media,” “sharing economy,” “consumer” and “artificial intelligence.”
The theme “tourist satisfaction” continues to be the most important theme despite the COVID-19 pandemic. During these two last years studied, the number of studies dealing with BD see continued growth, particularly in reviews concerning the prediction of customer purchase preferences and its impact, and in looking at user experiences and perceptions through content analysis or making use of data gathered from platforms such as TripAdvisor. Specifically, areas being investigated include consumer behavior and social media marketing ( Nilashi et al. , 2021 ), and engagement with social exchange theory ( Song et al. , 2020 ).
The most extensively studied theme in this respect is sentiment analysis applied to text-based and photographic UGC shared on social media platforms, particularly Twitter. This analysis has allowed researchers to deepen and advance their understanding of destination marketing in the promotion of products and services.
The “sharing economy” is another theme that has gained importance in this last time period, with most research focusing on the social media site Airbnb ( Canziani and Nemati, 2021 ).
In addition, during this period, AI has become a consolidated topic with machine learning emerging as the most widely used technique to study the tourism ecosystem. Several Spanish authors specialize in the use of these techniques ( Marine-Roig and Huertas, 2020 ; Sánchez-Martín et al. , 2020 ; Valls and Roca, 2021 ) and they have been applied particularly successfully in the areas of tourism innovation and forecasting, decision-making and the analysis of performance and strategy.
5.5.2 Underlying and transversal themes: “hotel attributes” and “deep learning”.
These two themes are consolidated during the two years of the COVID-19 pandemic becoming transversal topics. In particular, “hotel attributes” have been studied in relation to competitiveness, rating and the effect they have on WOM. The forms of data gathering most widely used include text and data mining which enable the analysis of language and emotions through text. “Deep learning” is another important tool as it facilitates visual analysis, the prediction of occupancy and opinion classification ( Gómez et al. , 2021 ), all of which help tourism managers to develop and promote appropriate response strategies informed by service management theory ( Zhu et al. , 2021 ). In this area, China appears to be the most visible.
5.5.3 Emergent themes: “sustainability,” “tourist recommendation,” “social media analysis,” “values,” “prices” and “gastronomy.”
The bibliometric analysis undertaken has allowed us to identify the emergent themes that are likely to become increasingly important in the future.
Sustainability. The number of studies concerning profitability and perceptions in ecotourism is growing exponentially. The principal sources of data for this work are Google data and geo-tagged photographs. Analyzing trends in ecotourism is part of a strategic approach to assessing progress toward the UN’s Sustainable Development Goals ( Go et al. , 2020 ).
Tourist recommendation. An emergent theme in the third time period, market segmentation continues to be important in this time period, and as before, it is driven by tourist recommendation. Researchers continue to use BD to analyses tourist recommendations, and additionally we see this source of data being applied to new variables such as types of tourism, length of stay, attachment and quality of service ( Penagos-Londoño et al. , 2021 ).
Social media analysis. A particular use of this type of analysis is to look at revisit intentions in hospitality. This concept is integral to the relationship between marketing and customer loyalty, and has traditionally been investigated largely through customer surveys using closed-ended questions ( Liu and Beldona, 2021 ). Currently, there is an exponential growth in revisit intention analysis, particularly to look at decision making in hotel management, with researchers now turning to supervised machine learning rather than using social media analysis.
Values. Little is known about the influence of cultural factors in consumers’ evaluations of review helpfulness, and as a result, research into values, particularly using the theory of dominant logic, must be categorized as an emergent theme ( Filieri and Mariani, 2021 ).
Prices. Researchers are beginning to apply BD techniques to understanding how differences in market perception and information create a price differential ( Casamatta et al. , 2022 ). Until now, setting the price for new accommodation has been often based largely on location, number of beds and type of house, among other physical factors. However, the use of machine learning and intention analysis is beginning to take over as the means for price prediction in online booking systems ( Trang et al. , 2021 ).
Gastronomy. In the third time period studied, there were only three articles considering this topic and thus, it was considered isolated and highly specialized. In the fourth time period, however, we identified 14 articles concerning gastronomy, and thanks to this increased research interest, it must now be considered an emerging theme. Particular work worth highlighting includes a study using neural networks, an otherwise rarely used technique in the tourism sector, to construct gastronomic tourist profiles through behavioral analysis ( Moral-Cuadra et al. , 2021 ). In addition, new research is emerging concerning the design of gastronomic experiences based on consumer opinion, that is, involving co-creation ( Lin et al. , 2022 ). The exponential growth in co-creation strategies has already been pointed out by other authors.
5.6 Ten thematic areas across 27 years
Here, we give a structural analysis of the evolution of an academic field that has matured over the past 27 years. This analysis shows the development of 10 key areas (shaded with 10 different colors in Figure 3 ): “destination marketing,” “mobility patterns,” “co-creation,” “gastronomy,” “sustainability,” “tourist behavior,” “market segmentation,” “artificial neural networks,” “pricing” and “tourist satisfaction.” The literature demonstrates a solid cohesion because many of the same themes appear in all four of the different periods of development identified, showing the consolidation of these themes.
In the first period we examined, there are two thematic areas which might be described as classic: “mobility patterns” (81 papers and 988 citations) and “tourist behavior” (81 papers and 1,474 citations). In the second period , two further topics are added to the list: “tourist satisfaction” (541 papers and 4,379 citations) and “pricing” (181 papers and 1,195 citations). In the third period , two further topics are added to the list: “destination marketing” (220 papers and 1,450 citations) and “co-creation” (40 papers and 639 citations). These three periods represent the basis of BD tourism marketing research and show a highly developed line of investigation: the prediction of behavior patterns based on geo-tagged content enabling the improvement of strategies for destination marketing.
The fourth period of study , composed of articles published most recently (2020–2022) and thus affected by the COVID-19 pandemic, contains several emergent themes that may well gain importance in the future. These topics include, “gastronomy” (17 papers and 86 citations), “market segmentation” (75 papers and 1,577 citations), “sustainability” (55 papers and 768 citations) and “artificial neural networks” (158 papers and 2,447 citations). Artificial neural networks in particular have been in use from the beginnings of applied artificial intelligence (AI) in tourism marketing. However, it is only in recent years that their use has become widespread, and they should now be considered among the most important tools in tourism marketing ( Mariani and Baggio, 2021 ).
The two themes that stand out most in terms of impact indices are tourist satisfaction and destination marketing. These topics can, therefore, be considered as those of central importance are fundamental to the development of the whole field.
The “tourist satisfaction” theme shows a definitive upward trend with respect to relevant indices and citation numbers. This theme starts with a very small footprint which has grown and reflects the rapid development of this topic such that it is now considered as one of the leading areas of research. On the other hand, topics such as “astro-tourism” initially achieved high impact, but this has not grown over time. Other areas exist that have maintained their relevance throughout the 27 years studied, for example, “pricing” and still others, such as “co-creation” and “gastronomy” that have expanded, branching into new themes and gaining relevance in each subsequent time period.
The fourth period indicates the expanding use of BD in the field of tourism marketing and the increasing multidisciplinarity of the areas under investigation.
6. Discussion
There are several conclusions in the present study. Among the most important of these is revealing the direction of future research trends as well as identifying the structure of relationships between current and past themes in the research areas of BD, tourism and marketing.
This is the first study to apply a bibliometric approach to a clear gap in the research, in that it covers these three thematic areas simultaneously. In addition, it is unique in covering such a wide time period, from 1996 to 2022; thus, it includes the two years corresponding to the COVID-19 pandemic. This two-year period is significant as it was particularly productive and saw the emergence of several new themes.
In this way, we have been able to identify tools, types of BD techniques, authors and most importantly, conceptual themes that have played the most vital roles in this research field throughout the 27 years studied. Thus, as explained previously, this work constitutes a significant contribution to the field by uniquely covering BD, tourism and marketing.
We developed a schematic diagram to show the evolution of principal research themes from 1996 to 2022, divided into four individual time periods. To this end, we used the SciMAT to make an initial, exhaustive bibliometric search of the literature with 1,152 articles published on WoS. This constitutes the entire academic output in this field to date and publications can be divided into four categories corresponding to different periods: digitalization of information (1996–2006); acceleration (2007–2016); consolidation (2017–2020); and COVID-19 (2020–2022).
To aid analysis, the body of research considered in this study was separated into ten major thematic areas: “destination marketing,” “mobility patterns,” “co-creation,” “gastronomy,” “sustainability,” “tourist behavior,” “market segmentation,” “artificial neural networks,” “pricing” and “tourist satisfaction.”
A particularly important area was “tourist satisfaction,” which shows an upward trend through the full 27-year span of this study, reaching what might be called its golden era in the third time period considered. Tourism research defines the general concept of tourist satisfaction and also identifies several dimensions, among which one of the most important is visitor perceptions of hotel attributes. The analysis of tourist satisfaction has been assisted primarily by marketing platforms on social media networks. In recent times, certain networks, such as Twitter, have declined in importance, giving way to other UGC platforms like TripAdvisor which allows access to tourists’ opinions through the reviews they leave.
The most important aspect of this work has been the identification of future lines of investigation and where there is a need to deepen our understanding in certain fields.
7. Implications
This investigation highlights the relevance of BD in tourism marketing research, demonstrates its importance to business and offers relevant and empirical information to tourism-related organizations and private businesses.
In the first place, this review suggests that researchers are interested in BD, tourism and marketing in many different disciplines. In fact, our analysis shows that many of the academics contributing to the field of BD and tourism do not publish in marketing journals. Thus, we would suggest that more interdisciplinary collaboration would help advance the field and, perhaps, this observation constitutes one of the principal contributions of this work. Through this analysis, we hope to provide information concerning new opportunities for research and help to strengthen lines of investigation that may be of potential interest both for academics and practitioners in this field. This is especially important for establishing possible collaborations between these two groups.
In the second place, marketing professionals should invest in more research into the problems they wish to solve using BD and AI since, as we have seen, their current uses are many and varied: predicting tourism demand, analyzing tourist satisfaction, or market segmentation. On the basis of such research, businesses could obtain a variety of appropriate data for every type of analysis or purpose proposed.
In the third place, while the tourism industry is making effective investment in the management of BD and its analysis of AI, this bibliometric analysis demonstrates that the contribution of academic research is also significant. Thus, collaboration between industry and academia would further invigorate this area of research and facilitate its advance.
Finally, given that the rate of evolution in marketing strategies based on new technologies is extremely fast moving, leading hotel and tourism businesses, and indeed, marketing consultants, must make use of AI to improve, innovate and extract the maximum value from data. Furthermore, this may be even more important in the wake of the COVID-19 pandemic, as this work demonstrates that the correct management of data is increasingly invaluable to the industry being able to respond and adapt to external shocks. This information can then be used to plan more efficient business strategies focused on specific types of clients.
8. Limitations and future research
It is necessary to address the limitations of this study. The use of other databases such as Scopus or Google scholar might have provided additional results. Thus, WoS was considered adequate for our purposes.
Despite this limitation, we feel this investigation is of undoubted interest. It provides a novel, possibly the only, presentation of the major trends in this area of research and as a result provides a point of departure for academics and practitioners to discover new avenues of investigation, as well as strengthening already established lines of research, for example, the “sustainability” theme in which it recommends considering the profitability of hotel businesses and tourist perceptions; or “gastronomy,” where there is a large gap in the literature concerning the gastronomical profiling of tourists, and this could be solved by the use of techniques such as neural networks. Other emergent themes are “social media analysis” to study tourist decision-making, “values” and “prices.”
Analytical process implemented
Strategic diagrams between 1996 and 2022 (cites and papers ): (a) 1996–2006; (b) 2007–2016; (c) 2017–2020 (March); (d) 2020 (April)–2022 (April)
Thematic map of big data tourism marketing literature (1996–2022)
Summary of the most important aspects of the four periods
Alegre , J. and Garau , J. ( 2010 ), “ Tourist satisfaction and dissatisfaction ”, Annals of Tourism Research , Vol. 37 No. 1 , pp. 52 - 73 .
Batista e Silva , F. , Marín Herrera , M.A. , Rosina , K. , Ribeiro Barranco , R. , Freire , S. and Schiavina , M. ( 2018 ), “ Analysing spatiotemporal patterns of tourism in Europe at high-resolution with conventional and big data sources ”, Tourism Management , Vol. 68 , pp. 101 - 115 .
Berezina , K. , Bilgihan , A. , Cobanoglu , C. and Okumus , F. ( 2016 ), “ Understanding satisfied and dissatisfied hotel customers: text mining of online hotel reviews ”, Journal of Hospitality Marketing and Management , Vol. 25 No. 1 , pp. 1 - 24 .
Bloom , J.Z. ( 2004 ), “ Tourist market segmentation with linear and non-linear techniques ”, Tourism Management , Vol. 25 No. 6 , pp. 723 - 733 .
Brida , J.G. , Disegna , M. and Osti , L. ( 2012 ), “ Segmenting visitors of cultural events by motivation: a sequential non-linear clustering analysis of Italian christmas market visitors ”, Expert Systems with Applications , Vol. 39 No. 13 , pp. 11349 - 11356 .
Calof , J. , Søilen , K.S. , Klavans , R. , Abdulkader , B. and Moudni , I.E. ( 2022 ), “ Understanding the structure, characteristics, and future of collective intelligence using local and global bibliometric analyses ”, Technological Forecasting and Social Change , Vol. 178 , p. 121561 .
Canziani , B. and Nemati , H.R. ( 2021 ), “ Core and supplemental elements of hospitality in the sharing economy: insights from semantic and tonal cues in airbnb property listings ”, Tourism Management , Vol. 87 , p. 104377 .
Casamatta , G. , Giannoni , S. , Brunstein , D. and Jouve , J. ( 2022 ), “ Host type and pricing on airbnb: seasonality and perceived market power ”, Tourism Management , Vol. 88 , p. 104433 .
Chan , I.C.C. , Fong , D.K.C. , Law , R. and Fong , L.H.N. ( 2018 ), “ State-of-the-art social customer relationship management ”, Asia Pacific Journal of Tourism Research , Vol. 23 No. 5 , pp. 423 - 436 .
Claveria , O. and Torra , S. ( 2014 ), “ Forecasting tourism demand to Catalonia: neural networks vs. time series models ”, Economic Modelling , Vol. 36 , pp. 220 - 228 .
Cobo , M.J. , López-Herrera , A.G. , Herrera-Viedma , E. and Herrera , F. ( 2011a ), “ An approach for detecting, quantifying, and visualizing the evolution of a research field: a practical application to the fuzzy sets theory field ”, Journal of Informetrics , Vol. 5 No. 1 , pp. 146 - 166 .
Cobo , M.J. , López-Herrera , A.G. , Herrera-Viedma , E. and Herrera , F. ( 2011b ), “ Science mapping software tools: review, analysis, and cooperative study among tools ”, Journal of the American Society for Information Science and Technology , Vol. 62 No. 7 , pp. 1382 - 1402 .
Cobo , M.J. , López-Herrera , A.G. , Herrera-Viedma , E. and Herrera , F. ( 2012 ), “ SciMAT: a new science mapping analysis software tool ”, Journal of the American Society for Information Science and Technology , Vol. 63 No. 8 , pp. 1609 - 1630 .
Curry , B. , Davies , F. , Phillips , P. , Evans , M. and Moutinho , L. ( 2001 ), “ The Kohonen self-organizing map: an application to the study of strategic groups in the UK hotel industry ”, Expert Systems , Vol. 18 No. 1 , pp. 19 - 31 .
Deng , N. and Li , X.R. ( 2018 ), “ Feeling a destination through the ‘right’ photos: a machine learning model for DMOs’ photo selection ”, Tourism Management , Vol. 65 , pp. 267 - 278 .
Filieri , R. and Mariani , M. ( 2021 ), “ The role of cultural values in consumers’ evaluation of online review helpfulness: a big data approach ”, International Marketing Review , Vol. 38 No. 6 , pp. 1267 - 1288 .
García-Palomares , J.C. , Gutiérrez , J. and Mínguez , C. ( 2015 ), “ Identification of tourist hot spots based on social networks: a comparative analysis of European metropolises using photo-sharing services and GIS ”, Applied Geography , Vol. 63 , pp. 408 - 417 .
Ghose , A. , Ipeirotis , P.G. and Li , B. ( 2012 ), “ Designing ranking systems for hotels on travel search engines by mining user-generated and crowdsourced content ”, Marketing Science , Vol. 31 No. 3 , pp. 493 - 520 .
Go , H. , Kang , M. and Nam , Y. ( 2020 ), “ The traces of ecotourism in a digital world: spatial and trend analysis of geotagged photographs on social media and Google search data for sustainable development ”, Journal of Hospitality and Tourism Technology , Vol. 11 No. 2 , pp. 183 - 202 .
Gómez , M. , Tinoco Guerrero , N.S. and Tinoco Guerrero , L.M. ( 2021 ), “ The influence of airbnb on hotel occupancy in Mexico: a big data analysis (2007-2018) ”, Revista Cimexus , Vol. 16 No. 1 , pp. 9 - 32 .
Guo , Y. , Barnes , S.J. and Jia , Q. ( 2017 ), “ Mining meaning from online ratings and reviews: tourist satisfaction analysis using latent Dirichlet allocation ”, Tourism Management , Vol. 59 , pp. 467 - 483 .
Hall , C.M. ( 2011 ), “ Publish and perish? Bibliometric analysis, journal ranking and the assessment of research quality in tourism ”, Tourism Management , Vol. 32 No. 1 , pp. 16 - 27 .
Hsu , C.H.C. and Huang , S. ( 2012 ), “ An extension of the theory of planned behavior model for tourists ”, Journal of Hospitality and Tourism Research , Vol. 36 No. 3 , pp. 390 - 417 .
Karimi , S. , Shakery , A. and Verma , R. ( 2020 ), “ Online news media website ranking using user-generated content ”, Journal of Information Science , Vol. 47 No. 3 , pp. 340 - 358 .
Kim , H. and So , K.K.F. ( 2022 ), “ Two decades of customer experience research in hospitality and tourism: a bibliometric analysis and thematic content analysis ”, International Journal of Hospitality Management , Vol. 100 , p. 103082 .
Kim , J. , Wei , S. and Ruys , H. ( 2003 ), “ Segmenting the market of west Australian senior tourists using an artificial neural network ”, Tourism Management , Vol. 24 No. 1 , pp. 25 - 34 .
Kontogianni , A. and Alepis , E. ( 2020 ), “ Smart tourism: state of the art and literature review for the last six years ”, Array , Vol. 6 , p. 100020 .
Köseoglu , M.A. , Sehitoglu , Y. and Craft , J. ( 2015 ), “ Academic foundations of hospitality management research with an emerging country focus: a citation and co-citation analysis ”, International Journal of Hospitality Management , Vol. 45 , pp. 130 - 144 .
Köseoglu , M.A. , Rahimi , R. , Okumus , F. and Liu , J. ( 2016 ), “ Bibliometric studies in tourism ”, Annals of Tourism Research , Vol. 61 , pp. 180 - 198 .
Krawczyk , M. and Xiang , Z. ( 2016 ), “ Perceptual mapping of hotel brands using online reviews: a text analytics approach ”, Information Technology and Tourism , Vol. 16 No. 1 , pp. 23 - 43 .
Leong , L.Y. , Hew , T.S. , Lee , V.H. and Ooi , K.B. ( 2015 ), “ An SEM-artificial-neural-network analysis of the relationships between SERVPERF, customer satisfaction and loyalty among low-cost and full-service airline ”, Expert Systems with Applications , Vol. 42 No. 19 , pp. 6620 - 6634 .
Levin , N. , Kark , S. and Crandall , D. ( 2015 ), “ Where have all the people gone? Enhancing global conservation using night lights and social media ”, Ecological Applications , Vol. 25 No. 8 , pp. 2153 - 2167 .
Li , J. , Xu , L. , Tang , L. , Wang , S. and Li , L. ( 2018 ), “ Big data in tourism research: a literature review ”, Tourism Management , Vol. 68 , pp. 301 - 323 .
Lin , M.-P. , Marine-Roig , E. and Llonch-Molina , N. ( 2022 ), “ Gastronomic experience (co)creation: evidence from Taiwan and Catalonia ”, Tourism Recreation Research , Vol. 47 No. 3 , pp. 277 - 292 .
Liu , Y. and Beldona , S. ( 2021 ), “ Extracting revisit intentions from social media big data: a rule-based classification model ”, International Journal of Contemporary Hospitality Management , Vol. 33 No. 6 , pp. 2176 - 2193 .
Liu , P. , Zhang , H. , Zhang , J. , Sun , Y. and Qiu , M. ( 2019 ), “ Spatial-temporal response patterns of tourist flow under impulse pre-trip information search: from online to arrival ”, Tourism Management , Vol. 73 , pp. 105 - 114 .
Ma , S. , Kirilenko , A.P. and Stepchenkova , S. ( 2020 ), “ Special interest tourism is not so special after all: big data evidence from the 2017 great American solar eclipse ”, Tourism Management , Vol. 77 , p. 104021 .
Mariani , M. and Baggio , R. ( 2021 ), “ Big data and analytics in hospitality and tourism: a systematic literature review ”, International Journal of Contemporary Hospitality Management , Vol. 34 No. 1 , pp. 231 - 278 .
Mariani , M. , Di Felice , M. and Mura , M. ( 2016 ), “ Facebook as a destination marketing tool: evidence from Italian regional destination management organizations ”, Tourism Management , Vol. 54 , pp. 321 - 343 .
Mariani , M. , Mura , M. and Di Felice , M. ( 2018 ), “ The determinants of Facebook social engagement for national tourism organizations’ Facebook pages: a quantitative approach ”, Journal of Destination Marketing and Management , Vol. 8 , pp. 312 - 325 .
Marine-Roig , E. and Huertas , A. ( 2020 ), “ How safety affects destination image projected through online travel reviews ”, Journal of Destination Marketing and Management , Vol. 18 , p. 100469 .
Moral-Cuadra , S. , Solano-Sánchez , M.Á. , Menor-Campos , A. and López-Guzmán , T. ( 2021 ), “ Discovering gastronomic tourists’ profiles through artificial neural networks: analysis, opinions and attitudes ”, Tourism Recreation Research , Vol. 47 No. 3 , pp. 347 - 358 .
Moutinho , L. , Caber , M. , Silva , M.M. and Albayrak , T. ( 2015 ), “ Impact of group package tour dimensions on customer satisfaction (an ANNs application) ”, Tourism Analysis , Vol. 20 No. 6 , pp. 619 - 629 .
Nilashi , M. , Asadi , S. , Minaei-Bidgoli , B. , Ali Abumalloh , R. , Samad , S. , Ghabban , F. and Ahani , A. ( 2021 ), “ Recommendation agents and information sharing through social media for coronavirus outbreak ”, Telematics and Informatics , Vol. 61 , p. 101597 .
Palmer , A. , Montaño , J. and Sesé , A. ( 2006 ), “ Designing an artificial neural network for forecasting tourism time series ”, Tourism Management , Vol. 27 No. 5 , pp. 781 - 790 .
Pan , B. and Yang , Y. ( 2017 ), “ Forecasting destination weekly hotel occupancy with big data ”, Journal of Travel Research , Vol. 56 No. 7 , pp. 957 - 970 .
Park , S.B. , Ok , C.M. and Chae , B.K. ( 2016 ), “ Using twitter data for cruise tourism marketing and research ”, Journal of Travel and Tourism Marketing , Vol. 33 No. 6 , pp. 885 - 898 .
Pattie , D.C. and Snyder , J. ( 1996 ), “ Using a neural network to forecast visitor behavior ”, Annals of Tourism Research , Vol. 23 No. 1 , pp. 151 - 164 .
Penagos-Londoño , G.I. , Rodriguez-Sanchez , C. , Ruiz-Moreno , F. and Torres , E. ( 2021 ), “ A machine learning approach to segmentation of tourists based on perceived destination sustainability and trustworthiness ”, Journal of Destination Marketing and Management , Vol. 19 , p. 100532 .
Salas-Olmedo , M.H. , Moya-Gómez , B. , García-Palomares , J.C. and Gutiérrez , J. ( 2018 ), “ Tourists’ digital footprint in cities: comparing big data sources ”, Tourism Management , Vol. 66 , pp. 13 - 25 .
Samara , D. , Magnisalis , I. and Peristeras , V. ( 2020 ), “ Artificial intelligence and big data in tourism: a systematic literature review ”, Journal of Hospitality and Tourism Technology , Vol. 11 No. 2 , pp. 343 - 367 .
Sánchez-Martín , J.M. , Gurría-Gascón , J.L. and Rengifo-Gallego , J.I. ( 2020 ), “ The distribution of rural accommodation in Extremadura, Spain-between the randomness and the suitability achieved by means of regression models (OLS vs. GWR) ”, Sustainability , Vol. 12 No. 11 , p. 4737 .
Shahrabi , J. , Hadavandi , E. and Asadi , S. ( 2013 ), “ Developing a hybrid intelligent model for forecasting problems: case study of tourism demand time series ”, Knowledge-Based Systems , Vol. 43 , pp. 112 - 122 .
Smithson , S. , Devece , C.A. and Lapiedra , R. ( 2011 ), “ Online visibility as a source of competitive advantage for small- and medium-sized tourism accommodation enterprises ”, The Service Industries Journal , Vol. 31 No. 10 , pp. 1573 - 1587 .
Song , S. , Park , S.B. and Park , K. ( 2020 ), “ Thematic analysis of destination images for social media engagement marketing ”, Industrial Management and Data Systems , Vol. 121 No. 6 , pp. 1375 - 1397 .
Sota , S. , Chaudhry , H. and Srivastava , M.K. ( 2020 ), “ Customer relationship management research in hospitality industry: a review and classification ”, Journal of Hospitality Marketing and Management , Vol. 29 No. 1 , pp. 39 - 64 .
Supak , S. , Brothers , G. , Bohnenstiehl , D.W. and Devine , H. ( 2015 ), “ Geospatial analytics for federally managed tourism destinations and their demand markets ”, Journal of Destination Marketing and Management , Vol. 4 No. 3 , pp. 173 - 186 .
Tranfield , D. , Denyer , D. and Smart , P. ( 2003 ), “ Towards a methodology for developing evidence-informed management knowledge by means of systematic review ”, British Journal of Management , Vol. 14 No. 3 , pp. 207 - 222 .
Trang , L.H. , Huy , T.D. and Le , A.N. ( 2021 ), “ Clustering helps to improve price prediction in online booking systems ”, International Journal of Web Information Systems , Vol. 17 No. 1 , pp. 45 - 53 .
Valls , F. and Roca , J. ( 2021 ), “ Visualizing digital traces for sustainable urban management: mapping tourism activity on the virtual public space ”, Sustainability , Vol. 13 No. 6 , p. 3159 .
Villamediana-Pedrosa , J.D. , Vila-Lopez , N. and Küster-Boluda , I. ( 2019 ), “ Secrets to design an effective message on Facebook: an application to a touristic destination based on big data analysis ”, Current Issues in Tourism , Vol. 22 No. 15 , pp. 1841 - 1861 .
Witt , S.F. and Witt , C.A. ( 1995 ), “ Forecasting tourism demand: a review of empirical research ”, International Journal of Forecasting , Vol. 11 No. 3 , pp. 447 - 475 .
Xiang , Z. ( 2018 ), “ From digitization to the age of acceleration: on information technology and tourism ”, Tourism Management Perspectives , Vol. 25 , pp. 147 - 150 .
Xie , K.L. , Zhang , Z. and Zhang , Z. ( 2014 ), “ The business value of online consumer reviews and management response to hotel performance ”, International Journal of Hospitality Management , Vol. 43 , pp. 1 - 12 .
Zhu , J.J. , Chang , Y.C. , Ku , C.H. , Li , S.Y. and Chen , C.J. ( 2021 ), “ Online critical review classification in response strategy and service provider rating: algorithms from heuristic processing, sentiment analysis to deep learning ”, Journal of Business Research , Vol. 129 , pp. 860 - 877 .
Zola , P. , Cortez , P. , Ragno , C. and Brentari , E. ( 2019 ), “ Social media cross-source and cross-domain sentiment classification ”, International Journal of Information Technology and Decision Making , Vol. 18 No. 5 , pp. 1469 - 1499 .
Acknowledgements
This research was funded by Ministerio de Industria, Comercio y Turismo (Spain), AEI-010500–2020-253 (DTI^A Project: 4.0 technological tools for measurement, evaluation and monitoring of the Friendliness concept linked to the Smart Tourist Destinations)
Declaration of interest: None
Corresponding author
Related articles, all feedback is valuable.
Please share your general feedback
Report an issue or find answers to frequently asked questions
Contact Customer Support
The Ever-Evolving Landscape of Marketing Research for Air Transport and Tourism
- Conference paper
- First Online: 02 July 2024
- Cite this conference paper
- Erasmia Leonidou 2
Part of the book series: Springer Proceedings in Business and Economics ((SPBE))
Included in the following conference series:
- GMA-GAMMA Joint Symposium
Covid-19 pandemic affected the everyday life of the entire world and disrupted the global economy. Consequently, industries like air travel and tourism faced unprecedented upheavals and now all stakeholders need to navigate through these transformative changes. Based on this, marketing research becomes an essential tool for adapting and successfully navigating in this volatile environment. The chapter deals with this evolving landscape of marketing research and air transport within the tourism industry. In particular, the focus of this chapter is Marketing's role in empowering airlines and tourism stakeholders to align to the changing needs of their customers in the evolving digital landscape, which is becoming fertile ground for environmentally conscious travel practices and innovative green strategies.
This is a preview of subscription content, log in via an institution to check access.
Access this chapter
Subscribe and save.
- Get 10 units per month
- Download Article/Chapter or Ebook
- 1 Unit = 1 Article or 1 Chapter
- Cancel anytime
- Available as PDF
- Read on any device
- Instant download
- Own it forever
- Available as EPUB and PDF
- Durable hardcover edition
- Dispatched in 3 to 5 business days
- Free shipping worldwide - see info
Tax calculation will be finalised at checkout
Purchases are for personal use only
Institutional subscriptions
Abbas, J., Mubeen, R., Iorember, P.T., Raza, S., Mamirkulova, G.: Exploring the impact of COVID-19 on tourism: transformational potential and implications for a sustainable recovery of the travel and leisure industry. Curr. Res. Behav. Sci. 2 , 100033 (2021). https://doi.org/10.1016/j.crbeha.2021.100033
Article Google Scholar
Bai, Y., Yao, L., Wei, T., Tian, F., Jin, D.Y., Chen, L., Wang, M.: Presumed asymptomatic carrier transmission of COVID-19. JAMA 323 (14), 1406–1407 (2020). https://doi.org/10.1001/jama.2020.2565
Brozovic, D.: Strategic flexibility: a review of the literature. Int. J. Manag. Rev. 20 (1), 3–31 (2018). https://doi.org/10.1111/ijmr.12111
Cole, A., Baker, J.S., Stivas, D.: Trust, transparency and transnational lessons from COVID-19. J. Risk Financ. Manag. 14 (12), 607 (2021). https://doi.org/10.3390/jrfm14120607
Connell, J.: Film tourism–Evolution, progress and prospects. Tour. Manag. 33 (5), 1007–1029 (2012). https://doi.org/10.1016/j.tourman.2012.02.008
Darbellay, F., Stock, M.: Tourism as complex interdisciplinary research object. Ann. Tour. Res. 39 (1), 441–458 (2012). https://doi.org/10.1016/j.annals.2011.07.002
de Fine Licht, J.: Do we really want to know? The potentially negative effect of transparency in decision making on perceived legitimacy. Scand. Polit. Stud. 34 (3), 183–201 (2011). https://doi.org/10.1111/j.1467-9477.2011.00268.x
Dierikx, M.: Clipping the Clouds: How Air Travel Changed the World: How Air Travel Changed the World. ABC-CLIO (2008)
Google Scholar
Dube, K., Nhamo, G., Swart, M.P.: Conclusion: practical and policy perspectives in reshaping the tourism and hospitality industry post-COVID-19 industry. In: COVID-19, Tourist Destinations and Prospects for Recovery: Volume One: A Global Perspective, pp. 359–370. Springer International Publishing, Cham (2023). https://doi.org/10.1007/978-3-031-22257-3_19
EC.: Strategic Foresight Report—charting the course towards a more resilient Europe. European Commission COM, 493. Brussels (2020). https://commission.europa.eu/system/files/2021-04/strategic_foresight_report_2020_1_0.pdf. Accessed 19 Aug 2023
Fang, B., Ye, Q., Kucukusta, D., Law, R.: Analysis of the perceived value of online tourism reviews: influence of readability and reviewer characteristics. Tour. Manag. 52 , 498–506 (2016). https://doi.org/10.1016/J.TOURMAN.2015.07.018
Feyers, S., Stein, T., Klizentyte, K.: Bridging worlds: utilizing a multi-stakeholder framework to create extension–tourism partnerships. Sustainability 12 (1), 80 (2019). https://doi.org/10.3390/su12010080
Fritsche, U., Brunori, G., Chiaramonti, D., Galanakis, C., Matthews, R., Panoutsou, C., Borzacchiello, M.T., Stoermer, E., Avraamides, M., European Commission. Joint Research Centre.: Future transitions for the bioeconomy towards sustainable development and a climate-neutral economy : foresight scenarios for the EU bioeconomy in 2050 (n.d.) (2021)
Galanakis, C.M., Brunori, G., Chiaramonti, D., Matthews, R., Panoutsou, C., Fritsche, U.R.: Bioeconomy and green recovery in a post-COVID-19 era. Sci. Total Environ. 808 , 152180 (2022). https://doi.org/10.1016/J.SCITOTENV.2021.152180
Gössling, S., Scott, D., Hall, C.M.: Pandemics, tourism and global change: a rapid assessment of COVID-19. J. Sustain. Tour. 29 (1), 1–20 (2020). https://doi.org/10.1080/09669582.2020.1758708
Gunter, U., Smeral, E., Zekan, B.: Forecasting tourism in the EU after the COVID-19 crisis. J. Hosp. Tour. Res. 10963480221125130 (2022). https://doi.org/10.1177/10963480221125130
He, H., Harris, L.: The impact of Covid-19 pandemic on corporate social responsibility and marketing philosophy. J. Bus. Res. 116 , 176–182 (2020). https://doi.org/10.1016/J.JBUSRES.2020.05.030
IATA.: Economic Performance of the Airline Industry (2018). https://www.iata.org/contentassets/f88f0ceb28b64b7e9b46de44b917b98f/iata-economic-performance-of-the-industry-end-year-2018-report.pdf
ILO.: The Future of Work in the Tourism Sector: Sustainable and Safe Recovery and Decent Work in the Context of the COVID-19 Pandemic. International Labour Organization (ILO), Geneva (2022). https://www.ilo.org/wcmsp5/groups/public/---ed_dialogue/---sector/documents/meetingdocument/wcms_840403.pdf . Accessed 19 Aug 2023
Ivanova, M., Ivanov, I.K., Ivanov, S.: Travel behaviour after the pandemic: the case of Bulgaria. Anatolia 32 (1), 1–11 (2021). https://doi.org/10.1080/13032917.2020.1818267
Khwaja, M.G., Zaman, U.: Configuring the evolving role of ewom on the consumers information adoption. J. Open Innov.: Technol. Mark. Complex. 6 (4), 125 (2020). https://doi.org/10.3390/joitmc6040125
Kock, F., Nørfelt, A., Josiassen, A., Assaf, A.G., Tsionas, M.G.: Understanding the COVID-19 tourist psyche: the evolutionary tourism paradigm. Ann. Tour. Res. 85 , 103053 (2020). https://doi.org/10.1016/J.ANNALS.2020.103053
Liu, A., Kim, Y.R., O’Connell, J.F.: COVID-19 and the aviation industry: the interrelationship between the spread of the COVID-19 pandemic and the frequency of flights on the EU market. Ann. Tour. Res. 91 , 103298 (2021). https://doi.org/10.1016/j.annals.2021.103298
Mertens, G., Gerritsen, L., Duijndam, S., Salemink, E., Engelhard, I.M.: Fear of the coronavirus (COVID-19): predictors in an online study conducted in March 2020. J. Anxiety Disord. 74 , 102258 (2020). https://doi.org/10.1016/j.janxdis.2020.102258
Moisey, R.N., McCool, S.F.: Sustainable tourism in the 21st century: lessons from the past, challenges to address. In: Tourism, Recreation and Sustainability: Linking Culture and the Environment, pp. 283–291. CABI, Wallingford UK (2008)
Chapter Google Scholar
Nunes, S., Cooke, P.: New global tourism innovation in a post-coronavirus era. Eur. Plan. Stud. 29 (1), 1–19 (2021). https://doi.org/10.1080/09654313.2020.1852534
OECD.: OECD Tourism Trends and Policies (2022). https://www.oecd.org/cfe/oecd-tourism-trends-and-policies-20767773.htm . Accessed 8 Aug 2023
Orîndaru, A., Popescu, M.F., Alexoaei, A.P., Căescu, ȘC., Florescu, M.S., Orzan, A.O.: Tourism in a post-COVID-19 era: sustainable strategies for industry’s recovery. Sustainability 13 (12), 6781 (2021). https://doi.org/10.3390/su13126781
Scott, N., Laws, E., Prideaux, B.: Tourism crises and marketing recovery strategies. J. Travel Tour. Mark. 23 (2–4), 1–13 (2008). https://doi.org/10.1300/J073v23n02_01
Sigala, M.: Tourism and COVID-19: Impacts and implications for advancing and resetting industry and research. J. Bus. Res. 117 , 312–321 (2020). https://doi.org/10.1016/j.jbusres.2020.06.015
Sun, Y., Song, H., Jara, A.J., Bie, R.: Internet of things and big data analytics for smart and connected communities. IEEE Access 4 , 766–773 (2016)
Tariyal, A., Bisht, S., Rana, V., Roy, S., Pratap, S.: Utilitarian and hedonic values of eWOM media and online booking decisions for tourist destinations in India. J. Open Innov.: Technol. Mark. Complex. 8 (3), 137 (2022). https://doi.org/10.3390/joitmc8030137
Toubes, D.R., Vila, N.A., Fraiz Brea, J.A.: Changes in consumption patterns and tourist promotion after the covid-19 pandemic. J. Theor. Appl. Electron. Commer. Res. 16 (5), 1332–1352 (2021). https://doi.org/10.3390/jtaer16050075
Yoo, M., Lee, S., Bai, B.: Hospitality marketing research from 2000 to 2009: topics, methods, and trends. Int. J. Contemp. Hosp. Manag. 23 (4), 517–532 (2011). https://doi.org/10.1108/09596111111130010
Yousaf, Z., Radulescu, M., Sinisi, C.I., Serbanescu, L., Paunescu, L.M.:. Harmonization of green motives and green business strategies towards sustainable development of hospitality and tourism industry: green environmental policies. Sustainability (Switzerland) 13 (12) (2021). https://doi.org/10.3390/su13126592
Zhang, L., Yang, H., Wang, K., Bian, L., Zhang, X.: The impact of COVID-19 on airline passenger travel behavior: an exploratory analysis on the Chinese aviation market. J. Air Transp. Manag. 95 , 102084 (2021). https://doi.org/10.1016/j.jairtraman.2021.102084
Download references
Author information
Authors and affiliations.
Cyprus University of Technology, 30 Archbishop Kyprianos Street, 3036, Limassol, Cyprus
Erasmia Leonidou
You can also search for this author in PubMed Google Scholar
Corresponding author
Correspondence to Erasmia Leonidou .
Editor information
Editors and affiliations.
Department of Communication and Marketing, Cyprus University of Technology, LIMASSOL, Cyprus
Anna Zarkada
Rights and permissions
Reprints and permissions

Copyright information
© 2024 The Author(s), under exclusive license to Springer Nature Switzerland AG
About this paper
Cite this paper.
Leonidou, E. (2024). The Ever-Evolving Landscape of Marketing Research for Air Transport and Tourism. In: Zarkada, A. (eds) Marketing Solutions to the Challenges of a VUCA Environment. GMA-GAMMA 2023. Springer Proceedings in Business and Economics. Springer, Cham. https://doi.org/10.1007/978-3-031-58429-9_2
Download citation
DOI : https://doi.org/10.1007/978-3-031-58429-9_2
Published : 02 July 2024
Publisher Name : Springer, Cham
Print ISBN : 978-3-031-58428-2
Online ISBN : 978-3-031-58429-9
eBook Packages : Business and Management Business and Management (R0)
Share this paper
Anyone you share the following link with will be able to read this content:
Sorry, a shareable link is not currently available for this article.
Provided by the Springer Nature SharedIt content-sharing initiative
- Publish with us
Policies and ethics
- Find a journal
- Track your research
Academia.edu no longer supports Internet Explorer.
To browse Academia.edu and the wider internet faster and more securely, please take a few seconds to upgrade your browser .
Enter the email address you signed up with and we'll email you a reset link.
- We're Hiring!
- Help Center
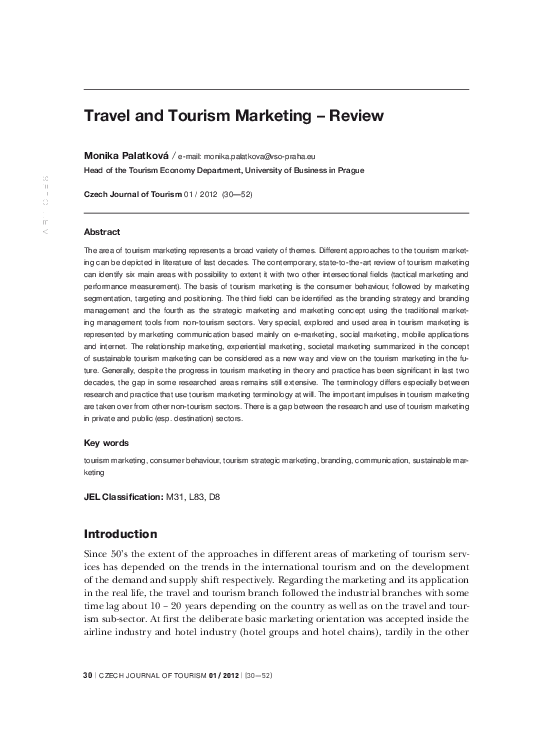
Travel and Tourism Marketing – Review

Related Papers
Jurnal IPTA
Rety Palupi
Tourism is one of the leading and most dynamic sectors of the world economy, and for its rapid pace, it is recognized as the economic phenomenon of the century. Modern tourism is an increasingly intensive, business-oriented, and commercially organized group of activities, and tourism marketing is becoming ever more important for a destination’s competitiveness. In this context, our research aims to analyze and synthesize the challenges marketing of tourist destinations is facing. The approach taken in this paper is to assess the related literature and focus on the key points for future research on tourism marketing. We argue that destination marketing must lead to the optimization of the effects of tourism and the achievement of national tourism organizations' strategic goals. This paper contributes to marketing professionals and academics, pointing out the uniqueness of tourism marketing.
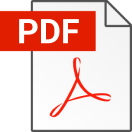
Ann' Arissara
Sara Dolnicar
This paper creates a Tourism Marketing Knowledge Grid and uses it as a framework for the review. The grid reveals that extant tourism marketing research has primarily focused on how service promises are made and kept, and has mostly generated frameworks to improve managerial decision making or provided insights about associations between constructs. Strategic principles, underpinned by the understanding of cause-effect relationships, are rare. These findings point to exciting opportunities for future research, including increased attention on enabling promises made to tourists and development of strategic and research principles; increased use of experimental, quasi-experimental and longitudinal research designs, as well as unstructured qualitative designs; and an increased focus on the study of actual behavior.
International Journal of Tourism and Hospitality in Asia Pasific
Jhonathan Whiryawan
Travel and Tourism is an assemblage of all the leisure, luxuries, comfort, travel products, and services provided by suppliers including airlines, hotels, transportation like self-drive agencies, cruise lines, restaurants, etc. All these functions require marketing. This study aims to explore the marketing patterns of tourist agencies to increase customer awareness. The tourism sector also helps to promote the various hotels, restaurants, rental agencies by giving a platform for all these services to promote their services and also provide a customer discount for customer satisfaction.
IJMSBR Open Access Journal
The new buzz word of success of tourism industry is Destination Marketing. We cannot neglect the role of marketing in finding out the needs, wants, selling and promotion of a product or a service. As we all are aware that tourism is one of the fastest growing service industry which requires consonant efforts because of many reasons likewise the ever-changing demand of tourists, change in climate, changes in the desires of tourists etc. When we talk about the success factors for making tourism industry incredibly growing one we can notify the changing promotional strategies of the Tourism Organizations .We have good examples in India like – Rajasthan Tourism ,Goa Tourism ,Kerala Tourism ,Gujarat Tourism ,Madhya Pradesh Tourism etc. They all using the Destination Marketing for attracting more tourists towards their states .The present paper is an attempt to highlight the importance of Destination Marketing, the process and life cycle of the same .For making it more informative the researcher has stressed upon some major Destination Marketing strategies adopted by different states of India.
Mark Camilleri
This authoritative book is a valuable resource for industry practitioners like consultants, senior executives and managers who work for destination management organizations, hotels, restaurants, inbound / outbound tour operators or travel agents, as well as for academic researchers and students who are aspiring to pursue a career in tourism and hospitality. This title features case studies from real-life businesses and a thorough review of the relevant academic literature. It describes different tourism marketing strategies that are related to cultural tourism, events tourism, food tourism, religious tourism as well as spa and wellness tourism, among others, as they may be utilized by destination managers. At the same time, it comprises discursive contributions that elaborate about critical matters that affect the tourism industry, including consumer (or tourist) behavior; the planning, organization and implementation of responsible tourism practices in different contexts; crisis management; marketing environment issues affecting the long term sustainability of destinations like seasonality factors; as well as the utilization of smart tourism technologies and digital marketing channels, among other topics.
Rodoula Tsiotsou
Annals of Tourism Research
Luiz Moutinho
Annals of the University of Bucharest: Geography Series
Ana-Irina Dinca
Marketing is an essential domain for tourism, being recently more and more theoretically approached and conceptualized by research papers (e specially in the Anglo-Saxon literature). An economic term by excellence, marketing is as well ad opted by geographic literature and by the geography of tourism, one of the major specializati ons of Romanian faculties of geography as numerous students opt for it. Consequently there is a great need for geography students and geographers to study tourism oriented concepts even if mainly coming from other domains such as economy, social sciences, etc. and to elaborate appropriate studies without getting far from their topic of interest. In this respect the develo pment of methods and instruments of research and study is a necessity already underlined by bibliogr aphic references in the domain. They represented th e departure point of this paper which enlarges the deb ate upon a tourism destination marketing study work paper developed as an operati...
International Journal of Strategic Innovative Marketing
Andrej Malachovský
RELATED PAPERS
Journal of Veterinary Medicine and Animal Health
Anthony Ameh
Computers & education
Anh Nguyet Diep
Journal of Oleo Science
merve başak
Applied Surface Science
Virginija Jankauskaite
Franco Provenzano
shabrina ailsa
Journal of Autism and Developmental Disorders
Gonzalo Salazar De Pablo
The Journal of Clinical Endocrinology and Metabolism
Teresa M Seccia
Journal of Lightwave Technology
Jen-inn Chyi
Hakhyun Nam
RELATED TOPICS
- We're Hiring!
- Help Center
- Find new research papers in:
- Health Sciences
- Earth Sciences
- Cognitive Science
- Mathematics
- Computer Science
- Academia ©2024
Thank you for visiting nature.com. You are using a browser version with limited support for CSS. To obtain the best experience, we recommend you use a more up to date browser (or turn off compatibility mode in Internet Explorer). In the meantime, to ensure continued support, we are displaying the site without styles and JavaScript.
- View all journals
- My Account Login
- Explore content
- About the journal
- Publish with us
- Sign up for alerts
- Open access
- Published: 05 July 2024
Assessing the reactions of tourist markets to reinstated travel restrictions in the destination during the post-COVID-19 phase
- Xuankai Ma 1 , 2 , 4 , 5 ,
- Rongxi Ma 2 , 4 ,
- Zijing Ma 5 ,
- Jingzhe Wang 6 ,
- Zhaoping Yang 3 , 4 ,
- Cuirong Wang 2 , 4 &
- Fang Han 2 , 4
Scientific Reports volume 14 , Article number: 15495 ( 2024 ) Cite this article
67 Accesses
Metrics details
- Socioeconomic scenarios
- Sustainability
This study, leveraging search engine data, investigates the dynamics of China's domestic tourism markets in response to the August 2022 epidemic outbreak in Xinjiang. It focuses on understanding the reaction mechanisms of tourist-origin markets during destination crises in the post-pandemic phase. Notably, the research identifies a continuous rise in the potential tourism demand from tourist origin cities, despite the challenges posed by the epidemic. Further analysis uncovers a regional disparity in the growth of tourism demand, primarily influenced by the economic stratification of origin markets. Additionally, the study examines key tourism attractions such as Duku Road, highlighting its resilient competitive system, which consists of distinctive tourism experiences, economically robust tourist origins, diverse tourist markets, and spatial pattern stability driven by economic factors in source cities, illustrating an adaptive response to external challenges such as crises. The findings provide new insights into the dynamics of tourism demand, offering a foundation for developing strategies to bolster destination resilience and competitiveness in times of health crises.
Similar content being viewed by others
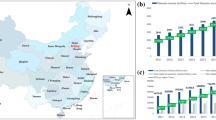
Analysis of spatial patterns and driving factors of provincial tourism demand in China
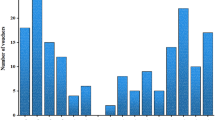
Tourism in pandemic: the role of digital travel vouchers in China
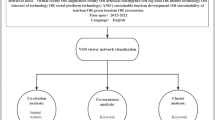
A ten-year review analysis of the impact of digitization on tourism development (2012–2022)
Introduction.
The COVID-19 pandemic has had an enormous and long-lasting social and economic impact on the tourism industry 1 . International tourist arrivals (overnight visitors) plummeted by 73% in 2020 due to a global embargo, widespread travel restrictions, and a massive drop in demand. Compared to 2019, there were approximately 1 billion fewer international visitors that year 2 . The year 2021 is considered by scholars as the Year of Recovery for tourism, with tourism indicators signaling recovery 3 , 4 . The climate crisis and the European return to war are expected to restrict international travel 5 , and the domestic market will be an appropriate approach to drive tourism recovery 6 . The expansion of domestic tourism is propelled by a considerable accumulation of suppressed demand, a rising preference for domestic locales, and stringent border entry and exit controls.
Nevertheless, the mutation of the Omicron virus resulting in greater contagiousness will lead to localized areas being re-closed to counter the diffusion of the Virus 7 . It is an additional shock to the recovering tourist destinations. The investigation of the response of origin markets to the reclosure of destinations during the tourism recovery period is of significant relevance for regional authorities to assess the impact of the outbreak and the maintenance of tourist origins.
This paper aims to investigate what changes occur in origin market demand for destinations affected by reclosure during the tourism recovery period, what spatial differences such changes show, and how the differences in response exhibited by origin markets arise. The response in tourism demand is quantified through the analysis of annual growth rates in the domestic market during periods of reclosure. Based on tourism demand theory, demographic, economic, and environmental conditions, COVID-19 epidemic status, healthcare conditions, Internet access coverage, and traffic activation will be potential influential factors to account for the variation in origins market response. We add new insights to the COVID-19 study of short-term effects and spatial differences in tourism demand by examining the pattern of origins market response in the face of destination reclosure from the tourist origins' perspective (cities).
Literature review
The covid-19 and the tourism industry.
The dramatic impact of COVID-19 on the tourism industry has intensified the involvement of scholars in studies on the issue. In the initial phase of the pandemic (2020), UNWTO reported international tourist arrivals to decline by 70–75% for 2020. Academics have addressed the effects of the pandemic on national tourism markets 8 , 9 , the response of the tourism industry to the crisis 10 , 11 , and the framework for sustainable development 12 , 13 , 14 . Greece, with its developed tourism industry, the loss of tourism revenue due to the pandemic has directly contributed to the overall economic decline of the country 15 . The pandemic has declined tourist arrivals and affected employment and productivity in the Balearic Islands, revealing the region’s decline 1 . Hotel revenues in Italy fell by 66% relative to Turkey due to the embargo policies 16 . The U.S. hotel industry lost about $30 billion in revenue in the spring of 2020 17 , while the restaurant industry also faced a notable crackdown 18 . The multidimensional assessments of the influence of the pandemic on tourism demand were performed by comparing this crisis with the normal state of affairs before the crisis, which pointed to a significant negative consequence of tourism with its sibling sectors along the entire tourism industrial chain 19 . Restoring tourist arrivals to pre-crisis standards will probably confront prolonged pain 20 .
Some improvement in the epidemic accompanies the crisis response and recovery period (after 2021). Regardless, the pace of recovery continues to be sluggish and uneven in all regions of the world due to varying degrees of mobility restrictions, vaccination rates, and traveler confidence. Dynamic adjustment of travel restrictions within the country during the post-pandemic period could stimulate a recovery to a limited extent and allow domestic tourism to dominate 21 , 22 . Empirical studies show that regions with monoculture industries have no buffers to adapt to the crisis in the face of shocks that significantly reduce tourism demand 23 , 24 . In contrast, regions with prosperous industries and strong tourism specialization will be highly resilient to disruptions in the context of less restrictive movement of people, presenting a competitive advantage with a slight decline in demand 25 , 26 . Scholars believe this is the best time to promote equitable and sustainable tourism development, and the disconnect between tourist demand and destination development (growth expansion) needs to be repaired 27 , 28 . The construction of tourism sustainability will focus more on the changing needs of tourists themselves and their demand preferences. In the future, regulating the balanced development of regional tourism by changing tourists’ needs is the focus of tourism sustainability in the post-epidemic era 29 , 30 .
Measures of tourism recovery
In the tourism literature, scholars have proposed and implemented the concept of tourism resilience to quantify post-crisis tourism outcomes, particularly the ability and magnitude of regional tourism to recover from the COVID-19 disruptions 31 . Metrics such as employment rates, tourist arrival rates, and tourism revenues are measures to assess regions’ tourism resilience within geographically large areas 32 , 33 , 34 . It is noteworthy that the recoverability of tourism in different regions varies by geographic area and thus shows distinct patterns of recovery. Thus, the spatial heterogeneity of tourism recovery must be considered 35 . Scholars have argued that location quotients 36 , the share of an industry in the local total divided by the share of the industry in the national total, can better account for the speed of tourism recovery from that region’s crisis 37 .
Tourism demand change observation
In the era of Big Data, the utilization of search engines by tourists to acquire travel-related information ahead of their trips has become the initial step in travel planning 38 . The significance of search engine data in characterizing and predicting tourism demand has been gradually acknowledged by tourism researchers since 2010 and has been incorporated into various models for analytical work 39 , 40 , 41 , 42 . Due to the search behavior of tourists based on their destinations of interest, these electronic behavior records are normalized into search engine indexes to indicate tourism demand, preferences, travel intentions, and the location of the tourists’ origin 43 . Researchers have conducted studies on tourism demand response to pandemics and tourism recovery rates with search engine data, confirming the significant advantages of search engine data in fitting economic indicators during the COVID-19 pandemic 22 , 44 , 45 . Therefore, we attempted to characterize the rate of change in tourism demand in the origins market by investigating search engine data for a specific period (destination reclosure) as search engine data gives high-frequency information on the travel intentions of Internet users in different regions for a defined destination.
Overview summary
In the post-epidemic era of tourism recovery, a sub-black swan event of local reclosure is inevitable. We are interested in how origin markets will respond to destination reclosure, the characteristics of the spatial response patterns, and the reasons for this heterogeneity that need to be urgently explored. While the literature has usefully explored the recovery of tourism with mature domestic markets, it is crucial to focus on the changes in tourism demand for destinations at the national level, where the origin markets are the roots of tourism demand. To address the limitations of previous research, this paper contributes to the tourism literature by using a search engine to quantify short-term tourism changes during tourism crises and constructing models of tourism demand changes in different regions to explore the drivers of different response patterns.
Materials and methodologies
Tourism demand data.
Search engine data is identified as an efficacious data source for measuring high-frequency tourism demand. Google Trends has extensive applications worldwide, whereas Baidu has a more indicative role in China. The Baidu search engine has two data products: Keyword Search Index and Brand Index ( https://index.baidu.com ). The Keyword Search Index contains data from 2011 till now, and the Brand Index is a more professional industry-based index that will be available from August 2021. This paper utilizes daily keyword indices from 2011 to 2022 to investigate the background of tourism demand for the Duku Road and brand indexes from August 10 to October 31 in 2021 and 2022 to measure changes in tourism demand for the Duku Road from different origins regions during the destination closure period under the impact of the pandemic.
where i is a tourist origin city; j is a date from August 10 to October 31; BBI is the Baidu Brand Index of a city search for Duku Road on a date. s is a spatial series of origin cities or an individual city; t is a temporal series of dates; T.D. is the sum of demand from s during t .
The Chinese domestic tourism market was chosen as the study case due to the robust intervention policies adopted by China during the first pandemic, dramatically impacting international and domestic tourism in China. In the post-epidemic era, a multitude of countries have eased international travel restrictions. The massive mobility of individuals within China and the mutation of the Virus made travel policies contingent on the consequences of the containment of the pandemic. In this context, domestic tourism demand has emerged a robust recovery. This phenomenon is also prevalent in other countries, and scholars are confident that domestic tourism will be the recovery engine in the short term. It is a matter of significant concern that tourist arrivals may abruptly decline to zero in instances where destinations are compelled to shut down anew amidst a pandemic resurgence. Notwithstanding, it is pertinent to note that latent tourism demand persists. In response, this study meticulously tracks variations in demand at these destinations and corresponding responses from origin markets, employing high-frequency search engine data as a quantitative metric of demand. Distinct from conventional statistical methods, the monitoring of such rapid changes necessitates the utilization of big data analytics.
Xinjiang was one of the most popular destinations for domestic tourism in 2022, with millions of tourists entering Xinjiang by self-drive, high-speed rail, and air. The Duku Road (high-rank landscape driveway) is the most attractive destination for tourists, with 28.35% of tourism demand in Xinjiang. It serves as a tourist hub, radiating tourists to other attractions 46 . Tourism prosperity has made Xinjiang an unignorable destination during domestic tourism recovery. Nevertheless, the massive tourist flows have planted the potential for the spread of the epidemic. The entire territory of Xinjiang entered a region-wide silent management to control the epidemic on August 10 and will remain in effect through the winter. During this period, all travel activities had to be ceased, and the government organized the transfer of healthy travelers back to their origins. This unexpected outbreak has had a fatal impact on Xinjiang’s thriving tourism industry. The study case has typical implications. This paper investigates the origins market’s response to the destination’s reclosure, considering the Duku Road as the destination and cities outside Xinjiang as the domestic origin markets.
Three hundred and seven cities of China’s domestic tourism market were adopted as the tourist origins for the case study. The Duku Road, located in Xinjiang, served as the destination (Fig. 1 a). The Duku Road is a mysterious and fascinating landscaped driveway that stretches through the north and south of the Tianshan Mountains, a World Natural Heritage Site, which is also known as the Tianshan Road. The Duku Road runs with a unique topography, with numerous sharp curves and steep slopes, more than 280 km of road sections above 2000 m above sea level, 1/3 of the whole course is a cliff, 1/5 of the lot is in the high mountain permafrost, crossing nearly ten major rivers in the Tianshan Mountains, and over four ice-pass of mountains that accumulate snow all year round (Fig. 1 b). Driving on Duku Road, travelers experience the seasonal transformations in a single day, which shows the magnificent scenery of “four seasons in one day, ten miles in different skies” to off-road enthusiasts and self-driving tourists.
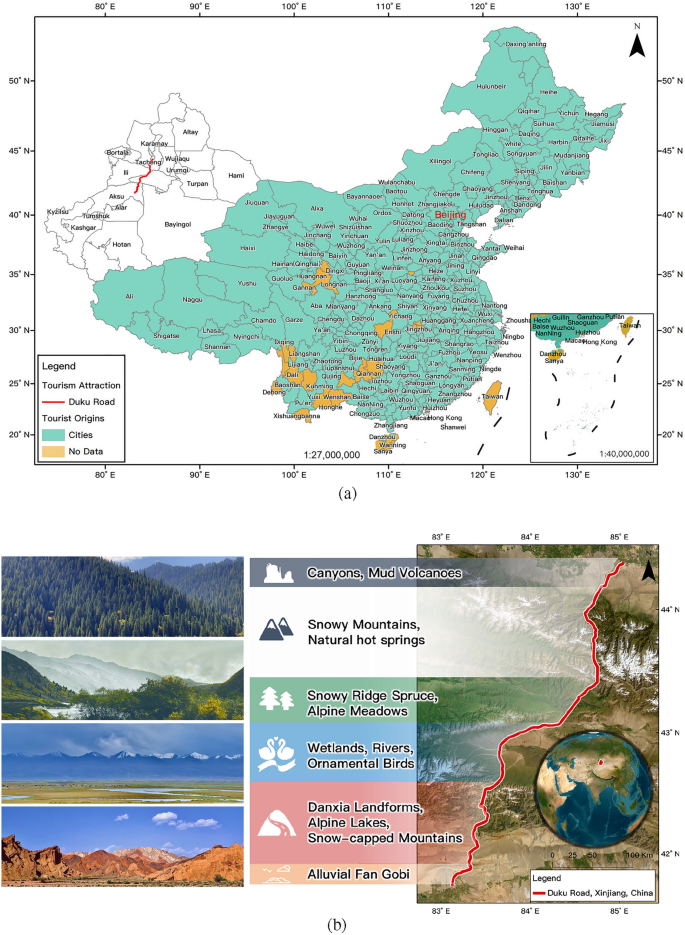
The study case. ( a ) The domestic tourist markets of Duku Road. ( b ) The location of Duku Road, Xinjiang, China. Notes: The map in ( a ) was produced using ESRI ArcMap 10.2, and the standard map service was provided by the Ministry of Natural Resources of China, accessible at http://bzdt.ch.mnr.gov.cn/ with Grant No. GS (2021) 5448. The photographs in ( b ) were taken by the first author for tourism resources investigation in September 2021.
Dependent variables
The literature has proposed numerous valid measures of change in tourism demand, and scholars have characterized tourism resilience based on micro-level expenditure elasticities and annual percentage changes between the pre-and post-pandemic periods 6 , 25 . This paper pays attention to measuring the instantaneous response of origin markets to the public health crisis in a destination that caused the closure period (August 10–October 31), where typically, growth rates are employed to capture the difference in performance relative to a benchmark 26 , 47 . Thus, tourism demand in 2021 is considered the initial period of the post-pandemic recovery period, and the growth rate for the corresponding period in 2022 versus the benchmark period is adopted as a measure of Tourism Demand Growth Rates (TDGR). The demand ratio is chosen indicator of short-term resilience 48 , and we generate the Tourism Demand Ratio (TDR) as the alternative dependent variable to test the robustness of conclusions. To illustrate the distribution of these dependent variables, Fig. 2 presents a histogram, kernel function density estimation plot, and maximum likelihood Gaussian distribution fit, providing a comprehensive view of the underlying data structure and the variability in Tourism Demand Growth Rates (TDGR) and Tourism Demand Ratio (TDR).
where i is a tourist origin city; t' is the current year (2022), and t is the control year (2021); TDGR i is the tourism demand variation of the city i; \({TD}_{i}^{t}\) is the sum of demand from city i in year t .

Statistical distribution analysis of TD, TDGR, and TDR variables. Notes: TD. is the Baidu brand index during the closure period, metric tourism demand, and the number in parentheses is the year; TDGR is the growth rates of tourism demand, and TDR is 2022 vs. 2021 ratio of tourism demand. The values of the above variables are processed by f = ln(x).
Determinants and proxy variables
The willingness of tourists to explore a destination depends on the determinants of the origin’s financial capacity, physical constraints, and psychological tendencies, in addition to the destination’s attractiveness. Furthermore, challenged by pandemic restrictions on mobility, we considered the origin markets’ epidemic circumstances and medical availability. We have selected the population, economics, environmental conditions, COVID-19, medical healthcare, Internet accessibility, and traffic activeness of the origin cities as potential determinants of tourism demand to analyze their impact on tourism demand changes, as detailed in Table 1 43 , 49 , 50 , 51 , 52 , 53 .
China has a substantial tourism market with spatially non-stationary development levels of cities in each region, and origins cities have significant spatial stratification heterogeneity. Accordingly, following large administrative geographical regions, we disaggregate the origin markets into East China, South China, Southwest China, North China, Northeast China, and Northwest China. The statistical data of each city are obtained from the National Bureau of Statistics ( http://www.stats.gov.cn/ ), and the epidemic data sources of COVID-19 are informed by the National Health and Health Commission and the provincial health and health commissions ( https://2019ncov.chinacdc.cn/2019-nCoV/ ). The advantages of this paper’s dataset are that it measures the seven explanatory variables mentioned above through twenty-five proxy variables, which captures the diversity of factors driving the tourism demand changes. Additionally, all the proxy variables will be standardized within large administrative geographical regions (Fig. 3 ), which helps to reveal significant differences in the explanatory variables performing distinct roles in different local regions.
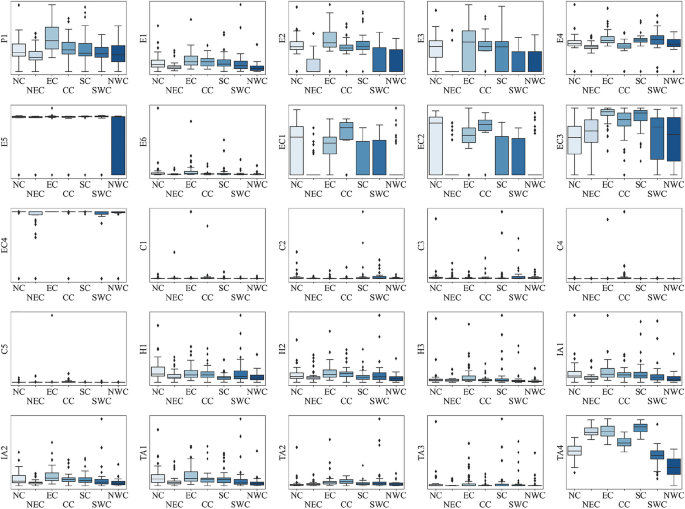
Proxy variable box plots group by regions. Notes: The labels of the X-axis, East China, South China, Southwest China, North China, Central China, Northeast China, and Northwest China are abbreviated as N.C., NEC, E.C., CC, SC, and NWC, respectively. The Y-axis labels are abbreviations for the proxy variables in Table 1 .
Research framework
The research framework, as illustrated in Fig. 4 , consists of three stages: temporal and spatial change analysis of tourism demand, exploratory regression analysis, and model evaluation.
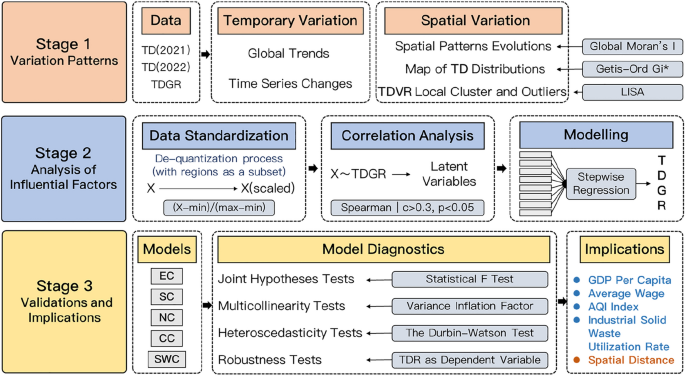
The research framework. Notes: TD-Tourism Demand; TDGR-Tourism Demand Growth Rates; LISA- Local Indicators of Spatial Association; EC, SC, NC, CC, SWC are the models in different regions (East China, South China, North China, Central China, and Southwest China) respectively.
The first stage involves depicting the spatial pattern of the Duku Road tourist origin markets and its changing process in the temporal dimension. The Global Spatial Autocorrelation method is applied to assess the spatial patterns of the origin markets, the Getis-Ord Gi* Hotspot Analysis method 54 detects the dominant origin market clusters, and the Local Spatial Autocorrelation Analysis method 55 is assigned to reveal clusters and outliers areas of tourism demand variation. The spatial distribution characteristics will map out the origin markets and pinpoint the dominant origin regions.
In the second stage, this paper uses an exploratory knowledge discovery strategy, i.e., finding the most significant drivers of each region among the many proxy variables that may impact tourism demand changes. Candidate proxies that are significantly correlated are initially filtered out from the Correlation Analysis between the proxies and explanatory variables. Then the candidate proxies are imported into a multi-round Linear Regression Analysis for iterative modeling and comparisons, thereby investigating the factors influencing the tourism demand variation in each region. Since the proxy variables in this paper are not entirely customarily distributed, Spearman’s Correlation Analysis 56 has the advantage of handling mixed data. The proxy variables with correlation coefficients greater than 0.3 and a significance over 95% will be considered candidate proxy variables. The Stepwise Regression Analysis 57 can perform multiple turns of regression analysis on candidate proxy variables and automatically remove the insignificant variables, resulting in the exploration of the model with the optimal performance with significance.
The third stage is the diagnostic evaluation and robustness testing of the model. Multiple linear regression, autocorrelation, normality of residuals, and eteroscedasticity are used to diagnose the model’s performances 57 . We compare the conclusions obtained from Stepwise Regression Analysis with the results obtained by substituting the dependent variable to evaluate the validity of the conclusions. The above experiments will be replicated with the ratio of tourism demand during the destination closure in 2022 to the corresponding period in 2021 as the dependent variable, and the conclusions will be considered dependable and robust in case of substantial consistency.
Spatial and temporal changes in origin markets demand
The paper utilizes the Baidu search engine to garner search records of the Duku Road in China over the past decade (Fig. 5 a). Results indicate a rapid surge in the attraction of the Duku Road since 2015, peaking in 2019. Despite the impact of COVID-19 in 2020, the demand for tourism quickly rebounded, hitting a new high in 2022. It is imperative to note that due to safety risks associated with the Duku Road, it is only accessible to tourists from May to October every year. During this period, the tourism demand for Duku Road exhibits a primary and secondary peak, with a critical point at the main peak, dividing this phase into a rising and a declining period. Specifically, the rising period commences in early May, peaking towards the end of July, followed by a continual decline. However, a second surge forms during the National Day Golden Tourism Week, which subsequently dwindles gradually.
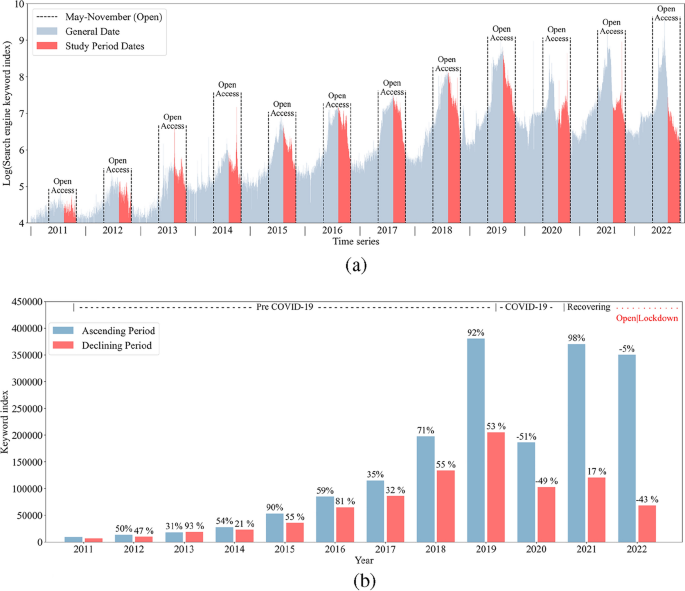
Variation of Baidu index for Duku Road (2011–2022). ( a ) The daily Baidu Index trend for Duku Road (2011–2022). ( b ) The Baidu Index during the years of unrestricted access for The Duku Road (2011–2022).
Considering a sudden outbreak of COVID-19 across Xinjiang, local authorities initiated a silent management policy, imposing traffic restrictions from August 10 to October 31. According to historical time series characteristics of the Duku Road, this time point coincides closely with the critical date when the tourism demand transitions from a peak to a decline. A comparison of the keyword index during the rising and declining periods of each year reveals that the tourism demand maintained a high level before the travel restrictions, while the destination closure significantly impacted the tourism demand, causing a sharp decline (Fig. 5 b).
Although post August 10, 2022, marked the declining phase of tourism demand for the Duku Road, the Baidu index for the keyword “Duku Road” remained high (only a 5% decrease compared to the same period in 2021) before August 10, 2022. This suggests a stable tourism demand for the Duku Road prior to the pandemic closure. However, post August 10, the Baidu index plummeted by 43% compared to the same period in 2021, inferring a direct impact on the tourism demand for the Duku Road due to the outbreak of COVID-19 in Xinjiang and the traffic control measures. Noteworthy is that despite the inability to conduct any tourism activities in Xinjiang post August 10, the source market maintained a robust potential tourism demand for the Duku Road from August 10 to October 31. During the COVID-19 outbreak and lockdown in Xinjiang, the tourism demand from seven administrative regions in China for the Duku Road grew by an average of 31.49% compared to 2021, indicating a strong resilience in the tourism demand for the Duku Road. This reflects that despite the restrictions imposed by the pandemic and traffic control policies, the restrained tourism demand will gradually be released once the situation is under control, highlighting a significant potential for recovery.
Based on the spatial autocorrelation analysis results (Table 2 ), the tourism demand from the source market demonstrates a significant spatial clustering pattern, with the spatial pattern of the Duku Road’s source market transitioning from a low level to a moderate level of spatial clustering. The spatial distribution of tourism demand from source cities to the Duku Road has been impacted by the pandemic crisis, exhibiting a trend of contraction and clustering towards core source cities.
Viewed from the perspective of source cities, this section utilizes the Jenks Natural Breaks method to categorize source cities into five groups based on the intensity of tourism demand towards Duku Road and constructs a spatial distribution map of tourism demand from the source market. The map employs a color gradient from blue to red to represent the intensity of tourism demand from source cities to the Duku Road, where red and orange cities signify core and secondary source cities, respectively. The domestic source market is primarily distributed in the developed cities in eastern China. In Fig. 6 a,b, cities like Beijing and Shanghai are identified as core source cities, while Chengdu, Chongqing, Hangzhou, and Guangzhou are recognized as secondary source cities. A comparison between Fig. 6 a,b reveals that cities like Lanzhou, Xi’an, and Nanjing no longer serve as secondary source locations, indicating that some secondary source cities are more sensitive to the tourism demand for Duku Road under Xinjiang pandemic crisis than others, which exhibiting a variability within the secondary source market.
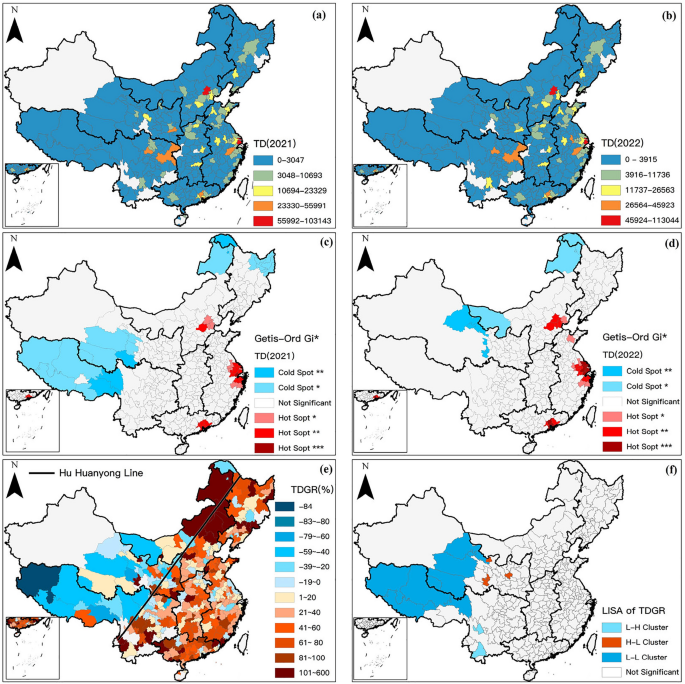
The spatial patterns of tourism demand and its' variation. Notes: Hu Huanyong Line in ( e ) is a comparison divider proposed by Chinese geographer Hu Huanyong (1901–1998) in 1935 to delineate the population density of China, which has significant heterogeneity in the population, society, and economy between the east and west of the line.
Upon further hotspot analysis of the source market, the results exhibit significant statistical clustering in the spatial domain for the Duku Road’s source market. As depicted in Fig. 6 c,d, the red zones represent the hotspots of tourism demand, encompassing the Jing-Jin-Ji region, Yangtze River Delta, and the Pearl River Delta, which emerge as the most crucial source markets with lesser impact from the pandemic crisis on Duku Road. On the other side, the blue zones signify the cold spots of tourism demand, where cities within these regions exhibit an exceedingly low tourism demand towards the Duku Road. A comparative inspection of Fig. 6 c,d unveils a shift in some cold spot areas (for instance, the disappearance of the cold spot on the Qinghai-Tibet Plateau, and the emergence of a new cold spot area north of the Hexi Corridor), while the stability of cold spot regions in Northeast China remains high (such as Hulunbuir and Daxing’anling area).
This section analyzes the growth rate of tourism demand, designating cities with growth rates below zero with a gradient of cool colors (blue), and those with growth rates above zero with a gradient of warm colors (red), with intervals of (±) 20%. As shown in Fig. 6 e, cities with a growth rate exceeding 100% are rendered in deep red. There is a significant positive correlation in the spatial distribution of tourism demand growth rates, indicating neighboring cities share a consistent response pattern to the pandemic. A distinct contrast is formed on either side of the Hu Huanyong Line; source markets to the east primarily exhibit positive growth rates, while those in the western regions display negative growth rates. Analysis of local clustering and outliers reveals the formation of three distinct local clusters in the spatial distribution of tourism demand growth rates (Fig. 6 f). Cities near the Qinghai-Tibet Plateau and Hexi Corridor in Northwestern China respond strongly to the pandemic crisis, with rapid declines in tourism demand towards Duku Road in this area (L–L). A sporadic distribution of outlier cities (H–L) emerges on the eastern side of Northwestern China, exhibiting superior risk resilience and higher tourism demand compared to other local areas. In Southwest China, Yunnan province has higher demand growth, with Pu’er and Lijiang being typical tourist cities. The fluctuations in their demand are impacted by their respective tourism industries, exhibiting a phenomenon where they are encircled by proximate cities experiencing high demand growth rates (L–H).
By dividing the source market according to administrative regions, summing up the tourism demand from cities within each administrative region to Duku Road, and averaging the growth rate of tourism demand, regional differences are identified. As shown in Fig. 7 , a comparison of tourism demand scale and growth between 2021 and 2022 across administrative regions is made. Using the Jenks Natural Breaks method, they are categorized into high, medium, and low levels. This corresponds to East China being the primary source market for Duku Road, while North, Central, South, Southwest China are secondary source markets, and Northeast and Northwest China are potential source markets. Northeast and South China have the highest growth rates in tourism demand, followed by North, Central, and East China, with Southwest and Northwest China having the lowest. There is minimal variation in the scale of tourism demand from cities within each region to Duku Road, but a larger difference in the growth rates of tourism demand.
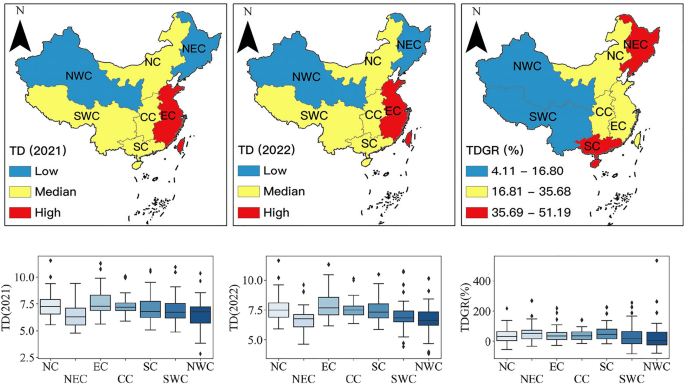
The maps with box plots of the tourism demand and growth rates.
Correlation analysis for TDGR
The correlation between explanatory variables and the growth rate of tourism demand significantly varies across different regions, suggesting that a more nuanced analysis could be obtained by partitioning the source market for modeling. In the South China region, 64% of the explanatory variables are negatively correlated with the growth rate of tourism demand, while in the Northwest region, no explanatory variables exhibit significant correlation. In the Northeast region, only two explanatory variables are negatively correlated with the tourism demand growth rate. In East China, Southwest, North China, and Central China, about one-third of the explanatory variables show a correlation with tourism demand growth rate at a significance level better than 0.05.
The correlation results indicate that under the “dynamic zeroing” policy control, new cases in source areas do not correlate with the potential tourism demand growth rate of residents. For instance, the numbers of newly confirmed and asymptomatic cases only show a negative correlation with the tourism demand growth rate in the South and Southwest regions of China. The relationship between the number of COVID-19 recovered patients and the tourism demand growth rate differs between the southern and northern regions, showing a weak positive correlation in the Southwest region and a weak negative correlation in the North China region. Notably, a significant moderate negative correlation exists between public medical resources of local source cities and the tourism demand growth rate. In the post-pandemic era, factors related to tourism demand growth rate are constantly changing, and the relationship between influential factors and tourism demand growth rate shows spatial non-stationarity across different regions.
Significant explanatory variables in the East China region include per capita disposable income, average wages, consumer price index, savings deposits, the number of people covered by basic medical insurance, and highway passenger traffic. In South China, the variables include the resident population, per capita GDP, per capita disposable income, per capita consumer spending, savings deposits, PM2.5 concentration, AQI index, newly confirmed cases, newly confirmed asymptomatic cases, the number of hospitals, hospital bed count, basic medical insurance coverage, mobile phone user count, broadband access user count, private car ownership, and civil aviation passenger traffic. In Southwest, the variables include per capita GDP, infection count, newly confirmed cases, newly confirmed asymptomatic cases, recoveries, private car ownership, highway passenger traffic, and spatial distance. In North China, significant variables include savings deposits, industrial solid waste utilization rate, recoveries, mobile phone user count, broadband access user count, and private car ownership. In Central China, the variables include per capita GDP, savings deposits, AQI index, hospital bed count, basic medical insurance coverage, mobile phone user count, broadband access user count, private car ownership, and spatial distance. In Northeast, the significant variables are average wages and savings deposits. In Northwest, no significant explanatory variables have statistically meaningful correlation with tourism demand growth rate.
Stepwise regressions
Diverging from studies that directly engage in regression analysis with correlation tests 6 , this chapter adopts a stepwise regression modeling approach. It sequentially incorporates significant explanatory variables from Table 3 (highlighted in bold) for each region into the model to explore influential factors under optimal fitting circumstances. However, the results of stepwise regression analysis reveal that in the Northeast and Northwest regions (Table 4 ), due to a limited number of significant explanatory variables and a lack of significant linear relationships between these variables and the dependent variable, no effective models are obtained. In other regions, there is a noticeable disparity between influential factors and model fit.
In East China, average wages are the sole factor affecting the growth rate of tourism demand, albeit with weak explanatory power (R 2 = 0.08). In South China, the AQI index exerts a negative impact on the tourism demand growth rate, contributing to 17.9% of the variance explanation. In North China, the growth rate of tourism demand is negatively affected by the industrial solid waste utilization rate, with an explanatory power of 17.8%. In Central China, per capita GDP is a significant factor negatively impacting the tourism demand growth rate. In the Southwest region, the tourism demand growth rate is influenced by multiple factors, where spatial distance has a positive impact, and per capita GDP has a significant negative impact. Combined, they account for 42% of the explanatory power concerning the growth rate of tourism demand.
In summation, the growth rate of tourism demand in source markets chiefly hinges on local economic, environmental, and transportation factors. Although there is a significant weak correlation between the local pandemic situation and the tourism demand growth rate in certain areas, it fails to exert a statistically significant impact on the local tourism demand towards the Duku Road.
The efficacy of the models has been corroborated by the diagnostic items and parameters delineated in Table 5 . The results indicate that the models passed the F-test (p < 0.05), implying that the independent variables in each model significantly affect the dependent variable TDGR, denoting the models are meaningful. With a limited number of dependent variables in the models, the VIF (Variance Inflation Factor) values are well below 5, suggesting that there is no multicollinearity issue, and the models are well-constructed.
In the autocorrelation test of the models, the D-W (Durbin-Watson) value of the model for the South China region deviates significantly from 2 (1.508 < 1.7), indicating that the significance testing and goodness of fit for this model would be unreliable; hence, this model failed the validation, while the other models are deemed credible. Moreover, this section re-models using TDR from Sect. 7.2.2 as an alternative dependent variable following the framework procedure. The results of the new model are consistent with the conclusions previously obtained, eventually leading to linear regression models for the tourism demand growth rate in four regions.
Factors affecting TDGR
The response to the changes in tourism demand for the Duku Road during the pandemic period is manifested through the tourism demand growth rate of the source cities. The results of the regional model construction discussed earlier (see section " Stepwise regressions ") indicate that the factors affecting the growth of tourism demand for the Duku Road vary significantly across different regions. As shown in Fig. 8 , on a national scale, the models for the North China, Central China, East China, and Southwest regions have all passed the significance test and robustness test. Each model corresponds to a subplot, with the title of the subplot being the model formula for the respective region, and the content of the subplot displaying the spatial distribution of the tourism demand growth rate and explanatory variables. All indicators are divided into five intervals using the Jenks Natural Breaks classification method, with colors from blue to red representing intervals from low to high.
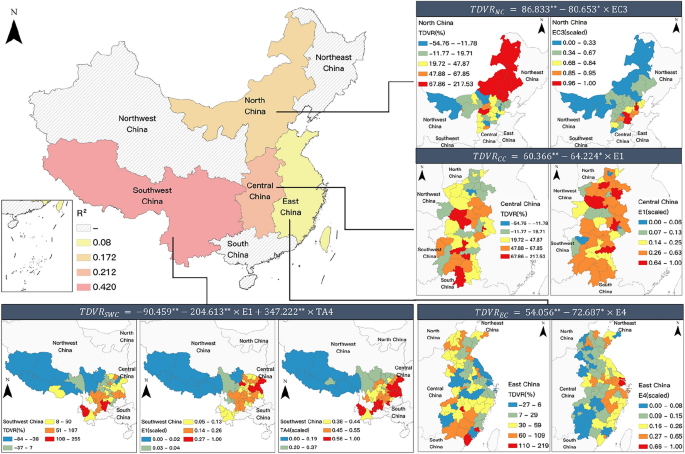
The influential factors models of response heterogeneity of tourist origin markets to Duku Road.
Upon comparing the similarities and differences across regional models, it is found that during the pandemic period in Xinjiang, the primary factors influencing the response differences to destination lockdowns in the domestic source markets for the Duku Road include Per Capita GDP, average wages, industrial solid waste utilization rate, and spatial distance. Economic factors have a counteractive effect on the tourism demand growth of the source cities, an impact widely present in East China, Central China, and Southwest regions, exhibiting robust spatial consistency. Notably, environmental factors indicate that the growth of tourism demand is constrained by the industrial solid waste utilization rate, a significant relationship found only in North China. This section observes that in industrially prosperous areas within this region (Hebei-Tianjin), the growth of tourism demand is limited, or even decreased, whereas in remote areas with lower industrial levels (Inner Mongolia), the tourism demand is not high, but generally has a high rate of growth. The significant positive impact produced by the distance factor in the Southwest region can be attributed to the distinct differences between the Qinghai-Tibet Plateau and the Sichuan-Chongqing-Yunnan-Guizhou Plateau; the former has low levels of tourism demand and growth rate, while the latter has relatively higher tourism demand and growth rate, thereby leading to a higher tourism demand growth response in source cities farther from the Duku Road.
Factors influencing tourism demand towards Duku road
Understanding the factors influencing the tourism demand from source cities to Duku Road is crucial for grasping the heterogeneity in tourism demand growth rates. This section employs a loop iteration approach to individually examine the linear fit between twenty-five explanatory variables of source cities within seven administrative regions, and the tourism demand scale of these cities towards Duku Road, to investigate the factors affecting the tourism demand scale of source cities to Duku Road. From a total of 175 linear models, eight models were identified as statistically significant and demonstrating a superior degree of fit ultimately. These models, with a significance level exceeding 0.01 and an R 2 value greater than 0.8, effectively explain the scale of tourism demand across more than four administrative regions.
As illustrated in Table 6 , different variables significantly positively impact the demand for tourism to the Duku Road across various regions. The rise in the number of broadband and mobile phone users suggests more individuals have mobile communication capabilities, enhancing the accessibility to information regarding Duku Road. This improved digital outreach fosters the online visibility and awareness of the destination, consequently boosting the local residents’ desire to travel there. Savings deposits reflect the economic capacity of individuals or families in the source cities, laying the foundation for affording travel to Duku Road. A higher per capita Gross Domestic Product indicates a relatively higher income level and consumer spending capacity, enabling more discretionary income for long-distance travel expenditures. The economic stature of the source cities implies a higher preference for tourism and a more open cultural backdrop, collectively fostering an increased interest and demand for traveling to Duku Road. An augmentation in the number of individuals covered by basic medical insurance and the quantity of hospital beds signifies enhanced medical security for more residents. Better medical services and emergency response capabilities help mitigate the impact of local epidemics on residents’ travel activities. Simultaneously, it alleviates tourists’ concerns about the travel risks and uncertainties associated with the epidemic situation in Xinjiang, bolstering their confidence in traveling to Duku Road. Higher air passenger traffic denotes more flight connections between the residents and the destination, supporting the accessibility for inland tourists to Duku Road. Elevated private car ownership in source cities inclines residents towards choosing self-driving tours to Duku Road, offering greater flexibility, freedom, comfort, and convenience. This mode of travel satisfies tourists’ desire to explore the scenic routes of Duku Road and indulge in personalized experiences.
In summary, determinants such as the extent of internet coverage, economic conditions, medical security, and transportation facilities in the source markets influence the residents’ demand for tourism to the Duku Road in various aspects. The extent of internet coverage lays the foundation for environmental awareness of the Duku Road among residents of the source cities; whereas economic conditions are essential for residents to travel to the Duku Road. Amid an ongoing pandemic, medical security in the source cities can mitigate the adverse effects of the local epidemic on residents’ travel behaviors; transportation conditions provide diversified accessibility for the residents of the source cities. In the source markets of the Duku Road, areas with a large scale of mobile phone users, high residents’ savings, comprehensive medical insurance coverage, and developed passenger aviation contribute the most to the tourism demand to the Duku Road. This conclusion aligns with the spatial pattern of tourism demand discussed in section " Spatial and temporal changes in origin markets demand ". As illustrated in Fig. 9 , box plots of the number of mobile phone users (IA1), savings deposits (E6), the number of individuals covered by basic medical insurance (H3), and the volume of civil aviation passengers (TA3) demonstrate that the Eastern China region ranks highest in these four indicators nationwide, and concurrently, it has the highest demand for tourism to the Duku Road. Furthermore, summarizing the determinants in Table 6 and arranging them in descending order based on the average R 2 reveals that the economic conditions > transportation accessibility > medical conditions > extent of internet coverage, indicating that economic conditions are the most crucial determinant affecting the scale and spatial pattern of tourism demand in the source markets.
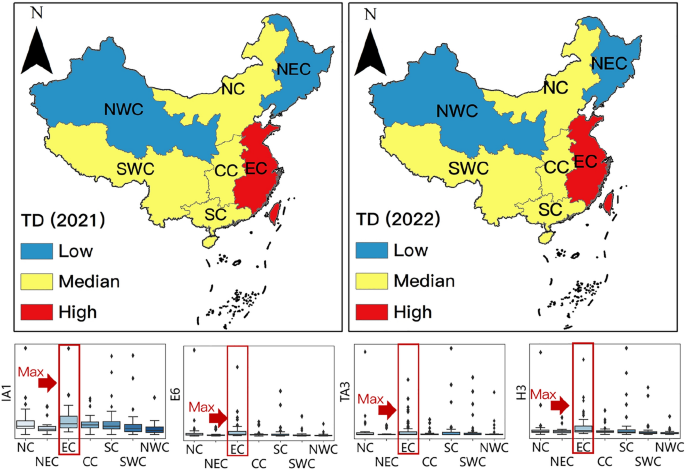
Tourism origins market maps corresponding with boxplots of variables in regions.
The competitive resilience of core tourist attractions
The study unveils a nuanced understanding of tourism demand dynamics, particularly illuminating the interplay between spatial structures of source markets and economic factors amidst unprecedented health crises such as COVID-19. Even during the crisis, the potential demand from tourist origin markets for a leading tourist attraction continues to be remarkably high and will have considerable potential to counteract the crisis in the post-pandemic era 11 . By delving into the spatial patterns and demand determinants for Duku Road in Xinjiang during the epidemic silent management period, the study extrapolates the driving forces behind the resilience and demand sustenance of core tourism attractions.
Firstly, the notable positive spatial autocorrelation within Duku Road’s source markets elucidates a significant conceptual insight: geographically proximate source cities harbor similar tourism demands. The absence of a Matthew Effect, and the ensuing market dispersion and diversity, underscores Duku Road’s ability to allure visitors from a broad geographic spectrum, thereby diluting over-reliance on singular markets. This diversification not only embodies adaptive capacity to navigate tourism adversities but also buffers the blow from crises by enabling a continuum of demand from unaffected markets. The relative unscathed potential tourism demand during the epidemic crisis manifests the merits of such market diversification.
Secondly, the decisive role of economic factors extends beyond merely shaping the tourism demand from tourist markets towards Duku Road; it critically impacts the spatial configuration of its source markets 58 . The stratified heterogeneity in crisis response, with economically robust core source cities demonstrating stability versus the sensitivity of secondary source cities with moderate economic levels, unveils an inherent inertia in the spatial structure steered by economic dynamics. This is a salient contribution to understanding the spatial-economic nexus in tourism demand studies.
Lastly, the regional variance captured through sectional models divulges that core source cities with high economic indicators face a saturation effect in tourism demand scale for Duku Road, contrasting with potential source cities that possess a larger scope for escalating tourism demand growth rates. It also identifies regional differences in the factors influencing tourism demand variations 19 , 59 , 60 . This saturation effect, negatively impacting tourism demand growth rates, accentuates the regional disparities in factors influencing tourism demand variations. The knowledge will help to provide insights into the influential factors driving tourism demand variations in distinct regions 59 .
In synthesis, the resilience of core tourism attractions within a region is constructed on a quadrate foundation: the uniqueness of tourism experience, market dispersion, heterogeneity in crisis response, and spatial structural stability induced by economic factors in source cities. Post-crisis, core attractions sustain a substantial scale of potential tourism demand, with economic factors significantly steering the tourism demand scale, leading to a spatial structural inertia towards core cities. The marked discrepancies in response patterns among source cities, tied to the saturation effects on local economic factors, spotlight the imperative of diversifying source market risks to accrue potential tourism demand, thereby accelerating recovery and sustaining competitive edge post-crisis.
These insights are seminal for policymakers and industry stakeholders to craft efficacious strategies for mitigating crises impacts, fostering tourism recovery, and bolstering resilience. The discourse propels a deeper comprehension of the factors instigating regional variances in tourism demand, furnishing a robust theoretical scaffold for future empirical explorations in the realm of tourism demand studies amidst crises. This narrative, therefore, extends a substantial theoretical contribution towards understanding the ramifications of COVID-19 on tourism demand, while amplifying the criticality of domestic demand in substituting the downturn in international tourism, thereby laying a robust groundwork for successful post-crisis recovery and long-term resilience in regional tourism sectors.
Limitations and prospects
The limitation in dataset is that the dependent variable does not measure the actual visits, revenues, or employment in tourism in the destination area. Instead, it measures Internet searches for a particular attraction. As Internet interest is indeed correlated with actual trips, the possibility of inferring short-term variations in demand on the search alone is limited. Regarding our emphasis on core tourist attractions within a regional destination has inadvertently disregarded the vulnerabilities of a broader array of regional attractions. Forthcoming studies will encompass an evaluation of the comprehensive repercussions that tourism crises exert on the destination system, alongside an analysis of the source market’s reactions. Additionally, studies will classify tourist attractions by their level or type and will subsequently discuss the risk resilience and the mitigating strategies employed for each group of tourist attractions. An integrated and differentiated methodology such as this promises to yield a more systematic understanding, benefiting the strategic planning and operational decisions of tourism management entities.
In contemplating future research directions, several potential areas warrant further investigation. Initially, a novel comprehensive indicator should be generated for monitoring tourism demand by integrating search data, user-generated content on social media platforms, and statistical data. This integration would avoid the original error in sample coverage of the source market due to internet access disparities. Furthermore, expanding the research framework to encompass additional destinations and broader dimensional factors, and employing mixed-effect models to study the overall impact of these factors on tourism demand, would yield a more holistic understanding of tourism demand dynamics during crisis situations. Additionally, examining the economic downturn in the post-epidemic era, with a particular focus on the influence of decreased travel intentions following the lifting of travel restrictions and the subsequent general reopening, could offer crucial insights into the resilience of various destinations and origin markets. Lastly, an in-depth analysis of the long-term consequences of crisis events on tourism demand, as well as the recovery trajectories of destination and origin markets, would be instrumental in providing essential guidance for policymakers and industry planners aiming to mitigate the adverse effects of crises on the tourism sector.
Conclusions
This inquiry delved into the dynamics of potential tourism demand from China's domestic origin markets towards Duku Road in Xinjiang during the epidemic closure in August 2022. The exploration unfolded heterogeneous response patterns of tourism demand in the face of unexpected epidemic crises, particularly accentuating the post-epidemic era. The study homed in on the impact of demographic, economic, environmental, epidemic, medical, digital, and transportation facets on local tourism demand fluctuations within the origin markets, encompassing 308 cities. Utilizing spatial statistical and stepwise regression analyses, the investigation spotlighted spatial disparities in tourism demand variation rates across seven major regions.
A salient revelation is the altered response patterns of origin markets to epidemic crises at destinations, transcending the initial reactions during the 2019 onset of COVID-19. There is a notable uptick in tourism demand gravitating towards primary origins. While local epidemics and medical care in origins correlate with tourism demand variations, they do not forge a meaningful relationship. Economic determinants emerge as dominant negative influencers, with tangible regional disparities in factors affecting local tourism demand rates. Economically affluent regions surface as the core tourist origin markets, exhibiting resilience in tourism demand amidst destination tourism crises. Concurrently, core tourist-origin areas with high economic indicators appear to reach a saturation point, curtailing the growth rate of tourism demand. Conversely, potential tourist origin markets highlight significant variability with a pronounced growth potential, revealing a correlation with distance and an inverse relationship with industrial solid waste utilization rate.
Central to the findings above is the competitive resilience of core tourism attractions like Duku Road, demonstrated by its ability to maintain a stable tourism demand even amidst adversities such as the epidemic crisis. Firstly, the study demonstrates that core tourist attractions within destination systems can accumulate potential tourism demand during crises through their intrinsic appeal and diversified market structure. This accumulated demand provides substantial momentum for recovery, highlighting the importance of maintaining and enhancing the attractiveness of core tourism assets to sustain potential tourism demand. Secondly, core tourist attractions serve as both growth poles within destination systems and recovery nodes for tourism revival. The spatial differentiation and clustering of tourism demand driven predominantly by economic factors within origin markets contribute significantly to the risk resilience of core attractions. These factors suggest that economically robust regions can act as stable sources of tourism demand, reinforcing the importance of understanding and leveraging economic conditions in origin markets to enhance the resilience and competitive edge of core attractions.
The insights are pivotal for stakeholders aiming to design efficacious strategies to navigate through crises, promoting tourism recovery and resilience, thereby maintaining the competitive edge of core tourist attractions, and regional tourism sustainability by strategies as followed: (1) Destination management organizations should focus on diversifying their market structures to include a mix of economically strong and emerging markets to buffer against localized crises. (2) Continuous monitoring of economic indicators in origin markets can provide early warnings and strategic insights for tourism demand management, allowing for more resilient destination planning. (3) Policymakers should prioritize strategies that enhance the core attractiveness of key tourism assets while simultaneously fostering diversified and resilient origin markets to safeguard against potential crises.
Data availability
The raw datasets utilized in this study can be accessed through the Baidu search engine at https://index.baidu.com and the National Bureau of Statistics of China at http://www.stats.gov.cn/ . The processed datasets that were used and analyzed during this research are available from the corresponding author upon reasonable request.
Mariolis, T., Rodousakis, N. & Soklis, G. The COVID-19 multiplier effects of tourism on the Greek economy. Tour. Econ. 27 , 1848–1855 (2021).
Article Google Scholar
UNWTO. The Economic Contribution of Tourism and the Impact of COVID-19 . (World Tourism Organization (UNWTO), 2021). https://doi.org/10.18111/9789284423200 .
UNWTO. World Tourism Barometer and Statistical Annex, September 2022. UNWTO World Tour. Barom. Engl. Version 20 , 1–40 (2022).
UNWTO. World Tourism Barometer and Statistical Annex, November 2021. UNWTO World Tour. Barom. Engl. Version 19 , 1–36 (2021).
Dolnicar, S. & McCabe, S. Solidarity tourism how can tourism help the Ukraine and other war-torn countries?. Ann. Tour. Res. 94 , 103386 (2022).
Boto-Garcia, D. & Mayor, M. Domestic tourism and the resilience of hotel demand. Ann. Tour. Res. 93 , (2022).
Cai, J. et al. Modeling transmission of SARS-CoV-2 Omicron in China. Nat. Med. 28 , 1468–1475 (2022).
Article CAS PubMed PubMed Central Google Scholar
Han, L., Goetz, S. J., Eades, D., Entsminger, J. & Arbogast, D. An early assessment of COVID-19’s impact on tourism in U.S. counties. Tour. Econ. 13548166221107814. https://doi.org/10.1177/13548166221107814 (2022).
Pham, T. D., Dwyer, L., Su, J.-J. & Ngo, T. COVID-19 impacts of inbound tourism on Australian economy. Ann. Tour. Res. 88 , 1 (2021).
Abbas, J., Mubeen, R., Iorember, P. T., Raza, S. & Mamirkulova, G. Exploring the impact of COVID-19 on tourism: Transformational potential and implications for a sustainable recovery of the travel and leisure industry. Curr. Res. Behav. Sci. 2 , 100033 (2021).
Article PubMed PubMed Central Google Scholar
Okafor, L. E., Khalid, U. & Burzynska, K. Does the level of a country’s resilience moderate the link between the tourism industry and the economic policy response to the COVID-19 pandemic?. Curr. Issues Tour. 25 , 303–318 (2022).
Adamiak, C. Current state and development of Airbnb accommodation offer in 167 countries. Curr. Issues Tour. 25 , 3131–3149 (2022).
OECD. OECD Tourism Trends and Policies 2020 . (OECD, 2020). https://doi.org/10.1787/6b47b985-en .
Sharma, G. D., Thomas, A. & Paul, J. Reviving tourism industry post-COVID-19: A resilience-based framework. Tour. Manag. Perspect. 37 , 100786 (2021).
PubMed Google Scholar
Arbulú, I., Razumova, M., Rey-Maquieira, J. & Sastre, F. Measuring risks and vulnerability of tourism to the COVID-19 crisis in the context of extreme uncertainty: The case of the Balearic Islands. Tour. Manag. Perspect. 39 , 100857 (2021).
PubMed PubMed Central Google Scholar
Polemis, M. L. National lockdown under COVID-19 and hotel performance. Ann. Tour. Res. Empir. Insights 2 , 100012 (2021).
Ozdemir, O., Dogru, T., Kizildag, M., Mody, M. & Suess, C. Quantifying the economic impact of COVID-19 on the U.S. hotel industry: Examination of hotel segments and operational structures. Tour. Manag. Perspect. 39 , 100864 (2021).
Yang, Y., Liu, H. & Chen, X. COVID-19 and restaurant demand: Early effects of the pandemic and stay-at-home orders. Int. J. Contemp. Hosp. Manag. 32 , 3809–3834 (2020).
Watson, P. & Deller, S. Tourism and economic resilience. Tour. Econ. 28 , 1193–1215 (2022).
Fotiadis, A., Polyzos, S. & Huan, T.-C.T.C. The good, the bad and the ugly on COVID-19 tourism recovery. Ann. Tour. Res. 87 , 103117 (2021).
Article PubMed Google Scholar
Orden-Mejía, M. et al. Post-COVID-19 tourists’ preferences, attitudes and travel expectations: A study in Guayaquil, Ecuador. Int. J. Environ. Res. Public. Health 19 , 4822 (2022).
Zhang, H., Song, H., Wen, L. & Liu, C. Forecasting tourism recovery amid COVID-19. Ann. Tour. Res. 87 , 1 (2021).
Angulo, A. M., Mur, J. & Trívez, F. J. Measuring resilience to economic shocks: An application to Spain. Ann. Reg. Sci. 60 , 349–373 (2018).
Ezcurra, R. & Rios, V. Quality of government and regional resilience in the European Union: Evidence from the Great Recession. Pap. Reg. Sci. 98 , 1267–1290 (2019).
Bernini, C., Cracolici, M. F. & Nijkamp, P. Micro and macro resilience measures of an economic crisis. Netw. Spat. Econ. 20 , 47–71 (2020).
Romao, J. Tourism, smart specialisation, growth, and resilience. Ann. Tour. Res. 84 , 1 (2020).
Brouder, P. et al. Reflections and discussions: Tourism matters in the new normal post COVID-19. Tour. Geogr. 22 , 735–746 (2020).
Nepal, S. K. Adventure travel and tourism after COVID-19—business as usual or opportunity to reset?. Tour. Geogr. 22 , 646–650 (2020).
Benjamin, S., Dillette, A. & Alderman, D. H. “We can’t return to normal”: Committing to tourism equity in the post-pandemic age. Tour. Geogr. 22 , 476–483 (2020).
Rowen, I. The transformational festival as a subversive toolbox for a transformed tourism: lessons from Burning Man for a COVID-19 world. Tour. Geogr. (2020).
Yang, E. & Smith, J. W. The spatial and temporal resilience of the tourism and outdoor recreation industries in the United States throughout the COVID-19 pandemic. Tour. Manag. 95 , 104661 (2023).
Bangwayo-Skeete, P. F. & Skeete, R. W. Modelling tourism resilience in small island states: A tale of two countries. Tour. Geogr. 23 , 436–457 (2021).
Khan, A., Bibi, S., Lyu, J., Latif, A. & Lorenzo, A. COVID-19 and sectoral employment trends: Assessing resilience in the US leisure and hospitality industry. Curr. Issues Tour. 24 , 952–969 (2021).
Torres, A. P., Marshall, M. I. & Sydnor, S. Does social capital pay off? The case of small business resilience after Hurricane Katrina. J. Contingencies Crisis Manag. 27 , 168–181 (2019).
Cutter, S. L. The landscape of disaster resilience indicators in the USA. Nat. Hazards 80 , 741–758 (2016).
Jang, S., Park, J. S. & Choi, Y. T. Organizational resource and resilience in tourism. Ann. Tour. Res. 93 , 103322 (2022).
Yang, E., Kim, J., Pennington-Gray, L. & Ash, K. Does tourism matter in measuring community resilience?. Ann. Tour. Res. 89 , 103222 (2021).
Fesenmaier, D. R., Xiang, Z., Pan, B. & Law, R. An analysis of search engine use for travel planning. In Information and Communication Technologies in Tourism 2010 (eds. Gretzel, U., Law, R. & Fuchs, M.) 381–392 (Springer Vienna, Vienna, 2010).
Choi, H. & Varian, H. Predicting the present with google trends. Econ. Rec. 88 , 2–9 (2012).
Li, X., Pan, B., Law, R. & Huang, X. Forecasting tourism demand with composite search index. Tour. Manag. 59 , 57–66 (2017).
Song, H., Qiu, R. T. R. & Park, J. Progress in tourism demand research: Theory and empirics. Tour. Manag. 94 , 104655 (2023).
Yang, X., Pan, B., Evans, J. A. & Lv, B. Forecasting Chinese tourist volume with search engine data. Tour. Manag. 46 , 386–397 (2015).
Ma, X., Yang, Z. & Zheng, J. Analysis of spatial patterns and driving factors of provincial tourism demand in China. Sci. Rep. 12 , 2260 (2022).
Article ADS CAS PubMed PubMed Central Google Scholar
Gössling, S., Scott, D. & Hall, C. M. Pandemics, tourism and global change: A rapid assessment of COVID-19. J. Sustain. Tour. 29 , 1–20 (2021).
Polyzos, S., Samitas, A. & Spyridou, A. Tourism demand and the COVID-19 pandemic: An LSTM approach. Tour. Recreat. Res. 46 , 175–187 (2021).
Google Scholar
Ma, X., Han, F., Wang, T., Fan, S. & Ma, L. Detecting potential cooperative network for tourist attractions in a destination using search data. PLOS ONE 19 , e0298035 (2024).
Cellini, R. & Cuccia, T. The economic resilience of tourism industry in Italy: What the ‘great recession’ data show. Tour. Manag. Perspect. 16 , 346–356 (2015).
Duro, J., Perez-Laborda, A. & Fernandez, M. Territorial tourism resilience in the COVID-19 summer. Ann. Tour. Res. Empir. Insights 3 , 100039 (2022).
Dritsakis, N. Tourism development and economic growth in seven mediterranean countries: A panel data approach. Tour. Econ. 18 , 801–816 (2012).
Eugenio-Martin, J. L., Martín-Morales, N. & Sinclair, M. T. The role of economic development in tourism demand. Tour. Econ. 14 , 673–690 (2008).
Falk, M., Hagsten, E. & Lin, X. Uneven domestic tourism demand in times of pandemic. Tour. Econ. 15 , 94. https://doi.org/10.1177/13548166211059409 (2022).
Martins, L. F., Gan, Y. & Ferreira-Lopes, A. An empirical analysis of the influence of macroeconomic determinants on World tourism demand. Tour. Manag. 61 , 248–260 (2017).
Wang, Y.-S. The impact of crisis events and macroeconomic activity on Taiwan’s international inbound tourism demand. Tour. Manag. 30 , 75–82 (2009).
Getis, A. & Ord, J. K. The analysis of spatial association by use of distance statistics. In Perspectives on Spatial Data Analysis (eds. Anselin, L. & Rey, S. J.) 127–145 (Springer, Berlin, Heidelberg, 2010). https://doi.org/10.1007/978-3-642-01976-0_10 .
Anselin, L. Spatial econometrics: Methods and models Vol. 4 (Springer, 1988).
Book Google Scholar
Spearman, C. The proof and measurement of association between two things. Int. J. Epidemiol. 39 , 1137–1150 (2010).
Article CAS PubMed Google Scholar
Hocking, R. R. A Biometrics invited paper: The analysis and selection of variables in linear regression. Biometrics 32 , 1–49 (1976).
Article MathSciNet Google Scholar
Tang, C. F., Law, S. H. & Harun, M. Demand for inbound educational tourism: a note on relative differences and non-linear relationships. Curr. Issues Tour. 1 , 1–6 (2022).
Almeida, A., Golpe, A. & Justo, R. Regional tourist heterogeneity in Spain: A dynamic spatial analysis. J. Destin. Mark. Manag. 21 , 100643 (2021).
Duro, J. A., Perez-Laborda, A., Turrion-Prats, J. & Fernández-Fernández, M. Covid-19 and tourism vulnerability. Tour. Manag. Perspect. 38 , 100819 (2021).
Download references
Acknowledgements
The authors would like to thank the financial supports from the Xinjiang Major Science and Technology Projects (No. 2022A03002), the Xinjiang Social Science Foundation Projects (No. 2022VZJ028), Guangdong Basic and Applied Basic Research Foundation (2023A1515011273), Basic Research Program of Shenzhen (20220811173316001), and Specific Innovation Program of the department of Education of Guangdong Province (2023KTSCX315).
Author information
Authors and affiliations.
Urumqi Urban Institute of Geotechnical Investigation Surveying and Mapping, Urumqi, 830000, China
State Key Laboratory of Desert and Oasis Ecology, Xinjiang Institute of Ecology and Geography, Chinese Academy of Sciences, Urumqi, 830011, China
Xuankai Ma, Rongxi Ma, Cuirong Wang & Fang Han
Institute of Geographic Sciences and Natural Resources Research, Chinese Academy of Sciences, Beijing, No. 818, Beijing South Road, Urumqi, 100101, Xinjiang, China
Zhaoping Yang
University of Chinese Academy of Sciences, Beijing, 100049, China
Xuankai Ma, Rongxi Ma, Zhaoping Yang, Cuirong Wang & Fang Han
College of Geography and Remote Sensing Sciences, Xinjiang University, Urumqi, 800046, China
Xuankai Ma & Zijing Ma
School of Artificial Intelligence, Shenzhen Polytechnic University, Shenzhen, 518055, China
Jingzhe Wang
You can also search for this author in PubMed Google Scholar
Contributions
Xuankai MA conceived and designed the study, conducted the data analysis and visualization, and wrote the manuscript. Rongxi MA and Zijing MA provided revisions. Jingzhe WANG gave guidance to improve the results in revision. Zhaoping YANG, Cuirong WANG, Fang HAN provided project administration, supervision, validation. All authors reviewed and commented on the manuscript.
Corresponding author
Correspondence to Zhaoping Yang .
Ethics declarations
Competing interests.
The authors declare no competing interests.
Additional information
Publisher's note.
Springer Nature remains neutral with regard to jurisdictional claims in published maps and institutional affiliations.
Rights and permissions
Open Access This article is licensed under a Creative Commons Attribution 4.0 International License, which permits use, sharing, adaptation, distribution and reproduction in any medium or format, as long as you give appropriate credit to the original author(s) and the source, provide a link to the Creative Commons licence, and indicate if changes were made. The images or other third party material in this article are included in the article's Creative Commons licence, unless indicated otherwise in a credit line to the material. If material is not included in the article's Creative Commons licence and your intended use is not permitted by statutory regulation or exceeds the permitted use, you will need to obtain permission directly from the copyright holder. To view a copy of this licence, visit http://creativecommons.org/licenses/by/4.0/ .
Reprints and permissions
About this article
Cite this article.
Ma, X., Ma, R., Ma, Z. et al. Assessing the reactions of tourist markets to reinstated travel restrictions in the destination during the post-COVID-19 phase. Sci Rep 14 , 15495 (2024). https://doi.org/10.1038/s41598-024-66459-2
Download citation
Received : 07 November 2023
Accepted : 01 July 2024
Published : 05 July 2024
DOI : https://doi.org/10.1038/s41598-024-66459-2
Share this article
Anyone you share the following link with will be able to read this content:
Sorry, a shareable link is not currently available for this article.
Provided by the Springer Nature SharedIt content-sharing initiative
- Tourism demand
- Post-COVID-19
- Tourism crisis management
- Search engine data analysis
- Tourism resilience
By submitting a comment you agree to abide by our Terms and Community Guidelines . If you find something abusive or that does not comply with our terms or guidelines please flag it as inappropriate.
Quick links
- Explore articles by subject
- Guide to authors
- Editorial policies
Sign up for the Nature Briefing newsletter — what matters in science, free to your inbox daily.


IMAGES
VIDEO
COMMENTS
Abstract. This paper creates a Tourism Marketing Knowledge Grid and uses it as a framework for the. review. The grid reveals that extant tourism marketing research has primarily focused on. how ...
The Journal of Travel & Tourism Marketing® is a research journal that serves as a medium through which researchers and managers in the field of travel and tourism can exchange ideas and keep abreast with the latest developments in the field of tourism. JTTM welcomes submissions related to marketing management practices, applied research studies, critical reviews on major issues, development ...
This paper creates a Tourism Marketing Knowledge Grid and uses it as a framework for the review. The grid reveals that extant tourism marketing research has primarily focused on how service promises are made and kept, and has mostly generated frameworks to improve managerial decision making or provided insights about associations between constructs.
Some details are the followings: (1) Tourism marketing research is directly related to "tourism" and "marketing", and specifically, the category "hospitality, leisure, sport, tourism" has 530 articles, which is the most and accounts for 53.266 %, followed by "management" (225 papers, 22.613 %).
The purpose of this study was to uncover representative emergent areas and to examine the research area of marketing, tourism and big data (BD) to assess how these thematic areas have developed over a 27-year time period from 1996 to 2022. This study analyzed 1,152 studies to identify the principal thematic areas and emergent topics, principal ...
Marketing research plays a crucial role in tourism and hospitality marketing. It enables the marketer to conduct research into the marketing mix, to understand the market better, and to measure levels of consumer satisfaction. Furthermore, it minimises risk when managers make marketing management decisions.
Kaye Chon. This supplemental volume of JTTM covers a diverse range of topics relating to current issues in travel and tourism marketing. Since its first issue in 1992, JTTM has served an instrumental role in facilitating the exchange of knowledge in tourism research. Over the past 23 years, JTTM has seen several major changes in the way travel ...
At its core, tourism marketing is an intricate consumer-centered ballet, choreographed to the rhythm of consumers' evolving desires and anticipations. ... CiTUR—Centre for Tourism Research, Development and Innovation, Polytechnic of Leiria, 2520-641, Peniche, Portugal. Vasco Santos. ... Cite this paper.
TOURISM MARKETING RESEARCH: CURRENT ISSUES. This supplemental volume of JTTM covers a diverse range of topics relating to current issues in travel and tourism marketing. Since its first issue in 1992, JTTM has served an instrumental role in facilitating the exchange of knowledge in tourism research. Over the past 23 years, JTTM has seen several ...
Marketing of rural tourism - a study based on rural tourism lodgings in Portugal. L. Pato E. Kastenholz. Business, Geography. 2017. Purpose The purpose of this paper is to investigate the marketing actions developed for rural tourism lodgings and the effect of these actions on lodgings' performance. ….
Tourism has been characterized as a complex and globalized phenomenon, and one of the key characteristics of tourism research is its interdisciplinary nature (Connell 2012; Darbellay and Stock 2012). Based on this, marketing research in hospitality and tourism has been rising continuously for more than three decades (Yoo et al. 2011).
About tourism destination marketing research can be noted the papers of Perdue and Pitegoff (1990), Vukonic (1997), Muzaffer et al. (2011) and Ghiuta (2009). Regarding the valuable Bulgarian ...
The purpose of this paper is how the marketing development and changing the tourism industry in the world.Tourism marketing is the business discipline of attracting visitors to a specific location.
The approach taken in this paper is to assess the related literature and focus on the key points for future research on tourism marketing. We argue that destination marketing must lead to the optimization of the effects of tourism and the achievement of national tourism organizations' strategic goals.
This paper creates a Tourism Marketing Knowledge Grid and uses it as a framework for the review. The grid reveals that extant tourism marketing research has primarily focused on how service promises are made and kept, and has mostly generated frameworks to improve managerial decision making or provided insights about associations between constructs.
An application of geomarketing to coastal tourism areas. Geomarketing allows geographical units to be defined with a certain degree of homogeneity in terms of tourists' preferences, behaviours, needs, expectations, purchase and consumption patterns and analogous attitudes. The result is a... more. Download.
Notably, most of the previous research on the impact and roles of social media in tourism has focused only on particular aspects of the issue, such as word-of-mouth (Litvin et al., 2008), tourists' hotel-booking intentions (Sparks and Browning, 2011) and destination risk perceptions (Schroeder and Pennington-Gray, 2014).However, given that tourists consult different social media sites for ...
Several review papers on tourism research are now available and relevant to this study. ... 2019), tourism marketing (Mwinuka, 2017), tourism and education (Goh and King, ...
The review of tourism journal publications, O'Leary et al. (2004) stated that the tourism marketing research is one of most studied topic, other most studied topic found as per the report were management, motivation etc. It is evident that tourism marketing is most focused and important topic in tourism research.
This study concludes that destination research is a critical branch of tourism research because it considers multiple stakeholder groups, discusses macro-level governance issues, meso-organizational, and micro-level tourist perception and behavior, and analyzes destination resources and marketing to ensure a sustainable and smart future.
adopted for use in marketing tourism, their use in tourism is expected only to gain momentum. The purpose of this paper is to systematically review literature published in tourism-relate. journals on digital marketing tools in tourism from 2016-2020, and to discuss future trends. The analysis reveals that the incre.
Theoretical papers, essays and editorials. Research exploring the role of marketing in other disciplines rather than in tourism ... academic research focusing on the tourism marketing role in facilitating the sustainability of the destination in general (Higham & Moyle, 2016:170). Tourist destination quality is the key
Travel and tourism marketing as reflected by papers published in the Journal of Travel and Tourism Marketing surveyed by Hu (1996) in the periods 1992 - 1995 identified five main topics: 1. Economic psychology incl. consumer behaviour, choice modelling and decision-making and service quality and satisfaction. 2.
research on tourism marketing. We argue that destination marketing must lead to the optimization of the effects of tourism and the achievement of national tourism organizations' strategic goals. This paper contributes to marketing professionals and academics, pointing out the uniqueness of tourism marketing.
This paper utilizes daily keyword indices from 2011 to 2022 to investigate the background of tourism demand for the Duku Road and brand indexes from August 10 to October 31 in 2021 and 2022 to ...
The article examines the offers of marketing digital tools developed as a part of startup projects for participants in the tourism and travel industry, taking into account current tendencies and trends in the development of the tourism, hospitality and leisure industries. Startups offer both pioneering ideas, business models and technologies that can fundamentally change and influence the ...
The authors confirm contribution to the paper as follows: Study conception and design - Paul Blaise Issock Issock, Abby Jacobs ... Backman, K. F., Backman, S. J., & Chang, L. L. (2016). Exploring the implications of virtual reality technology in tourism marketing: An integrated research framework. International Journal of Tourism Research, 18 ...